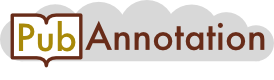
PMC:7402624 / 44085-45165
Annnotations
LitCovid-PD-FMA-UBERON
{"project":"LitCovid-PD-FMA-UBERON","denotations":[{"id":"T391","span":{"begin":391,"end":395},"obj":"Body_part"},{"id":"T392","span":{"begin":692,"end":696},"obj":"Body_part"},{"id":"T393","span":{"begin":742,"end":746},"obj":"Body_part"},{"id":"T394","span":{"begin":754,"end":758},"obj":"Body_part"},{"id":"T395","span":{"begin":765,"end":769},"obj":"Body_part"},{"id":"T396","span":{"begin":977,"end":982},"obj":"Body_part"},{"id":"T397","span":{"begin":1007,"end":1011},"obj":"Body_part"}],"attributes":[{"id":"A391","pred":"fma_id","subj":"T391","obj":"http://purl.org/sig/ont/fma/fma68646"},{"id":"A392","pred":"fma_id","subj":"T392","obj":"http://purl.org/sig/ont/fma/fma68646"},{"id":"A393","pred":"fma_id","subj":"T393","obj":"http://purl.org/sig/ont/fma/fma68646"},{"id":"A394","pred":"fma_id","subj":"T394","obj":"http://purl.org/sig/ont/fma/fma68646"},{"id":"A395","pred":"fma_id","subj":"T395","obj":"http://purl.org/sig/ont/fma/fma68646"},{"id":"A396","pred":"fma_id","subj":"T396","obj":"http://purl.org/sig/ont/fma/fma68646"},{"id":"A397","pred":"fma_id","subj":"T397","obj":"http://purl.org/sig/ont/fma/fma68646"}],"text":"There were two challenges with extracting meaning from these data. First, there was considerable inter-patient heterogeneity for each of these immune features related to disease severity score. Second, these binary comparisons (e.g., one immune subset versus one clinical feature) vastly underutilized the high dimensional information in this dataset. Thus, we next visualized major T and B cell subpopulation data as it related to clinical disease severity score (Fig. 6C). Data were clustered based on immune features and then overlaid with the disease severity score over time for each patient. This analysis revealed groups of patients with similar composite immune signatures of T and B cell populations (Fig. 6C). When individual CD8 T cell, CD4 T cell, or B cell populations were examined, a similar concept of patient subgroups emerged (fig. S7, F, G, and H). These data suggested the idea of “immunotypes” of COVID-19 patients based on integrated responses of T and B cells, though some individual cell types and/or phenotypes separated patients more clearly than others."}
LitCovid-PubTator
{"project":"LitCovid-PubTator","denotations":[{"id":"1521","span":{"begin":736,"end":739},"obj":"Gene"},{"id":"1522","span":{"begin":748,"end":751},"obj":"Gene"},{"id":"1523","span":{"begin":103,"end":110},"obj":"Species"},{"id":"1524","span":{"begin":589,"end":596},"obj":"Species"},{"id":"1525","span":{"begin":631,"end":639},"obj":"Species"},{"id":"1526","span":{"begin":818,"end":825},"obj":"Species"},{"id":"1527","span":{"begin":927,"end":935},"obj":"Species"},{"id":"1528","span":{"begin":1046,"end":1054},"obj":"Species"},{"id":"1529","span":{"begin":918,"end":926},"obj":"Disease"}],"attributes":[{"id":"A1521","pred":"tao:has_database_id","subj":"1521","obj":"Gene:925"},{"id":"A1522","pred":"tao:has_database_id","subj":"1522","obj":"Gene:920"},{"id":"A1523","pred":"tao:has_database_id","subj":"1523","obj":"Tax:9606"},{"id":"A1524","pred":"tao:has_database_id","subj":"1524","obj":"Tax:9606"},{"id":"A1525","pred":"tao:has_database_id","subj":"1525","obj":"Tax:9606"},{"id":"A1526","pred":"tao:has_database_id","subj":"1526","obj":"Tax:9606"},{"id":"A1527","pred":"tao:has_database_id","subj":"1527","obj":"Tax:9606"},{"id":"A1528","pred":"tao:has_database_id","subj":"1528","obj":"Tax:9606"},{"id":"A1529","pred":"tao:has_database_id","subj":"1529","obj":"MESH:C000657245"}],"namespaces":[{"prefix":"Tax","uri":"https://www.ncbi.nlm.nih.gov/taxonomy/"},{"prefix":"MESH","uri":"https://id.nlm.nih.gov/mesh/"},{"prefix":"Gene","uri":"https://www.ncbi.nlm.nih.gov/gene/"},{"prefix":"CVCL","uri":"https://web.expasy.org/cellosaurus/CVCL_"}],"text":"There