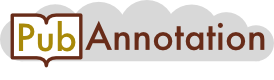
Projects
Name | T | Description | # Ann. | Author | Maintainer | Updated_at | Status | |
---|---|---|---|---|---|---|---|---|
61-80 / 593 show all | ||||||||
MENA-example2 | 3 | Jin-Dong Kim | 2023-11-24 | Testing | ||||
tees-test | 3.39 K | Nico Colic | Nico Colic | 2023-11-24 | Developing | |||
sonoma | 19.3 K | Standigm | 2023-11-24 | Testing | ||||
OryzaGP_2022 | 41.3 K | larmande | 2023-11-24 | |||||
LitCoin-training-merged | 14.8 K | Jin-Dong Kim | 2023-11-24 | |||||
bionlp-ost-19-BB-rel-ner-test | 125 | ldeleger | 2023-11-24 | Developing | ||||
genia-medco-coref | 45.9 K | MedCo project & Genia project | Jin-Dong Kim | 2023-11-24 | Developing | |||
Trait curation | 479 | Sachiko Shirasawa | Sachiko Shirasawa | 2023-11-24 | Testing | |||
tmVarCorpus | 1.43 K | Chih-Hsuan Wei , Bethany R. Harris , Hung-Yu Kao and Zhiyong Lu | Chih-Hsuan Wei | 2023-11-24 | Released | |||
CoMAGC | 1.53 K | Lee et al | Hee-Jin Lee | 2023-11-24 | Released | |||
LitCoin-GeneOrGeneProduct-v3 | 4.67 K | Jin-Dong Kim | 2023-11-24 | |||||
Covid19_manual_annotation_v2 | 4.58 K | AikoHIRAKI | 2023-11-24 | Developing | ||||
proj_h_1 | 6.7 K | 2023-11-24 | ||||||
DisGeNET5_variant_disease | 144 K | IBI Group | Yue Wang | 2023-11-24 | Released | |||
PubMed-German-test | 0 | Jin-Dong Kim | 2023-11-24 | Developing | ||||
PT_NER_NEL_Diana | 318 | dpavot | 2023-11-24 | Developing | ||||
OryzaGP | 29.1 K | Huy Do and Pierre Larmande | Yue Wang | 2023-11-24 | Uploading | |||
PubMed-2017 | 0 | Jin-Dong Kim | 2023-11-24 | Developing | ||||
jnlpba-st-training | 51.1 K | GENIA | Yue Wang | 2023-11-26 | Released | |||
Oncogenesis | 0 | Sophie Nam | 2023-11-26 |
Name | T | # Ann. | Author | Maintainer | Updated_at | Status | |
---|---|---|---|---|---|---|---|
61-80 / 593 show all | |||||||
MENA-example2 | 3 | Jin-Dong Kim | 2023-11-24 | Testing | |||
tees-test | 3.39 K | Nico Colic | Nico Colic | 2023-11-24 | Developing | ||
sonoma | 19.3 K | Standigm | 2023-11-24 | Testing | |||
OryzaGP_2022 | 41.3 K | larmande | 2023-11-24 | ||||
LitCoin-training-merged | 14.8 K | Jin-Dong Kim | 2023-11-24 | ||||
bionlp-ost-19-BB-rel-ner-test | 125 | ldeleger | 2023-11-24 | Developing | |||
genia-medco-coref | 45.9 K | MedCo project & Genia project | Jin-Dong Kim | 2023-11-24 | Developing | ||
Trait curation | 479 | Sachiko Shirasawa | Sachiko Shirasawa | 2023-11-24 | Testing | ||
tmVarCorpus | 1.43 K | Chih-Hsuan Wei , Bethany R. Harris , Hung-Yu Kao and Zhiyong Lu | Chih-Hsuan Wei | 2023-11-24 | Released | ||
CoMAGC | 1.53 K | Lee et al | Hee-Jin Lee | 2023-11-24 | Released | ||
LitCoin-GeneOrGeneProduct-v3 | 4.67 K | Jin-Dong Kim | 2023-11-24 | ||||
Covid19_manual_annotation_v2 | 4.58 K | AikoHIRAKI | 2023-11-24 | Developing | |||
proj_h_1 | 6.7 K | 2023-11-24 | |||||
DisGeNET5_variant_disease | 144 K | IBI Group | Yue Wang | 2023-11-24 | Released | ||
PubMed-German-test | 0 | Jin-Dong Kim | 2023-11-24 | Developing | |||
PT_NER_NEL_Diana | 318 | dpavot | 2023-11-24 | Developing | |||
OryzaGP | 29.1 K | Huy Do and Pierre Larmande | Yue Wang | 2023-11-24 | Uploading | ||
PubMed-2017 | 0 | Jin-Dong Kim | 2023-11-24 | Developing | |||
jnlpba-st-training | 51.1 K | GENIA | Yue Wang | 2023-11-26 | Released | ||
Oncogenesis | 0 | Sophie Nam | 2023-11-26 |