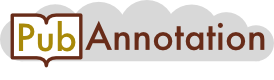
PMC:7786642 / 12723-15180
Annnotations
{"target":"https://pubannotation.org/docs/sourcedb/PMC/sourceid/7786642","sourcedb":"PMC","sourceid":"7786642","source_url":"https://www.ncbi.nlm.nih.gov/pmc/7786642","text":"2.5 Statistical methods\nWe specified Bayesian hierarchical Poisson log-linear models to investigate the association of COVID-19 deaths and NO2 and PM2.5 independently. The LSOA specific standardised mortality ratio is known to be an unstable estimator with high variance when the number of expected deaths is small. To overcome this problem, we used a well-established hierarchical framework, specifying spatially structured and unstructured random effects, so that the model borrows strength from the other areas across the entire study region, as well as from the neighbouring ones (Best et al., 2005, Wakefield et al., 2000, Wakefield, 2006). We model these random effects using a re-parametrisation of the Besag-York-Molliè conditional autoregressive prior distribution (Besag et al., 1991, Simpson et al., 2017). We fitted four models including: 1) each pollutant (model 1), 2) each pollutant and the spatial autocorrelation term (model 2), 3) each pollutant and all confounders (model 3) and 4) each pollutant, the spatial autocorrelation term and all confounders (model 4). All models were adjusted for age, sex and ethnicity using indirect standardisation; we used the English population as the standard population to calculate the rates. We do not report results from the joint analysis including both pollutants since they are highly correlated (Supplemental Material Figure S5).\nIn order to propagate the uncertainty resulted from the sampling we used for the downscaling, we fitted the models over 100 downscaled samples and then performed Bayesian model averaging to combine the estimates (Gómez-Rubio et al. 2020). We performed a complete case analysis since for only 1.1% of the cases information about age, sex and ethnicity is missing. We report results as posterior median of % increase in mortality risk for every 1 μg/m3 increase in the air-pollutants, 95% credible intervals (CrI) and posterior probability that the estimated effect is positive. We also report posterior median of spatial mortality relative risks (exponential of the spatial autocorrelation term) and posterior probabilities that the spatial relative risks are larger than 1.\nThe mathematical formulation of the models and prior specifications are given in the Supplemental Material Text S1.2.\nAll models were fitted in INLA (Rue et al. 2009). Covariate data and code for running the analysis are available at https://github.com/gkonstantinoudis/COVID19AirpollutionEn.","divisions":[{"label":"label","span":{"begin":0,"end":3}},{"label":"title","span":{"begin":5,"end":24}},{"label":"p","span":{"begin":25,"end":1390}},{"label":"p","span":{"begin":1391,"end":2164}},{"label":"p","span":{"begin":2165,"end":2282}}],"tracks":[{"project":"LitCovid-PubTator","denotations":[{"id":"130","span":{"begin":120,"end":128},"obj":"Disease"},{"id":"131","span":{"begin":129,"end":135},"obj":"Disease"},{"id":"132","span":{"begin":200,"end":209},"obj":"Disease"},{"id":"133","span":{"begin":300,"end":306},"obj":"Disease"},{"id":"136","span":{"begin":1809,"end":1818},"obj":"Disease"},{"id":"137","span":{"begin":2011,"end":2020},"obj":"Disease"},{"id":"139","span":{"begin":2277,"end":2281},"obj":"Gene"}],"attributes":[{"id":"A130","pred":"tao:has_database_id","subj":"130","obj":"MESH:C000657245"},{"id":"A131","pred":"tao:has_database_id","subj":"131","obj":"MESH:D003643"},{"id":"A132","pred":"tao:has_database_id","subj":"132","obj":"MESH:D003643"},{"id":"A133","pred":"tao:has_database_id","subj":"133","obj":"MESH:D003643"},{"id":"A136","pred":"tao:has_database_id","subj":"136","obj":"MESH:D003643"},{"id":"A137","pred":"tao:has_database_id","subj":"137","obj":"MESH:D003643"},{"id":"A139","pred":"tao:has_database_id","subj":"139","obj":"Gene:6268"},{"subj":"130","pred":"source","obj":"LitCovid-PubTator"},{"subj":"131","pred":"source","obj":"LitCovid-PubTator"},{"subj":"132","pred":"source","obj":"LitCovid-PubTator"},{"subj":"133","pred":"source","obj":"LitCovid-PubTator"},{"subj":"136","pred":"source","obj":"LitCovid-PubTator"},{"subj":"137","pred":"source","obj":"LitCovid-PubTator"},{"subj":"139","pred":"source","obj":"LitCovid-PubTator"}],"namespaces":[{"prefix":"Tax","uri":"https://www.ncbi.nlm.nih.gov/taxonomy/"},{"prefix":"MESH","uri":"https://id.nlm.nih.gov/mesh/"},{"prefix":"Gene","uri":"https://www.ncbi.nlm.nih.gov/gene/"},{"prefix":"CVCL","uri":"https://web.expasy.org/cellosaurus/CVCL_"}]},{"project":"LitCovid-sentences","denotations":[{"id":"T103","span":{"begin":0,"end":24},"obj":"Sentence"},{"id":"T104","span":{"begin":25,"end":168},"obj":"Sentence"},{"id":"T105","span":{"begin":169,"end":316},"obj":"Sentence"},{"id":"T106","span":{"begin":317,"end":646},"obj":"Sentence"},{"id":"T107","span":{"begin":647,"end":818},"obj":"Sentence"},{"id":"T108","span":{"begin":819,"end":851},"obj":"Sentence"},{"id":"T109","span":{"begin":852,"end":1081},"obj":"Sentence"},{"id":"T110","span":{"begin":1082,"end":1247},"obj":"Sentence"},{"id":"T111","span":{"begin":1248,"end":1390},"obj":"Sentence"},{"id":"T112","span":{"begin":1391,"end":1622},"obj":"Sentence"},{"id":"T113","span":{"begin":1623,"end":1629},"obj":"Sentence"},{"id":"T114","span":{"begin":1630,"end":1753},"obj":"Sentence"},{"id":"T115","span":{"begin":1754,"end":1967},"obj":"Sentence"},{"id":"T116","span":{"begin":1968,"end":2164},"obj":"Sentence"},{"id":"T117","span":{"begin":2165,"end":2282},"obj":"Sentence"},{"id":"T118","span":{"begin":2283,"end":2325},"obj":"Sentence"},{"id":"T119","span":{"begin":2326,"end":2332},"obj":"Sentence"},{"id":"T120","span":{"begin":2333,"end":2457},"obj":"Sentence"}],"namespaces":[{"prefix":"_base","uri":"http://pubannotation.org/ontology/tao.owl#"}],"attributes":[{"subj":"T103","pred":"source","obj":"LitCovid-sentences"},{"subj":"T104","pred":"source","obj":"LitCovid-sentences"},{"subj":"T105","pred":"source","obj":"LitCovid-sentences"},{"subj":"T106","pred":"source","obj":"LitCovid-sentences"},{"subj":"T107","pred":"source","obj":"LitCovid-sentences"},{"subj":"T108","pred":"source","obj":"LitCovid-sentences"},{"subj":"T109","pred":"source","obj":"LitCovid-sentences"},{"subj":"T110","pred":"source","obj":"LitCovid-sentences"},{"subj":"T111","pred":"source","obj":"LitCovid-sentences"},{"subj":"T112","pred":"source","obj":"LitCovid-sentences"},{"subj":"T113","pred":"source","obj":"LitCovid-sentences"},{"subj":"T114","pred":"source","obj":"LitCovid-sentences"},{"subj":"T115","pred":"source","obj":"LitCovid-sentences"},{"subj":"T116","pred":"source","obj":"LitCovid-sentences"},{"subj":"T117","pred":"source","obj":"LitCovid-sentences"},{"subj":"T118","pred":"source","obj":"LitCovid-sentences"},{"subj":"T119","pred":"source","obj":"LitCovid-sentences"},{"subj":"T120","pred":"source","obj":"LitCovid-sentences"}]}],"config":{"attribute types":[{"pred":"source","value type":"selection","values":[{"id":"LitCovid-PubTator","color":"#ec93e4","default":true},{"id":"LitCovid-sentences","color":"#93ecda"}]}]}}