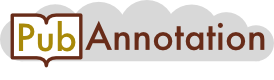
PMC:7796058 / 17937-23629
Annnotations
LitCovid-PubTator
{"project":"LitCovid-PubTator","denotations":[{"id":"143","span":{"begin":541,"end":555},"obj":"Species"},{"id":"152","span":{"begin":2624,"end":2630},"obj":"Species"},{"id":"153","span":{"begin":932,"end":940},"obj":"Disease"},{"id":"154","span":{"begin":1105,"end":1114},"obj":"Disease"},{"id":"155","span":{"begin":1311,"end":1320},"obj":"Disease"},{"id":"156","span":{"begin":1591,"end":1600},"obj":"Disease"},{"id":"157","span":{"begin":2210,"end":2218},"obj":"Disease"},{"id":"158","span":{"begin":2761,"end":2770},"obj":"Disease"},{"id":"159","span":{"begin":2839,"end":2847},"obj":"Disease"},{"id":"161","span":{"begin":3719,"end":3728},"obj":"Disease"},{"id":"163","span":{"begin":5272,"end":5278},"obj":"Gene"}],"attributes":[{"id":"A143","pred":"tao:has_database_id","subj":"143","obj":"Tax:2697049"},{"id":"A152","pred":"tao:has_database_id","subj":"152","obj":"Tax:9606"},{"id":"A153","pred":"tao:has_database_id","subj":"153","obj":"MESH:C000657245"},{"id":"A154","pred":"tao:has_database_id","subj":"154","obj":"MESH:D007239"},{"id":"A155","pred":"tao:has_database_id","subj":"155","obj":"MESH:D007239"},{"id":"A156","pred":"tao:has_database_id","subj":"156","obj":"MESH:D007239"},{"id":"A157","pred":"tao:has_database_id","subj":"157","obj":"MESH:C000657245"},{"id":"A158","pred":"tao:has_database_id","subj":"158","obj":"MESH:D007239"},{"id":"A159","pred":"tao:has_database_id","subj":"159","obj":"MESH:C000657245"},{"id":"A161","pred":"tao:has_database_id","subj":"161","obj":"MESH:D006973"},{"id":"A163","pred":"tao:has_database_id","subj":"163","obj":"Gene:59286"}],"namespaces":[{"prefix":"Tax","uri":"https://www.ncbi.nlm.nih.gov/taxonomy/"},{"prefix":"MESH","uri":"https://id.nlm.nih.gov/mesh/"},{"prefix":"Gene","uri":"https://www.ncbi.nlm.nih.gov/gene/"},{"prefix":"CVCL","uri":"https://web.expasy.org/cellosaurus/CVCL_"}],"text":"2.3. Interior Space Modelling Using IndoorGML\nIndoor spaces differ from outdoor spaces in many aspects. Basic concepts, data models, and standards of spatial information need to be redefined in order to meet the requirements of indoor spatial applications. The proper representation of indoor spaces is a key issue for indoor spatial information modelling and analytics. In recent years, the topic of 3D geospatial indoor modelling has been the focus of attention for location-based services and indoor navigation [42,43,44]. As the risk of COVID-19 virus transmission is higher in indoor environments, indoor space modelling is an important topic that facilitates the interoperability between different indoor and outdoor data collection methods and builds a consistent framework for collaborative research and development of the IoCT.\nAggregating different sensor observations for each room is essential for estimating the room’s COVID-19 risk and cleaning requirements. The visualization of Interior Space Risk State is another task which requires interior modelling. In order to represent the risk of infection and to identify which specific areas of a building require the most cleaning, the status of individual floors should be able to be viewed separately from other floors in the building. The risk of infection for various parts of each floor should be represented in a map with different ways of representing the data that is necessary for determining the risk in each area. Moreover, in order to visualize trajectories for contact tracing and to quickly identify the location of infection spreading behavior within an indoor space, the buildings should be visible as a 3D construct on the map. Therefore, in order to analyze the IoCT multi-sensors system and visualize it in indoor scenarios, an interoperable 3D building modelling standard, such as the “CityGML” Level of Detail 4 [45], “OGC IndoorGML” [46], building construction standards (e.g., “Building Information Modelling” (BIM), or “Industry Foundation Classes” (IFC) [47]), is necessary. The main concern for using those models is their fit and how often they are updated. Construction features of indoor spaces are not a major focus of COVID-19 workplace reopening scenarios. Instead, the aggregation of sensors in each room, and the connectivity between the rooms, is fundamental for risk assessment and tracing. Thus, the OGC IndoorGML is used for the IoCT indoor modelling. According to Ryoo et al. [43], the OGC IndoorGML can be used more effectively than CityGML or any other geometric representations of space for analyzing the trajectories of people inside buildings. This allows for more accurate appraisal of the types of intersection of trajectories, contact, and exposure for infection risk evaluation. Applications such as cleaning risk assessments for COVID-19 workplace reopenings that need to operate efficiently together with indoor scales, various sensors, and objects that are moving and changing over time would benefit from using the OGC IndoorGML. 3D geometry can be included in an IndoorGML document, and the overlap with other standards (e.g., OGC CityGML) can be addressed by adding external references.