were two challenges with extracting meaning from these data. First, there was considerable inter-patient heterogeneity for each of these immune features related to disease severity score. Second, these binary comparisons (e.g., one immune subset versus one clinical feature) vastly underutilized the high dimensional information in this dataset. Thus, we next visualized major T and B cell subpopulation data as it related to clinical disease severity score (Fig. 6C). Data were clustered based on immune features and then overlaid with the disease severity score over time for each patient. This analysis revealed groups of patients with similar composite immune signatures of T and B cell populations (Fig. 6C). When individual CD8 T cell, CD4 T cell, or B cell populations were examined, a similar concept of patient subgroups emerged (fig. S7, F, G, and H). These data suggested the idea of “immunotypes” of COVID-19 patients based on integrated responses of T and B cells, though some individual cell types and/or phenotypes separated patients more clearly than others."}
LitCovid-PD-MONDO
{"project":"LitCovid-PD-MONDO","denotations":[{"id":"T325","span":{"begin":918,"end":926},"obj":"Disease"}],"attributes":[{"id":"A325","pred":"mondo_id","subj":"T325","obj":"http://purl.obolibrary.org/obo/MONDO_0100096"}],"text":"There were two challenges with extracting meaning from these data. First, there was considerable inter-patient heterogeneity for each of these immune features related to disease severity score. Second, these binary comparisons (e.g., one immune subset versus one clinical feature) vastly underutilized the high dimensional information in this dataset. Thus, we next visualized major T and B cell subpopulation data as it related to clinical disease severity score (Fig. 6C). Data were clustered based on immune features and then overlaid with the disease severity score over time for each patient. This analysis revealed groups of patients with similar composite immune signatures of T and B cell populations (Fig. 6C). When individual CD8 T cell, CD4 T cell, or B cell populations were examined, a similar concept of patient subgroups emerged (fig. S7, F, G, and H). These data suggested the idea of “immunotypes” of COVID-19 patients based on integrated responses of T and B cells, though some individual cell types and/or phenotypes separated patients more clearly than others."}
LitCovid-PD-CLO
{"project":"LitCovid-PD-CLO","denotations":[{"id":"T713","span":{"begin":389,"end":395},"obj":"http://purl.obolibrary.org/obo/CL_0000236"},{"id":"T714","span":{"begin":690,"end":696},"obj":"http://purl.obolibrary.org/obo/CL_0000236"},{"id":"T715","span":{"begin":736,"end":739},"obj":"http://purl.obolibrary.org/obo/CLO_0053438"},{"id":"T716","span":{"begin":740,"end":746},"obj":"http://purl.obolibrary.org/obo/CL_0000084"},{"id":"T717","span":{"begin":748,"end":751},"obj":"http://purl.obolibrary.org/obo/PR_000001004"},{"id":"T718","span":{"begin":752,"end":758},"obj":"http://purl.obolibrary.org/obo/CL_0000084"},{"id":"T719","span":{"begin":763,"end":769},"obj":"http://purl.obolibrary.org/obo/CL_0000236"},{"id":"T720","span":{"begin":797,"end":798},"obj":"http://purl.obolibrary.org/obo/CLO_0001020"},{"id":"T721","span":{"begin":975,"end":982},"obj":"http://purl.obolibrary.org/obo/CL_0000236"},{"id":"T722","span":{"begin":1007,"end":1017},"obj":"http://purl.obolibrary.org/obo/CL_0000000"}],"text":"There were two challenges with extracting meaning from these data. First, there was considerable inter-patient heterogeneity for each of these immune features related to disease severity score. Second, these binary comparisons (e.g., one immune subset versus one clinical feature) vastly underutilized the high dimensional information in this dataset. Thus, we next visualized major T and B cell subpopulation data as it