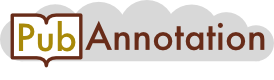
PMC:7543267 / 14231-29988
Annnotations
LitCovid-PD-FMA-UBERON
{"project":"LitCovid-PD-FMA-UBERON","denotations":[{"id":"T3","span":{"begin":7297,"end":7305},"obj":"Body_part"},{"id":"T4","span":{"begin":11008,"end":11016},"obj":"Body_part"}],"attributes":[{"id":"A3","pred":"fma_id","subj":"T3","obj":"http://purl.org/sig/ont/fma/fma14542"},{"id":"A4","pred":"fma_id","subj":"T4","obj":"http://purl.org/sig/ont/fma/fma14542"}],"text":"Method\n\nParticipants and Recruitment\nSamples of Australian (N = 495, 50.1% female) and U.S. (N = 701, 48.9% female) residents were recruited via an online research panel company. To be eligible for inclusion, participants needed to be aged 18 years or older and not subject to formal quarantine for COVID-19. Participants were also screened for age, gender, and geographical region and quotas imposed during recruitment to ensure that the final samples closely matched the national distributions for these characteristics in each country. Data were collected between April 1 and May 6, 2020. All participants in the Australian sample were subject to a national “shelter-in-place” order issued by the federal government. However, issuance of orders in the USA was devolved to state governments resulting in some variations. The vast majority of participants in the U.S. sample (n = 610, 87.0%) were subject to “shelter-in-place” orders for the duration of the study. However, some states did not impose “shelter-in-place” orders at all (Arkansas, Iowa, Nebraska, North Dakota, South Dakota, Utah, and Wyoming), so a minority of participants in the U.S. sample (n = 37, 5.3%) were never subject to an order. Furthermore, in some cases in the U.S. sample (n = 47, 6.7%), “shelter-in-place” orders had been lifted prior to follow-up data collection. However, among the states in the USA that did not have “shelter-in-place” orders, or lifted their orders during the study, all issued social distancing guidelines and encouraged the population to follow those guidelines. Baseline sample characteristics are presented in Table 1.\nTable 1. Sample characteristics and descriptive statistics for study variables at baseline and at 1 week follow-up\nVariable Australia sample U.S. sample\nBaseline Follow-up Baseline Follow-up\nParticipants 495 365 701 440\nAge, M years (SD) 47.09 (17.11) 49.78 (16.89) 45.55 (17.40) 51.77 (16.26)\nGender, n (%)a\n Female 252 (51.1) 182 (50.1) 352 (48.9) 205 (46.6)\n Male 241 (48.9) 181 (49.9) 341 (50.5) 231 (52.5)\n Not specified/prefer not to answer 0 (0.0) 0 (0.0) 4 (0.6) 4 (0.9)\nEmployment status, n (%)b\n Currently unemployed/full-time caregiver 231 (46.7) 180 (49.3) 330 (47.3) 216 (49.5)\n Part-time/casual employed 97 (19.6) 65 (17.8) 106 (15.2) 60 (13.8)\n Currently employed full time 140 (28.3) 104 (28.5) 233 (33.4) 147 (33.7)\n Leave without pay/furloughed 27 (5.5) 16 (4.4) 28 (4.0) 13 (3.0)\nMarital status, n (%)c\n Married 184 (37.2) 146 (40.0) 300 (43.0) 224 (51.4)\n Widowed 8 (1.6) 7 (1.9) 22 (3.2) 18 (4.1)\n Separated/divorced 53 (10.7) 39 (10.7) 69 (9.9) 47 (10.8)\n Never married 160 (32.3) 103 (28.2) 255 (36.6) 126(28.9)\n Married de facto 90 (18.2) 70 (19.2) 51 (7.3) 21 (4.8)\nEthnicity, n (%)d\n Black 3 (0.6) 1 (0.3) 52 (7.5) 26 (6.0)\n Caucasian/White 392 (79.2) 304 (83.3) 566 (81.2) 376 (86.2)\n Asian (South-East Asia/South Asia) 71 (14.3) 43 (11.8) 39 (5.6) 24 (5.5)\n Middle-Eastern 6 (1.2) 3 (0.8) 1 (0.1) 0 (0.0)\n Other 13 (2.6) 6 (1.6) 27 (3.9) 8 (1.8)\n Prefer not to answer 10 (2.0) 8 (2.2) 12 (1.7) 2 (0.5)\nIncome, n (%)e\n Zero income 8 (1.7) 4 (1.2) 31 (4.4) 19 (4.4)\n $1–$199 ($1–$10,399) 9 (2.0) 6 (1.8) 40 (5.7) 24 (5.5)\n $200–$299 ($10,400–$15,599) 12 (2.6) 8 (2.4) 34 (4.9) 23 (5.3)\n $300–$399 ($15,600–$20,799) 19 (4.1) 12 (3.6) 38 (5.5) 23 (5.3)\n $400–$599 ($20,800–$31,199) 42 (9.2) 33 (9.9) 62 (8.9) 33 (7.6)\n $600–$799 ($31,200–$41,599) 57 (12.4) 42 (12.6) 61 (8.8) 39 (8.9)\n $800–$999 ($41,600–$51,999) 45 (9.8) 31 (9.3) 68 (9.8) 46 (10.6)\n $1,000–$1,249 ($52,000–$64,999) 39 (8.5) 32 (9.6) 48 (6.9) 38 (8.7)\n $1,250–$1,499 ($65,000–$77,999) 28 (6.1) 22 (6.6) 59 (8.5) 41 (9.4)\n $1,500–$1,999 ($78,000–$103,999) 72 (15.7) 50 (15.0) 72 (10.3) 48 (11.0)\n $2,000 or more ($104,000 or more) 81 (17.6) 62 (18.6) 108 (15.5) 74 (17.0)\n Prefer not to answer 47 (10.2) 32 (9.6) 76 (10.9) 28 (6.4)\nEducation level, n (%)\n Completed junior/lower/primary school 18 (3.6) 17 (4.7) 6 (0.9) 0 (0.0)\n Completed senior/high/secondary school 133 (26.9) 98 (26.8) 265 (37.8) 132 (30.0)\n Postschool vocational qualification/diploma 147 (29.7) 111 (30.4) 138 (19.7) 94 (21.4)\n Undergraduate university degree 131 (26.5) 93 (25.5) 214 (30.5) 159 (36.1)\n Postgraduate university degree 66 (13.3) 46 (12.6) 78 (11.1) 55 (12.5)\nSD standard deviation.\naTwo participants in the Australian sample did not report their gender and four participants in the U.S. sample did not report their gender.\nbFour participants in the U.S. sample did not report their employment status.\ncFour participants in the U.S. sample did not report their marital status.\ndFour participants in the U.S. sample did not report their ethnicity.\neThirty-one participants in the Australian sample did not report their income and four participants in the U.S. sample did not report their income.\n\nDesign and Procedure\nThe study adopted a prospective correlational design with self-report measures of social cognition constructs from the proposed integrated model, intention, and past social distancing behavior administered at an initial data collection occasion in a survey administered using the Qualtrics online survey tool. Social cognition measures included the theory of planned behavior (attitude, subjective norm, and PBC), moral norm, anticipated regret, action planning, and habit constructs. Participants were informed that they were participating in a survey on their social distancing behavior and provided with information outlining study requirements. They were required to provide informed consent before proceeding with the survey. Participants were also provided with instructions on how to complete study measures and a definition of the target behavior: “The following survey will ask about your beliefs and attitudes about ‘social distancing’. What do we mean by social distancing? Social distancing (also known as ‘physical distancing’) is deliberately increasing the physical space between people to avoid spreading illness. The World Health Organization and other world leading health authorities suggest that you should maintain at least a 1–2 meter (3–6 feet) distance from other people to lessen the chances of getting infected with COVID-19. When answering the questions in this survey, think about your social distancing behavior (i.e., maintaining at least a 1–2 m (3–6 ft) distance from other people).” One week later, participants were re-contacted by the panel company and asked to self-report their habit and social distancing behavior over the previous week using the same measures used at the initial data collection occasion. Participants received a fixed sum of money for their participation based on expected completion time consistent with the panel company’s published rates. Approval for study procedures was granted prior to data collection from the Griffith University Human Research Ethics Committee.\n\nMeasures\nStudy measures were multi-item self-report measures of constructs based on published guidelines and measures used in previous studies [13, 29, 30]. Participants provided their responses on scales with seven-point response options. Complete study measures are provided in Supplementary Appendix A.\n\nSocial cognition constructs\nMulti-item measures of attitude, subjective norm, PBC, moral norm, anticipated regret, and action planning were developed according to published guidelines [13, 29]. Each measure made explicit reference to the target behavior of social distancing, and participants were reminded of the definition of social distancing before completing the measures.\n\nIntention\nParticipants’ intention to participate in social distancing behavior over the next week was measured using a scale developed according to published guidelines [31].\n\nHabit\nHabit was measured at both time points using the behavioral automaticity items of Verplanken and Orbell’s self-report habit index [25]. The measure measures individuals’ reflections on the extent to which the behavior is experienced as automatic and enacted without thought.\n\nPast behavior and behavior\nParticipants self-reported their participation in social distancing behavior to minimize the transmission of the SARS-CoV-2 virus that causes COVID-19. The measure comprised two items prompting participants to report their frequency of social distancing behavior in the previous week. This is based on previously used self-report behavioral measures that have demonstrated concurrent validity with non-self-report measures in other behavioral contexts [32].\n\nDemographic variables\nParticipants self-reported their age in years, gender, employment status (currently unemployed/full-time caregiver, currently full-time employed, part-time employed, or on leave without pay/furloughed), marital status (married, widowed, separated/divorced, never married, or in a de factor relationship), annual household income stratified by 11 income levels based on Australian and U.S. national averages, highest level of formal education (completed junior/lower/primary school, completed senior/high/secondary school, postschool vocational qualification/diploma, further education diploma, undergraduate university degree, or postgraduate university degree), and ethnicity (Black, Caucasian/White, Asian, or Middle-eastern). Binary income (low income vs. middle/high income), highest education level (completed school education only vs. completed postschool education), and ethnicity (Caucasian/White vs. non-White) variables were computed for use in subsequent analyses.\n\nData Analysis\nHypothesized relations among the integrated model constructs were tested in the Australian and U.S. samples separately using variance-based structural equation modeling implemented in the WARP 7.0 analysis package [33]. Model parameters and standard errors (SEs) were computed using the “Stable3” estimation method, which has been shown to provide the most precise parameter estimates in complex structural models in smaller samples and outperforms bootstrapping methods in simulation studies [33]. Simulation studies have also shown this method to provide more consistent and precise estimates in data containing outliers, which may inflate SEs and lead to abnormally high p-values [33]. Two models were estimated in each sample: a model testing predictions of the proposed integrated model with the binary demographic variables also included as covariates (Model 1; Fig. 1, upper panel) and a model that included effects of past social distancing behavior (Model 2; Fig. 1, lower panel). All constructs were latent variables indicated by single or multiple items. There were no missing data for the social cognition and self-reported behavioral variables. There were a few instances of missing data for the demographic variables ranging from 0.5% to 8.8% in the Australia sample, and 0.9% to 6.4% in the U.S. sample. Missing data are reported in Supplementary Appendix B. Missing data were imputed using stochastic hierarchical regression [33].\nThe analysis afforded a number of analyses to evaluate the adequacy of measures used to indicate the latent variables in the model. Construct validity of the latent factors for the social cognition, intention, and behavioral variables was established using the normalized factor pattern loadings after oblique rotation and Kaiser normalization [33] and the average variance extracted (AVE), which should approach or exceed .700 and .500, respectively. Internal consistency of the factors was estimated using omega reliability coefficients (ω) and composite reliability coefficients (ρ), which should exceed .700 and ideally approach .900. We also conducted tests of the discriminant validity of the constructs in the model. Discriminant validity was supported when the square root of the AVE for each latent variable exceeded its correlation with other latent variables.\nAdequacy of the proposed model in describing the data was established using the goodness-of-fit (GoF) index with values of .100, .250, and .360 corresponding to small, medium, and large effect sizes. Further information on model quality was provided by the average path coefficient and average R2 coefficient. These indices summarize the average parameter estimates of relations in the model and the amount of variance explained in each dependent variable, respectively, and should be statistically significant for a good-quality model. In addition, an overall GoF index is provided by the average block variance inflation factor for model parameters and the average full collinearity variance inflation factor, which should be equal to or lower than 3.3 for well-fitting models. These indices indicate the extent to which latent variables in the model overlap and contribute to model multicollinearity. They, therefore, provide an indication as to the uniqueness of the existing latent variables in the model. Four further indices were also used to evaluate model quality: the Simpson’s paradox ratio (SPR), R2 contribution ratio (R2CR), the statistical suppression ratio (SSR), and the nonlinear bivariate causality direction ratio (NLBCDR). The SPR indicates whether the model is free from incidences of Simpson’s paradox (i.e., when the path coefficient and the correlation associated with a latent variable have opposite signs), indicating a causality problem. The SPR should exceed .700 and ideally approach 1.000. The R2CR and SSR provide indication of the extent to which models are free from instances of negative R2 contributions and statistical suppression. The R2CR and SSR should exceed .900 and .700, respectively. The NLBCDR provides an estimate of the extent to which the proposed “causal” associations in the proposed model are more tenable than those in the opposite direction and provide an initial indicator of support for the hypothesized directions of the causal links in the proposed model compared to if the proposed direction were reversed. The NLBCDR should exceed .700 for high-quality models. Kock [33] provides further technical details on model fit and quality indices.\nModel effects were estimated using standardized path coefficients with confidence intervals (CIs) and test statistics. Effect sizes were estimated using a variant of Cohen’s f-square coefficient and represent the individual contribution of the predictor variable to the R2 coefficients of the criterion latent variable. Values of .02, .15, and .35 represent small, medium, and large effect sizes, respectively. Differences in the path coefficients in the models across the samples were tested using multiple-group analysis using the Satterthwaite method with two-tailed significance tests.\nWe also tested whether the inclusion of participants that were never under a “shelter-in-place” order, or had the “shelter-in-place” order lifted during the study, affected predicted relations in the models. The small numbers of participants that were, at some point, not subjected to “shelter-in-place” orders meant we could not conduct a formal moderator analysis, so we conducted a sensitivity analysis testing whether effects in the models differed if data from these participants were excluded. Models excluding and including past behavior were estimated in samples excluding participants who were never subject to a “shelter-in place” order, and in the sample that were never subject to an order, or who had the order lifted at some stage during the study. Formal comparisons of parameter estimates in these models with those from the full sample were made using the Satterthwaite method. Data files, analysis scripts, and output files for all analyses are available online: https://osf.io/x9tms/."}
LitCovid-PD-UBERON
