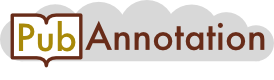
PMC:7782580 / 27187-28459
Annnotations
LitCovid-PubTator
{"project":"LitCovid-PubTator","denotations":[{"id":"205","span":{"begin":229,"end":237},"obj":"Disease"},{"id":"206","span":{"begin":587,"end":595},"obj":"Disease"},{"id":"207","span":{"begin":815,"end":823},"obj":"Disease"}],"attributes":[{"id":"A205","pred":"tao:has_database_id","subj":"205","obj":"MESH:C000657245"},{"id":"A206","pred":"tao:has_database_id","subj":"206","obj":"MESH:C000657245"},{"id":"A207","pred":"tao:has_database_id","subj":"207","obj":"MESH:C000657245"}],"namespaces":[{"prefix":"Tax","uri":"https://www.ncbi.nlm.nih.gov/taxonomy/"},{"prefix":"MESH","uri":"https://id.nlm.nih.gov/mesh/"},{"prefix":"Gene","uri":"https://www.ncbi.nlm.nih.gov/gene/"},{"prefix":"CVCL","uri":"https://web.expasy.org/cellosaurus/CVCL_"}],"text":"In clinical practice, the diagnostic decision of a clinician relies on the identification of the SAs in the medical images by radiologists. The statistical results show that the performance of the CNNCF for the identification of COVID-19 is as good as that of the experts. A comparison consisting of two parts was performed to evaluate the discriminatory ability of the CNNCF. In the first part, we used Grad-CAM, which is a non-intrusive method to extract the salient features in medical images, to create a heatmap of the CNNCF result. Figure 2b shows the heatmaps of four examples of COVID-19 cases in the X-data and CT-data. In the second part, we used density-based spatial clustering of applications with noise (DBSCAN) to calculate the center pixel coordinates (CPC) of the salient features corresponding to COVID-19. All CPCs were normalized to a range of 0 to 1. Subsequently, we used a significance test (ST)42 to analyze the relationship between the CPC of the CNNCF output and the CPC annotated by the experts. A good performance was obtained, with a mean square error (MSE) of 0.0108, a mean absolute error (MAE) of 0.0722, a root mean squared error (RMSE) of 0.1040, a correlation coefficient (r) of 0.9761, and a coefficient of determination (R2) of 0.8801."}
LitCovid-sentences
{"project":"LitCovid-sentences","denotations":[{"id":"T208","span":{"begin":0,"end":139},"obj":"Sentence"},{"id":"T209","span":{"begin":140,"end":272},"obj":"Sentence"},{"id":"T210","span":{"begin":273,"end":376},"obj":"Sentence"},{"id":"T211","span":{"begin":377,"end":537},"obj":"Sentence"},{"id":"T212","span":{"begin":538,"end":628},"obj":"Sentence"},{"id":"T213","span":{"begin":629,"end":824},"obj":"Sentence"},{"id":"T214","span":{"begin":825,"end":871},"obj":"Sentence"},{"id":"T215","span":{"begin":872,"end":1022},"obj":"Sentence"},{"id":"T216","span":{"begin":1023,"end":1272},"obj":"Sentence"}],"namespaces":[{"prefix":"_base","uri":"http://pubannotation.org/ontology/tao.owl#"}],"text":"In clinical practice, the diagnostic decision of a clinician relies on the identification of the SAs in the medical images by radiologists. The statistical results show that the performance of the CNNCF for the identification of COVID-19 is as good as that of the experts. A comparison consisting of two parts was performed to evaluate the discriminatory ability of the CNNCF. In the first part, we used Grad-CAM, which is a non-intrusive method to extract the salient features in medical images, to create a heatmap of the CNNCF result. Figure 2b shows the heatmaps of four examples of COVID-19 cases in the X-data and CT-data. In the second part, we used density-based spatial clustering of applications with noise (DBSCAN) to calculate the center pixel coordinates (CPC) of the salient features corresponding to COVID-19. All CPCs were normalized to a range of 0 to 1. Subsequently, we used a significance test (ST)42 to analyze the relationship between the CPC of the CNNCF output and the CPC annotated by the experts. A good performance was obtained, with a mean square error (MSE) of 0.0108, a mean absolute error (MAE) of 0.0722, a root mean squared error (RMSE) of 0.1040, a correlation coefficient (r) of 0.9761, and a coefficient of determination (R2) of 0.8801."}