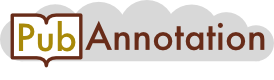
CORD-19:12af471f81b05440047e30b963b3733bd6f33693 / 0-236 3 Projects
Mechanistic and topological explanations in medicine: the case of medical genetics and network medicine
Abstract
Medical explanations have often been thought on the model of biological ones and are frequently defined as mechanistic explanations of a biological dysfunction. In this paper, I argue that topological explanations, which have been described in ecology or in cognitive sciences, can also be found in medicine and I discuss the relationships between mechanistic and topological explanations in medicine, through the example of network medicine and medical genetics. Network medicine is a recent discipline that relies on the analysis of various disease networks (including diseasegene networks) in order to find organizing principles in disease explanation. My aim is to show how topological explanations in network medicine can help solving the conceptual issues that pure mechanistic explanations of the genetics of disease are currently facing, namely the crisis of the concept of genetic disease, the progressive geneticization of diseases and the dissolution of the distinction between monogenic and polygenic diseases. However, I will also argue that topological explanations should not be considered as independent and radically different from mechanistic explanations for at least two reasons. First, in network medicine, topological explanations depend on and use mechanistic information. Second, they leave out some missing gaps in disease explanation that require, in turn, the development of new mechanistic explanations. Finally, I will insist on the specific contribution of topological explanations in medicine: they push us to develop an explanation of disease in general, instead of focusing on single explanations of individual diseases. This last point may have major consequences for biomedical research.
B Marie Darrason marie.darrason@gmail.com Keywords Philosophy of science · Philosophy of medicine · Mechanistic explanation · Topological explanation · Network medicine · Genetic disease · Geneticization
While most biomedical explanations have been considered to be mechanistic, some philosophers have recently pointed out the existence of more abstract or ideal types of explanations, such as topological explanations. This gave rise to a hot debate in philosophy of science revolving around whether topological explanations are real explanations or mere descriptions of biomedical phenomena and about the way mechanistic and topological explanations relate to each other.
My aim in this paper is to contribute to this debate by focusing on the case study of medical genetics and network medicine. Indeed, network medicine is a new discipline that relies on topological explanations to answer some research questions that traditional mechanistic explanations of medical genetics are currently struggling with. By focusing on this example, I aim at defending three claims. First, there are topological explanations in medicine whose impact on our understanding of disease in terms of robustness and functional redundancy is crucial. Second, topological explanations and mechanistic explanations do constitute two distinct explanatory types, since they do not explain the same phenomenon in virtue of the same properties (topological properties vs. material properties). However, they are not completely independent from each other: while pure mechanistic and pure topological explanations may exist, topological explanations often rely on mechanisms and raise new issues that, in turn, require new mechanistic explanations. Third, I want to emphasize that in the case of medicine and medical genetics, the specific contribution of topological explanations is to foster a general explanation of disease and of the role of genes in disease, as opposed to pure mechanistic explanations that tend to focus on detailed explanations of the genetics of individual diseases.
Since Bechtel and Richardson's book «Discovering complexity» (Bechtel and Richardson 1993) , there has been a strong focus on mechanisms and on mechanistic explanations in biology (Bechtel and Abrahamsen 2005; Craver 2006; Glennan 2005; Machamer et al. 2000; Machamer 2004; Woodward 2013) . In this neo-mechanistic trend, several philosophers distinguish between different concepts of mechanisms (Kuorikoski 2009; Nicholson 2012) or between different theses about why we need mechanistic explanations (Levy 2013) . Still, some core ideas at the root of this concept can be spelled out: giving a mechanistic explanation of a phenomenon implies to identify the mechanism in virtue of which the given phenomenon is produced. Identifying a mechanism thus implies to decompose a physical system, to individuate its components, including both its "parts" (also called "entities") and its "activities" (also called "operations"), and finally to describe the relationships between its components, namely its overall organization. It is the way these entities and activities are organized in a continuous and temporal process in order to produce "regular changes" that gives explanatory power to the mechanistic explanation of a phenomenon. Some philosophers have heavily stressed concreteness and completeness as major features of a good mechanistic explanation: according to them, the more detailed, the more fine-grained a mechanism is, the more explanatory it is of the exhibited phenomenon (Craver 2006; Kaplan and Craver 2011) . However, according to others, sometimes it appears that "less is more" and that abstracting away from the structural specifics of a mechanism is actually quite useful to understand its overall organization (Levy and Bechtel 2013) . Since medical explanations have been often thought on the model of biological ones, this neo-mechanistic trend in philosophy of biology has progressively invaded philosophy of medicine. For example, Paul Thagard explicitly refers in his 2006 article to the Machamer Darden and Craver characterization of mechanisms (Machamer et al. 2000) and defines medical explanations as "the representation, […] , of mechanisms whose proper and improper functioning generate the states and symptoms of a disease" (Thagard 2006, p. 59) . In this view, disease is thought as the product of broken/dysfunctional/altered biological mechanisms. Thagard takes the example of the SARS coronavirus. It is possible to describe the mechanism of SARS infection by identifying the parts and the activities of the virus and of the host. It is the way these different parts and activities are organized in a continuous spatial-temporal process that allows the SARS coronavirus to infect the host cell, then to generate and cause SARS symptoms. Of course, in the same way that there are many differences between mechanistic accounts in philosophy of biology, there are several disputes over what is a disease mechanism and whether diseases mechanisms should be viewed as fundamentally different from physiological mechanisms or not (on this controvery, see Moghaddam-Taaheri 2011; Nervi 2010) . Nonetheless, most medical explanations are considered mechanistic explanations: in order to explain a disease, you need to localize and decompose the mechanism that produces the disease symptoms.