{"project":"LitCovid-PD-UBERON","denotations":[{"id":"T4","span":{"begin":6236,"end":6240},"obj":"Body_part"},{"id":"T5","span":{"begin":7201,"end":7207},"obj":"Body_part"},{"id":"T6","span":{"begin":7808,"end":7813},"obj":"Body_part"}],"attributes":[{"id":"A4","pred":"uberon_id","subj":"T4","obj":"http://purl.obolibrary.org/obo/UBERON_0002387"},{"id":"A5","pred":"uberon_id","subj":"T5","obj":"http://purl.obolibrary.org/obo/UBERON_0002542"},{"id":"A6","pred":"uberon_id","subj":"T6","obj":"http://purl.obolibrary.org/obo/UBERON_0002542"}],"text":"Method\n\nParticipants and Recruitment\nSamples of Australian (N = 495, 50.1% female) and U.S. (N = 701, 48.9% female) residents were recruited via an online research panel company. To be eligible for inclusion, participants needed to be aged 18 years or older and not subject to formal quarantine for COVID-19. Participants were also screened for age, gender, and geographical region and quotas imposed during recruitment to ensure that the final samples closely matched the national distributions for these characteristics in each country. Data were collected between April 1 and May 6, 2020. All participants in the Australian sample were subject to a national “shelter-in-place” order issued by the federal government. However, issuance of orders in the USA was devolved to state governments resulting in some variations. The vast majority of participants in the U.S. sample (n = 610, 87.0%) were subject to “shelter-in-place” orders for the duration of the study. However, some states did not impose “shelter-in-place” orders at all (Arkansas, Iowa, Nebraska, North Dakota, South Dakota, Utah, and Wyoming), so a minority of participants in the U.S. sample (n = 37, 5.3%) were never subject to an order. Furthermore, in some cases in the U.S. sample (n = 47, 6.7%), “shelter-in-place” orders had been lifted prior to follow-up data collection. However, among the states in the USA that did not have “shelter-in-place” orders, or lifted their orders during the study, all issued social distancing guidelines and encouraged the population to follow those guidelines. Baseline sample characteristics are presented in Table 1.\nTable 1. Sample characteristics and descriptive statistics for study variables at baseline and at 1 week follow-up\nVariable Australia sample U.S. sample\nBaseline Follow-up Baseline Follow-up\nParticipants 495 365 701 440\nAge, M years (SD) 47.09 (17.11) 49.78 (16.89) 45.55 (17.40) 51.77 (16.26)\nGender, n (%)a\n Female 252 (51.1) 182 (50.1) 352 (48.9) 205 (46.6)\n Male 241 (48.9) 181 (49.9) 341 (50.5) 231 (52.5)\n Not specified/prefer not to answer 0 (0.0) 0 (0.0) 4 (0.6) 4 (0.9)\nEmployment status, n (%)b\n Currently unemployed/full-time caregiver 231 (46.7) 180 (49.3) 330 (47.3) 216 (49.5)\n Part-time/casual employed 97 (19.6) 65 (17.8) 106 (15.2) 60 (13.8)\n Currently employed full time 140 (28.3) 104 (28.5) 233 (33.4) 147 (33.7)\n Leave without pay/furloughed 27 (5.5) 16 (4.4) 28 (4.0) 13 (3.0)\nMarital status, n (%)c\n Married 184 (37.2) 146 (40.0) 300 (43.0) 224 (51.4)\n Widowed 8 (1.6) 7 (1.9) 22 (3.2) 18 (4.1)\n Separated/divorced 53 (10.7) 39 (10.7) 69 (9.9) 47 (10.8)\n Never married 160 (32.3) 103 (28.2) 255 (36.6) 126(28.9)\n Married de facto 90 (18.2) 70 (19.2) 51 (7.3) 21 (4.8)\nEthnicity, n (%)d\n Black 3 (0.6) 1 (0.3) 52 (7.5) 26 (6.0)\n Caucasian/White 392 (79.2) 304 (83.3) 566 (81.2) 376 (86.2)\n Asian (South-East Asia/South Asia) 71 (14.3) 43 (11.8) 39 (5.6) 24 (5.5)\n Middle-Eastern 6 (1.2) 3 (0.8) 1 (0.1) 0 (0.0)\n Other 13 (2.6) 6 (1.6) 27 (3.9) 8 (1.8)\n Prefer not to answer 10 (2.0) 8 (2.2) 12 (1.7) 2 (0.5)\nIncome, n (%)e\n Zero income 8 (1.7) 4 (1.2) 31 (4.4) 19 (4.4)\n $1–$199 ($1–$10,399) 9 (2.0) 6 (1.8) 40 (5.7) 24 (5.5)\n $200–$299 ($10,400–$15,599) 12 (2.6) 8 (2.4) 34 (4.9) 23 (5.3)\n $300–$399 ($15,600–$20,799) 19 (4.1) 12 (3.6) 38 (5.5) 23 (5.3)\n $400–$599 ($20,800–$31,199) 42 (9.2) 33 (9.9) 62 (8.9) 33 (7.6)\n $600–$799 ($31,200–$41,599) 57 (12.4) 42 (12.6) 61 (8.8) 39 (8.9)\n $800–$999 ($41,600–$51,999) 45 (9.8) 31 (9.3) 68 (9.8) 46 (10.6)\n $1,000–$1,249 ($52,000–$64,999) 39 (8.5) 32 (9.6) 48 (6.9) 38 (8.7)\n $1,250–$1,499 ($65,000–$77,999) 28 (6.1) 22 (6.6) 59 (8.5) 41 (9.4)\n $1,500–$1,999 ($78,000–$103,999) 72 (15.7) 50 (15.0) 72 (10.3) 48 (11.0)\n $2,000 or more ($104,000 or more) 81 (17.6) 62 (18.6) 108 (15.5) 74 (17.0)\n Prefer not to answer 47 (10.2) 32 (9.6) 76 (10.9) 28 (6.4)\nEducation level, n (%)\n Completed junior/lower/primary school 18 (3.6) 17 (4.7) 6 (0.9) 0 (0.0)\n Completed senior/high/secondary school 133 (26.9) 98 (26.8) 265 (37.8) 132 (30.0)\n Postschool vocational qualification/diploma 147 (29.7) 111 (30.4) 138 (19.7) 94 (21.4)\n Undergraduate university degree 131 (26.5) 93 (25.5) 214 (30.5) 159 (36.1)\n Postgraduate university degree 66 (13.3) 46 (12.6) 78 (11.1) 55 (12.5)\nSD standard deviation.\naTwo participants in the Australian sample did not report their gender and four participants in the U.S. sample did not report their gender.\nbFour participants in the U.S. sample did not report their employment status.\ncFour participants in the U.S. sample did not report their marital status.\ndFour participants in the U.S. sample did not report their ethnicity.\neThirty-one participants in the Australian sample did not report their income and four participants in the U.S. sample did not report their income.\n\nDesign and Procedure\nThe study adopted a prospective correlational design with self-report measures of social cognition constructs from the proposed integrated model, intention, and past social distancing behavior administered at an initial data collection occasion in a survey administered using the Qualtrics online survey tool. Social cognition measures included the theory of planned behavior (attitude, subjective norm, and PBC), moral norm, anticipated regret, action planning, and habit constructs. Participants were informed that they were participating in a survey on their social distancing behavior and provided with information outlining study requirements. They were required to provide informed consent before proceeding with the survey. Participants were also provided with instructions on how to complete study measures and a definition of the target behavior: “The following survey will ask about your beliefs and attitudes about ‘social distancing’. What do we mean by social distancing? Social distancing (also known as ‘physical distancing’) is deliberately increasing the physical space between people to avoid spreading illness. The World Health Organization and other world leading health authorities suggest that you should maintain at least a 1–2 meter (3–6 feet) distance from other people to lessen the chances of getting infected with COVID-19. When answering the questions in this survey, think about your social distancing behavior (i.e., maintaining at least a 1–2 m (3–6 ft) distance from other people).” One week later, participants were re-contacted by the panel company and asked to self-report their habit and social distancing behavior over the previous week using the same measures used at the initial data collection occasion. Participants received a fixed sum of money for their participation based on expected completion time consistent with the panel company’s published rates. Approval for study procedures was granted prior to data collection from the Griffith University Human Research Ethics Committee.\n\nMeasures\nStudy measures were multi-item self-report measures of constructs based on published guidelines and measures used in previous studies [13, 29, 30]. Participants provided their responses on scales with seven-point response options. Complete study measures are provided in Supplementary Appendix A.\n\nSocial cognition constructs\nMulti-item measures of attitude, subjective norm, PBC, moral norm, anticipated regret, and action planning were developed according to published guidelines [13, 29]. Each measure made explicit reference to the target behavior of social distancing, and participants were reminded of the definition of social distancing before completing the measures.\n\nIntention\nParticipants’ intention to participate in social distancing behavior over the next week was measured using a scale developed according to published guidelines [31].\n\nHabit\nHabit was measured at both time points using the behavioral automaticity items of Verplanken and Orbell’s self-report habit index [25]. The measure measures individuals’ reflections on the extent to which the behavior is experienced as automatic and enacted without thought.\n\nPast behavior and behavior\nParticipants self-reported their participation in social distancing behavior to minimize the transmission of the SARS-CoV-2 virus that causes COVID-19. The measure comprised two items prompting participants to report their frequency of social distancing behavior in the previous week. This is based on previously used self-report behavioral measures that have demonstrated concurrent validity with non-self-report measures in other behavioral contexts [32].\n\nDemographic variables\nParticipants self-reported their age in years, gender, employment status (currently unemployed/full-time caregiver, currently full-time employed, part-time employed, or on leave without pay/furloughed), marital status (married, widowed, separated/divorced, never married, or in a de factor relationship), annual household income stratified by 11 income levels based on Australian and U.S. national averages, highest level of formal education (completed junior/lower/primary school, completed senior/high/secondary school, postschool vocational qualification/diploma, further education diploma, undergraduate university degree, or postgraduate university degree), and ethnicity (Black, Caucasian/White, Asian, or Middle-eastern). Binary income (low income vs. middle/high income), highest education level (completed school education only vs. completed postschool education), and ethnicity (Caucasian/White vs. non-White) variables were computed for use in subsequent analyses.\n\nData Analysis\nHypothesized relations among the integrated model constructs were tested in the Australian and U.S. samples separately using variance-based structural equation modeling implemented in the WARP 7.0 analysis package [33]. Model parameters and standard errors (SEs) were computed using the “Stable3” estimation method, which has been shown to provide the most precise parameter estimates in complex structural models in smaller samples and outperforms bootstrapping methods in simulation studies [33]. Simulation studies have also shown this method to provide more consistent and precise estimates in data containing outliers, which may inflate SEs and lead to abnormally high p-values [33]. Two models were estimated in each sample: a model testing predictions of the proposed integrated model with the binary demographic variables also included as covariates (Model 1; Fig. 1, upper panel) and a model that included effects of past social distancing behavior (Model 2; Fig. 1, lower panel). All constructs were latent variables indicated by single or multiple items. There were no missing data for the social cognition and self-reported behavioral variables. There were a few instances of missing data for the demographic variables ranging from 0.5% to 8.8% in the Australia sample, and 0.9% to 6.4% in the U.S. sample. Missing data are reported in Supplementary Appendix B. Missing data were imputed using stochastic hierarchical regression [33].\nThe analysis afforded a number of analyses to evaluate the adequacy of measures used to indicate the latent variables in the model. Construct validity of the latent factors for the social cognition, intention, and behavioral variables was established using the normalized factor pattern loadings after oblique rotation and Kaiser normalization [33] and the average variance extracted (AVE), which should approach or exceed .700 and .500, respectively. Internal consistency of the factors was estimated using omega reliability coefficients (ω) and composite reliability coefficients (ρ), which should exceed .700 and ideally approach .900. We also conducted tests of the discriminant validity of the constructs in the model. Discriminant validity was supported when the square root of the AVE for each latent variable exceeded its correlation with other latent variables.\nAdequacy of the proposed model in describing the data was established using the goodness-of-fit (GoF) index with values of .100, .250, and .360 corresponding to small, medium, and large effect sizes. Further information on model quality was provided by the average path coefficient and average R2 coefficient. These indices summarize the average parameter estimates of relations in the model and the amount of variance explained in each dependent variable, respectively, and should be statistically significant for a good-quality model. In addition, an overall GoF index is provided by the average block variance inflation factor for model parameters and the average full collinearity variance inflation factor, which should be equal to or lower than 3.3 for well-fitting models. These indices indicate the extent to which latent variables in the model overlap and contribute to model multicollinearity. They, therefore, provide an indication as to the uniqueness of the existing latent variables in the model. Four further indices were also used to evaluate model quality: the Simpson’s paradox ratio (SPR), R2 contribution ratio (R2CR), the statistical suppression ratio (SSR), and the nonlinear bivariate causality direction ratio (NLBCDR). The SPR indicates whether the model is free from incidences of Simpson’s paradox (i.e., when the path coefficient and the correlation associated with a latent variable have opposite signs), indicating a causality problem. The SPR should exceed .700 and ideally approach 1.000. The R2CR and SSR provide indication of the extent to which models are free from instances of negative R2 contributions and statistical suppression. The R2CR and SSR should exceed .900 and .700, respectively. The NLBCDR provides an estimate of the extent to which the proposed “causal” associations in the proposed model are more tenable than those in the opposite direction and provide an initial indicator of support for the hypothesized directions of the causal links in the proposed model compared to if the proposed direction were reversed. The NLBCDR should exceed .700 for high-quality models. Kock [33] provides further technical details on model fit and quality indices.\nModel effects were estimated using standardized path coefficients with confidence intervals (CIs) and test statistics. Effect sizes were estimated using a variant of Cohen’s f-square coefficient and represent the individual contribution of the predictor variable to the R2 coefficients of the criterion latent variable. Values of .02, .15, and .35 represent small, medium, and large effect sizes, respectively. Differences in the path coefficients in the models across the samples were tested using multiple-group analysis using the Satterthwaite method with two-tailed significance tests.\nWe also tested whether the inclusion of participants that were never under a “shelter-in-place” order, or had the “shelter-in-place” order lifted during the study, affected predicted relations in the models. The small numbers of participants that were, at some point, not subjected to “shelter-in-place” orders meant we could not conduct a formal moderator analysis, so we conducted a sensitivity analysis testing whether effects in the models differed if data from these participants were excluded. Models excluding and including past behavior were estimated in samples excluding participants who were never subject to a “shelter-in place” order, and in the sample that were never subject to an order, or who had the order lifted at some stage during the study. Formal comparisons of parameter estimates in these models with those from the full sample were made using the Satterthwaite method. Data files, analysis scripts, and output files for all analyses are available online: https://osf.io/x9tms/."}
LitCovid-PD-MONDO
