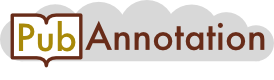
PMC:8010866 / 6541-6542
Forecasting Seizure Storms Using Epilepsy Wristband Sensors
Abstract
Machine Learning From Wristband Sensor Data for Wearable, Noninvasive Seizure Forecasting
Meisel C, El Atrache R, Jackson M, Schubach S, Ufongene C, Loddenkemper T. Epilepsia. 2020;61(12):2653-2666. doi:10.1111/epi.16719
Objective:
Seizure forecasting may provide patients with timely warnings to adapt their daily activities and help clinicians deliver more objective, personalized treatments. Although recent work has convincingly demonstrated that seizure risk assessment is in principle possible, these early approaches relied largely on complex, often invasive setups including intracranial electrocorticography, implanted devices, and multichannel electroencephalography and required patient-specific adaptation or learning to perform optimally, all of which limit translation to broad clinical application. To facilitate broader adaptation of seizure forecasting in clinical practice, noninvasive, easily applicable techniques that reliably assess seizure risk without much prior tuning are crucial. Wristbands that continuously record physiological parameters, including electrodermal activity, body temperature, blood volume pulse, and actigraphy, may afford monitoring of autonomous nervous system function and movement relevant for such a task, hence minimizing potential complications associated with invasive monitoring and avoiding stigma associated with bulky external monitoring devices on the head.
Methods:
Here, we applied deep learning on multimodal wristband sensor data from 69 patients with epilepsy (total duration >2311 hours, 452 seizures) to assess its capability to forecast seizures in a statistically significant way.
Results:
Using a leave-one-subject-out cross-validation approach, we identified better-than-chance predictability in 43% of the patients. Time-matched seizure surrogate data analyses indicated forecasting not to be driven simply by time of day or vigilance state. Prediction performance peaked when all sensor modalities were used and did not differ between generalized and focal seizure types but generally increased with the size of the training data set, indicating potential further improvement with larger data sets in the future.
Significance:
Collectively, these results show that statistically significant seizure risk assessments are feasible from easy-to-use, noninvasive wearable devices without the need of patient-specific training or parameter optimization.
Commentary
Devices worn by persons with epilepsy to detect seizures have become more accurate owing to recent advances. The development of wearable seizure-detection equipment has been driven by the fact that human reporting undercounts seizures by more than half compared to video electroencephalography (EEG) or ambulatory EEG monitoring.1 In a study of 100 patients who had previously participated in an anti-seizure medication research trial, most had stopped recording their seizures for a variety of reasons.2 Nevertheless, these patients expressed interest in seizure detection using wristband sensors.2 Wearable devices are now beginning to meet the need for seizure counting (to guide treatment decisions) and alerting caregivers (to possibly reduce the chance of injury and sudden unexpected death in epilepsy [SUDEP]). Even though these devices may offer some benefit to patients, it has been a long-term hope that devices would become available that have the capacity to go beyond detection of events to actual forecasting of seizures. The article by Meisel et al3 is groundbreaking because it suggests that future wearable devices actually may be able to do just that.