While mechanistic explanation are pervasive in biology and medicine, some authors (philosophers as well as scientists) have recently insisted on the existence of more abstract, mathematical or ideal types of explanations (Batterman 2010; Brigandt 2013; Huneman 2010) in biology, ecology (Montoya et al. 2006) or neurosciences (Bullmore and Sporns 2009; Sporns 2012) . I will focus here on topological explanations that Philippe Huneman defines as "a kind of explanation that abstracts away from causal relationships and interactions in a system, in order to pick up some sort of "topological" properties of that system and draw from those properties mathematical consequences that explain the features of the system they target" (Huneman 2010, p. 214) . In order to provide a topological explanation for a given system, the system shall first be represented in an idealized space (usually, a graph or a network) where the parts of the system are represented as nodes. It is then possible to use graph-theoretical concepts such as hubs, modules, motifs or coefficient clusters to derive topological properties from the location of the parts in the space and from the way the nodes are linked together. To illustrate this definition, Huneman takes the example of an ecological community S, composed of various species (A, B, C, D), tied together by many different kinds of relationships, including predation relationships. If you want to explain how this given ecological community behaves and what happens to the system when one species (let's say species B) goes extinct, you may give a mechanistic explanation, based on the physical properties of the parts of the system (i.e. the organisms) and on the activities (i.e. the predation relationship, for instance) in order to explain how the disappearance of the species B affects the ecological community as a whole. Such an explanation would constitute of a linear and organized sequence of causal-mechanistic interactions: "Species B usually preys on species C that preys on species D. In the absence of species B, species C will multiply and prey both species D and species A, etc." However, another way to understand the behavior of the ecological community S when species B goes extinct is to choose one relevant mechanistic relationship between the species of your ecological community, for example, the predation-relationship and to represent it on a graph S', each species being a node and two nodes being connected by an oriented edge if one species prey on the other one. Now if species B is connected to many other species by a predation relationship and if you remove it from your network (removal corresponding to extinction), it is easy to understand that this is going to affect your global network (the ecological community) in a different way than if species B was loosely connected to the whole network. In doing so, you explain the behavior of the ecological community not in virtue of the material and physical properties of the ecological community, but in virtue of the topological properties of the ecological community, once it has been represented as an abstract system, once that its parts (the species) and its activities (the predation relationships) have been stripped of any materiality.
To be perfectly clear, let's specify that material properties and topological properties are not merely distinct but completely different kinds of properties. Material properties are directly related to the physical and concrete properties of an object. In my example, the fact that species B preys on species C depends on many properties, some of which being the material properties of the individuals of species B, such as having sharp canines for example. Material properties of an object are somewhere independent from the interactions of a given object with the system in which you consider it. Let's say that species B preys on species C, but that species C goes extinct. Whether species B finds another species to prey on or whether species B also goes extinct, it will not change the material properties according to which species B has sharp canines and is usually a predator of species C. On the contrary, topological properties of a given object are derived from its spatial relationships with the other parts of a system. It is not a property constituent of a given object, but a property that concerns "how, to put it vaguely, it fills the space; how parts of the system are located regarding one another and whether those relations can still hold under some continuous deformations of the system (and which ones)" (Huneman 2010, p. 214) . Here, the term "space" refers to the technical, abstract and mathematical notion of space used in graph theory and not to the vulgar notion of physical space. Thus, topological properties of a system have nothing to do with physical distances, but with the ability of the system and its parts to resist some types of spatial perturbations (such as removing a highly connected species in an ecological network).
When put like this, the contrast between mechanistic and topological explanations seems quite obvious. First, whereas mechanistic explanations consist in breaking down a system into entities and activities in order to consider the causal relationships that are responsible for the production of regular changes in this system, topological expla-nations abstract away from the physical and material features of its parts and rely on the topological properties of a system, i.e, on the location that these parts occupy in a given space (i.e, in our example, species B being a hub (highly-connected node). Second, while mechanistic explanations are firmly ground in temporal conditions, topological explanations may be (and usually are) completely independent of them. 1 Third, instead of explaining the causal mechanistic interactions between the parts of the system, topological properties provide an explanation for the robustness of a system against different perturbations (how does the system react to the extinction of species B versus the extinction of species C for example).
However, in spite of theses apparently clear-cut distinct features, the status of topological explanations in biomedical sciences and the extent to which they actually differ from mechanistic explanations has became a hot topic in philosophy of science, for at least three reasons. First, some philosophers, such as Kaplan and Craver, claimed that there are no explanations in biomedical sciences other than mechanistic explanations (Kaplan and Craver 2011) . In this view, other types of explanations can either be considered as extensions of mechanistic explanations or should be denied the status of "explanations" and be only considered as mere descriptions of a phenomenon.
Second, even the proponents of the existence of topological explanations (Huneman 2010; Silberstein and Chemero 2013; Woodward 2013) claim that there is no dichotomy between topological explanations and mechanistic ones. They defend the existence of a continuum between these two types of explanations, going from pure mechanistic explanations to pure topological explanations. Indeed, topological explanations frequently build on mechanistic information and usually entail that some causal mechanistic interactions of the system have been considered explanatorily relevant enough to enter the network. If we take back the example of the ecological community, it is true that what explains the behavior of the system in the absence of B is the fact that B is a major hub (highly connected node in the network). Nonetheless, to build such a network implies a choice between what would count as explanatory relevant relationships, i.e., in this case, predation relationships. According to Huneman, it is thus possible to define a continuum between pure topological explanations, "when all the relations are explanatorily equivalent and enter into S' as nodes, vertices, points or sides" and pure mechanistic explanations when "all differences between causal interactions are relevant" (Huneman 2010, p. 225) .
As a consequence of this continuum, these philosophers do not necessarily consider topological and mechanistic explanations as competing or mutually exclusive from one another, but rather as complementary explanations of the same phenomenon. So, in this view, the debate should not be about whether we should choose between a mechanistic and a topological explanation of a given biomedical phenomenon, but whether we need both types of explanations to explain the same phenomenon, depending on which features we are the most interested in Brigandt (2013) , Woodward (2013) .
Finally, another reason why the debate is so complicated and threatens to be a mere "semantic" one is that, as I mentioned at the beginning of this paper, there are many ways to define mechanisms and there seems to co-exist today at least one strict definition of mechanism and a broader one. 2 Following this liberalization of the concept of mechanism, it became obvious that the more one might want to defend a strong and strict concept of mechanism, the more topological explanations and mechanistic explanations may be seen as two radically different ways of explaining a phenomenon, while the more liberal one might be with the concept of mechanism and the easier it would be to consider that mechanistic explanations can somewhat encompass topological analyses. It is precisely in these terms that Woodward analyses the controversy between Craver, Kaplan and Bechtel over what should be considered mechanistic explanations and what should be considered topological ones. 3 To sum it up, the current controversy on topological and mechanistic explanations raises three issues: are topological explanations real explanations and do they exist in biomedical sciences? In what sense topological explanations differ from mechanistic explanations? And what is the specific contribution of topological explanations to our understanding of a given phenomenon, compared to mechanistic explanations?