{"project":"LitCovid-PD-MONDO","denotations":[{"id":"T45","span":{"begin":299,"end":307},"obj":"Disease"},{"id":"T46","span":{"begin":5056,"end":5072},"obj":"Disease"},{"id":"T47","span":{"begin":5284,"end":5300},"obj":"Disease"},{"id":"T48","span":{"begin":5382,"end":5385},"obj":"Disease"},{"id":"T49","span":{"begin":6316,"end":6324},"obj":"Disease"},{"id":"T50","span":{"begin":7310,"end":7326},"obj":"Disease"},{"id":"T51","span":{"begin":7388,"end":7391},"obj":"Disease"},{"id":"T52","span":{"begin":8287,"end":8295},"obj":"Disease"},{"id":"T53","span":{"begin":8316,"end":8324},"obj":"Disease"},{"id":"T54","span":{"begin":10747,"end":10763},"obj":"Disease"},{"id":"T55","span":{"begin":11274,"end":11290},"obj":"Disease"},{"id":"T56","span":{"begin":12258,"end":12260},"obj":"Disease"},{"id":"T57","span":{"begin":13073,"end":13075},"obj":"Disease"},{"id":"T58","span":{"begin":13587,"end":13589},"obj":"Disease"},{"id":"T59","span":{"begin":14434,"end":14436},"obj":"Disease"}],"attributes":[{"id":"A45","pred":"mondo_id","subj":"T45","obj":"http://purl.obolibrary.org/obo/MONDO_0100096"},{"id":"A46","pred":"mondo_id","subj":"T46","obj":"http://purl.obolibrary.org/obo/MONDO_0010244"},{"id":"A47","pred":"mondo_id","subj":"T47","obj":"http://purl.obolibrary.org/obo/MONDO_0010244"},{"id":"A48","pred":"mondo_id","subj":"T48","obj":"http://purl.obolibrary.org/obo/MONDO_0005388"},{"id":"A49","pred":"mondo_id","subj":"T49","obj":"http://purl.obolibrary.org/obo/MONDO_0100096"},{"id":"A50","pred":"mondo_id","subj":"T50","obj":"http://purl.obolibrary.org/obo/MONDO_0010244"},{"id":"A51","pred":"mondo_id","subj":"T51","obj":"http://purl.obolibrary.org/obo/MONDO_0005388"},{"id":"A52","pred":"mondo_id","subj":"T52","obj":"http://purl.obolibrary.org/obo/MONDO_0005091"},{"id":"A53","pred":"mondo_id","subj":"T53","obj":"http://purl.obolibrary.org/obo/MONDO_0100096"},{"id":"A54","pred":"mondo_id","subj":"T54","obj":"http://purl.obolibrary.org/obo/MONDO_0010244"},{"id":"A55","pred":"mondo_id","subj":"T55","obj":"http://purl.obolibrary.org/obo/MONDO_0010244"},{"id":"A56","pred":"mondo_id","subj":"T56","obj":"http://purl.obolibrary.org/obo/MONDO_0019903"},{"id":"A57","pred":"mondo_id","subj":"T57","obj":"http://purl.obolibrary.org/obo/MONDO_0019903"},{"id":"A58","pred":"mondo_id","subj":"T58","obj":"http://purl.obolibrary.org/obo/MONDO_0019903"},{"id":"A59","pred":"mondo_id","subj":"T59","obj":"http://purl.obolibrary.org/obo/MONDO_0019903"}],"text":"Method\n\nParticipants and Recruitment\nSamples of Australian (N = 495, 50.1% female) and U.S. (N = 701, 48.9% female) residents were recruited via an online research panel company. To be eligible for inclusion, participants needed to be aged 18 years or older and not subject to formal quarantine for COVID-19. Participants were also screened for age, gender, and geographical region and quotas imposed during recruitment to ensure that the final samples closely matched the national distributions for these characteristics in each country. Data were collected between April 1 and May 6, 2020. All participants in the Australian sample were subject to a national “shelter-in-place” order issued by the federal government. However, issuance of orders in the USA was devolved to state governments resulting in some variations. The vast majority of participants in the U.S. sample (n = 610, 87.0%) were subject to “shelter-in-place” orders for the duration of the study. However, some states did not impose “shelter-in-place” orders at all (Arkansas, Iowa, Nebraska, North Dakota, South Dakota, Utah, and Wyoming), so a minority of participants in the U.S. sample (n = 37, 5.3%) were never subject to an order. Furthermore, in some cases in the U.S. sample (n = 47, 6.7%), “shelter-in-place” orders had been lifted prior to follow-up data collection. However, among the states in the USA that did not have “shelter-in-place” orders, or lifted their orders during the study, all issued social distancing guidelines and encouraged the population to follow those guidelines. Baseline sample characteristics are presented in Table 1.\nTable 1. Sample characteristics and descriptive statistics for study variables at baseline and at 1 week follow-up\nVariable Australia sample U.S. sample\nBaseline Follow-up Baseline Follow-up\nParticipants 495 365 701 440\nAge, M years (SD) 47.09 (17.11) 49.78 (16.89) 45.55 (17.40) 51.77 (16.26)\nGender, n (%)a\n Female 252 (51.1) 182 (50.1) 352 (48.9) 205 (46.6)\n Male 241 (48.9) 181 (49.9) 341 (50.5) 231 (52.5)\n Not specified/prefer not to answer 0 (0.0) 0 (0.0) 4 (0.6) 4 (0.9)\nEmployment status, n (%)b\n Currently unemployed/full-time caregiver 231 (46.7) 180 (49.3) 330 (47.3) 216 (49.5)\n Part-time/casual employed 97 (19.6) 65 (17.8) 106 (15.2) 60 (13.8)\n Currently employed full time 140 (28.3) 104 (28.5) 233 (33.4) 147 (33.7)\n Leave without pay/furloughed 27 (5.5) 16 (4.4) 28 (4.0) 13 (3.0)\nMarital status, n (%)c\n Married 184 (37.2) 146 (40.0) 300 (43.0) 224 (51.4)\n Widowed 8 (1.6) 7 (1.9) 22 (3.2) 18 (4.1)\n Separated/divorced 53 (10.7) 39 (10.7) 69 (9.9) 47 (10.8)\n Never married 160 (32.3) 103 (28.2) 255 (36.6) 126(28.9)\n Married de facto 90 (18.2) 70 (19.2) 51 (7.3) 21 (4.8)\nEthnicity, n (%)d\n Black 3 (0.6) 1 (0.3) 52 (7.5) 26 (6.0)\n Caucasian/White 392 (79.2) 304 (83.3) 566 (81.2) 376 (86.2)\n Asian (South-East Asia/South Asia) 71 (14.3) 43 (11.8) 39 (5.6) 24 (5.5)\n Middle-Eastern 6 (1.2) 3 (0.8) 1 (0.1) 0 (0.0)\n Other 13 (2.6) 6 (1.6) 27 (3.9) 8 (1.8)\n Prefer not to answer 10 (2.0) 8 (2.2) 12 (1.7) 2 (0.5)\nIncome, n (%)e\n Zero income 8 (1.7) 4 (1.2) 31 (4.4) 19 (4.4)\n $1–$199 ($1–$10,399) 9 (2.0) 6 (1.8) 40 (5.7) 24 (5.5)\n $200–$299 ($10,400–$15,599) 12 (2.6) 8 (2.4) 34 (4.9) 23 (5.3)\n $300–$399 ($15,600–$20,799) 19 (4.1) 12 (3.6) 38 (5.5) 23 (5.3)\n $400–$599 ($20,800–$31,199) 42 (9.2) 33 (9.9) 62 (8.9) 33 (7.6)\n $600–$799 ($31,200–$41,599) 57 (12.4) 42 (12.6) 61 (8.8) 39 (8.9)\n $800–$999 ($41,600–$51,999) 45 (9.8) 31 (9.3) 68 (9.8) 46 (10.6)\n $1,000–$1,249 ($52,000–$64,999) 39 (8.5) 32 (9.6) 48 (6.9) 38 (8.7)\n $1,250–$1,499 ($65,000–$77,999) 28 (6.1) 22 (6.6) 59 (8.5) 41 (9.4)\n $1,500–$1,999 ($78,000–$103,999) 72 (15.7) 50 (15.0) 72 (10.3) 48 (11.0)\n $2,000 or more ($104,000 or more) 81 (17.6) 62 (18.6) 108 (15.5) 74 (17.0)\n Prefer not to answer 47 (10.2) 32 (9.6) 76 (10.9) 28 (6.4)\nEducation level, n (%)\n Completed junior/lower/primary school 18 (3.6) 17 (4.7) 6 (0.9) 0 (0.0)\n Completed senior/high/secondary school 133 (26.9) 98 (26.8) 265 (37.8) 132 (30.0)\n Postschool vocational qualification/diploma 147 (29.7) 111 (30.4) 138 (19.7) 94 (21.4)\n Undergraduate university degree 131 (26.5) 93 (25.5) 214 (30.5) 159 (36.1)\n Postgraduate university degree 66 (13.3) 46 (12.6) 78 (11.1) 55 (12.5)\nSD standard deviation.\naTwo participants in the Australian sample did not report their gender and four participants in the U.S. sample did not report their gender.\nbFour participants in the U.S. sample did not report their employment status.\ncFour participants in the U.S. sample did not report their marital status.\ndFour participants in the U.S. sample did not report their ethnicity.\neThirty-one participants in the Australian sample did not report their income and four participants in the U.S. sample did not report their income.\n\nDesign and Procedure\nThe study adopted a prospective correlational design with self-report measures of social cognition constructs from the proposed integrated model, intention, and past social distancing behavior administered at an initial data collection occasion in a survey administered using the Qualtrics online survey tool. Social cognition measures included the theory of planned behavior (attitude, subjective norm, and PBC), moral norm, anticipated regret, action planning, and habit constructs. Participants were informed that they were participating in a survey on their social distancing behavior and provided with information outlining study requirements. They were required to provide informed consent before proceeding with the survey. Participants were also provided with instructions on how to complete study measures and a definition of the target behavior: “The following survey will ask about your beliefs and attitudes about ‘social distancing’. What do we mean by social distancing? Social distancing (also known as ‘physical distancing’) is deliberately increasing the physical space between people to avoid spreading illness. The World Health Organization and other world leading health authorities suggest that you should maintain at least a 1–2 meter (3–6 feet) distance from other people to lessen the chances of getting infected with COVID-19. When answering the questions in this survey, think about your social distancing behavior (i.e., maintaining at least a 1–2 m (3–6 ft) distance from other people).” One week later, participants were re-contacted by the panel company and asked to self-report their habit and social distancing behavior over the previous week using the same measures used at the initial data collection occasion. Participants received a fixed sum of money for their participation based on expected completion time consistent with the panel company’s published rates. Approval for study procedures was granted prior to data collection from the Griffith University Human Research Ethics Committee.\n\nMeasures\nStudy measures were multi-item self-report measures of constructs based on published guidelines and measures used in previous studies [13, 29, 30]. Participants provided their responses on scales with seven-point response options. Complete study measures are provided in Supplementary Appendix A.\n\nSocial cognition constructs\nMulti-item measures of attitude, subjective norm, PBC, moral norm, anticipated regret, and action planning were developed according to published guidelines [13, 29]. Each measure made explicit reference to the target behavior of social distancing, and participants were reminded of the definition of social distancing before completing the measures.\n\nIntention\nParticipants’ intention to participate in social distancing behavior over the next week was measured using a scale developed according to published guidelines [31].\n\nHabit\nHabit was measured at both time points using the behavioral automaticity items of Verplanken and Orbell’s self-report habit index [25]. The measure measures individuals’ reflections on the extent to which the behavior is experienced as automatic and enacted without thought.\n\nPast behavior and behavior\nParticipants self-reported their participation in social distancing behavior to minimize the transmission of the SARS-CoV-2 virus that causes COVID-19. The measure comprised two items prompting participants to report their frequency of social distancing behavior in the previous week. This is based on previously used self-report behavioral measures that have demonstrated concurrent validity with non-self-report measures in other behavioral contexts [32].\n\nDemographic variables\nParticipants self-reported their age in years, gender, employment status (currently unemployed/full-time caregiver, currently full-time employed, part-time employed, or on leave without pay/furloughed), marital status (married, widowed, separated/divorced, never married, or in a de factor relationship), annual household income stratified by 11 income levels based on Australian and U.S. national averages, highest level of formal education (completed junior/lower/primary school, completed senior/high/secondary school, postschool vocational qualification/diploma, further education diploma, undergraduate university degree, or postgraduate university degree), and ethnicity (Black, Caucasian/White, Asian, or Middle-eastern). Binary income (low income vs. middle/high income), highest education level (completed school education only vs. completed postschool education), and ethnicity (Caucasian/White vs. non-White) variables were computed for use in subsequent analyses.\n\nData Analysis\nHypothesized relations among the integrated model constructs were tested in the Australian and U.S. samples separately using variance-based structural equation modeling implemented in the WARP 7.0 analysis package [33]. Model parameters and standard errors (SEs) were computed using the “Stable3” estimation method, which has been shown to provide the most precise parameter estimates in complex structural models in smaller samples and outperforms bootstrapping methods in simulation studies [33]. Simulation studies have also shown this method to provide more consistent and precise estimates in data containing outliers, which may inflate SEs and lead to abnormally high p-values [33]. Two models were estimated in each sample: a model testing predictions of the proposed integrated model with the binary demographic variables also included as covariates (Model 1; Fig. 1, upper panel) and a model that included effects of past social distancing behavior (Model 2; Fig. 1, lower panel). All constructs were latent variables indicated by single or multiple items. There were no missing data for the social cognition and self-reported behavioral variables. There were a few instances of missing data for the demographic variables ranging from 0.5% to 8.8% in the Australia sample, and 0.9% to 6.4% in the U.S. sample. Missing data are reported in Supplementary Appendix B. Missing data were imputed using stochastic hierarchical regression [33].\nThe analysis afforded a number of analyses to evaluate the adequacy of measures used to indicate the latent variables in the model. Construct validity of the latent factors for the social cognition, intention, and behavioral variables was established using the normalized factor pattern loadings after oblique rotation and Kaiser normalization [33] and the average variance extracted (AVE), which should approach or exceed .700 and .500, respectively. Internal consistency of the factors was estimated using omega reliability coefficients (ω) and composite reliability coefficients (ρ), which should exceed .700 and ideally approach .900. We also conducted tests of the discriminant validity of the constructs in the model. Discriminant validity was supported when the square root of the AVE for each latent variable exceeded its correlation with other latent variables.\nAdequacy of the proposed model in describing the data was established using the goodness-of-fit (GoF) index with values of .100, .250, and .360 corresponding to small, medium, and large effect sizes. Further information on model quality was provided by the average path coefficient and average R2 coefficient. These indices summarize the average parameter estimates of relations in the model and the amount of variance explained in each dependent variable, respectively, and should be statistically significant for a good-quality model. In addition, an overall GoF index is provided by the average block variance inflation factor for model parameters and the average full collinearity variance inflation factor, which should be equal to or lower than 3.3 for well-fitting models. These indices indicate the extent to which latent variables in the model overlap and contribute to model multicollinearity. They, therefore, provide an indication as to the uniqueness of the existing latent variables in the model. Four further indices were also used to evaluate model quality: the Simpson’s paradox ratio (SPR), R2 contribution ratio (R2CR), the statistical suppression ratio (SSR), and the nonlinear bivariate causality direction ratio (NLBCDR). The SPR indicates whether the model is free from incidences of Simpson’s paradox (i.e., when the path coefficient and the correlation associated with a latent variable have opposite signs), indicating a causality problem. The SPR should exceed .700 and ideally approach 1.000. The R2CR and SSR provide indication of the extent to which models are free from instances of negative R2 contributions and statistical suppression. The R2CR and SSR should exceed .900 and .700, respectively. The NLBCDR provides an estimate of the extent to which the proposed “causal” associations in the proposed model are more tenable than those in the opposite direction and provide an initial indicator of support for the hypothesized directions of the causal links in the proposed model compared to if the proposed direction were reversed. The NLBCDR should exceed .700 for high-quality models. Kock [33] provides further technical details on model fit and quality indices.\nModel effects were estimated using standardized path coefficients with confidence intervals (CIs) and test statistics. Effect sizes were estimated using a variant of Cohen’s f-square coefficient and represent the individual contribution of the predictor variable to the R2 coefficients of the criterion latent variable. Values of .02, .15, and .35 represent small, medium, and large effect sizes, respectively. Differences in the path coefficients in the models across the samples were tested using multiple-group analysis using the Satterthwaite method with two-tailed significance tests.\nWe also tested whether the inclusion of participants that were never under a “shelter-in-place” order, or had the “shelter-in-place” order lifted during the study, affected predicted relations in the models. The small numbers of participants that were, at some point, not subjected to “shelter-in-place” orders meant we could not conduct a formal moderator analysis, so we conducted a sensitivity analysis testing whether effects in the models differed if data from these participants were excluded. Models excluding and including past behavior were estimated in samples excluding participants who were never subject to a “shelter-in place” order, and in the sample that were never subject to an order, or who had the order lifted at some stage during the study. Formal comparisons of parameter estimates in these models with those from the full sample were made using the Satterthwaite method. Data files, analysis scripts, and output files for all analyses are available online: https://osf.io/x9tms/."}
LitCovid-PD-CLO