\nThere were no specific standards in the field of indoor geospatial modelling until the OGC standard IndoorGML was introduced in 2014. The OGC IndoorGML intentionally focused on modelling indoor spaces using connected dual graphs for navigation purposes whilst considering various semantics [46]. OGC IndoorGML standard specifies an open data model and Extensible Markup Language (XML) schema for indoor spatial information [46]. Indoor space is comprised of connected constructs such as rooms, corridors, stairs, and elevators, all of which can be considered “Cells”. This sets it apart from other standards in the field of 3D modelling, such as CityGML or IFC, as they model the building features (e.g., walls, windows) instead of the indoor space itself. They also do not consider the connectivity and semantics of indoor spaces.\nAs shown in Figure 3, the nodes of the IndoorGML graph in this paper are considered the smallest organizational or structural units for the building and are called Cells [19]. Every Cell has an identifier (e.g., room number) and a location (x, y, z) to provide more precise location details. Cells are connected and have a common boundary with other cells but do not overlap with them. “Geometric” features and “Topological” relationships, such as adjacency and connectivity, amongst indoor cells can be defined by an IndoorGML graph [48]. The topological relationships in IndoorGML are explicitly described using the xlink concept of XML provided by Geography Markup Language (GML) and the referencing is realized with the use of href attributes (xlink:href). Semantics are also an important characteristic of the Cells in the IndoorGML. “Semantics” allow us to define cells which can be important for cleaning risk assessment. For example, the most commonly used areas are public rooms, corridors, and doors, and thus present higher risk. For this paper, an indoor space is represented as a topographic cellular space comprised of rooms, corridors, and stairs. At the same time, it is also represented as different cellular spaces with beacon or camera coverage Cells.\nEach semantic interpretation layer creates a different indoor model, and each model forms a separate dual graph layer (e.g., connectivity, sensor) for the same cellular space. This multi-layered space model (Figure 3), is an aggregation of the space layers and inter-layer connections or relations. The Indoor GML for the implementation of the structure space model is shown in Figure 4."}
LitCovid-sentences
{"project":"LitCovid-sentences","denotations":[{"id":"T107","span":{"begin":0,"end":4},"obj":"Sentence"},{"id":"T108","span":{"begin":5,"end":45},"obj":"Sentence"},{"id":"T109","span":{"begin":46,"end":103},"obj":"Sentence"},{"id":"T110","span":{"begin":104,"end":256},"obj":"Sentence"},{"id":"T111","span":{"begin":257,"end":370},"obj":"Sentence"},{"id":"T112","span":{"begin":371,"end":525},"obj":"Sentence"},{"id":"T113","span":{"begin":526,"end":836},"obj":"Sentence"},{"id":"T114","span":{"begin":837,"end":972},"obj":"Sentence"},{"id":"T115","span":{"begin":973,"end":1070},"obj":"Sentence"},{"id":"T116","span":{"begin":1071,"end":1298},"obj":"Sentence"},{"id":"T117","span":{"begin":1299,"end":1485},"obj":"Sentence"},{"id":"T118","span":{"begin":1486,"end":1705},"obj":"Sentence"},{"id":"T119","span":{"begin":1706,"end":2060},"obj":"Sentence"},{"id":"T120","span":{"begin":2061,"end":2145},"obj":"Sentence"},{"id":"T121","span":{"begin":2146,"end":2249},"obj":"Sentence"},{"id":"T122","span":{"begin":2250,"end":2387},"obj":"Sentence"},{"id":"T123","span":{"begin":2388,"end":2450},"obj":"Sentence"},{"id":"T124","span":{"begin":2451,"end":2648},"obj":"Sentence"},{"id":"T125","span":{"begin":2649,"end":2787},"obj":"Sentence"},{"id":"T126","span":{"begin":2788,"end":3042},"obj":"Sentence"},{"id":"T127","span":{"begin":3043,"end":3201},"obj":"Sentence"},{"id":"T128","span":{"begin":3202,"end":3335},"obj":"Sentence"},{"id":"T129","span":{"begin":3336,"end":3497},"obj":"Sentence"},{"id":"T130","span":{"begin":3498,"end":3630},"obj":"Sentence"},{"id":"T131","span":{"begin":3631,"end":3769},"obj":"Sentence"},{"id":"T132","span":{"begin":3770,"end":3958},"obj":"Sentence"},{"id":"T133","span":{"begin":3959,"end":4033},"obj":"Sentence"},{"id":"T134","span":{"begin":4034,"end":4209},"obj":"Sentence"},{"id":"T135","span":{"begin":4210,"end":4325},"obj":"Sentence"},{"id":"T136","span":{"begin":4326,"end":4573},"obj":"Sentence"},{"id":"T137","span":{"begin":4574,"end":4794},"obj":"Sentence"},{"id":"T138","span":{"begin":4795,"end":4962},"obj":"Sentence"},{"id":"T139","span":{"begin":4963,"end":5074},"obj":"Sentence"},{"id":"T140","span":{"begin":5075,"end":5196},"obj":"Sentence"},{"id":"T141","span":{"begin":5197,"end":5304},"obj":"Sentence"},{"id":"T142","span":{"begin":5305,"end":5480},"obj":"Sentence"},{"id":"T143","span":{"begin":5481,"end":5603},"obj":"Sentence"},{"id":"T144","span":{"begin":5604,"end":5692},"obj":"Sentence"}],"namespaces":[{"prefix":"_base","uri":"http://pubannotation.org/ontology/tao.owl#"}],"text":"2.3. Interior Space Modelling Using IndoorGML\nIndoor spaces differ from outdoor spaces in many aspects. Basic concepts, data models, and standards of spatial information need to be redefined in order to meet the requirements of indoor spatial applications. The proper representation of indoor spaces is a key issue for indoor spatial information modelling and analytics. In recent years, the topic of 3D geospatial indoor modelling has been the focus of attention for location-based services and indoor navigation [42,43,44]. As the risk of COVID-19 virus transmission is higher in indoor environments, indoor space modelling is an important topic that facilitates the interoperability between different indoor and outdoor data collection methods and builds a consistent framework for collaborative research and development of the IoCT.