In order to explore these three interrelated issues, I focus on the case of network medicine and medical genetics. I will first point out three main shortcomings of the current mechanistic explanations of genetic diseases in contemporary medical genetics, namely the collapse of the mechanistic definition of monogenic disease, the progressive geneticization of every disease and the dissolution of the distinction between monogenic and polygenic diseases. Second, I will introduce network medicine, a recent discipline born form the synthesis between genomics, systems biology and network theory. I will especially focus on one of the main tools of network medicine: the diseasome whose aim is to represent as a network the relationships between every human disease gene and every human disease. Third, I will show how the topological properties of the diseasome partially renew the traditional mechanistic explanation of the genetics of disease. However, I will argue that network medicine does not provide pure topological explanations, since topological explanations developed by network medicine are highly dependent on mechanistic information. I will also point that some gaps remain in our understanding of the genetics of diseases and that new mechanistic explanations are needed in order to fill these explanatory gaps. Finally, I will conclude on the specific contribution of topological explanations to our understanding of diseases: instead of focusing on the explanation of single diseases, they push us to develop a general explanation of disease. 4 2 In this paper, I will stick to the most widely accepted, but also stricter conception of mechanisms, namely the MDC account. 3 To get a detailed account of this analysis, see Woodward (2013) .
Among the reasons why philosophers of medicine are interested in mechanistic explanations of diseases, some of them, such as Thagard (2000 Thagard ( , 2006 , highlight their classificatory power. In this view, the identification of mechanisms can be used for classificatory purposes, thus moving away from pure phenotypic characterization of disease towards mechanism-based characterization of diseases and allowing us to distinguish between disease classes (such as infectious diseases, autoimmune diseases, etc.), each disease class being defined by one or a series of mechanism(s):
Not all diseases are caused by germs, but other major kinds have been amenable to mechanistic explanation. Nutritional diseases such as scurvy are caused by deprivation of vitamins, and the mechanisms by which vitamins work are now understood. For example, vitamin C is crucial for collagen synthesis and the metabolism and synthesis of various chemical structures, which explains why its deficiency produces the symptoms of scurvy. Some diseases are caused by the immune system becoming overactive and attacking parts of the body, as when white blood cells remove myelin from axons between neurons, producing the symptoms of multiple sclerosis. Other diseases such as cystic fibrosis are directly caused by genetic factors, and the connection between mutated genes and defective metabolism is increasingly well understood. The final major category of human disease is cancer, and the genetic mutations that convert a normal cell into an invasive carcinoma, as well as the biochemical pathways that are thereby affected, are becoming well mapped out. (Thagard 2008, p. 384) This is of tremendous interest in medicine, since there seems to be a very intuitive link between identifying the parts and the activities of the mechanisms responsible for the disease and finding a treatment aiming at restoring the dysfunctional mechanism or at altering its course. Now, such a seemingly simplistic classification of diseases classes in contemporary medicine is probably debatable, since, for example, this way of classifying diseases does not mirror the categories presented in the International Classification of Disease -ICD 10. But the point that I want to make and what Thagard has in mind here, is that, once a general mechanism has been identified for a disease class, each individual disease belonging to this class can get a detailed mechanistic explanation, where the parts and activities involved in this given disease are specified. However and more importantly, while I will not assert that each disease class is identified with a mechanistic explanation in medicine, it is true that in the specific case of the history of medical genetics, mechanistic explanations have played an important classificatory role, with major consequences on biomedical research. In order to understand the current conceptual challenges of medical genetics, one needs to go back to the 1960s, when genetic diseases were considered to be monogenic diseases, when genetic diseases were a specific class of rare, inherited, Mendelian, monogenic disorders and when the distinction between monogenic and polygenic diseases was strongly delineated. Phenylketonuria then embodied this concept of genetic disease viewed as synonymous with monogenic disease (Lindee 2000 (Lindee , 2002 Paul 1994 Paul , 2000 Paul , 2013 . Indeed, phenylketonuria is a rare disease whose prevalence varies from 1/4000 to 1/40,000. It is an inherited disease, as it is passed down from parents to children. It is a Mendelian disease, with an autosomal recessive transmission; meaning two mutated alleles are necessary for the disease to occur. It is a monogenic disease, that is, caused by the mutation of one gene: the PAH gene, which codes for the phenylalanine hydroxylase enzyme. Phenylalanine hydroxylase is necessary to convert phenylalanine, an essential amino acid found in food, into another amino acid, tyrosine. When this enzyme is mutated, phenylalanine cannot be converted in tyrosine and builds up in the blood, thus exerting a toxic effect on the central nervous system. When untreated, phenylketonuria (PKU) leads to severe mental retardation. However, a simple diet without phenylalanine, administered from birth, prevents the onset of disease. On the model of this mechanistic explanation of phenylketonuria, the mechanistic explanation of monogenic disease in the 1960s can thus be described as: one inherited Mendelian mutation in one gene causes one dysfunctional protein that, in turn, causes the symptoms and the states of one disease. This mechanistic explanation of genetic disease had a huge impact on the development of specific research methods for identifying the genes involved in monogenic diseases, giving rise to the development of monozygotic twin studies, linkage analysis, candidate-gene approach to name a few, and leading to major successes in reverse genetic (Badano and Katsanis 2002; Jordan 1988 Jordan , 2006 .