{"project":"LitCovid-PD-CLO","denotations":[{"id":"T57","span":{"begin":75,"end":81},"obj":"http://purl.obolibrary.org/obo/UBERON_0003100"},{"id":"T58","span":{"begin":108,"end":114},"obj":"http://purl.obolibrary.org/obo/UBERON_0003100"},{"id":"T59","span":{"begin":240,"end":242},"obj":"http://purl.obolibrary.org/obo/CLO_0050510"},{"id":"T60","span":{"begin":650,"end":651},"obj":"http://purl.obolibrary.org/obo/CLO_0001020"},{"id":"T61","span":{"begin":1113,"end":1114},"obj":"http://purl.obolibrary.org/obo/CLO_0001020"},{"id":"T62","span":{"begin":1721,"end":1725},"obj":"http://purl.obolibrary.org/obo/CLO_0001757"},{"id":"T63","span":{"begin":1946,"end":1947},"obj":"http://purl.obolibrary.org/obo/CLO_0001020"},{"id":"T64","span":{"begin":1949,"end":1955},"obj":"http://purl.obolibrary.org/obo/UBERON_0003100"},{"id":"T65","span":{"begin":2005,"end":2009},"obj":"http://purl.obolibrary.org/obo/UBERON_0003101"},{"id":"T66","span":{"begin":2005,"end":2009},"obj":"http://www.ebi.ac.uk/efo/EFO_0000970"},{"id":"T67","span":{"begin":2023,"end":2026},"obj":"http://purl.obolibrary.org/obo/CLO_0054057"},{"id":"T68","span":{"begin":2154,"end":2155},"obj":"http://purl.obolibrary.org/obo/CLO_0001021"},{"id":"T69","span":{"begin":2427,"end":2429},"obj":"http://purl.obolibrary.org/obo/CLO_0050509"},{"id":"T70","span":{"begin":2574,"end":2576},"obj":"http://purl.obolibrary.org/obo/CLO_0050507"},{"id":"T71","span":{"begin":2584,"end":2586},"obj":"http://purl.obolibrary.org/obo/CLO_0050510"},{"id":"T72","span":{"begin":2822,"end":2824},"obj":"http://purl.obolibrary.org/obo/CLO_0001407"},{"id":"T73","span":{"begin":2943,"end":2945},"obj":"http://purl.obolibrary.org/obo/CLO_0054055"},{"id":"T74","span":{"begin":3063,"end":3065},"obj":"http://purl.obolibrary.org/obo/CLO_0050509"},{"id":"T75","span":{"begin":3149,"end":3155},"obj":"http://purl.obolibrary.org/obo/CLO_0008149"},{"id":"T76","span":{"begin":3316,"end":3318},"obj":"http://purl.obolibrary.org/obo/CLO_0001302"},{"id":"T77","span":{"begin":3574,"end":3576},"obj":"http://purl.obolibrary.org/obo/CLO_0053799"},{"id":"T78","span":{"begin":3668,"end":3670},"obj":"http://purl.obolibrary.org/obo/CLO_0001382"},{"id":"T79","span":{"begin":3731,"end":3733},"obj":"http://purl.obolibrary.org/obo/CLO_0050507"},{"id":"T80","span":{"begin":3751,"end":3753},"obj":"http://purl.obolibrary.org/obo/CLO_0053794"},{"id":"T81","span":{"begin":3828,"end":3830},"obj":"http://purl.obolibrary.org/obo/CLO_0001382"},{"id":"T82","span":{"begin":4045,"end":4047},"obj":"http://purl.obolibrary.org/obo/CLO_0050510"},{"id":"T83","span":{"begin":4158,"end":4161},"obj":"http://purl.obolibrary.org/obo/CLO_0054061"},{"id":"T84","span":{"begin":4251,"end":4253},"obj":"http://purl.obolibrary.org/obo/CLO_0001527"},{"id":"T85","span":{"begin":4992,"end":4993},"obj":"http://purl.obolibrary.org/obo/CLO_0001020"},{"id":"T86","span":{"begin":5222,"end":5223},"obj":"http://purl.obolibrary.org/obo/CLO_0001020"},{"id":"T87","span":{"begin":5518,"end":5519},"obj":"http://purl.obolibrary.org/obo/CLO_0001020"},{"id":"T88","span":{"begin":5793,"end":5794},"obj":"http://purl.obolibrary.org/obo/CLO_0001020"},{"id":"T89","span":{"begin":5857,"end":5860},"obj":"http://purl.obolibrary.org/obo/CLO_0001755"},{"id":"T90","span":{"begin":6121,"end":6133},"obj":"http://purl.obolibrary.org/obo/OBI_0000245"},{"id":"T91","span":{"begin":6219,"end":6220},"obj":"http://purl.obolibrary.org/obo/CLO_0001020"},{"id":"T92","span":{"begin":6232,"end":6235},"obj":"http://purl.obolibrary.org/obo/CLO_0001313"},{"id":"T93","span":{"begin":6443,"end":6444},"obj":"http://purl.obolibrary.org/obo/CLO_0001020"},{"id":"T94","span":{"begin":6452,"end":6455},"obj":"http://purl.obolibrary.org/obo/CLO_0001313"},{"id":"T95","span":{"begin":6456,"end":6458},"obj":"http://purl.obolibrary.org/obo/CLO_0003401"},{"id":"T96","span":{"begin":6741,"end":6742},"obj":"http://purl.obolibrary.org/obo/CLO_0001020"},{"id":"T97","span":{"begin":6969,"end":6974},"obj":"http://purl.obolibrary.org/obo/NCBITaxon_9606"},{"id":"T98","span":{"begin":7306,"end":7307},"obj":"http://purl.obolibrary.org/obo/CLO_0001020"},{"id":"T99","span":{"begin":7806,"end":7807},"obj":"http://purl.obolibrary.org/obo/CLO_0001020"},{"id":"T100","span":{"begin":8298,"end":8303},"obj":"http://purl.obolibrary.org/obo/NCBITaxon_10239"},{"id":"T101","span":{"begin":8933,"end":8934},"obj":"http://purl.obolibrary.org/obo/CLO_0001020"},{"id":"T102","span":{"begin":8998,"end":9000},"obj":"http://purl.obolibrary.org/obo/CLO_0053733"},{"id":"T103","span":{"begin":9712,"end":9718},"obj":"http://purl.obolibrary.org/obo/UBERON_0000473"},{"id":"T104","span":{"begin":9968,"end":9971},"obj":"http://purl.obolibrary.org/obo/CLO_0051582"},{"id":"T105","span":{"begin":10377,"end":10378},"obj":"http://purl.obolibrary.org/obo/CLO_0001020"},{"id":"T106","span":{"begin":10385,"end":10392},"obj":"http://purl.obolibrary.org/obo/UBERON_0000473"},{"id":"T107","span":{"begin":10539,"end":10540},"obj":"http://purl.obolibrary.org/obo/CLO_0001020"},{"id":"T108","span":{"begin":10815,"end":10816},"obj":"http://purl.obolibrary.org/obo/CLO_0001020"},{"id":"T109","span":{"begin":11017,"end":11018},"obj":"http://purl.obolibrary.org/obo/CLO_0001021"},{"id":"T110","span":{"begin":11115,"end":11116},"obj":"http://purl.obolibrary.org/obo/CLO_0001020"},{"id":"T111","span":{"begin":11750,"end":11755},"obj":"http://purl.obolibrary.org/obo/UBERON_0000473"},{"id":"T112","span":{"begin":12479,"end":12480},"obj":"http://purl.obolibrary.org/obo/CLO_0001020"},{"id":"T113","span":{"begin":13358,"end":13359},"obj":"http://purl.obolibrary.org/obo/CLO_0001020"},{"id":"T114","span":{"begin":13409,"end":13410},"obj":"http://purl.obolibrary.org/obo/CLO_0001020"},{"id":"T115","span":{"begin":14266,"end":14270},"obj":"http://purl.obolibrary.org/obo/UBERON_0000473"},{"id":"T116","span":{"begin":14317,"end":14318},"obj":"http://purl.obolibrary.org/obo/CLO_0001020"},{"id":"T117","span":{"begin":14509,"end":14511},"obj":"http://purl.obolibrary.org/obo/CLO_0001000"},{"id":"T118","span":{"begin":14650,"end":14656},"obj":"http://purl.obolibrary.org/obo/UBERON_0000473"},{"id":"T119","span":{"begin":14727,"end":14733},"obj":"http://purl.obolibrary.org/obo/UBERON_0002415"},{"id":"T120","span":{"begin":14747,"end":14752},"obj":"http://purl.obolibrary.org/obo/UBERON_0000473"},{"id":"T121","span":{"begin":14762,"end":14768},"obj":"http://purl.obolibrary.org/obo/UBERON_0000473"},{"id":"T122","span":{"begin":14829,"end":14830},"obj":"http://purl.obolibrary.org/obo/CLO_0001020"},{"id":"T123","span":{"begin":15092,"end":15093},"obj":"http://purl.obolibrary.org/obo/CLO_0001020"},{"id":"T124","span":{"begin":15137,"end":15138},"obj":"http://purl.obolibrary.org/obo/CLO_0001020"},{"id":"T125","span":{"begin":15160,"end":15167},"obj":"http://purl.obolibrary.org/obo/UBERON_0000473"},{"id":"T126","span":{"begin":15374,"end":15375},"obj":"http://purl.obolibrary.org/obo/CLO_0001020"}],"text":"Method\n\nParticipants and Recruitment\nSamples of Australian (N = 495, 50.1% female) and U.S. (N = 701, 48.9% female) residents were recruited via an online research panel company. To be eligible for inclusion, participants needed to be aged 18 years or older and not subject to formal quarantine for COVID-19. Participants were also screened for age, gender, and geographical region and quotas imposed during recruitment to ensure that the final samples closely matched the national distributions for these characteristics in each country. Data were collected between April 1 and May 6, 2020. All participants in the Australian sample were subject to a national “shelter-in-place” order issued by the federal government. However, issuance of orders in the USA was devolved to state governments resulting in some variations. The vast majority of participants in the U.S. sample (n = 610, 87.0%) were subject to “shelter-in-place” orders for the duration of the study. However, some states did not impose “shelter-in-place” orders at all (Arkansas, Iowa, Nebraska, North Dakota, South Dakota, Utah, and Wyoming), so a minority of participants in the U.S. sample (n = 37, 5.3%) were never subject to an order. Furthermore, in some cases in the U.S. sample (n = 47, 6.7%), “shelter-in-place” orders had been lifted prior to follow-up data collection. However, among the states in the USA that did not have “shelter-in-place” orders, or lifted their orders during the study, all issued social distancing guidelines and encouraged the population to follow those guidelines. Baseline sample characteristics are presented in Table 1.\nTable 1. Sample characteristics and descriptive statistics for study variables at baseline and at 1 week follow-up\nVariable Australia sample U.S. sample\nBaseline Follow-up Baseline Follow-up\nParticipants 495 365 701 440\nAge, M years (SD) 47.09 (17.11) 49.78 (16.89) 45.55 (17.40) 51.77 (16.26)\nGender, n (%)a\n Female 252 (51.1) 182 (50.1) 352 (48.9) 205 (46.6)\n Male 241 (48.9) 181 (49.9) 341 (50.5) 231 (52.5)\n Not specified/prefer not to answer 0 (0.0) 0 (0.0) 4 (0.6) 4 (0.9)\nEmployment status, n (%)b\n Currently unemployed/full-time caregiver 231 (46.7) 180 (49.3) 330 (47.3) 216 (49.5)\n Part-time/casual employed 97 (19.6) 65 (17.8) 106 (15.2) 60 (13.8)\n Currently employed full time 140 (28.3) 104 (28.5) 233 (33.4) 147 (33.7)\n Leave without pay/furloughed 27 (5.5) 16 (4.4) 28 (4.0) 13 (3.0)\nMarital status, n (%)c\n Married 184 (37.2) 146 (40.0) 300 (43.0) 224 (51.4)\n Widowed 8 (1.6) 7 (1.9) 22 (3.2) 18 (4.1)\n Separated/divorced 53 (10.7) 39 (10.7) 69 (9.9) 47 (10.8)\n Never married 160 (32.3) 103 (28.2) 255 (36.6) 126(28.9)\n Married de facto 90 (18.2) 70 (19.2) 51 (7.3) 21 (4.8)\nEthnicity, n (%)d\n Black 3 (0.6) 1 (0.3) 52 (7.5) 26 (6.0)\n Caucasian/White 392 (79.2) 304 (83.3) 566 (81.2) 376 (86.2)\n Asian (South-East Asia/South Asia) 71 (14.3) 43 (11.8) 39 (5.6) 24 (5.5)\n Middle-Eastern 6 (1.2) 3 (0.8) 1 (0.1) 0 (0.0)\n Other 13 (2.6) 6 (1.6) 27 (3.9) 8 (1.8)\n Prefer not to answer 10 (2.0) 8 (2.2) 12 (1.7) 2 (0.5)\nIncome, n (%)e\n Zero income 8 (1.7) 4 (1.2) 31 (4.4) 19 (4.4)\n $1–$199 ($1–$10,399) 9 (2.0) 6 (1.8) 40 (5.7) 24 (5.5)\n $200–$299 ($10,400–$15,599) 12 (2.6) 8 (2.4) 34 (4.9) 23 (5.3)\n $300–$399 ($15,600–$20,799) 19 (4.1) 12 (3.6) 38 (5.5) 23 (5.3)\n $400–$599 ($20,800–$31,199) 42 (9.2) 33 (9.9) 62 (8.9) 33 (7.6)\n $600–$799 ($31,200–$41,599) 57 (12.4) 42 (12.6) 61 (8.8) 39 (8.9)\n $800–$999 ($41,600–$51,999) 45 (9.8) 31 (9.3) 68 (9.8) 46 (10.6)\n $1,000–$1,249 ($52,000–$64,999) 39 (8.5) 32 (9.6) 48 (6.9) 38 (8.7)\n $1,250–$1,499 ($65,000–$77,999) 28 (6.1) 22 (6.6) 59 (8.5) 41 (9.4)\n $1,500–$1,999 ($78,000–$103,999) 72 (15.7) 50 (15.0) 72 (10.3) 48 (11.0)\n $2,000 or more ($104,000 or more) 81 (17.6) 62 (18.6) 108 (15.5) 74 (17.0)\n Prefer not to answer 47 (10.2) 32 (9.6) 76 (10.9) 28 (6.4)\nEducation level, n (%)\n Completed junior/lower/primary school 18 (3.6) 17 (4.7) 6 (0.9) 0 (0.0)\n Completed senior/high/secondary school 133 (26.9) 98 (26.8) 265 (37.8) 132 (30.0)\n Postschool vocational qualification/diploma 147 (29.7) 111 (30.4) 138 (19.7) 94 (21.4)\n Undergraduate university degree 131 (26.5) 93 (25.5) 214 (30.5) 159 (36.1)\n Postgraduate university degree 66 (13.3) 46 (12.6) 78 (11.1) 55 (12.5)\nSD standard deviation.\naTwo participants in the Australian sample did not report their gender and four participants in the U.S. sample did not report their gender.\nbFour participants in the U.S. sample did not report their employment status.\ncFour participants in the U.S. sample did not report their marital status.\ndFour participants in the U.S. sample did not report their ethnicity.\neThirty-one participants in the Australian sample did not report their income and four participants in the U.S. sample did not report their income.\n\nDesign and Procedure\nThe study adopted a prospective correlational design with self-report measures of social cognition constructs from the proposed integrated model, intention, and past social distancing behavior administered at an initial data collection occasion in a survey administered using the Qualtrics online survey tool. Social cognition measures included the theory of planned behavior (attitude, subjective norm, and PBC), moral norm, anticipated regret, action planning, and habit constructs. Participants were informed that they were participating in a survey on their social distancing behavior and provided with information outlining study requirements. They were required to provide informed consent before proceeding with the survey. Participants were also provided with instructions on how to complete study measures and a definition of the target behavior: “The following survey will ask about your beliefs and attitudes about ‘social distancing’. What do we mean by social distancing? Social distancing (also known as ‘physical distancing’) is deliberately increasing the physical space between people to avoid spreading illness. The World Health Organization and other world leading health authorities suggest that you should maintain at least a 1–2 meter (3–6 feet) distance from other people to lessen the chances of getting infected with COVID-19. When answering the questions in this survey, think about your social distancing behavior (i.e., maintaining at least a 1–2 m (3–6 ft) distance from other people).” One week later, participants were re-contacted by the panel company and asked to self-report their habit and social distancing behavior over the previous week using the same measures used at the initial data collection occasion. Participants received a fixed sum of money for their participation based on expected completion time consistent with the panel company’s published rates. Approval for study procedures was granted prior to data collection from the Griffith University Human Research Ethics Committee.\n\nMeasures\nStudy measures were multi-item self-report measures of constructs based on published guidelines and measures used in previous studies [13, 29, 30]. Participants provided their responses on scales with seven-point response options. Complete study measures are provided in Supplementary Appendix A.\n\nSocial cognition constructs\nMulti-item measures of attitude, subjective norm, PBC, moral norm, anticipated regret, and action planning were developed according to published guidelines [13, 29]. Each measure made explicit reference to the target behavior of social distancing, and participants were reminded of the definition of social distancing before completing the measures.\n\nIntention\nParticipants’ intention to participate in social distancing behavior over the next week was measured using a scale developed according to published guidelines [31].\n\nHabit\nHabit was measured at both time points using the behavioral automaticity items of Verplanken and Orbell’s self-report habit index [25]. The measure measures individuals’ reflections on the extent to which the behavior is experienced as automatic and enacted without thought.\n\nPast behavior and behavior\nParticipants self-reported their participation in social distancing behavior to minimize the transmission of the SARS-CoV-2 virus that causes COVID-19. The measure comprised two items prompting participants to report their frequency of social distancing behavior in the previous week. This is based on previously used self-report behavioral measures that have demonstrated concurrent validity with non-self-report measures in other behavioral contexts [32].\n\nDemographic variables\nParticipants self-reported their age in years, gender, employment status (currently unemployed/full-time caregiver, currently full-time employed, part-time employed, or on leave without pay/furloughed), marital status (married, widowed, separated/divorced, never married, or in a de factor relationship), annual household income stratified by 11 income levels based on Australian and U.S. national averages, highest level of formal education (completed junior/lower/primary school, completed senior/high/secondary school, postschool vocational qualification/diploma, further education diploma, undergraduate university degree, or postgraduate university degree), and ethnicity (Black, Caucasian/White, Asian, or Middle-eastern). Binary income (low income vs. middle/high income), highest education level (completed school education only vs. completed postschool education), and ethnicity (Caucasian/White vs. non-White) variables were computed for use in subsequent analyses.\n\nData Analysis\nHypothesized relations among the integrated model constructs were tested in the Australian and U.S. samples separately using variance-based structural equation modeling implemented in the WARP 7.0 analysis package [33]. Model parameters and standard errors (SEs) were computed using the “Stable3” estimation method, which has been shown to provide the most precise parameter estimates in complex structural models in smaller samples and outperforms bootstrapping methods in simulation studies [33]. Simulation studies have also shown this method to provide more consistent and precise estimates in data containing outliers, which may inflate SEs and lead to abnormally high p-values [33]. Two models were estimated in each sample: a model testing predictions of the proposed integrated model with the binary demographic variables also included as covariates (Model 1; Fig. 1, upper panel) and a model that included effects of past social distancing behavior (Model 2; Fig. 1, lower panel). All constructs were latent variables indicated by single or multiple items. There were no missing data for the social cognition and self-reported behavioral variables. There were a few instances of missing data for the demographic variables ranging from 0.5% to 8.8% in the Australia sample, and 0.9% to 6.4% in the U.S. sample. Missing data are reported in Supplementary Appendix B. Missing data were imputed using stochastic hierarchical regression [33].\nThe analysis afforded a number of analyses to evaluate the adequacy of measures used to indicate the latent variables in the model. Construct validity of the latent factors for the social cognition, intention, and behavioral variables was established using the normalized factor pattern loadings after oblique rotation and Kaiser normalization [33] and the average variance extracted (AVE), which should approach or exceed .700 and .500, respectively. Internal consistency of the factors was estimated using omega reliability coefficients (ω) and composite reliability coefficients (ρ), which should exceed .700 and ideally approach .900. We also conducted tests of the discriminant validity of the constructs in the model. Discriminant validity was supported when the square root of the AVE for each latent variable exceeded its correlation with other latent variables.