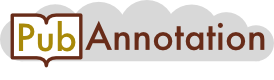
PubMed:39278954 10 Projects
Integrating meta-heuristic with named data networking for secure edge computing in IoT enabled healthcare monitoring system.
The advancement in technology, with the "Internet of Things (IoT) is continuing a crucial task to accomplish distance medical care observation, where the effective and secure healthcare information retrieval is complex. However, the IoT systems have restricted resources hence it is complex to attain effective and secure healthcare information acquisition. The idea of smart healthcare has developed in diverse regions, where small-scale implementations of medical facilities are evaluated. In the IoT-aided medical devices, the security of the IoT systems and related information is highly essential on the other hand, the edge computing is a significant framework that rectifies their processing and computational issues. The edge computing is inexpensive, and it is a powerful framework to offer low latency information assistance by enhancing the computation and the transmission speed of the IoT systems in the medical sectors. The main intention of this work is to design a secure framework for Edge computing in IoT-enabled healthcare systems using heuristic-based authentication and "Named Data Networking (NDN)". There are three layers in the proposed model. In the first layer, many IoT devices are connected together, and using the cluster head formation, the patients are transmitting their data to the edge cloud layer. The edge cloud layer is responsible for storage and computing resources for rapidly caching and providing medical data. Hence, the patient layer is a new heuristic-based sanitization algorithm called Revised Position of Cat Swarm Optimization (RPCSO) with NDN for hiding the sensitive data that should not be leaked to unauthorized users. This authentication procedure is adopted as a multi-objective function key generation procedure considering constraints like hiding failure rate, information preservation rate, and degree of modification. Further, the data from the edge cloud layer is transferred to the user layer, where the optimal key generation with NDN-based restoration is adopted, thus achieving efficient and secure medical data retrieval. The framework is evaluated quantitatively on diverse healthcare datasets from University of California (UCI) and Kaggle repository and experimental analysis shows the superior performance of the proposed model in terms of latency and cost when compared to existing solutions. The proposed model performs the comparative analysis of the existing algorithms such as Cat Swarm Optimization (CSO), Osprey Optimization Algorithm (OOA), Mexican Axolotl Optimization (MAO), Single candidate optimizer (SCO). Similarly, the cryptography tasks like "Rivest-Shamir-Adleman (RSA), Advanced Encryption Standard (AES), Elliptic Curve Cryptography (ECC), and Data sanitization and Restoration (DSR) are applied and compared with the RPCSO in the proposed work. The results of the proposed model is compared on the basis of the best, worst, mean, median and standard deviation. The proposed RPCSO outperforms all other models with values of 0.018069361, 0.50564046, 0.112643119, 0.018069361, 0.156968355 and 0.283597992, 0.467442652, 0.32920734, 0.328581887, 0.063687386 for both dataset 1 and dataset 2 respectively.
|
Annnotations
last updated at 2024-12-01 08:00:22 UTC
- Denotations: 0
- Blocks: 0
- Relations: 0