However, since the establishment of phenylketonuria as a paradigmatic example of genetic disease, a double shift has occurred in medical genetics (Melendro-Oliver 2004) . On the one hand, the concept of genetic disease has extended far beyond the concept of monogenic disease, which it was synonymous with. Several scientific discoveries have contributed to this extension of the concept of genetic disease. The discovery of susceptibility genes in the 1970s (genes that are associated to the occurrence of a disease but whose presence is not sufficient to cause it) and the discovery of oncogenes and anti-oncogenes in the 1980s (genes whose activation or repression plays a major part in the development of cancer) have drawn attention to the genetics of polygenic common diseases. The rise of DNA sequencing and genetic engineering techniques has allowed the development of various methods for identifying allelic variants and an upsurge of gene-disease associations. In the contemporary biomedical literature, every disease whose occurrence is statistically associated to an allelic variant (a variation of one or more nucleotides in a gene) tends to be considered genetic. Nowadays, the concept of genetic disease thus applies to common diseases. These diseases are not hereditary, but due to de novo mutations (mutations that appear in a gamete of one of the parents or in the fertilized egg itself) or to acquired mutations (mutations due to environmental effects, for example). Their transmission does not necessarily follow Mendel's laws and they are said to be polygenic or complex, because their physiopathology implies the joint action of several genes and many environmental factors. There are several mechanistic models of polygenic diseases (Badano and Katsanis 2002) . "Major gene effect" designates a mechanism where one main genetic mutation with a major effect on the phenotype is associated to several other genes with a low effect and several environmental factors. "Oligogenic" disease designates a mechanism where a few genes have a major effect on the disease occurrence but are associated to several other genes with minor effects and to environmental factors. Finally, "true" polygenic diseases are diseases whose occurrence depends on multiple genes with a minor effect and multiple environmental factors. Thus, cancer, diabetes, hypertension and even tuberculosis-usually considered a paradigmatic example of environmental diseases, as an infectious agent causes it-have progressively been considered genetic. This phenomenon is usually called "the geneticization of diseases" and has been well explored in sociology of medicine. 5 On the other hand, several scientific discoveries have disrupted our understanding of monogenic disease and blurred the distinction between simple monogenic diseases and those that are complex and polygenic. Indeed, three major new mechanisms have been recently revealed in the pathophysiology of phenylketonuria (Scriver and Waters 1999; Scriver 1995 Scriver , 2007 Scriver and Waters 1999; Scriver 1995 Scriver , 2007 : allelic heterogeneity (over 500 mutations of the PAH gene can cause phenylketonuria), genetic heterogeneity (when the gene PAH is normal, a mutation of the BH4 gene that codes for its receptor can be sufficient to cause the disease) and modifier genes (the BH4 gene influences the expression of the PAH gene and the consequences of its mutations on severity and variability of symptoms). These new mechanisms have undermined the linear and specific correspondence between a mutation in the PAH gene, the production of a mutated PAH protein and the occurrence of phenylketonuria. It is now widely acknowledged that these three new mechanisms, namely allelic heterogeneity (several mutations in the same gene can cause the same disease), genetic heterogeneity (several genes can cause the same disease) and modifier genes (one or more gene(s) can influence the disease phenotype) are at play in monogenic diseases and have called into question the apparent simplicity of monogenic diseases (Dipple and McCabe 2000a, b) .
A paradox thus lies at the heart of contemporary medical genetics. On the one hand, every disease seems to be considered genetic and we have discovered several mechanisms involved in the genetics of disease. On the other hand, there is no consensual definition of what is a genetic disease and the distinction between monogenic disease and polygenic disease keeps getting blurrier and blurrier (Table 1) .
I do not claim here that mechanistic explanations of individual genetic diseases are vain or irrelevant. From a mechanistic point of view, our understanding of phenylketonuria is definitely much more detailed now than it was in the 1960s and the same can be claimed about many so-called "monogenic" diseases. What I claim is that there is no longer a unified schematic mechanistic account (such as the "one mutation in one gene > one dysfunctional protein > one disease") that would hold for every monogenic disease and that would successfully discriminate between genetic disease and non-genetic diseases or even between monogenic diseases and polygenic diseases. So, mechanistic genetic explanations do not allow us to identify a mechanism-based disease class called "genetic diseases", since the physiopathology of every disease can imply genetic mechanisms. And they do not allow us to distinguish between monogenic diseases and polygenic diseases, since the difference between some mechanisms The distinction between monogenic and polygenic diseases is blurry exhibited in monogenic diseases (such as modifier genes) and polygenic diseases (such as "major gene effect") seems to be highly relative and since most mechanisms at play in monogenic diseases (allelic heterogeneity, genetic heterogeneity, modifier genes) can also be found in polygenic diseases. Therefore, even if mechanistic explanations in medical genetics still are needed in medical genetics, they do not fulfill anymore their classificatory or unifying purpose and they struggle to answer three research questions, namely what a monogenic disease is, the geneticization of diseases and the difference between monogenic and polygenic diseases. There have been some attempts to integrate these shifts in regional mechanistic explanations. For example, Casanova and Abel, two French geneticists at the Necker Hospital, have successfully developed a genetic theory of infectious diseases (Alcaïs et al. 2009; Abel 2007, 2013) . This theory aims to explain interindividual variability to infections by identifying four genetic mechanisms at play in infectious diseases: Mendelian monogenic predisposition to one infection, Mendelian monogenic predisposition to several infections, major gene/resistance to one infection and polygenic predisposition to one infection ( Table 2 ). The strength of their theory relies on the fact that every mechanism does not correspond to a subclass of infectious diseases, but that several mechanisms can be at play in the same disease (Darrason 2013 ).
For example, the genetics of tuberculosis can involve, depending on individuals, either Mendelian monogenic predisposition to several infections or major gene/resistance to one infection or polygenic predisposition (Abel and Casanova 2000; Alcaïs et al. 2005; Baghdadi et al. 2006) . While these mechanisms might be extrapolated to other disease classes and while their identification constitutes a progress in the explanation of the genetics of infectious diseases, they still rely on oversimplifications of the underlying mechanisms since, as I have previously discussed, the difficulty lies precisely in distinguishing between Mendelian monogenic and polygenic diseases. One way to solve this situation would be to acknowledge that it is very difficult to get general genetic mechanisms in disease explanations and that we should stick at localizing and decomposing the specific genetic mechanisms at play in each individual disease and eventually at finding very schematic regional genetic mechanisms for some disease classes. However, these shortcomings of mechanistic explanations have very concrete consequences on clinical research in medical genetics today. Indeed, while the clear-cut mechanistic explanation of monogenic diseases in the sixties led to the development of gene identification techniques and to many successes in reverse genetics, the increasing complexity of mechanistic explanations of polygenic diseases made it more difficult to develop similarly successful and efficient gene identification techniques for polygenic diseases (Botstein and Risch 2003; Feingold 2005) . To some extent, the final outcome of this increasing complexity precisely led to the development of genome-wide association studies, which is a gene identification technique that is specifically designed in order to require as least biological hypotheses as possible about the underlying mechanisms of the disease under investigation. While genome-wide association studies raised great hopes, they were also quite deceptive, since many of the disease-gene associations they identify were not confirmed (Feingold 2005; Hirschhorn and Daly 2005; Visscher et al. 2012) . In other words, I claim that the current complexity and concreteness of mechanistic explanations in the genetics of diseases lead genomic research in a corner, with the seemingly insurmountable task to decipher the molecular mechanisms of thousand of individual diseases, without the help of general identification research methods. However, there is another way to solve this current paradox of medical genetics: it is to look for a different type of disease explanation, that abstracts away from the complex mechanistic explanations of the role of individuals genes in individual diseases in order to consider the general role of genes in disease explanations. This is precisely what network medicine suggests doing.