\nAdequacy of the proposed model in describing the data was established using the goodness-of-fit (GoF) index with values of .100, .250, and .360 corresponding to small, medium, and large effect sizes. Further information on model quality was provided by the average path coefficient and average R2 coefficient. These indices summarize the average parameter estimates of relations in the model and the amount of variance explained in each dependent variable, respectively, and should be statistically significant for a good-quality model. In addition, an overall GoF index is provided by the average block variance inflation factor for model parameters and the average full collinearity variance inflation factor, which should be equal to or lower than 3.3 for well-fitting models. These indices indicate the extent to which latent variables in the model overlap and contribute to model multicollinearity. They, therefore, provide an indication as to the uniqueness of the existing latent variables in the model. Four further indices were also used to evaluate model quality: the Simpson’s paradox ratio (SPR), R2 contribution ratio (R2CR), the statistical suppression ratio (SSR), and the nonlinear bivariate causality direction ratio (NLBCDR). The SPR indicates whether the model is free from incidences of Simpson’s paradox (i.e., when the path coefficient and the correlation associated with a latent variable have opposite signs), indicating a causality problem. The SPR should exceed .700 and ideally approach 1.000. The R2CR and SSR provide indication of the extent to which models are free from instances of negative R2 contributions and statistical suppression. The R2CR and SSR should exceed .900 and .700, respectively. The NLBCDR provides an estimate of the extent to which the proposed “causal” associations in the proposed model are more tenable than those in the opposite direction and provide an initial indicator of support for the hypothesized directions of the causal links in the proposed model compared to if the proposed direction were reversed. The NLBCDR should exceed .700 for high-quality models. Kock [33] provides further technical details on model fit and quality indices.\nModel effects were estimated using standardized path coefficients with confidence intervals (CIs) and test statistics. Effect sizes were estimated using a variant of Cohen’s f-square coefficient and represent the individual contribution of the predictor variable to the R2 coefficients of the criterion latent variable. Values of .02, .15, and .35 represent small, medium, and large effect sizes, respectively. Differences in the path coefficients in the models across the samples were tested using multiple-group analysis using the Satterthwaite method with two-tailed significance tests.\nWe also tested whether the inclusion of participants that were never under a “shelter-in-place” order, or had the “shelter-in-place” order lifted during the study, affected predicted relations in the models. The small numbers of participants that were, at some point, not subjected to “shelter-in-place” orders meant we could not conduct a formal moderator analysis, so we conducted a sensitivity analysis testing whether effects in the models differed if data from these participants were excluded. Models excluding and including past behavior were estimated in samples excluding participants who were never subject to a “shelter-in place” order, and in the sample that were never subject to an order, or who had the order lifted at some stage during the study. Formal comparisons of parameter estimates in these models with those from the full sample were made using the Satterthwaite method. Data files, analysis scripts, and output files for all analyses are available online: https://osf.io/x9tms/."}
LitCovid-PD-CHEBI
{"project":"LitCovid-PD-CHEBI","denotations":[{"id":"T6","span":{"begin":277,"end":283},"obj":"Chemical"},{"id":"T7","span":{"begin":1869,"end":1871},"obj":"Chemical"},{"id":"T8","span":{"begin":4417,"end":4419},"obj":"Chemical"},{"id":"T9","span":{"begin":9080,"end":9086},"obj":"Chemical"},{"id":"T10","span":{"begin":13138,"end":13141},"obj":"Chemical"},{"id":"T11","span":{"begin":13498,"end":13501},"obj":"Chemical"},{"id":"T12","span":{"begin":13646,"end":13649},"obj":"Chemical"},{"id":"T13","span":{"begin":13882,"end":13891},"obj":"Chemical"},{"id":"T14","span":{"begin":14672,"end":14677},"obj":"Chemical"},{"id":"T15","span":{"begin":15094,"end":15100},"obj":"Chemical"}],"attributes":[{"id":"A6","pred":"chebi_id","subj":"T6","obj":"http://purl.obolibrary.org/obo/CHEBI_48341"},{"id":"A7","pred":"chebi_id","subj":"T7","obj":"http://purl.obolibrary.org/obo/CHEBI_74807"},{"id":"A8","pred":"chebi_id","subj":"T8","obj":"http://purl.obolibrary.org/obo/CHEBI_74807"},{"id":"A9","pred":"chebi_id","subj":"T9","obj":"http://purl.obolibrary.org/obo/CHEBI_48341"},{"id":"A10","pred":"chebi_id","subj":"T10","obj":"http://purl.obolibrary.org/obo/CHEBI_73280"},{"id":"A11","pred":"chebi_id","subj":"T11","obj":"http://purl.obolibrary.org/obo/CHEBI_73280"},{"id":"A12","pred":"chebi_id","subj":"T12","obj":"http://purl.obolibrary.org/obo/CHEBI_73280"},{"id":"A13","pred":"chebi_id","subj":"T13","obj":"http://purl.obolibrary.org/obo/CHEBI_47867"},{"id":"A14","pred":"chebi_id","subj":"T14","obj":"http://purl.obolibrary.org/obo/CHEBI_24433"},{"id":"A15","pred":"chebi_id","subj":"T15","obj":"http://purl.obolibrary.org/obo/CHEBI_48341"}],"text":"Method\n\nParticipants and Recruitment\nSamples of Australian (N = 495, 50.1% female) and U.S. (N = 701, 48.9% female) residents were recruited via an online research panel company. To be eligible for inclusion, participants needed to be aged 18 years or older and not subject to formal quarantine for COVID-19. Participants were also screened for age, gender, and geographical region and quotas imposed during recruitment to ensure that the final samples closely matched the national distributions for these characteristics in each country. Data were collected between April 1 and May 6, 2020. All participants in the Australian sample were subject to a national “shelter-in-place” order issued by the federal government. However, issuance of orders in the USA was devolved to state governments resulting in some variations. The vast majority of participants in the U.S. sample (n = 610, 87.0%) were subject to “shelter-in-place” orders for the duration of the study. However, some states did not impose “shelter-in-place” orders at all (Arkansas, Iowa, Nebraska, North Dakota, South Dakota, Utah, and Wyoming), so a minority of participants in the U.S. sample (n = 37, 5.3%) were never subject to an order. Furthermore, in some cases in the U.S. sample (n = 47, 6.7%), “shelter-in-place” orders had been lifted prior to follow-up data collection. However, among the states in the USA that did not have “shelter-in-place” orders, or lifted their orders during the study, all issued social distancing guidelines and encouraged the population to follow those guidelines. Baseline sample characteristics are presented in Table 1.\nTable 1. Sample characteristics and descriptive statistics for study variables at baseline and at 1 week follow-up\nVariable Australia sample U.S. sample\nBaseline Follow-up Baseline Follow-up\nParticipants 495 365 701 440\nAge, M years (SD) 47.09 (17.11) 49.78 (16.89) 45.55 (17.40) 51.77 (16.26)\nGender, n (%)a\n Female 252 (51.1) 182 (50.1) 352 (48.9) 205 (46.6)\n Male 241 (48.9) 181 (49.9) 341 (50.5) 231 (52.5)\n Not specified/prefer not to answer 0 (0.0) 0 (0.0) 4 (0.6) 4 (0.9)\nEmployment status, n (%)b\n Currently unemployed/full-time caregiver 231 (46.7) 180 (49.3) 330 (47.3) 216 (49.5)\n Part-time/casual employed 97 (19.6) 65 (17.8) 106 (15.2) 60 (13.8)\n Currently employed full time 140 (28.3) 104 (28.5) 233 (33.4) 147 (33.7)\n Leave without pay/furloughed 27 (5.5) 16 (4.4) 28 (4.0) 13 (3.0)\nMarital status, n (%)c\n Married 184 (37.2) 146 (40.0) 300 (43.0) 224 (51.4)\n Widowed 8 (1.6) 7 (1.9) 22 (3.2) 18 (4.1)\n Separated/divorced 53 (10.7) 39 (10.7) 69 (9.9) 47 (10.8)\n Never married 160 (32.3) 103 (28.2) 255 (36.6) 126(28.9)\n Married de facto 90 (18.2) 70 (19.2) 51 (7.3) 21 (4.8)\nEthnicity, n (%)d\n Black 3 (0.6) 1 (0.3) 52 (7.5) 26 (6.0)\n Caucasian/White 392 (79.2) 304 (83.3) 566 (81.2) 376 (86.2)\n Asian (South-East Asia/South Asia) 71 (14.3) 43 (11.8) 39 (5.6) 24 (5.5)\n Middle-Eastern 6 (1.2) 3 (0.8) 1 (0.1) 0 (0.0)\n Other 13 (2.6) 6 (1.6) 27 (3.9) 8 (1.8)\n Prefer not to answer 10 (2.0) 8 (2.2) 12 (1.7) 2 (0.5)\nIncome, n (%)e\n Zero income 8 (1.7) 4 (1.2) 31 (4.4) 19 (4.4)\n $1–$199 ($1–$10,399) 9 (2.0) 6 (1.8) 40 (5.7) 24 (5.5)\n $200–$299 ($10,400–$15,599) 12 (2.6) 8 (2.4) 34 (4.9) 23 (5.3)\n $300–$399 ($15,600–$20,799) 19 (4.1) 12 (3.6) 38 (5.5) 23 (5.3)\n $400–$599 ($20,800–$31,199) 42 (9.2) 33 (9.9) 62 (8.9) 33 (7.6)\n $600–$799 ($31,200–$41,599) 57 (12.4) 42 (12.6) 61 (8.8) 39 (8.9)\n $800–$999 ($41,600–$51,999) 45 (9.8) 31 (9.3) 68 (9.8) 46 (10.6)\n $1,000–$1,249 ($52,000–$64,999) 39 (8.5) 32 (9.6) 48 (6.9) 38 (8.7)\n $1,250–$1,499 ($65,000–$77,999) 28 (6.1) 22 (6.6) 59 (8.5) 41 (9.4)\n $1,500–$1,999 ($78,000–$103,999) 72 (15.7) 50 (15.0) 72 (10.3) 48 (11.0)\n $2,000 or more ($104,000 or more) 81 (17.6) 62 (18.6) 108 (15.5) 74 (17.0)\n Prefer not to answer 47 (10.2) 32 (9.6) 76 (10.9) 28 (6.4)\nEducation level, n (%)\n Completed junior/lower/primary school 18 (3.6) 17 (4.7) 6 (0.9) 0 (0.0)\n Completed senior/high/secondary school 133 (26.9) 98 (26.8) 265 (37.8) 132 (30.0)\n Postschool vocational qualification/diploma 147 (29.7) 111 (30.4) 138 (19.7) 94 (21.4)\n Undergraduate university degree 131 (26.5) 93 (25.5) 214 (30.5) 159 (36.1)\n Postgraduate university degree 66 (13.3) 46 (12.6) 78 (11.1) 55 (12.5)\nSD standard deviation.\naTwo participants in the Australian sample did not report their gender and four participants in the U.S. sample did not report their gender.\nbFour participants in the U.S. sample did not report their employment status.\ncFour participants in the U.S. sample did not report their marital status.\ndFour participants in the U.S. sample did not report their ethnicity.\neThirty-one participants in the Australian sample did not report their income and four participants in the U.S. sample did not report their income.\n\nDesign and Procedure\nThe study adopted a prospective correlational design with self-report measures of social cognition constructs from the proposed integrated model, intention, and past social distancing behavior administered at an initial data collection occasion in a survey administered using the Qualtrics online survey tool. Social cognition measures included the theory of planned behavior (attitude, subjective norm, and PBC), moral norm, anticipated regret, action planning, and habit constructs. Participants were informed that they were participating in a survey on their social distancing behavior and provided with information outlining study requirements. They were required to provide informed consent before proceeding with the survey. Participants were also provided with instructions on how to complete study measures and a definition of the target behavior: “The following survey will ask about your beliefs and attitudes about ‘social distancing’. What do we mean by social distancing? Social distancing (also known as ‘physical distancing’) is deliberately increasing the physical space between people to avoid spreading illness. The World Health Organization and other world leading health authorities suggest that you should maintain at least a 1–2 meter (3–6 feet) distance from other people to lessen the chances of getting infected with COVID-19. When answering the questions in this survey, think about your social distancing behavior (i.e., maintaining at least a 1–2 m (3–6 ft) distance from other people).” One week later, participants were re-contacted by the panel company and asked to self-report their habit and social distancing behavior over the previous week using the same measures used at the initial data collection occasion. Participants received a fixed sum of money for their participation based on expected completion time consistent with the panel company’s published rates. Approval for study procedures was granted prior to data collection from the Griffith University Human Research Ethics Committee.\n\nMeasures\nStudy measures were multi-item self-report measures of constructs based on published guidelines and measures used in previous studies [13, 29, 30]. Participants provided their responses on scales with seven-point response options. Complete study measures are provided in Supplementary Appendix A.\n\nSocial cognition constructs\nMulti-item measures of attitude, subjective norm, PBC, moral norm, anticipated regret, and action planning were developed according to published guidelines [13, 29]. Each measure made explicit reference to the target behavior of social distancing, and participants were reminded of the definition of social distancing before completing the measures.\n\nIntention\nParticipants’ intention to participate in social distancing behavior over the next week was measured using a scale developed according to published guidelines [31].\n\nHabit\nHabit was measured at both time points using the behavioral automaticity items of Verplanken and Orbell’s self-report habit index [25]. The measure measures individuals’ reflections on the extent to which the behavior is experienced as automatic and enacted without thought.\n\nPast behavior and behavior\nParticipants self-reported their participation in social distancing behavior to minimize the transmission of the SARS-CoV-2 virus that causes COVID-19. The measure comprised two items prompting participants to report their frequency of social distancing behavior in the previous week. This is based on previously used self-report behavioral measures that have demonstrated concurrent validity with non-self-report measures in other behavioral contexts [32].\n\nDemographic variables\nParticipants self-reported their age in years, gender, employment status (currently unemployed/full-time caregiver, currently full-time employed, part-time employed, or on leave without pay/furloughed), marital status (married, widowed, separated/divorced, never married, or in a de factor relationship), annual household income stratified by 11 income levels based on Australian and U.S. national averages, highest level of formal education (completed junior/lower/primary school, completed senior/high/secondary school, postschool vocational qualification/diploma, further education diploma, undergraduate university degree, or postgraduate university degree), and ethnicity (Black, Caucasian/White, Asian, or Middle-eastern). Binary income (low income vs. middle/high income), highest education level (completed school education only vs. completed postschool education), and ethnicity (Caucasian/White vs. non-White) variables were computed for use in subsequent analyses.\n\nData Analysis\nHypothesized relations among the integrated model constructs were tested in the Australian and U.S. samples separately using variance-based structural equation modeling implemented in the WARP 7.0 analysis package [33]. Model parameters and standard errors (SEs) were computed using the “Stable3” estimation method, which has been shown to provide the most precise parameter estimates in complex structural models in smaller samples and outperforms bootstrapping methods in simulation studies [33]. Simulation studies have also shown this method to provide more consistent and precise estimates in data containing outliers, which may inflate SEs and lead to abnormally high p-values [33]. Two models were estimated in each sample: a model testing predictions of the proposed integrated model with the binary demographic variables also included as covariates (Model 1; Fig. 1, upper panel) and a model that included effects of past social distancing behavior (Model 2; Fig. 1, lower panel). All constructs were latent variables indicated by single or multiple items. There were no missing data for the social cognition and self-reported behavioral variables. There were a few instances of missing data for the demographic variables ranging from 0.5% to 8.8% in the Australia sample, and 0.9% to 6.4% in the U.S. sample. Missing data are reported in Supplementary Appendix B. Missing data were imputed using stochastic hierarchical regression [33].\nThe analysis afforded a number of analyses to evaluate the adequacy of measures used to indicate the latent variables in the model. Construct validity of the latent factors for the social cognition, intention, and behavioral variables was established using the normalized factor pattern loadings after oblique rotation and Kaiser normalization [33] and the average variance extracted (AVE), which should approach or exceed .700 and .500, respectively. Internal consistency of the factors was estimated using omega reliability coefficients (ω) and composite reliability coefficients (ρ), which should exceed .700 and ideally approach .900. We also conducted tests of the discriminant validity of the constructs in the model. Discriminant validity was supported when the square root of the AVE for each latent variable exceeded its correlation with other latent variables.\nAdequacy of the proposed model in describing the data was established using the goodness-of-fit (GoF) index with values of .100, .250, and .360 corresponding to small, medium, and large effect sizes. Further information on model quality was provided by the average path coefficient and average R2 coefficient. These indices summarize the average parameter estimates of relations in the model and the amount of variance explained in each dependent variable, respectively, and should be statistically significant for a good-quality model. In addition, an overall GoF index is provided by the average block variance inflation factor for model parameters and the average full collinearity variance inflation factor, which should be equal to or lower than 3.3 for well-fitting models. These indices indicate the extent to which latent variables in the model overlap and contribute to model multicollinearity. They, therefore, provide an indication as to the uniqueness of the existing latent variables in the model. Four further indices were also used to evaluate model quality: the Simpson’s paradox ratio (SPR), R2 contribution ratio (R2CR), the statistical suppression ratio (SSR), and the nonlinear bivariate causality direction ratio (NLBCDR). The SPR indicates whether the model is free from incidences of Simpson’s paradox (i.e., when the path coefficient and the correlation associated with a latent variable have opposite signs), indicating a causality problem. The SPR should exceed .700 and ideally approach 1.000. The R2CR and SSR provide indication of the extent to which models are free from instances of negative R2 contributions and statistical suppression. The R2CR and SSR should exceed .900 and .700, respectively. The NLBCDR provides an estimate of the extent to which the proposed “causal” associations in the proposed model are more tenable than those in the opposite direction and provide an initial indicator of support for the hypothesized directions of the causal links in the proposed model compared to if the proposed direction were reversed. The NLBCDR should exceed .700 for high-quality models. Kock [33] provides further technical details on model fit and quality indices.\nModel effects were estimated using standardized path coefficients with confidence intervals (CIs) and test statistics. Effect sizes were estimated using a variant of Cohen’s f-square coefficient and represent the individual contribution of the predictor variable to the R2 coefficients of the criterion latent variable. Values of .02, .15, and .35 represent small, medium, and large effect sizes, respectively. Differences in the path coefficients in the models across the samples were tested using multiple-group analysis using the Satterthwaite method with two-tailed significance tests.\nWe also tested whether the inclusion of participants that were never under a “shelter-in-place” order, or had the “shelter-in-place” order lifted during the study, affected predicted relations in the models. The small numbers of participants that were, at some point, not subjected to “shelter-in-place” orders meant we could not conduct a formal moderator analysis, so we conducted a sensitivity analysis testing whether effects in the models differed if data from these participants were excluded. Models excluding and including past behavior were estimated in samples excluding participants who were never subject to a “shelter-in place” order, and in the sample that were never subject to an order, or who had the order lifted at some stage during the study. Formal comparisons of parameter estimates in these models with those from the full sample were made using the Satterthwaite method. Data files, analysis scripts, and output files for all analyses are available online: https://osf.io/x9tms/."}
LitCovid-PubTator
{"project":"LitCovid-PubTator","denotations":[{"id":"63","span":{"begin":8,"end":20},"obj":"Species"},{"id":"70","span":{"begin":209,"end":221},"obj":"Species"},{"id":"71","span":{"begin":309,"end":321},"obj":"Species"},{"id":"72","span":{"begin":596,"end":608},"obj":"Species"},{"id":"73","span":{"begin":844,"end":856},"obj":"Species"},{"id":"74","span":{"begin":1127,"end":1139},"obj":"Species"},{"id":"75","span":{"begin":299,"end":307},"obj":"Disease"},{"id":"79","span":{"begin":1822,"end":1834},"obj":"Species"},{"id":"80","span":{"begin":2059,"end":2072},"obj":"Species"},{"id":"81","span":{"begin":4206,"end":4213},"obj":"Disease"},{"id":"84","span":{"begin":4445,"end":4457},"obj":"Species"},{"id":"85","span":{"begin":4520,"end":4532},"obj":"Species"},{"id":"87","span":{"begin":4587,"end":4599},"obj":"Species"},{"id":"89","span":{"begin":4665,"end":4677},"obj":"Species"},{"id":"91","span":{"begin":4740,"end":4752},"obj":"Species"},{"id":"94","span":{"begin":4816,"end":4828},"obj":"Species"},{"id":"95","span":{"begin":4891,"end":4903},"obj":"Species"},{"id":"106","span":{"begin":5459,"end":5471},"obj":"Species"},{"id":"107","span":{"begin":5705,"end":5717},"obj":"Species"},{"id":"108","span":{"begin":6069,"end":6075},"obj":"Species"},{"id":"109","span":{"begin":6262,"end":6268},"obj":"Species"},{"id":"110","span":{"begin":6480,"end":6486},"obj":"Species"},{"id":"111","span":{"begin":6506,"end":6518},"obj":"Species"},{"id":"112","span":{"begin":6719,"end":6731},"obj":"Species"},{"id":"113","span":{"begin":6969,"end":6974},"obj":"Species"},{"id":"114","span":{"begin":6302,"end":6310},"obj":"Disease"},{"id":"115","span":{"begin":6316,"end":6324},"obj":"Disease"},{"id":"117","span":{"begin":7160,"end":7172},"obj":"Species"},{"id":"119","span":{"begin":7590,"end":7602},"obj":"Species"},{"id":"121","span":{"begin":7699,"end":7711},"obj":"Species"},{"id":"126","span":{"begin":8174,"end":8186},"obj":"Species"},{"id":"127","span":{"begin":8287,"end":8297},"obj":"Species"},{"id":"128","span":{"begin":8368,"end":8380},"obj":"Species"},{"id":"129","span":{"begin":8316,"end":8324},"obj":"Disease"},{"id":"133","span":{"begin":8655,"end":8667},"obj":"Species"},{"id":"134","span":{"begin":9213,"end":9220},"obj":"Disease"},{"id":"135","span":{"begin":9240,"end":9247},"obj":"Disease"},{"id":"137","span":{"begin":10505,"end":10520},"obj":"Gene"},{"id":"139","span":{"begin":11395,"end":11411},"obj":"Disease"},{"id":"144","span":{"begin":14794,"end":14806},"obj":"Species"},{"id":"145","span":{"begin":14983,"end":14995},"obj":"Species"},{"id":"146","span":{"begin":15226,"end":15238},"obj":"Species"},{"id":"147","span":{"begin":15335,"end":15347},"obj":"Species"}],"attributes":[{"id":"A63","pred":"tao:has_database_id","subj":"63","obj":"Tax:9606"},{"id":"A70","pred":"tao:has_database_id","subj":"70","obj":"Tax:9606"},{"id":"A71","pred":"tao:has_database_id","subj":"71","obj":"Tax:9606"},{"id":"A72","pred":"tao:has_database_id","subj":"72","obj":"Tax:9606"},{"id":"A73","pred":"tao:has_database_id","subj":"73","obj":"Tax:9606"},{"id":"A74","pred":"tao:has_database_id","subj":"74","obj":"Tax:9606"},{"id":"A75","pred":"tao:has_database_id","subj":"75","obj":"MESH:C000657245"},{"id":"A79","pred":"tao:has_database_id","subj":"79","obj":"Tax:9606"},{"id":"A80","pred":"tao:has_database_id","subj":"80","obj":"Tax:32644"},{"id":"A84","pred":"tao:has_database_id","subj":"84","obj":"Tax:9606"},{"id":"A85","pred":"tao:has_database_id","subj":"85","obj":"Tax:9606"},{"id":"A87","pred":"tao:has_database_id","subj":"87","obj":"Tax:9606"},{"id":"A89","pred":"tao:has_database_id","subj":"89","obj":"Tax:9606"},{"id":"A91","pred":"tao:has_database_id","subj":"91","obj":"Tax:9606"},{"id":"A94","pred":"tao:has_database_id","subj":"94","obj":"Tax:9606"},{"id":"A95","pred":"tao:has_database_id","subj":"95","obj":"Tax:9606"},{"id":"A106","pred":"tao:has_database_id","subj":"106","obj":"Tax:9606"},{"id":"A107","pred":"tao:has_database_id","subj":"107","obj":"Tax:9606"},{"id":"A108","pred":"tao:has_database_id","subj":"108","obj":"Tax:9606"},{"id":"A109","pred":"tao:has_database_id","subj":"109","obj":"Tax:9606"},{"id":"A110","pred":"tao:has_database_id","subj":"110","obj":"Tax:9606"},{"id":"A111","pred":"tao:has_database_id","subj":"111","obj":"Tax:9606"},{"id":"A112","pred":"tao:has_database_id","subj":"112","obj":"Tax:9606"},{"id":"A113","pred":"tao:has_database_id","subj":"113","obj":"Tax:9606"},{"id":"A114","pred":"tao:has_database_id","subj":"114","obj":"MESH:D007239"},{"id":"A115","pred":"tao:has_database_id","subj":"115","obj":"MESH:C000657245"},{"id":"A117","pred":"tao:has_database_id","subj":"117","obj":"Tax:9606"},{"id":"A119","pred":"tao:has_database_id","subj":"119","obj":"Tax:9606"},{"id":"A121","pred":"tao:has_database_id","subj":"121","obj":"Tax:9606"},{"id":"A126","pred":"tao:has_database_id","subj":"126","obj":"Tax:9606"},{"id":"A127","pred":"tao:has_database_id","subj":"127","obj":"Tax:2697049"},{"id":"A128","pred":"tao:has_database_id","subj":"128","obj":"Tax:9606"},{"id":"A129","pred":"tao:has_database_id","subj":"129","obj":"MESH:C000657245"},{"id":"A133","pred":"tao:has_database_id","subj":"133","obj":"Tax:9606"},{"id":"A137","pred":"tao:has_database_id","subj":"137","obj":"Gene:259307"},{"id":"A139","pred":"tao:has_database_id","subj":"139","obj":"MESH:D009069"},{"id":"A144","pred":"tao:has_database_id","subj":"144","obj":"Tax:9606"},{"id":"A145","pred":"tao:has_database_id","subj":"145","obj":"Tax:9606"},{"id":"A146","pred":"tao:has_database_id","subj":"146","obj":"Tax:9606"},{"id":"A147","pred":"tao:has_database_id","subj":"147","obj":"Tax:9606"}],"namespaces":[{"prefix":"Tax","uri":"https://www.ncbi.nlm.nih.gov/taxonomy/"},{"prefix":"MESH","uri":"https://id.nlm.nih.gov/mesh/"},{"prefix":"Gene","uri":"https://www.ncbi.nlm.nih.gov/gene/"},{"prefix":"CVCL","uri":"https://web.expasy.org/cellosaurus/CVCL_"}],"text":"Method\n\nParticipants and Recruitment\nSamples of Australian (N = 495, 50.1% female) and U.S. (N = 701, 48.9% female) residents were recruited via an online research panel company. To be eligible for inclusion, participants needed to be aged 18 years or older and not subject to formal quarantine for COVID-19. Participants were also screened for age, gender, and geographical region and quotas imposed during recruitment to ensure that the final samples closely matched the national distributions for these characteristics in each country. Data were collected between April 1 and May 6, 2020. All participants in the Australian sample were subject to a national “shelter-in-place” order issued by the federal government. However, issuance of orders in the USA was devolved to state governments resulting in some variations. The vast majority of participants in the U.S. sample (n = 610, 87.0%) were subject to “shelter-in-place” orders for the duration of the study. However, some states did not impose “shelter-in-place” orders at all (Arkansas, Iowa, Nebraska, North Dakota, South Dakota, Utah, and Wyoming), so a minority of participants in the U.S. sample (n = 37, 5.3%) were never subject to an order. Furthermore, in some cases in the U.S. sample (n = 47, 6.7%), “shelter-in-place” orders had been lifted prior to follow-up data collection. However, among the states in the USA that did not have “shelter-in-place” orders, or lifted their orders during the study, all issued social distancing guidelines and encouraged the population to follow those guidelines. Baseline sample characteristics are presented in Table 1.\nTable 1. Sample characteristics and descriptive statistics for study variables at baseline and at 1 week follow-up\nVariable Australia sample U.S. sample\nBaseline Follow-up Baseline Follow-up\nParticipants 495 365 701 440\nAge, M years (SD) 47.09 (17.11) 49.78 (16.89) 45.55 (17.40) 51.77 (16.26)\nGender, n (%)a\n Female 252 (51.1) 182 (50.1) 352 (48.9) 205 (46.6)\n Male 241 (48.9) 181 (49.9) 341 (50.5) 231 (52.5)\n Not specified/prefer not to answer 0 (0.0) 0 (0.0) 4 (0.6) 4 (0.9)\nEmployment status, n (%)b\n Currently unemployed/full-time caregiver 231 (46.7) 180 (49.3) 330 (47.3) 216 (49.5)\n Part-time/casual employed 97 (19.6) 65 (17.8) 106 (15.2) 60 (13.8)\n Currently employed full time 140 (28.3) 104 (28.5) 233 (33.4) 147 (33.7)\n Leave without pay/furloughed 27 (5.5) 16 (4.4) 28 (4.0) 13 (3.0)\nMarital status, n (%)c\n Married 184 (37.2) 146 (40.0) 300 (43.0) 224 (51.4)\n Widowed 8 (1.6) 7 (1.9) 22 (3.2) 18 (4.1)\n Separated/divorced 53 (10.7) 39 (10.7) 69 (9.9) 47 (10.8)\n Never married 160 (32.3) 103 (28.2) 255 (36.6) 126(28.9)\n Married de facto 90 (18.2) 70 (19.2) 51 (7.3) 21 (4.8)\nEthnicity, n (%)d\n Black 3 (0.6) 1 (0.3) 52 (7.5) 26 (6.0)\n Caucasian/White 392 (79.2) 304 (83.3) 566 (81.2) 376 (86.2)\n Asian (South-East Asia/South Asia) 71 (14.3) 43 (11.8) 39 (5.6) 24 (5.5)\n Middle-Eastern 6 (1.2) 3 (0.8) 1 (0.1) 0 (0.0)\n Other 13 (2.6) 6 (1.6) 27 (3.9) 8 (1.8)\n Prefer not to answer 10 (2.0) 8 (2.2) 12 (1.7) 2 (0.5)\nIncome, n (%)e\n Zero income 8 (1.7) 4 (1.2) 31 (4.4) 19 (4.4)\n $1–$199 ($1–$10,399) 9 (2.0) 6 (1.8) 40 (5.7) 24 (5.5)\n $200–$299 ($10,400–$15,599) 12 (2.6) 8 (2.4) 34 (4.9) 23 (5.3)\n $300–$399 ($15,600–$20,799) 19 (4.1) 12 (3.6) 38 (5.5) 23 (5.3)\n $400–$599 ($20,800–$31,199) 42 (9.2) 33 (9.9) 62 (8.9) 33 (7.6)\n $600–$799 ($31,200–$41,599) 57 (12.4) 42 (12.6) 61 (8.8) 39 (8.9)\n $800–$999 ($41,600–$51,999) 45 (9.8) 31 (9.3) 68 (9.8) 46 (10.6)\n $1,000–$1,249 ($52,000–$64,999) 39 (8.5) 32 (9.6) 48 (6.9) 38 (8.7)\n $1,250–$1,499 ($65,000–$77,999) 28 (6.1) 22 (6.6) 59 (8.5) 41 (9.4)\n $1,500–$1,999 ($78,000–$103,999) 72 (15.7) 50 (15.0) 72 (10.3) 48 (11.0)\n $2,000 or more ($104,000 or more) 81 (17.6) 62 (18.6) 108 (15.5) 74 (17.0)\n Prefer not to answer 47 (10.2) 32 (9.6) 76 (10.9) 28 (6.4)\nEducation level, n (%)\n Completed junior/lower/primary school 18 (3.6) 17 (4.7) 6 (0.9) 0 (0.0)\n Completed senior/high/secondary school 133 (26.9) 98 (26.8) 265 (37.8) 132 (30.0)\n Postschool vocational qualification/diploma 147 (29.7) 111 (30.4) 138 (19.7) 94 (21.4)\n Undergraduate university degree 131 (26.5) 93 (25.5) 214 (30.5) 159 (36.1)\n Postgraduate university degree 66 (13.3) 46 (12.6) 78 (11.1) 55 (12.5)\nSD standard deviation.\naTwo participants in the Australian sample did not report their gender and four participants in the U.S. sample did not report their gender.\nbFour participants in the U.S. sample did not report their employment status.\ncFour participants in the U.S. sample did not report their marital status.\ndFour participants in the U.S. sample did not report their ethnicity.\neThirty-one participants in the Australian sample did not report their income and four participants in the U.S. sample did not report their income.\n\nDesign and Procedure\nThe study adopted a prospective correlational design with self-report measures of social cognition constructs from the proposed integrated model, intention, and past social distancing behavior administered at an initial data collection occasion in a survey administered using the Qualtrics online survey tool. Social cognition measures included the theory of planned behavior (attitude, subjective norm, and PBC), moral norm, anticipated regret, action planning, and habit constructs. Participants were informed that they were participating in a survey on their social distancing behavior and provided with information outlining study requirements. They were required to provide informed consent before proceeding with the survey. Participants were also provided with instructions on how to complete study measures and a definition of the target behavior: “The following survey will ask about your beliefs and attitudes about ‘social distancing’. What do we mean by social distancing? Social distancing (also known as ‘physical distancing’) is deliberately increasing the physical space between people to avoid spreading illness. The World Health Organization and other world leading health authorities suggest that you should maintain at least a 1–2 meter (3–6 feet) distance from other people to lessen the chances of getting infected with COVID-19. When answering the questions in this survey, think about your social distancing behavior (i.e., maintaining at least a 1–2 m (3–6 ft) distance from other people).” One week later, participants were re-contacted by the panel company and asked to self-report their habit and social distancing behavior over the previous week using the same measures used at the initial data collection occasion. Participants received a fixed sum of money for their participation based on expected completion time consistent with the panel company’s published rates. Approval for study procedures was granted prior to data collection from the Griffith University Human Research Ethics Committee.\n\nMeasures\nStudy measures were multi-item self-report measures of constructs based on published guidelines and measures used in previous studies [13, 29, 30]. Participants provided their responses on scales with seven-point response options. Complete study measures are provided in Supplementary Appendix A.\n\nSocial cognition constructs\nMulti-item measures of attitude, subjective norm, PBC, moral norm, anticipated regret, and action planning were developed according to published guidelines [13, 29]. Each measure made explicit reference to the target behavior of social distancing, and participants were reminded of the definition of social distancing before completing the measures.\n\nIntention\nParticipants’ intention to participate in social distancing behavior over the next week was measured using a scale developed according to published guidelines [31].\n\nHabit\nHabit was measured at both time points using the behavioral automaticity items of Verplanken and Orbell’s self-report habit index [25]. The measure measures individuals’ reflections on the extent to which the behavior is experienced as automatic and enacted without thought.