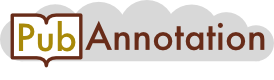
PubMed:37059219 13 Projects
Using ATCLSTM-Kcr to predict and generate the human lysine crotonylation database.
Lysine crotonylation (Kcr) is an evolutionarily conserved protein post-translational modifications, which plays an important role in cellular physiology and pathology, such as chromatin remodeling, gene transcription regulation, telomere maintenance, inflammation, and cancer. Tandem mass spectrometry (LC-MS/MS) has been used to identify the global Kcr profiling of human, at the same time, many computing methods have been developed to predict Kcr sites without high experiment cost. Deep learning network solves the problem of manual feature design and selection in traditional machine learning (NLP), especially the algorithms in natural language processing which treated peptides as sentences, thus can extract more in-depth information and obtain higher accuracy. In this work, we establish a Kcr prediction model named ATCLSTM-Kcr which use self-attention mechanism combined with NLP method to highlight the important features and further capture the internal correlation of the features, to realize the feature enhancement and noise reduction modules of the model. Independent tests have proved that ATCLSTM-Kcr has better accuracy and robustness than similar prediction tools. Then, we design pipeline to generate MS-based benchmark dataset to avoid the false negatives caused by MS-detectability and improve the sensitivity of Kcr prediction. Finally, we develop a Human Lysine Crotonylation Database (HLCD) which using ATCLSTM-Kcr and the two representative deep learning models to score all lysine sites of human proteome, and annotate all Kcr sites identified by MS of current published literatures. HLCD provides an integrated platform for human Kcr sites prediction and screening through multiple prediction scores and conditions, and can be accessed on the website:www.urimarker.com/HLCD/. SIGNIFICANCE: Lysine crotonylation (Kcr) plays an important role in cellular physiology and pathology, such as chromatin remodeling, gene transcription regulation and cancer. To better elucidate the molecular mechanisms of crotonylation and reduce the high experimental cost, we establish a deep learning Kcr prediction model and solve the problem of false negatives caused by the detectability of mass spectrometry (MS). Finally, we develop a Human Lysine Crotonylation Database to score all lysine sites of human proteome, and annotate all Kcr sites identified by MS of current published literatures. Our work provides a convenient platform for human Kcr sites prediction and screening through multiple prediction scores and conditions.
|
Annnotations
- Denotations: 0
- Blocks: 0
- Relations: 0