Network medicine is a recent research program, mainly developed by the team of Albert-László Barabási Barabási and Oltvai 2004; Barabási 2007) and born from the synthesis between the concept of "human disease genes", the development of systems biology and medicine and the formalization of network theory-three theoretical pillars that I am now going to detail.
The concept of "human disease genes" rests on a double distinction (Jimenez-Sanchez et al. 2001) . First of all, it aims at distinguishing between human genes and non-human genes (such as animal genes). Secondly, it distinguishes between disease genes and non-disease genes. The point is that human disease genes may have specific characteristics that differ from non-disease genes.
Systems biology (Bruggeman and Westerhoff 2007; Conti et al. 2008; Griffiths and Gray 2005; Kitano 2002 Kitano , 2007 is an interdisciplinary research program, that emphasizes that the study of the individual components of a system is not sufficient to get a full understanding of its complexity and of its properties. It relies on bioinformatics and mathematical modeling to represent and explore the interlevel and intralevel interactions between the components of complex systems and aims at finding general organizing principles in organisms. The definition of systems medicine and its relationships with systems biology have been the subject of many debates Clermont et al. 2009; Wolkenhauer and Green 2013) . Systems medicine aims at discovering some organizing principles in disease. In systems medicine, disease is not only a biological event, it is a very complex system composed of many interlevel components, going from DNA strands and tissue and organs to socio-economic factors, just to name a few. It thus partially rests on the results and findings developed by systems biology, but also requires developing its own specific tools and models.
Finally, network theory has developed solid mathematical and computer-based methods to decipher the underlying architecture behind apparently anarchic networks such as the World Wide Web, social networks and biological networks (Barabási and Bonabeau 2003; Barabási 2011 Barabási , 2012 Jeong et al. 2000) . The basic components of a network are nodes, connected together through edges. The basic properties of a network are the total numbers of nodes in the network (N) and the degree of a node (k), that is the number of nodes a given node is connected to. Depending on the degree distribution of a network, that is, on the probability distribution of these degree P(k) over the whole network, it is possible to distinguish between random networks and scale-free networks. In random networks, nodes follow a Poisson distribution, meaning that every node has on average the same number of connected nodes. Scale-free networks have a very different structure: their nodal distribution follows a Power law, meaning that there are both some highly interconnected nodes (that are called "hubs") and very sparsely connected nodes in scale-free networks. This is a "the rich-get-richer distribution": in this kind of network, the more a node is connected, the most connected he is going to become (Barabási 1999) .
Combined together, these three disciplines naturally led to network medicine that aims to develop network-based approaches to disease by analyzing the interactions between different kinds of networks in a given disease and between apparently distinct diseases. Indeed, one of the main hypotheses of the network medicine is the interconnectivity of the cell components. Based on this interconnectivity property, disease can never been understood as the result of a single mutation in a single gene. On the contrary, disease is defined as a perturbation in a complex network of intra and extracellular components in a tissue specific or in an organ specific system. In this framework, it is very likely that diseases are not discrete and clinically defined entities but have intertwined relationships with each other, since different diseases may share a same functional module of components, disrupted in different ways. Therefore, the aim of network medicine is both to identify the pathological network of each disease and to identify which diseases share which networks.
In order to do so, network medicine relies on the systematic comparison between the human interactome and various disease networks (Fig. 1) . In a narrow sense, the interactome is the whole set of molecular interactions existing in a giving cell at a giving time. So, it is the whole set of the gene-gene, gene-protein, protein-protein, transcription factors-protein interactions, and so on and so forth. In a broader sense, the interactome designates the whole set of molecular interactions existing in an organism under specified conditions (Cusick et al. 2005; Vidal et al. 2011 ). The differences between the interactome of a particular cell and the interactome of an organism are huge, since an organism consists in several cellular types. A complete human interactome that would roughly incorportate 25,000 human genes, around 10 6 proteins, and their interactions, is yet to be drawn. The partial human interactome that are used nowadays, roughly incorporates 50,000 unique proteins, involved in around 200,000 interactions (Janjic and Przulj 2012) . Disease networks may include disease genes networks (Goh and Choi 2012; Loscalzo et al. 2007 ), protein-protein interactions networks (Zhang et al. 2011) , metabolic networks (Jeong et al. 2000; Lee et al. 2008) .
However, since the aim of this paper is to discuss how network medicine deals with conceptual issues in contemporary medical genetics, I will focus especially here on Fig. 1 The theoretical pillars of network medicine. On the left side is represented the interactome, that is, the set of every physiological network of an individual, including gene-gene interactions, protein-protein interaction and metabolic networks. On the right side are represented the different pathological networks, including for example the diseasome. Network medicine consists in comparing these two sets of networks in order to understand the specificity of pathological networks. In order to do so, network medicine relies on three theoretical pillars, namely systems biology and medicine, the concept of human disease genes and network theory the diseasome (Loscalzo et al. 2007 ) and on its relationships to the interactome. The diseasome intends to represent the relationships between human diseases and diseasecausing genes. The construction of the diseasome is a two-step process. First, the researchers constructed a bipartite graph, consisting of two disjoint sets of nodes. One set corresponds to all known genetic disorders, whereas the other set corresponds to all known disease genes in the human genome. A disorder and a gene are then connected by an edge if mutations in this gene are involved in the disorder. The list of disorders, disease genes, and gene-disease association was obtained from the Online Mendelian Inheritance on Man (OMIM) database. OMIM represents the most complete and up-todate repository of all known disease genes (Amberger et al. 2009; McKusick 2007) . As of December 2005 the list contained 1,284 disorders and 1,777 disease genes. Once this bipartite graph is built, it is possible to construct two projections, which are basically the two faces of the same coin. On the one hand, there is the human disease network (HDN), where diseases are nodes and two diseases are connected if they share a same gene in their physiopathology (Fig. 2a) . On the other hand, there is the human disease gene network (DGN) where genes are nodes and two genes are connected if they are involved in the physiopathology of the same disease (Fig. 2b) . The first purpose of the diseasome is to pinpoint some unnoticed interactions between two types of diseases to direct more effectively the search of genes candidates and the understanding of the functional and topological modules in which the given genes interact. The second one is to characterize the specific properties of the "human diseases genes" by adding biological information on these genes to the topological analysis of the network. Goh et al. (2007 , p. 8687), Copyright (2007 , National Academy of Sciences, USA]. A node's size is proportional to its degree of connectivity. The color code allows for the distinction between different disease classes. b The disease gene network [reproduced with permission, from Goh et al. (2007 , p. 8687), Copyright (2007 , National Academy of Sciences, USA]. A node's size is proportional to its degree of connectivity. The color code allows for the distinction between different disease classes. (Color figure online) Finally, the third aim of the disease is to be compared to the interactome, in order to find some general organizing principles of the genetics of human disease.