Wearable devices can employ accelerometer (ACM), photoplethysmography (to measure heart rate via blood volume pulse), and electrodermal activity (EDA) sensors, as well as skin EMG and scalp EEG electrodes and peripheral temperature probes. Although ACM- and EMG-based sensors rarely detect nonmotor seizures, the newest devices provide good sensitivity and specificity in the detection of generalized tonic–clonic seizures (GTCS). In one long-term study, 55% of patients reported that events detected by their ACM device influenced them to log seizures in their diary, and in 40%, it was believed to contribute to fewer injuries.4
The Embrace 2 and E4 are wristband devices for seizure detection (Empatica Srl) that contain ACM, EDA, gyroscopic, and peripheral temperature sensors.5 The Embrace 2 wristband's 4 sensors are coupled to a machine learning-based algorithm for the detection of GTCS, and has both US Food and Drug Administration and E.U. CE clearance. The E4 has only E.U. CE approval. Two computer applications can be paired with the Embrace 2. The Alert app connects the patient’s smartphone via Bluetooth to the device to automatically log possible GTCS and alert caregivers. The Mate app takes data from the Alert app and allows patients to view their seizure, activity, medication adherence, and rest history. Ongoing research has improved the classifying algorithms that analyze the ACM and EDA data from Embrace 2. One such algorithm, “classifier III,” yields a sensitivity of 94.55% with no missed nocturnal GTCS and a false alarm rate of 0.2 events per day.5 The incorporation of EDA data confirmed the presence of postictal autonomic dysfunction in the majority of GTCS.5 These data and information on postictal immobility after a GTCS might be used in the future to reduce injury and to warn against SUDEP.5-8
In contrast to wristband and other surface sensors, sub-scalp (epicranial) EEG electrodes and recording devices are minimally invasive systems in which electrodes are implanted using local anesthesia under the scalp and above the galea and skull. They represent a new generation of ultra-long-term (>1 month) extracranial EEG monitoring systems. They use these subcutaneous electrodes along with an external unit held over the implanted device to provide power, signal transmission, and EEG recording.9 One such system, the 24/7 EEG SubQ (UNEEG Medical A/S) uses 3 electrodes to produce 2 bipolar EEG channels. It was studied for up to 3 months and showed good reliability and tolerability.9 Nine adults with temporal lobe epilepsy underwent subcutaneous EEG recordings in the ambulatory setting for a total of 490 days, during which 338 seizures were recorded.9 Some patients were found to have electrographic seizures that were unreported, and other patients reported clinical seizures during which no ictal discharges were seen.9 Although some of these electrographic seizure discharges may have been subclinical and some of the clinical seizures may have not had a visible scalp EEG ictal discharge, these findings are consistent with many older studies showing that human seizure counting can diverge from EEG findings. The 24/7 EEG SubQ is CE approved in the E.U. It is easy to imagine that such ultra-long-term EEG monitoring, particularly with widespread sub-scalp electrode coverage of the head, could provide data in the future that could not only detect but also predict most seizure types (not just GTCS).
While wearable devices are currently being used merely to detect seizures as they occur, the hope for the future is that they can be used to forecast impending seizures so that patients can take evasive action. In first-of-its-kind research, Meisel et al report the seizure forecasting performance of the E4 device worn on a wrist or ankle in 69 children and young adults with epilepsy who were undergoing inpatient video-EEG monitoring.3 Deep machine learning was employed to evaluate multimodal (ACM, EDA, pulse, and temperature) data from the device. A better-than-chance ability to forecast seizures was found in almost half the patients.3 This finding is particularly impressive because the E4 provides somewhat noisy data and is located on a limb far from the brain. Seizure forecasting performance peaked for most patients when data from all 4 sensors were used and (surprisingly) did not differ between patients who had generalized-onset and those who had focal-onset seizures.3 It is not yet known if the E4 device is detecting a preictal autonomic change (pulse or EDA) or an ACM signature that is not readily apparent on human visual inspection.
Retrospective analysis of preictal EEG changes was conducted by NeuroVista to develop a forecasting technique. Scalp EEG had too many limitations, but cortical recordings (ECoG) allowed the development of a computer algorithm. This algorithm was tested using an implanted recorder and intracranial ECoG electrode system connected via telemetry to a handheld device with warning lights.10 Fifteen subjects first underwent long-term ECoG recording to develop patient-specific algorithms.10 If their algorithm met seizure prediction performance criteria, they then entered an advisory phase during which the device was enabled to provide visual and audible advisories to the patient about the likelihood of imminent seizures.10 In only a minority of patients did seizures occur largely during times of a “high likelihood advisory.” This small proof-of-concept study showed that seizure prediction ECoG recording devices may be possible, but more work is needed.
The report of Meisel et al3 suggests that seizure forecasting may be possible merely using wristband sensors. Nevertheless, more research and development are needed because the E4 device performed only slightly better than chance in this study. If it is learned that wristband devices alone are inadequate to forecast seizures, then the prediction accuracy using a combination of wristband sensors and devices that record different physiologic parameters (e.g., long-term sub-scalp EEG systems) should be studied. This kind of multimodality approach may be the most successful one to forecast seizures for both a broad range and a large number of persons with epilepsy. By David G. Vossler
ORCID iD: David G. Vossler https://orcid.org/0000-0003-4823-0537
|
Annnotations
last updated at 2021-11-18 02:43:02 UTC
- Denotations: 1
- Blocks: 0
- Relations: 0