\nAggregating different sensor observations for each room is essential for estimating the room’s COVID-19 risk and cleaning requirements. The visualization of Interior Space Risk State is another task which requires interior modelling. In order to represent the risk of infection and to identify which specific areas of a building require the most cleaning, the status of individual floors should be able to be viewed separately from other floors in the building. The risk of infection for various parts of each floor should be represented in a map with different ways of representing the data that is necessary for determining the risk in each area. Moreover, in order to visualize trajectories for contact tracing and to quickly identify the location of infection spreading behavior within an indoor space, the buildings should be visible as a 3D construct on the map. Therefore, in order to analyze the IoCT multi-sensors system and visualize it in indoor scenarios, an interoperable 3D building modelling standard, such as the “CityGML” Level of Detail 4 [45], “OGC IndoorGML” [46], building construction standards (e.g., “Building Information Modelling” (BIM), or “Industry Foundation Classes” (IFC) [47]), is necessary. The main concern for using those models is their fit and how often they are updated. Construction features of indoor spaces are not a major focus of COVID-19 workplace reopening scenarios. Instead, the aggregation of sensors in each room, and the connectivity between the rooms, is fundamental for risk assessment and tracing. Thus, the OGC IndoorGML is used for the IoCT indoor modelling. According to Ryoo et al. [43], the OGC IndoorGML can be used more effectively than CityGML or any other geometric representations of space for analyzing the trajectories of people inside buildings. This allows for more accurate appraisal of the types of intersection of trajectories, contact, and exposure for infection risk evaluation. Applications such as cleaning risk assessments for COVID-19 workplace reopenings that need to operate efficiently together with indoor scales, various sensors, and objects that are moving and changing over time would benefit from using the OGC IndoorGML. 3D geometry can be included in an IndoorGML document, and the overlap with other standards (e.g., OGC CityGML) can be addressed by adding external references.\nThere were no specific standards in the field of indoor geospatial modelling until the OGC standard IndoorGML was introduced in 2014. The OGC IndoorGML intentionally focused on modelling indoor spaces using connected dual graphs for navigation purposes whilst considering various semantics [46]. OGC IndoorGML standard specifies an open data model and Extensible Markup Language (XML) schema for indoor spatial information [46]. Indoor space is comprised of connected constructs such as rooms, corridors, stairs, and elevators, all of which can be considered “Cells”. This sets it apart from other standards in the field of 3D modelling, such as CityGML or IFC, as they model the building features (e.g., walls, windows) instead of the indoor space itself. They also do not consider the connectivity and semantics of indoor spaces.\nAs shown in Figure 3, the nodes of the IndoorGML graph in this paper are considered the smallest organizational or structural units for the building and are called Cells [19]. Every Cell has an identifier (e.g., room number) and a location (x, y, z) to provide more precise location details. Cells are connected and have a common boundary with other cells but do not overlap with them. “Geometric” features and “Topological” relationships, such as adjacency and connectivity, amongst indoor cells can be defined by an IndoorGML graph [48]. The topological relationships in IndoorGML are explicitly described using the xlink concept of XML provided by Geography Markup Language (GML) and the referencing is realized with the use of href attributes (xlink:href). Semantics are also an important characteristic of the Cells in the IndoorGML. “Semantics” allow us to define cells which can be important for cleaning risk assessment. For example, the most commonly used areas are public rooms, corridors, and doors, and thus present higher risk. For this paper, an indoor space is represented as a topographic cellular space comprised of rooms, corridors, and stairs. At the same time, it is also represented as different cellular spaces with beacon or camera coverage Cells.\nEach semantic interpretation layer creates a different indoor model, and each model forms a separate dual graph layer (e.g., connectivity, sensor) for the same cellular space. This multi-layered space model (Figure 3), is an aggregation of the space layers and inter-layer connections or relations. The Indoor GML for the implementation of the structure space model is shown in Figure 4."}