Before analyzing the topological properties of the diseasome, let us make some general remarks on how the diseasome was built and on the robustness of its analysis.
Although OMIM is the most up-to-date repository on the genetics of human disease, it is important to specify that it was originally restricted to monogenic disorders and has only in recent years expanded to include complex traits and the associated genetic mutations. Moreover, the diseasome on which were performed the first topological analyses that I am now going to describe, only contains the OMIM data from 2005. It is however worth noting that there are several reasons to believe in the robustness of these analyses. Indeed, first, the researchers that built the first version of the diseasome simulated the inclusion of additional (but more noisy) gene-disease associations (thus going from 1,777 to 2,765 gene-disease associations): this in silico expansion of the diseasome did not affect the general structure of the obtained network. Second, the properties of scale-free networks, such as the diseasome, are called "overdetermined properties": theoretically speaking, it is not necessary to know the total number of nodes in the network to identify its general structure and properties. Finally, an expanded version of the diseasome was performed in 2012 (Zhang et al. 2011) . This new version of the diseasome does not only take into consideration gene-disease associations but also protein-disease associations. In order to create this expanded version, the researchers used a different database, called the Genetic Association Database (GAD). The properties of this new version of the diseasome are still very stable compared to the early version that I am now going to analyze.
Three main analyses can be drawn from the diseasome. The first one is a global analysis whose aim is to characterize the general structure of the network. The second one is a local analysis that compares topological properties from the human disease genes network with biological information on the pathophysiological role of these human disease genes. The third one consists in comparing topological properties of the human disease genes network with topological properties of the interactome, which represents the set of possible biological interactions in a human organism.
The first analysis of the diseasome is global and topological: the main aim is to qualify the general behavior and the topological properties of both networks, using the network theory's toolbox. In the human disease network, as in the human disease gene network, it appears that the nodes (respectively, the diseases and the genes) are highly interconnected (meaning there are very few nodes that have no connections at all to the general network) and that the degree distribution in both networks follows a power law distribution (meaning that a few nodes have far more connections in the network than the others and that they play the role of hubs in the network). To put it differently, from a topological point of view, this means that the human disease network and the disease gene network are scale-free networks. Indeed, over 1,284 diseases, 867 are connected to another disease and 516 (around 40 % of the represented diseases) form one giant cluster. Among the hub diseases, cancer is particularly well represented, with colon cancer being linked to fifty other diseases, while breast cancer is connected to thirty other diseases. There is a strong heterogeneity in gene-disease associations: some diseases involve around thirty genes, while others involve only one or two. For example, deafness is associated to 41 genes, leukemia to 31 and colon cancer to 34.
Conversely, some genes are involved in many diseases (and play the role of hubs in the disease gene network), while others are involved in only one or two diseases. For example, TP53, which is an extremely important gene in oncogenesis, is involved in more than ten diseases. This first topological analysis might seem quite simple: it still points toward a first strong hypothesis: the hypothesis of the common genetic origin of diseases. Indeed, would each human disease have a distinct genetic origin, the human disease network would either only exhibit disconnected sub-networks, composed of few isolated nodes, each one corresponding to a disease, or would be composed of small subgroups of similar disorders. But since the distribution of both networks significantly differs from these hypotheses and from the distribution of a random network, it suggests that most diseases share some interconnected genes and that genes involved in the same disease may be involved in some common pathways.
The second analysis is a local analysis: the aim is to test this hypothesis of a functional clustering of human disease genes and to analyze the behavior and the properties of genes that are involved in the same disease. In other words: when two genes are involved in the same disease, does that mean that they interact in the same functional module? And when two diseases involve the same gene, does that mean that they share some pathophysiological mechanisms? Testing this "local hypothesis" requires characterizing whether two genes involved in the same disease produce interacting proteins, whether they are co-expressed at the same time and in the same tissues and whether they have close molecular functions. In order to do so, it is necessary to include some biological information about the genes and the diseases represented in the diseasome. Part of this biological information was retrieved from OMIM, but the researchers also retrieved information from (a) a network of physical protein-protein interactions derived from high-quality systematic interactome mapping and literature curation and (b) GO 6 annotations for each gene (c) data on the time, place and importance of the expression of the genes represented in the diseasome derived from DNA and RNA biochips results inventoried in the database Entrez Gene ID (linked to OMIM). By comparing these biological data to the diseasome, it was possible to conclude that genes involved in the same disease tend to (a) interact via protein-protein interactions, (b) be expressed in the same specific tissues (c) be strongly co-expressed, (d) exhibit synchronized expression as a group (e) share the same Gene Ontology. Based on this confirmation of the local hypothesis, they develop the concept of disease functional module: Cellular networks are modular, consisting of groups of highly interconnected proteins responsible for specific cellular functions (21, 22) . A disorder then represents the perturbation or breakdown of a specific functional module caused by variation in one or more of the components producing recognizable developmental and/or physiological abnormalities. (Goh et al. 2007) This is a major hypothesis of network medicine: when diseases share genes or when several genes are associated to the same disease, they belong to the same functional module, that is, to a set of molecular elements consisting of transcription factors, genes, proteins, that interact in a certain way to achieve a given cellular or molecular function. A disease module consists of four main components. The primary disease genome G is the set of molecular anomalies that are associated to the phenotype. The secondary disease genome D is the set of modifiers genes that are susceptible to influence the primary genetic anomaly. The intermediate phenotype I is the set of polymorphisms that are susceptible to influence each of the generic answers of the organism to stress. Finally, E stands for the environmental determinants of a given disease.
