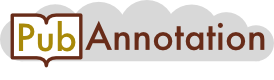
PubMed:24401455
Chemometrics-assisted simultaneous voltammetric determination of ascorbic acid, uric acid, dopamine and nitrite: application of non-bilinear voltammetric data for exploiting first-order advantage.
For the first time, several multivariate calibration (MVC) models including partial least squares-1 (PLS-1), continuum power regression (CPR), multiple linear regression-successive projections algorithm (MLR-SPA), robust continuum regression (RCR), partial robust M-regression (PRM), polynomial-PLS (PLY-PLS), spline-PLS (SPL-PLS), radial basis function-PLS (RBF-PLS), least squares-support vector machines (LS-SVM), wavelet transform-artificial neural network (WT-ANN), discrete wavelet transform-ANN (DWT-ANN), and back propagation-ANN (BP-ANN) have been constructed on the basis of non-bilinear first order square wave voltammetric (SWV) data for the simultaneous determination of ascorbic acid (AA), uric acid (UA), dopamine (DP) and nitrite (NT) at a glassy carbon electrode (GCE) to identify which technique offers the best predictions. The compositions of the calibration mixtures were selected according to a simplex lattice design (SLD) and validated with an external set of analytes' mixtures. An asymmetric least squares splines regression (AsLSSR) algorithm was applied for correcting the baselines. A correlation optimized warping (COW) algorithm was used to data alignment and lack of bilinearity was tackled by potential shift correction. The effects of several pre-processing techniques such as genetic algorithm (GA), orthogonal signal correction (OSC), mean centering (MC), robust median centering (RMC), wavelet denoising (WD), and Savitsky-Golay smoothing (SGS) on the predictive ability of the mentioned MVC models were examined. The best preprocessing technique was found for each model. According to the results obtained, the RBF-PLS was recommended to simultaneously assay the concentrations of AA, UA, DP and NT in human serum samples.
|
Annnotations
- Denotations: 56
- Blocks: 0
- Relations: 28