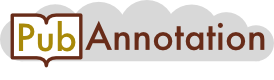
PubMed:31686039
Annnotations
Glycosmos15-CL
{"project":"Glycosmos15-CL","denotations":[{"id":"T1","span":{"begin":324,"end":332},"obj":"Cell"}],"attributes":[{"id":"A1","pred":"cl_id","subj":"T1","obj":"http://purl.obolibrary.org/obo/CL:0000540"}],"text":"Three-dimensional virtual refocusing of fluorescence microscopy images using deep learning.\nWe demonstrate that a deep neural network can be trained to virtually refocus a two-dimensional fluorescence image onto user-defined three-dimensional (3D) surfaces within the sample. Using this method, termed Deep-Z, we imaged the neuronal activity of a Caenorhabditis elegans worm in 3D using a time sequence of fluorescence images acquired at a single focal plane, digitally increasing the depth-of-field by 20-fold without any axial scanning, additional hardware or a trade-off of imaging resolution and speed. Furthermore, we demonstrate that this approach can correct for sample drift, tilt and other aberrations, all digitally performed after the acquisition of a single fluorescence image. This framework also cross-connects different imaging modalities to each other, enabling 3D refocusing of a single wide-field fluorescence image to match confocal microscopy images acquired at different sample planes. Deep-Z has the potential to improve volumetric imaging speed while reducing challenges relating to sample drift, aberration and defocusing that are associated with standard 3D fluorescence microscopy."}
GoldHamster
{"project":"GoldHamster","denotations":[{"id":"T2","span":{"begin":347,"end":369},"obj":"D017173"},{"id":"T3","span":{"begin":347,"end":369},"obj":"6239"},{"id":"T5","span":{"begin":394,"end":402},"obj":"SO:0000001"},{"id":"T6","span":{"begin":506,"end":510},"obj":"PR:000022679"},{"id":"T7","span":{"begin":506,"end":510},"obj":"PR:Q2FZJ6"},{"id":"T8","span":{"begin":506,"end":510},"obj":"PR:A5I112"},{"id":"T9","span":{"begin":506,"end":510},"obj":"PR:P24186"},{"id":"T10","span":{"begin":523,"end":528},"obj":"PR:Q07342"},{"id":"T11","span":{"begin":937,"end":942},"obj":"SO:0000343"}],"text":"Three-dimensional virtual refocusing of fluorescence microscopy images using deep learning.\nWe demonstrate that a deep neural network can be trained to virtually refocus a two-dimensional fluorescence image onto user-defined three-dimensional (3D) surfaces within the sample. Using this method, termed Deep-Z, we imaged the neuronal activity of a Caenorhabditis elegans worm in 3D using a time sequence of fluorescence images acquired at a single focal plane, digitally increasing the depth-of-field by 20-fold without any axial scanning, additional hardware or a trade-off of imaging resolution and speed. Furthermore, we demonstrate that this approach can correct for sample drift, tilt and other aberrations, all digitally performed after the acquisition of a single fluorescence image. This framework also cross-connects different imaging modalities to each other, enabling 3D refocusing of a single wide-field fluorescence image to match confocal microscopy images acquired at different sample planes. Deep-Z has the potential to improve volumetric imaging speed while reducing challenges relating to sample drift, aberration and defocusing that are associated with standard 3D fluorescence microscopy."}
PubMed_ArguminSci
{"project":"PubMed_ArguminSci","denotations":[{"id":"T1","span":{"begin":92,"end":275},"obj":"DRI_Outcome"},{"id":"T2","span":{"begin":276,"end":606},"obj":"DRI_Approach"},{"id":"T3","span":{"begin":607,"end":789},"obj":"DRI_Outcome"},{"id":"T4","span":{"begin":790,"end":1006},"obj":"DRI_Background"},{"id":"T5","span":{"begin":1007,"end":1207},"obj":"DRI_Outcome"}],"text":"Three-dimensional virtual refocusing of fluorescence microscopy images using deep learning.\nWe demonstrate that a deep neural network can be trained to virtually refocus a two-dimensional fluorescence image onto user-defined three-dimensional (3D) surfaces within the sample. Using this method, termed Deep-Z, we imaged the neuronal activity of a Caenorhabditis elegans worm in 3D using a time sequence of fluorescence images acquired at a single focal plane, digitally increasing the depth-of-field by 20-fold without any axial scanning, additional hardware or a trade-off of imaging resolution and speed. Furthermore, we demonstrate that this approach can correct for sample drift, tilt and other aberrations, all digitally performed after the acquisition of a single fluorescence image. This framework also cross-connects different imaging modalities to each other, enabling 3D refocusing of a single wide-field fluorescence image to match confocal microscopy images acquired at different sample planes. Deep-Z has the potential to improve volumetric imaging speed while reducing challenges relating to sample drift, aberration and defocusing that are associated with standard 3D fluorescence microscopy."}