The third analysis gets to another level, since it aims at comparing the diseasome with the interactome 7 and at characterizing human disease genes properties compared to human non-disease genes properties. One of the main topological properties of interest is the question of centrality-essentiality. The concept of "essential genes" is intrinsically linked to gene knockout experiments, in which an organism's gene is selectively made inoperative. A gene is considered to be essential for an organism if it is necessary for its survival, i.e., if a knockout of the corresponding gene leads to the lethal mutant. Since such experiments cannot be conducted on humans, a human gene is considered essential if the knockout of its murine orthologue leads to the death of the mutant (in the embryonary state, in the prenatal state or in the immediate postnatal state). Since previous analyses of the yeast protein interaction network seemed to prove that essential genes constitute central hubs in the yeast (Jeong et al. 2001) , human disease genes were expected to be essential genes and to constitute hubs in the diseasome. Indeed, a first topological analysis of the interactome seems to prove that proteins produced by human diseases genes have a higher connectivity than proteins whose genes are not involved in human diseases. So, if centrality (the capacity to be a hub) is taken as a proxy for being essential, this first analysis seems to confirm that human diseases genes are essential genes. However, when using murin orthologues of the human disease genes to determine a given gene's essentiality, the situation appears to be more complex: over the 7,533 genes of the reconstructed interactome, the researchers identified 1267 essential genes that are not associated to any known disease. Over the 1,777 human disease genes represented in the diseasome, there are 398 essential human disease genes and 1,379 human disease genes that are not essential. To put it shortly, it seems that the vast majority of human disease genes are not essential genes, do not encode hubs and are located at the periphery of the interactome, while a few of them are essential genes, encode hubs and are located at the center of the interactome. To explain this surprising result, the researchers made an evolutionary hypothesis: the vast majority of human disease genes are non-essential and centered at the periphery of the interactome because, when mutated, they only lead to disease instead of leading to death in utero. Not all genes can be diseases genes: some genes would be too essential for the development of the organism; were they mutated, there simply would not be an individual to pass on the mutations to offspring.
As I have pointed out previously, pure mechanistic explanations in medical genetics nowadays struggle with three issues: how to account for the role of genes in every disease? How to account for a unifying description of the genetics of a given disease? And, how to account for the relativity of the distinction between monogenic and polygenic diseases? On each of these issues, based on the results I have described, network medicine provides some new explanations.
First, the local hypothesis, that relies on the global and local analyses of the diseasome, provides an explanation for three phenomena linked to the geneticization of diseases, namely syndrome families, comorbidity and diseases classes. A syndrome disorder is usually a disorder that has no identified cause and that associates various symptoms without apparent links together. Syndrome families are a group of disorders that seem to have some symptoms in common but whose main cause is not understood. The local hypothesis means that, if syndrome families have some symptoms in common, it is because they share interconnected genes that interact in overlapping disease functional modules. The same goes for comorbidity. In its narrow sense, comorbidity means that two or more diseases occur together in the same individual. In a broader sense, comorbidity is the fact that having disease A raises your risk of having disease B. A way to explain this phenomenon is to make the hypothesis that diseases that tend to happen together imply the same genes encoding interacting proteins in the same metabolic pathways. To put it differently, if, being obese, an individual is more likely to get diabetes; it is partially because obesity and diabetes share common genes in their physiopathology. Finally, if diseases belong to the same disease class, whether this one is based on an etiological category (such as cancer) or on an anatomical localization (such as cardiovascular diseases), it is because they share some common genes that interact in overlapping disease modules.
The second point concerns the concept of genetic disease. Although not explicitly, network medicine abandons the concept of genetic disease to focus on an explanation of the genetics of every disease based on the identification of the disease functional module, which is defined by the four types of components that I have described (primary genome, secondary genome, intermediate pathophenotypes, environmental determinants). These four modules interact together to produce pathophysiological states (P), which are basically the symptoms of the disease. For example, in phenylketonuria, the primary genome would be the PAH gene, which codes the phenylalanine hydroxylase enzyme. The secondary genome would be the BH4 gene and all the modifier genes that are known to influence the expression of the PAH gene. The intermediate phenotypes would be all the physiopathological phenomena that lead from hyperphenylalanemia to brain damages: (a) direct toxicity of phenylalanine on brain cells, (b) the fact that, since the PAH enzyme aims at converting phenylalanine into tyrosine, a deficit in PAH enzyme also results in a deficit in tyrosine, which is a precursor of very important neurotransmitters, such as dopamine, adrenaline and noradrenaline (c) the fact that, in phenylketonuria, phenylalanine competes with other amino acids to enter the brain, since it shares the same transporters, thus altering the intracerebral protein synthesis. Finally, environmental determinants would include the amount of phenylalanine intake depending on the diet, treatments, etc. Not every disease includes a primary Loscalzo et al. (2007, p. 6) . Different types of diseases are identified based on the components of their disease modules. G primary disease genome, D secondary disease genome, I intermediate phenotypes, E environmental determinants, P pathophenotypes (i.e., symptoms of the disease) genome-typically, in true polygenic diseases, no gene is necessary and sufficient for the disease to occur. So, some disease modules would not include any primary genome. But such an explanation of the genetics of disease allows the description of several models for several kinds of diseases, from diseases that are closer to classic Mendelian disorders to "true" polygenic diseases (Fig. 3) .
Eventually, the concept of disease module explains how the difference between monogenic and polygenic diseases can be understood in terms of functional redundancy and robustness. In a system, for a given function, there is functional redundancy when several independent pathways in the system can achieve the same function. The more a system exhibits functional redundancy, the more robust it is. Based on these two properties, monogenic diseases can be redefined as diseases whose modules exhibit low functional redundancy and consequently, low robustness, while polygenic diseases are diseases whose modules exhibit high functional redundancy, and consequently, high robustness (Debret et al. 2011) . To put it differently, if the functional module of a given disease depends on one fundamental pathway to achieve the corresponding function, then any disruption (for example, one genetic mutation) is enough to inactivate the given function and disorganize the module to the point where the disease occurs. If, on the contrary, the functional module of a disease consists of several redundant sub-modules, then a conjunction of several events is necessary to inactivate several modules and cause the occurrence of the disease (Table 3) .