related to clinical disease severity score (Fig. 6C). Data were clustered based on immune features and then overlaid with the disease severity score over time for each patient. This analysis revealed groups of patients with similar composite immune signatures of T and B cell populations (Fig. 6C). When individual CD8 T cell, CD4 T cell, or B cell populations were examined, a similar concept of patient subgroups emerged (fig. S7, F, G, and H). These data suggested the idea of “immunotypes” of COVID-19 patients based on integrated responses of T and B cells, though some individual cell types and/or phenotypes separated patients more clearly than others."}
LitCovid-PD-CHEBI
{"project":"LitCovid-PD-CHEBI","denotations":[{"id":"T303","span":{"begin":470,"end":472},"obj":"Chemical"},{"id":"T304","span":{"begin":715,"end":717},"obj":"Chemical"}],"attributes":[{"id":"A303","pred":"chebi_id","subj":"T303","obj":"http://purl.obolibrary.org/obo/CHEBI_27594"},{"id":"A304","pred":"chebi_id","subj":"T304","obj":"http://purl.obolibrary.org/obo/CHEBI_27594"}],"text":"There were two challenges with extracting meaning from these data. First, there was considerable inter-patient heterogeneity for each of these immune features related to disease severity score. Second, these binary comparisons (e.g., one immune subset versus one clinical feature) vastly underutilized the high dimensional information in this dataset. Thus, we next visualized major T and B cell subpopulation data as it related to clinical disease severity score (Fig. 6C). Data were clustered based on immune features and then overlaid with the disease severity score over time for each patient. This analysis revealed groups of patients with similar composite immune signatures of T and B cell populations (Fig. 6C). When individual CD8 T cell, CD4 T cell, or B cell populations were examined, a similar concept of patient subgroups emerged (fig. S7, F, G, and H). These data suggested the idea of “immunotypes” of COVID-19 patients based on integrated responses of T and B cells, though some individual cell types and/or phenotypes separated patients more clearly than others."}
LitCovid-sentences
{"project":"LitCovid-sentences","denotations":[{"id":"T251","span":{"begin":0,"end":66},"obj":"Sentence"},{"id":"T252","span":{"begin":67,"end":193},"obj":"Sentence"},{"id":"T253","span":{"begin":194,"end":351},"obj":"Sentence"},{"id":"T254","span":{"begin":352,"end":474},"obj":"Sentence"},{"id":"T255","span":{"begin":475,"end":597},"obj":"Sentence"},{"id":"T256","span":{"begin":598,"end":719},"obj":"Sentence"},{"id":"T257","span":{"begin":720,"end":867},"obj":"Sentence"},{"id":"T258","span":{"begin":868,"end":1080},"obj":"Sentence"}],"namespaces":[{"prefix":"_base","uri":"http://pubannotation.org/ontology/tao.owl#"}],"text":"There were two challenges with extracting meaning from these data. First, there was considerable inter-patient heterogeneity for each of these immune features related to disease severity score. Second, these binary comparisons (e.g., one immune subset versus one clinical feature) vastly underutilized the high dimensional information in this dataset. Thus, we next visualized major T and B cell subpopulation data as it related to clinical disease severity score (Fig. 6C). Data were clustered based on immune features and then overlaid with the disease severity score over time for each patient. This analysis revealed groups of patients with similar composite immune signatures of T and B cell populations (Fig. 6C). When individual CD8 T cell, CD4 T cell, or B cell populations were examined, a similar concept of patient subgroups emerged (fig. S7, F, G, and H). These data suggested the idea of “immunotypes” of COVID-19 patients based on integrated responses of T and B cells, though some individual cell types and/or phenotypes separated patients more clearly than others."}