Goldhamster2_Cellosaurus
{"project":"Goldhamster2_Cellosaurus","denotations":[{"id":"T1","span":{"begin":92,"end":94},"obj":"CVCL_5M23|Cancer_cell_line|Mesocricetus auratus"},{"id":"T2","span":{"begin":112,"end":113},"obj":"CVCL_6479|Finite_cell_line|Mus musculus"},{"id":"T3","span":{"begin":170,"end":171},"obj":"CVCL_6479|Finite_cell_line|Mus musculus"},{"id":"T4","span":{"begin":310,"end":312},"obj":"CVCL_5M23|Cancer_cell_line|Mesocricetus auratus"},{"id":"T5","span":{"begin":333,"end":341},"obj":"CVCL_C410|Hybridoma|Mus musculus"},{"id":"T6","span":{"begin":345,"end":346},"obj":"CVCL_6479|Finite_cell_line|Mus musculus"},{"id":"T7","span":{"begin":387,"end":388},"obj":"CVCL_6479|Finite_cell_line|Mus musculus"},{"id":"T8","span":{"begin":389,"end":393},"obj":"CVCL_0047|Telomerase_immortalized_cell_line|Homo sapiens"},{"id":"T9","span":{"begin":438,"end":439},"obj":"CVCL_6479|Finite_cell_line|Mus musculus"},{"id":"T10","span":{"begin":503,"end":505},"obj":"CVCL_J923|Hybridoma|Mus musculus"},{"id":"T11","span":{"begin":562,"end":563},"obj":"CVCL_6479|Finite_cell_line|Mus musculus"},{"id":"T12","span":{"begin":620,"end":622},"obj":"CVCL_5M23|Cancer_cell_line|Mesocricetus auratus"},{"id":"T13","span":{"begin":761,"end":762},"obj":"CVCL_6479|Finite_cell_line|Mus musculus"},{"id":"T14","span":{"begin":895,"end":896},"obj":"CVCL_6479|Finite_cell_line|Mus musculus"},{"id":"T15","span":{"begin":1014,"end":1017},"obj":"CVCL_6758|Undefined_cell_line_type|Cricetulus griseus"},{"id":"T16","span":{"begin":1014,"end":1017},"obj":"CVCL_E689|Transformed_cell_line|Homo sapiens"}],"text":"Three-dimensional virtual refocusing of fluorescence microscopy images using deep learning.\nWe demonstrate that a deep neural network can be trained to virtually refocus a two-dimensional fluorescence image onto user-defined three-dimensional (3D) surfaces within the sample. Using this method, termed Deep-Z, we imaged the neuronal activity of a Caenorhabditis elegans worm in 3D using a time sequence of fluorescence images acquired at a single focal plane, digitally increasing the depth-of-field by 20-fold without any axial scanning, additional hardware or a trade-off of imaging resolution and speed. Furthermore, we demonstrate that this approach can correct for sample drift, tilt and other aberrations, all digitally performed after the acquisition of a single fluorescence image. This framework also cross-connects different imaging modalities to each other, enabling 3D refocusing of a single wide-field fluorescence image to match confocal microscopy images acquired at different sample planes. Deep-Z has the potential to improve volumetric imaging speed while reducing challenges relating to sample drift, aberration and defocusing that are associated with standard 3D fluorescence microscopy."}
NCBITAXON
{"project":"NCBITAXON","denotations":[{"id":"T1","span":{"begin":347,"end":369},"obj":"OrganismTaxon"},{"id":"T2","span":{"begin":999,"end":1005},"obj":"OrganismTaxon"}],"attributes":[{"id":"A1","pred":"db_id","subj":"T1","obj":"6239"},{"id":"A2","pred":"db_id","subj":"T2","obj":"106761"}],"text":"Three-dimensional virtual refocusing of fluorescence microscopy images using deep learning.\nWe demonstrate that a deep neural network can be trained to virtually refocus a two-dimensional fluorescence image onto user-defined three-dimensional (3D) surfaces within the sample. Using this method, termed Deep-Z, we imaged the neuronal activity of a Caenorhabditis elegans worm in 3D using a time sequence of fluorescence images acquired at a single focal plane, digitally increasing the depth-of-field by 20-fold without any axial scanning, additional hardware or a trade-off of imaging resolution and speed. Furthermore, we demonstrate that this approach can correct for sample drift, tilt and other aberrations, all digitally performed after the acquisition of a single fluorescence image. This framework also cross-connects different imaging modalities to each other, enabling 3D refocusing of a single wide-field fluorescence image to match confocal microscopy images acquired at different sample planes. Deep-Z has the potential to improve volumetric imaging speed while reducing challenges relating to sample drift, aberration and defocusing that are associated with standard 3D fluorescence microscopy."}