What do we learn from this case study on the relationships between topological and mechanistic explanations? My first conclusion is quite simple but not so trivial, given the current debates about the existence and the relevance of non-mechanistic explanations in biomedical sciences: there are topological explanations in medicine and they can be quite powerful. In this specific case study, I have shown how topological explanations can help solving issues in medical genetics that pure mechanistic explanations of disease have been struggling with. First, topological explanations in network medicine help to understand three phenomena linked to the geneticization of diseases (syndrome families, comorbidities and disease classes). Second, they allow us to abandon the concept of genetic disease in order to understand the various roles that genes can play in every disease through the identification of disease modules. Finally, they explain the difference between monogenic diseases and polygenic diseases not as a mechanistic difference, but as a difference in the structure of the disease module that can be understood in terms of robustness and functional redundancy.
However, and this my second point, it is obvious that network medicine does not rely only on pure topological explanations, or, to put it differently, that topological explanations in network medicine highly depend on mechanistic explanations, for at least two reasons. First, the relationships that are represented in networks are mechanistic, even though it is in virtue of the features of the network and not of the details of these relationships that an explanation is provided. Indeed, the diseasome is an abstract representation of gene-diseases associations, that is, of the mechanistic relationships that are, if not always proved, at least strongly supported, between a given gene and the occurrence of the corresponding disease. 8 So, in this sense, topological explanations cannot be understood as completely independent from mechanistic explanations, at least in network medicine.
But, in a stronger sense, I claim that even interpreting the topological properties of the network highly depends on mechanistic information. For example, the local hypothesis, according to which genes and gene products that are involved in the same disease have an increased tendency to interact together and to belong to the same disease module, depends highly both on a topological property of the diseasome (the scale-free network property) and on mechanistic information on the human disease genes represented in the diseasome (about protein-protein interactions, about the level, time and place of human disease genes expression, etc.). In a similar way, interpreting the degree of essentiality-centrality of human diseases genes is also highly dependent on the import of external mechanistic information on the systematic knockout of their murin orthologues. My point is not to claim that topological explanations and mechanistic explanations can always be seen as complementary. But in the case of network medicine, not only the network itself is an abstract representation of facts about mechanistic relationships, it needs to be interpreted in mechanistic terms: the local hypothesis and the concept of disease module are fundamentally mechanistic concepts about the relationships between various components (genes, proteins, transcription factors, metabolic reactions, phenotypes, symptoms…) and about how the way that these components are organized or disorganized can lead to disease.
Another point worth noting is that, if network medicine provides some interesting explanations about the genetics of disease, at least two types of major gaps remain. First, we may wonder why the diseasome has such topological properties, how it has evolved to be a scale-free network, why some functional disease modules are more robust than others to external perturbations and why the human disease genes are mostly at the periphery of the interactome. I have mentioned some evolutionary hypotheses about this last point: human disease genes would be located at the periphery of the interactome, because their mutations do not lead to death in utero. Second, there are still missing gaps in our understanding of what a disease module is, how it works and what kind of interactions cause the occurrence of the disease. From this point of view, it seems obvious that topological explanations are not enough to explain diseases but provide a strong incentive to search for new mechanistic explanations. Indeed, once diseases are defined as disease modules, the next step is to identify and localize the parts of each disease module and to understand the mechanistic relationships that link these parts together. For instance, once phenylketonuria has been redefined as a disease module, the next step is to understand how its primary genome, secondary genome, intermediate phenotype and environmental determinants interact together to produce the disease.
So, to put in a nutshell, following Huneman and Woodward, I claim that topological explanations and mechanistic explanations are different because they do not explain the same phenomenon (the genetics of disease, in this case) in virtue of the same properties (topological vs. material properties) and because they capture different features of the same phenomenon. Indeed, and this will be my final point, while mechanistic explanations can attain some level of generality, their main aim is to get concrete details about the way parts and activities are organized in a set of spatial-temporal conditions in order to produce a phenomenon. Topological explanations, on the other hand, are concerned about more general properties of the system, such as robustness and functional redundancy: their aim is to explain how a phenomenon can resist or react to a set of various perturbations. In the case of medicine, the specific contribution of topological explanations is to completely shift the conceptual background of our understanding of the role of genes in disease. Instead of considering single individual diseases as completely distinct entities, whose genetic mechanisms need to be investigated separately, topological explanations push us to understand diseases as intertwined phenomena that are linked together from a genetic point of view and that need to be investigated from a common and general perspective. In other words, topological explanations in network medicine push us towards the search for organizing principles in the genetics of disease, instead of focusing on mechanistic genetic explanations of single individual diseases.
This may have major consequences on biomedical research and has already led to the development of new ways of identifying new disease genes. Three methods have been developed. In the linkage-based method, candidate disease nodes (i.e. candidate disease genes) are identified by direct interaction with known disease node (i.e known disease gene). In disease module based methods, algorithms are used to group highly interconnected genes, in the hope of identifying potential functional disease modules in the interactome. In the disease-module based methods, algorithms or functional information are used in order to identify genes that closely neighbor a known disease module. For example, if two modules are involved in the same pathway by a common gene product, the genes belonging to the neighbor module are considered potential candidate disease genes (Chan and Loscalzo 2012) . Although these techniques are quite recent, they already meet some success in unraveling new disease genes in diseases as different and complex as asthma (Sharma et al. 2015) , breast cancer (Erler and Linding 2012) or cardiovascular diseases (Sharma et al. 2013 ).
In this paper, my aim was to examine the relationships between mechanistic and topological explanations through the case study of network medicine and medical genetics. Indeed, medical genetics has developed pure mechanistic explanations of the genetics of disease that meet with some serious issues. These pure mechanistic explanations cannot give a unifying and satisfying explanation of the concept of genetic disease, the geneticization of disease and the distinction between monogenic diseases. By relying on the topological properties of the human disease gene network, network medicine provides an explanation of the common genetic origin of diseases, reinterprets the concept of genetic diseases through the identification of disease modules and explains the difference between monogenic and polygenic diseases as a matter of functional redundancy and robustness. However, topological explanations cannot be seen as independent from mechanistic explanations for three reasons. First, the network itself is an abstract representation of mechanism. Second, interpreting the topological properties of the network depends on external mechanistic information. Third, topological explanations are not complete explanations: they provide an incentive to new mechanistic explanations. To put it in a nutshell, topological explanations in medicine challenge the way we traditionally explain diseases but should not be seen as independent and radically different from mechanistic explanations: instead of looking for specific mechanisms for each individual disease, topological explanations push us to explain disease in general.
|
Annnotations
- Denotations: 1
- Blocks: 0
- Relations: 0