\n\nPast behavior and behavior\nParticipants self-reported their participation in social distancing behavior to minimize the transmission of the SARS-CoV-2 virus that causes COVID-19. The measure comprised two items prompting participants to report their frequency of social distancing behavior in the previous week. This is based on previously used self-report behavioral measures that have demonstrated concurrent validity with non-self-report measures in other behavioral contexts [32].\n\nDemographic variables\nParticipants self-reported their age in years, gender, employment status (currently unemployed/full-time caregiver, currently full-time employed, part-time employed, or on leave without pay/furloughed), marital status (married, widowed, separated/divorced, never married, or in a de factor relationship), annual household income stratified by 11 income levels based on Australian and U.S. national averages, highest level of formal education (completed junior/lower/primary school, completed senior/high/secondary school, postschool vocational qualification/diploma, further education diploma, undergraduate university degree, or postgraduate university degree), and ethnicity (Black, Caucasian/White, Asian, or Middle-eastern). Binary income (low income vs. middle/high income), highest education level (completed school education only vs. completed postschool education), and ethnicity (Caucasian/White vs. non-White) variables were computed for use in subsequent analyses.\n\nData Analysis\nHypothesized relations among the integrated model constructs were tested in the Australian and U.S. samples separately using variance-based structural equation modeling implemented in the WARP 7.0 analysis package [33]. Model parameters and standard errors (SEs) were computed using the “Stable3” estimation method, which has been shown to provide the most precise parameter estimates in complex structural models in smaller samples and outperforms bootstrapping methods in simulation studies [33]. Simulation studies have also shown this method to provide more consistent and precise estimates in data containing outliers, which may inflate SEs and lead to abnormally high p-values [33]. Two models were estimated in each sample: a model testing predictions of the proposed integrated model with the binary demographic variables also included as covariates (Model 1; Fig. 1, upper panel) and a model that included effects of past social distancing behavior (Model 2; Fig. 1, lower panel). All constructs were latent variables indicated by single or multiple items. There were no missing data for the social cognition and self-reported behavioral variables. There were a few instances of missing data for the demographic variables ranging from 0.5% to 8.8% in the Australia sample, and 0.9% to 6.4% in the U.S. sample. Missing data are reported in Supplementary Appendix B. Missing data were imputed using stochastic hierarchical regression [33].\nThe analysis afforded a number of analyses to evaluate the adequacy of measures used to indicate the latent variables in the model. Construct validity of the latent factors for the social cognition, intention, and behavioral variables was established using the normalized factor pattern loadings after oblique rotation and Kaiser normalization [33] and the average variance extracted (AVE), which should approach or exceed .700 and .500, respectively. Internal consistency of the factors was estimated using omega reliability coefficients (ω) and composite reliability coefficients (ρ), which should exceed .700 and ideally approach .900. We also conducted tests of the discriminant validity of the constructs in the model. Discriminant validity was supported when the square root of the AVE for each latent variable exceeded its correlation with other latent variables.\nAdequacy of the proposed model in describing the data was established using the goodness-of-fit (GoF) index with values of .100, .250, and .360 corresponding to small, medium, and large effect sizes. Further information on model quality was provided by the average path coefficient and average R2 coefficient. These indices summarize the average parameter estimates of relations in the model and the amount of variance explained in each dependent variable, respectively, and should be statistically significant for a good-quality model. In addition, an overall GoF index is provided by the average block variance inflation factor for model parameters and the average full collinearity variance inflation factor, which should be equal to or lower than 3.3 for well-fitting models. These indices indicate the extent to which latent variables in the model overlap and contribute to model multicollinearity. They, therefore, provide an indication as to the uniqueness of the existing latent variables in the model. Four further indices were also used to evaluate model quality: the Simpson’s paradox ratio (SPR), R2 contribution ratio (R2CR), the statistical suppression ratio (SSR), and the nonlinear bivariate causality direction ratio (NLBCDR). The SPR indicates whether the model is free from incidences of Simpson’s paradox (i.e., when the path coefficient and the correlation associated with a latent variable have opposite signs), indicating a causality problem. The SPR should exceed .700 and ideally approach 1.000. The R2CR and SSR provide indication of the extent to which models are free from instances of negative R2 contributions and statistical suppression. The R2CR and SSR should exceed .900 and .700, respectively. The NLBCDR provides an estimate of the extent to which the proposed “causal” associations in the proposed model are more tenable than those in the opposite direction and provide an initial indicator of support for the hypothesized directions of the causal links in the proposed model compared to if the proposed direction were reversed. The NLBCDR should exceed .700 for high-quality models. Kock [33] provides further technical details on model fit and quality indices.\nModel effects were estimated using standardized path coefficients with confidence intervals (CIs) and test statistics. Effect sizes were estimated using a variant of Cohen’s f-square coefficient and represent the individual contribution of the predictor variable to the R2 coefficients of the criterion latent variable. Values of .02, .15, and .35 represent small, medium, and large effect sizes, respectively. Differences in the path coefficients in the models across the samples were tested using multiple-group analysis using the Satterthwaite method with two-tailed significance tests.\nWe also tested whether the inclusion of participants that were never under a “shelter-in-place” order, or had the “shelter-in-place” order lifted during the study, affected predicted relations in the models. The small numbers of participants that were, at some point, not subjected to “shelter-in-place” orders meant we could not conduct a formal moderator analysis, so we conducted a sensitivity analysis testing whether effects in the models differed if data from these participants were excluded. Models excluding and including past behavior were estimated in samples excluding participants who were never subject to a “shelter-in place” order, and in the sample that were never subject to an order, or who had the order lifted at some stage during the study. Formal comparisons of parameter estimates in these models with those from the full sample were made using the Satterthwaite method. Data files, analysis scripts, and output files for all analyses are available online: https://osf.io/x9tms/."}
LitCovid-PD-GO-BP
{"project":"LitCovid-PD-GO-BP","denotations":[{"id":"T95","span":{"begin":5063,"end":5072},"obj":"http://purl.obolibrary.org/obo/GO_0050890"},{"id":"T96","span":{"begin":5158,"end":5166},"obj":"http://purl.obolibrary.org/obo/GO_0007610"},{"id":"T97","span":{"begin":5291,"end":5300},"obj":"http://purl.obolibrary.org/obo/GO_0050890"},{"id":"T98","span":{"begin":5341,"end":5349},"obj":"http://purl.obolibrary.org/obo/GO_0007610"},{"id":"T99","span":{"begin":5554,"end":5562},"obj":"http://purl.obolibrary.org/obo/GO_0007610"},{"id":"T100","span":{"begin":5820,"end":5828},"obj":"http://purl.obolibrary.org/obo/GO_0007610"},{"id":"T101","span":{"begin":6406,"end":6414},"obj":"http://purl.obolibrary.org/obo/GO_0007610"},{"id":"T102","span":{"begin":6617,"end":6625},"obj":"http://purl.obolibrary.org/obo/GO_0007610"},{"id":"T103","span":{"begin":7317,"end":7326},"obj":"http://purl.obolibrary.org/obo/GO_0050890"},{"id":"T104","span":{"begin":7555,"end":7563},"obj":"http://purl.obolibrary.org/obo/GO_0007610"},{"id":"T105","span":{"begin":7759,"end":7767},"obj":"http://purl.obolibrary.org/obo/GO_0007610"},{"id":"T106","span":{"begin":8080,"end":8088},"obj":"http://purl.obolibrary.org/obo/GO_0007610"},{"id":"T107","span":{"begin":8152,"end":8160},"obj":"http://purl.obolibrary.org/obo/GO_0007610"},{"id":"T108","span":{"begin":8165,"end":8173},"obj":"http://purl.obolibrary.org/obo/GO_0007610"},{"id":"T109","span":{"begin":8242,"end":8250},"obj":"http://purl.obolibrary.org/obo/GO_0007610"},{"id":"T110","span":{"begin":8428,"end":8436},"obj":"http://purl.obolibrary.org/obo/GO_0007610"},{"id":"T111","span":{"begin":10595,"end":10603},"obj":"http://purl.obolibrary.org/obo/GO_0007610"},{"id":"T112","span":{"begin":10754,"end":10763},"obj":"http://purl.obolibrary.org/obo/GO_0050890"},{"id":"T113","span":{"begin":11281,"end":11290},"obj":"http://purl.obolibrary.org/obo/GO_0050890"},{"id":"T114","span":{"begin":15290,"end":15298},"obj":"http://purl.obolibrary.org/obo/GO_0007610"}],"text":"Method\n\nParticipants and Recruitment\nSamples of Australian (N = 495, 50.1% female) and U.S. (N = 701, 48.9% female) residents were recruited via an online research panel company. To be eligible for inclusion, participants needed to be aged 18 years or older and not subject to formal quarantine for COVID-19. Participants were also screened for age, gender, and geographical region and quotas imposed during recruitment to ensure that the final samples closely matched the national distributions for these characteristics in each country. Data were collected between April 1 and May 6, 2020. All participants in the Australian sample were subject to a national “shelter-in-place” order issued by the federal government. However, issuance of orders in the USA was devolved to state governments resulting in some variations. The vast majority of participants in the U.S. sample (n = 610, 87.0%) were subject to “shelter-in-place” orders for the duration of the study. However, some states did not impose “shelter-in-place” orders at all (Arkansas, Iowa, Nebraska, North Dakota, South Dakota, Utah, and Wyoming), so a minority of participants in the U.S. sample (n = 37, 5.3%) were never subject to an order. Furthermore, in some cases in the U.S. sample (n = 47, 6.7%), “shelter-in-place” orders had been lifted prior to follow-up data collection. However, among the states in the USA that did not have “shelter-in-place” orders, or lifted their orders during the study, all issued social distancing guidelines and encouraged the population to follow those guidelines. Baseline sample characteristics are presented in Table 1.\nTable 1. Sample characteristics and descriptive statistics for study variables at baseline and at 1 week follow-up\nVariable Australia sample U.S. sample\nBaseline Follow-up Baseline Follow-up\nParticipants 495 365 701 440\nAge, M years (SD) 47.09 (17.11) 49.78 (16.89) 45.55 (17.40) 51.77 (16.26)\nGender, n (%)a\n Female 252 (51.1) 182 (50.1) 352 (48.9) 205 (46.6)\n Male 241 (48.9) 181 (49.9) 341 (50.5) 231 (52.5)\n Not specified/prefer not to answer 0 (0.0) 0 (0.0) 4 (0.6) 4 (0.9)\nEmployment status, n (%)b\n Currently unemployed/full-time caregiver 231 (46.7) 180 (49.3) 330 (47.3) 216 (49.5)\n Part-time/casual employed 97 (19.6) 65 (17.8) 106 (15.2) 60 (13.8)\n Currently employed full time 140 (28.3) 104 (28.5) 233 (33.4) 147 (33.7)\n Leave without pay/furloughed 27 (5.5) 16 (4.4) 28 (4.0) 13 (3.0)\nMarital status, n (%)c\n Married 184 (37.2) 146 (40.0) 300 (43.0) 224 (51.4)\n Widowed 8 (1.6) 7 (1.9) 22 (3.2) 18 (4.1)\n Separated/divorced 53 (10.7) 39 (10.7) 69 (9.9) 47 (10.8)\n Never married 160 (32.3) 103 (28.2) 255 (36.6) 126(28.9)\n Married de facto 90 (18.2) 70 (19.2) 51 (7.3) 21 (4.8)\nEthnicity, n (%)d\n Black 3 (0.6) 1 (0.3) 52 (7.5) 26 (6.0)\n Caucasian/White 392 (79.2) 304 (83.3) 566 (81.2) 376 (86.2)\n Asian (South-East Asia/South Asia) 71 (14.3) 43 (11.8) 39 (5.6) 24 (5.5)\n Middle-Eastern 6 (1.2) 3 (0.8) 1 (0.1) 0 (0.0)\n Other 13 (2.6) 6 (1.6) 27 (3.9) 8 (1.8)\n Prefer not to answer 10 (2.0) 8 (2.2) 12 (1.7) 2 (0.5)\nIncome, n (%)e\n Zero income 8 (1.7) 4 (1.2) 31 (4.4) 19 (4.4)\n $1–$199 ($1–$10,399) 9 (2.0) 6 (1.8) 40 (5.7) 24 (5.5)\n $200–$299 ($10,400–$15,599) 12 (2.6) 8 (2.4) 34 (4.9) 23 (5.3)\n $300–$399 ($15,600–$20,799) 19 (4.1) 12 (3.6) 38 (5.5) 23 (5.3)\n $400–$599 ($20,800–$31,199) 42 (9.2) 33 (9.9) 62 (8.9) 33 (7.6)\n $600–$799 ($31,200–$41,599) 57 (12.4) 42 (12.6) 61 (8.8) 39 (8.9)\n $800–$999 ($41,600–$51,999) 45 (9.8) 31 (9.3) 68 (9.8) 46 (10.6)\n $1,000–$1,249 ($52,000–$64,999) 39 (8.5) 32 (9.6) 48 (6.9) 38 (8.7)\n $1,250–$1,499 ($65,000–$77,999) 28 (6.1) 22 (6.6) 59 (8.5) 41 (9.4)\n $1,500–$1,999 ($78,000–$103,999) 72 (15.7) 50 (15.0) 72 (10.3) 48 (11.0)\n $2,000 or more ($104,000 or more) 81 (17.6) 62 (18.6) 108 (15.5) 74 (17.0)\n Prefer not to answer 47 (10.2) 32 (9.6) 76 (10.9) 28 (6.4)\nEducation level, n (%)\n Completed junior/lower/primary school 18 (3.6) 17 (4.7) 6 (0.9) 0 (0.0)\n Completed senior/high/secondary school 133 (26.9) 98 (26.8) 265 (37.8) 132 (30.0)\n Postschool vocational qualification/diploma 147 (29.7) 111 (30.4) 138 (19.7) 94 (21.4)\n Undergraduate university degree 131 (26.5) 93 (25.5) 214 (30.5) 159 (36.1)\n Postgraduate university degree 66 (13.3) 46 (12.6) 78 (11.1) 55 (12.5)\nSD standard deviation.\naTwo participants in the Australian sample did not report their gender and four participants in the U.S. sample did not report their gender.\nbFour participants in the U.S. sample did not report their employment status.\ncFour participants in the U.S. sample did not report their marital status.\ndFour participants in the U.S. sample did not report their ethnicity.\neThirty-one participants in the Australian sample did not report their income and four participants in the U.S. sample did not report their income.\n\nDesign and Procedure\nThe study adopted a prospective correlational design with self-report measures of social cognition constructs from the proposed integrated model, intention, and past social distancing behavior administered at an initial data collection occasion in a survey administered using the Qualtrics online survey tool. Social cognition measures included the theory of planned behavior (attitude, subjective norm, and PBC), moral norm, anticipated regret, action planning, and habit constructs. Participants were informed that they were participating in a survey on their social distancing behavior and provided with information outlining study requirements. They were required to provide informed consent before proceeding with the survey. Participants were also provided with instructions on how to complete study measures and a definition of the target behavior: “The following survey will ask about your beliefs and attitudes about ‘social distancing’. What do we mean by social distancing? Social distancing (also known as ‘physical distancing’) is deliberately increasing the physical space between people to avoid spreading illness. The World Health Organization and other world leading health authorities suggest that you should maintain at least a 1–2 meter (3–6 feet) distance from other people to lessen the chances of getting infected with COVID-19. When answering the questions in this survey, think about your social distancing behavior (i.e., maintaining at least a 1–2 m (3–6 ft) distance from other people).” One week later, participants were re-contacted by the panel company and asked to self-report their habit and social distancing behavior over the previous week using the same measures used at the initial data collection occasion. Participants received a fixed sum of money for their participation based on expected completion time consistent with the panel company’s published rates. Approval for study procedures was granted prior to data collection from the Griffith University Human Research Ethics Committee.\n\nMeasures\nStudy measures were multi-item self-report measures of constructs based on published guidelines and measures used in previous studies [13, 29, 30]. Participants provided their responses on scales with seven-point response options. Complete study measures are provided in Supplementary Appendix A.\n\nSocial cognition constructs\nMulti-item measures of attitude, subjective norm, PBC, moral norm, anticipated regret, and action planning were developed according to published guidelines [13, 29]. Each measure made explicit reference to the target behavior of social distancing, and participants were reminded of the definition of social distancing before completing the measures.