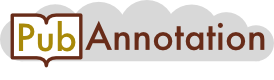
PMC:7782580 / 33213-34381
Annnotations
LitCovid-sentences
{"project":"LitCovid-sentences","denotations":[{"id":"T255","span":{"begin":0,"end":233},"obj":"Sentence"},{"id":"T256","span":{"begin":234,"end":365},"obj":"Sentence"},{"id":"T257","span":{"begin":366,"end":455},"obj":"Sentence"},{"id":"T258","span":{"begin":456,"end":645},"obj":"Sentence"},{"id":"T259","span":{"begin":646,"end":792},"obj":"Sentence"},{"id":"T260","span":{"begin":793,"end":1036},"obj":"Sentence"},{"id":"T261","span":{"begin":1037,"end":1168},"obj":"Sentence"}],"namespaces":[{"prefix":"_base","uri":"http://pubannotation.org/ontology/tao.owl#"}],"text":"We adopted a knowledge distillation method in the training phrase; a small model (called a student network) was trained to mimic the ensemble of multiple models (called teacher networks) to obtain a small model with high performance. In the distillation process, knowledge was transferred from the teacher networks to the student network to minimize knowledge loss. The target was the output of the teacher networks; these outputs were called soft labels. The student network also learned from the ground-truth labels (also called hard labels), thereby minimizing the knowledge loss from the student networks, whose targets were the hard labels. Therefore, the overall loss function of the student network incorporated both knowledge distillation and knowledge loss from the student networks. After the student network had been well-trained, the task of the teacher networks was complete, and the student model could be used on a regular computer with a fast speed, which is suitable for hospitals without extensive computing resources. As a result of the knowledge distillation method, the CNNCF achieved high performance with a few parameters in the teacher network."}