\n\nIntention\nParticipants’ intention to participate in social distancing behavior over the next week was measured using a scale developed according to published guidelines [31].\n\nHabit\nHabit was measured at both time points using the behavioral automaticity items of Verplanken and Orbell’s self-report habit index [25]. The measure measures individuals’ reflections on the extent to which the behavior is experienced as automatic and enacted without thought.\n\nPast behavior and behavior\nParticipants self-reported their participation in social distancing behavior to minimize the transmission of the SARS-CoV-2 virus that causes COVID-19. The measure comprised two items prompting participants to report their frequency of social distancing behavior in the previous week. This is based on previously used self-report behavioral measures that have demonstrated concurrent validity with non-self-report measures in other behavioral contexts [32].\n\nDemographic variables\nParticipants self-reported their age in years, gender, employment status (currently unemployed/full-time caregiver, currently full-time employed, part-time employed, or on leave without pay/furloughed), marital status (married, widowed, separated/divorced, never married, or in a de factor relationship), annual household income stratified by 11 income levels based on Australian and U.S. national averages, highest level of formal education (completed junior/lower/primary school, completed senior/high/secondary school, postschool vocational qualification/diploma, further education diploma, undergraduate university degree, or postgraduate university degree), and ethnicity (Black, Caucasian/White, Asian, or Middle-eastern). Binary income (low income vs. middle/high income), highest education level (completed school education only vs. completed postschool education), and ethnicity (Caucasian/White vs. non-White) variables were computed for use in subsequent analyses.\n\nData Analysis\nHypothesized relations among the integrated model constructs were tested in the Australian and U.S. samples separately using variance-based structural equation modeling implemented in the WARP 7.0 analysis package [33]. Model parameters and standard errors (SEs) were computed using the “Stable3” estimation method, which has been shown to provide the most precise parameter estimates in complex structural models in smaller samples and outperforms bootstrapping methods in simulation studies [33]. Simulation studies have also shown this method to provide more consistent and precise estimates in data containing outliers, which may inflate SEs and lead to abnormally high p-values [33]. Two models were estimated in each sample: a model testing predictions of the proposed integrated model with the binary demographic variables also included as covariates (Model 1; Fig. 1, upper panel) and a model that included effects of past social distancing behavior (Model 2; Fig. 1, lower panel). All constructs were latent variables indicated by single or multiple items. There were no missing data for the social cognition and self-reported behavioral variables. There were a few instances of missing data for the demographic variables ranging from 0.5% to 8.8% in the Australia sample, and 0.9% to 6.4% in the U.S. sample. Missing data are reported in Supplementary Appendix B. Missing data were imputed using stochastic hierarchical regression [33].\nThe analysis afforded a number of analyses to evaluate the adequacy of measures used to indicate the latent variables in the model. Construct validity of the latent factors for the social cognition, intention, and behavioral variables was established using the normalized factor pattern loadings after oblique rotation and Kaiser normalization [33] and the average variance extracted (AVE), which should approach or exceed .700 and .500, respectively. Internal consistency of the factors was estimated using omega reliability coefficients (ω) and composite reliability coefficients (ρ), which should exceed .700 and ideally approach .900. We also conducted tests of the discriminant validity of the constructs in the model. Discriminant validity was supported when the square root of the AVE for each latent variable exceeded its correlation with other latent variables.\nAdequacy of the proposed model in describing the data was established using the goodness-of-fit (GoF) index with values of .100, .250, and .360 corresponding to small, medium, and large effect sizes. Further information on model quality was provided by the average path coefficient and average R2 coefficient. These indices summarize the average parameter estimates of relations in the model and the amount of variance explained in each dependent variable, respectively, and should be statistically significant for a good-quality model. In addition, an overall GoF index is provided by the average block variance inflation factor for model parameters and the average full collinearity variance inflation factor, which should be equal to or lower than 3.3 for well-fitting models. These indices indicate the extent to which latent variables in the model overlap and contribute to model multicollinearity. They, therefore, provide an indication as to the uniqueness of the existing latent variables in the model. Four further indices were also used to evaluate model quality: the Simpson’s paradox ratio (SPR), R2 contribution ratio (R2CR), the statistical suppression ratio (SSR), and the nonlinear bivariate causality direction ratio (NLBCDR). The SPR indicates whether the model is free from incidences of Simpson’s paradox (i.e., when the path coefficient and the correlation associated with a latent variable have opposite signs), indicating a causality problem. The SPR should exceed .700 and ideally approach 1.000. The R2CR and SSR provide indication of the extent to which models are free from instances of negative R2 contributions and statistical suppression. The R2CR and SSR should exceed .900 and .700, respectively. The NLBCDR provides an estimate of the extent to which the proposed “causal” associations in the proposed model are more tenable than those in the opposite direction and provide an initial indicator of support for the hypothesized directions of the causal links in the proposed model compared to if the proposed direction were reversed. The NLBCDR should exceed .700 for high-quality models. Kock [33] provides further technical details on model fit and quality indices.\nModel effects were estimated using standardized path coefficients with confidence intervals (CIs) and test statistics. Effect sizes were estimated using a variant of Cohen’s f-square coefficient and represent the individual contribution of the predictor variable to the R2 coefficients of the criterion latent variable. Values of .02, .15, and .35 represent small, medium, and large effect sizes, respectively. Differences in the path coefficients in the models across the samples were tested using multiple-group analysis using the Satterthwaite method with two-tailed significance tests.\nWe also tested whether the inclusion of participants that were never under a “shelter-in-place” order, or had the “shelter-in-place” order lifted during the study, affected predicted relations in the models. The small numbers of participants that were, at some point, not subjected to “shelter-in-place” orders meant we could not conduct a formal moderator analysis, so we conducted a sensitivity analysis testing whether effects in the models differed if data from these participants were excluded. Models excluding and including past behavior were estimated in samples excluding participants who were never subject to a “shelter-in place” order, and in the sample that were never subject to an order, or who had the order lifted at some stage during the study. Formal comparisons of parameter estimates in these models with those from the full sample were made using the Satterthwaite method. Data files, analysis scripts, and output files for all analyses are available online: https://osf.io/x9tms/."}
LitCovid-sentences
{"project":"LitCovid-sentences","denotations":[{"id":"T90","span":{"begin":0,"end":6},"obj":"Sentence"},{"id":"T91","span":{"begin":8,"end":36},"obj":"Sentence"},{"id":"T92","span":{"begin":37,"end":178},"obj":"Sentence"},{"id":"T93","span":{"begin":179,"end":308},"obj":"Sentence"},{"id":"T94","span":{"begin":309,"end":538},"obj":"Sentence"},{"id":"T95","span":{"begin":539,"end":591},"obj":"Sentence"},{"id":"T96","span":{"begin":592,"end":719},"obj":"Sentence"},{"id":"T97","span":{"begin":720,"end":822},"obj":"Sentence"},{"id":"T98","span":{"begin":823,"end":965},"obj":"Sentence"},{"id":"T99","span":{"begin":966,"end":1205},"obj":"Sentence"},{"id":"T100","span":{"begin":1206,"end":1345},"obj":"Sentence"},{"id":"T101","span":{"begin":1346,"end":1566},"obj":"Sentence"},{"id":"T102","span":{"begin":1567,"end":1624},"obj":"Sentence"},{"id":"T103","span":{"begin":1625,"end":1633},"obj":"Sentence"},{"id":"T104","span":{"begin":1635,"end":1740},"obj":"Sentence"},{"id":"T105","span":{"begin":1741,"end":1780},"obj":"Sentence"},{"id":"T106","span":{"begin":1781,"end":1821},"obj":"Sentence"},{"id":"T107","span":{"begin":1822,"end":1854},"obj":"Sentence"},{"id":"T108","span":{"begin":1855,"end":1932},"obj":"Sentence"},{"id":"T109","span":{"begin":1933,"end":1947},"obj":"Sentence"},{"id":"T110","span":{"begin":1948,"end":2003},"obj":"Sentence"},{"id":"T111","span":{"begin":2004,"end":2057},"obj":"Sentence"},{"id":"T112","span":{"begin":2058,"end":2129},"obj":"Sentence"},{"id":"T113","span":{"begin":2130,"end":2155},"obj":"Sentence"},{"id":"T114","span":{"begin":2156,"end":2245},"obj":"Sentence"},{"id":"T115","span":{"begin":2246,"end":2317},"obj":"Sentence"},{"id":"T116","span":{"begin":2318,"end":2395},"obj":"Sentence"},{"id":"T117","span":{"begin":2396,"end":2465},"obj":"Sentence"},{"id":"T118","span":{"begin":2466,"end":2488},"obj":"Sentence"},{"id":"T119","span":{"begin":2489,"end":2545},"obj":"Sentence"},{"id":"T120","span":{"begin":2546,"end":2592},"obj":"Sentence"},{"id":"T121","span":{"begin":2593,"end":2655},"obj":"Sentence"},{"id":"T122","span":{"begin":2656,"end":2717},"obj":"Sentence"},{"id":"T123","span":{"begin":2718,"end":2777},"obj":"Sentence"},{"id":"T124","span":{"begin":2778,"end":2795},"obj":"Sentence"},{"id":"T125","span":{"begin":2796,"end":2840},"obj":"Sentence"},{"id":"T126","span":{"begin":2841,"end":2905},"obj":"Sentence"},{"id":"T127","span":{"begin":2906,"end":2983},"obj":"Sentence"},{"id":"T128","span":{"begin":2984,"end":3035},"obj":"Sentence"},{"id":"T129","span":{"begin":3036,"end":3080},"obj":"Sentence"},{"id":"T130","span":{"begin":3081,"end":3140},"obj":"Sentence"},{"id":"T131","span":{"begin":3141,"end":3155},"obj":"Sentence"},{"id":"T132","span":{"begin":3156,"end":3206},"obj":"Sentence"},{"id":"T133","span":{"begin":3207,"end":3266},"obj":"Sentence"},{"id":"T134","span":{"begin":3267,"end":3334},"obj":"Sentence"},{"id":"T135","span":{"begin":3335,"end":3403},"obj":"Sentence"},{"id":"T136","span":{"begin":3404,"end":3472},"obj":"Sentence"},{"id":"T137","span":{"begin":3473,"end":3543},"obj":"Sentence"},{"id":"T138","span":{"begin":3544,"end":3613},"obj":"Sentence"},{"id":"T139","span":{"begin":3614,"end":3686},"obj":"Sentence"},{"id":"T140","span":{"begin":3687,"end":3759},"obj":"Sentence"},{"id":"T141","span":{"begin":3760,"end":3837},"obj":"Sentence"},{"id":"T142","span":{"begin":3838,"end":3917},"obj":"Sentence"},{"id":"T143","span":{"begin":3918,"end":3981},"obj":"Sentence"},{"id":"T144","span":{"begin":3982,"end":4004},"obj":"Sentence"},{"id":"T145","span":{"begin":4005,"end":4081},"obj":"Sentence"},{"id":"T146","span":{"begin":4082,"end":4168},"obj":"Sentence"},{"id":"T147","span":{"begin":4169,"end":4260},"obj":"Sentence"},{"id":"T148","span":{"begin":4261,"end":4340},"obj":"Sentence"},{"id":"T149","span":{"begin":4341,"end":4416},"obj":"Sentence"},{"id":"T150","span":{"begin":4417,"end":4439},"obj":"Sentence"},{"id":"T151","span":{"begin":4440,"end":4580},"obj":"Sentence"},{"id":"T152","span":{"begin":4581,"end":4658},"obj":"Sentence"},{"id":"T153","span":{"begin":4659,"end":4733},"obj":"Sentence"},{"id":"T154","span":{"begin":4734,"end":4803},"obj":"Sentence"},{"id":"T155","span":{"begin":4804,"end":4951},"obj":"Sentence"},{"id":"T156","span":{"begin":4953,"end":4973},"obj":"Sentence"},{"id":"T157","span":{"begin":4974,"end":5283},"obj":"Sentence"},{"id":"T158","span":{"begin":5284,"end":5458},"obj":"Sentence"},{"id":"T159","span":{"begin":5459,"end":5622},"obj":"Sentence"},{"id":"T160","span":{"begin":5623,"end":5704},"obj":"Sentence"},{"id":"T161","span":{"begin":5705,"end":5920},"obj":"Sentence"},{"id":"T162","span":{"begin":5921,"end":5958},"obj":"Sentence"},{"id":"T163","span":{"begin":5959,"end":6103},"obj":"Sentence"},{"id":"T164","span":{"begin":6104,"end":6325},"obj":"Sentence"},{"id":"T165","span":{"begin":6326,"end":6718},"obj":"Sentence"},{"id":"T166","span":{"begin":6719,"end":6872},"obj":"Sentence"},{"id":"T167","span":{"begin":6873,"end":7001},"obj":"Sentence"},{"id":"T168","span":{"begin":7003,"end":7011},"obj":"Sentence"},{"id":"T169","span":{"begin":7012,"end":7159},"obj":"Sentence"},{"id":"T170","span":{"begin":7160,"end":7242},"obj":"Sentence"},{"id":"T171","span":{"begin":7243,"end":7308},"obj":"Sentence"},{"id":"T172","span":{"begin":7310,"end":7337},"obj":"Sentence"},{"id":"T173","span":{"begin":7338,"end":7503},"obj":"Sentence"},{"id":"T174","span":{"begin":7504,"end":7687},"obj":"Sentence"},{"id":"T175","span":{"begin":7689,"end":7698},"obj":"Sentence"},{"id":"T176","span":{"begin":7699,"end":7863},"obj":"Sentence"},{"id":"T177","span":{"begin":7865,"end":7870},"obj":"Sentence"},{"id":"T178","span":{"begin":7871,"end":8006},"obj":"Sentence"},{"id":"T179","span":{"begin":8007,"end":8145},"obj":"Sentence"},{"id":"T180","span":{"begin":8147,"end":8173},"obj":"Sentence"},{"id":"T181","span":{"begin":8174,"end":8325},"obj":"Sentence"},{"id":"T182","span":{"begin":8326,"end":8458},"obj":"Sentence"},{"id":"T183","span":{"begin":8459,"end":8631},"obj":"Sentence"},{"id":"T184","span":{"begin":8633,"end":8654},"obj":"Sentence"},{"id":"T185","span":{"begin":8655,"end":9383},"obj":"Sentence"},{"id":"T186","span":{"begin":9384,"end":9630},"obj":"Sentence"},{"id":"T187","span":{"begin":9632,"end":9645},"obj":"Sentence"},{"id":"T188","span":{"begin":9646,"end":9865},"obj":"Sentence"},{"id":"T189","span":{"begin":9866,"end":10144},"obj":"Sentence"},{"id":"T190","span":{"begin":10145,"end":10334},"obj":"Sentence"},{"id":"T191","span":{"begin":10335,"end":10635},"obj":"Sentence"},{"id":"T192","span":{"begin":10636,"end":10711},"obj":"Sentence"},{"id":"T193","span":{"begin":10712,"end":10803},"obj":"Sentence"},{"id":"T194","span":{"begin":10804,"end":10964},"obj":"Sentence"},{"id":"T195","span":{"begin":10965,"end":11019},"obj":"Sentence"},{"id":"T196","span":{"begin":11020,"end":11092},"obj":"Sentence"},{"id":"T197","span":{"begin":11093,"end":11224},"obj":"Sentence"},{"id":"T198","span":{"begin":11225,"end":11544},"obj":"Sentence"},{"id":"T199","span":{"begin":11545,"end":11731},"obj":"Sentence"},{"id":"T200","span":{"begin":11732,"end":11816},"obj":"Sentence"},{"id":"T201","span":{"begin":11817,"end":11963},"obj":"Sentence"},{"id":"T202","span":{"begin":11964,"end":12163},"obj":"Sentence"},{"id":"T203","span":{"begin":12164,"end":12273},"obj":"Sentence"},{"id":"T204","span":{"begin":12274,"end":12500},"obj":"Sentence"},{"id":"T205","span":{"begin":12501,"end":12743},"obj":"Sentence"},{"id":"T206","span":{"begin":12744,"end":12867},"obj":"Sentence"},{"id":"T207","span":{"begin":12868,"end":12974},"obj":"Sentence"},{"id":"T208","span":{"begin":12975,"end":13207},"obj":"Sentence"},{"id":"T209","span":{"begin":13208,"end":13429},"obj":"Sentence"},{"id":"T210","span":{"begin":13430,"end":13484},"obj":"Sentence"},{"id":"T211","span":{"begin":13485,"end":13632},"obj":"Sentence"},{"id":"T212","span":{"begin":13633,"end":13692},"obj":"Sentence"},{"id":"T213","span":{"begin":13693,"end":14029},"obj":"Sentence"},{"id":"T214","span":{"begin":14030,"end":14084},"obj":"Sentence"},{"id":"T215","span":{"begin":14085,"end":14163},"obj":"Sentence"},{"id":"T216","span":{"begin":14164,"end":14282},"obj":"Sentence"},{"id":"T217","span":{"begin":14283,"end":14483},"obj":"Sentence"},{"id":"T218","span":{"begin":14484,"end":14574},"obj":"Sentence"},{"id":"T219","span":{"begin":14575,"end":14753},"obj":"Sentence"},{"id":"T220","span":{"begin":14754,"end":14961},"obj":"Sentence"},{"id":"T221","span":{"begin":14962,"end":15253},"obj":"Sentence"},{"id":"T222","span":{"begin":15254,"end":15516},"obj":"Sentence"},{"id":"T223","span":{"begin":15517,"end":15648},"obj":"Sentence"},{"id":"T224","span":{"begin":15649,"end":15757},"obj":"Sentence"}],"namespaces":[{"prefix":"_base","uri":"http://pubannotation.org/ontology/tao.owl#"}],"text":"Method\n\nParticipants and Recruitment\nSamples of Australian (N = 495, 50.1% female) and U.S. (N = 701, 48.9% female) residents were recruited via an online research panel company. To be eligible for inclusion, participants needed to be aged 18 years or older and not subject to formal quarantine for COVID-19. Participants were also screened for age, gender, and geographical region and quotas imposed during recruitment to ensure that the final samples closely matched the national distributions for these characteristics in each country. Data were collected between April 1 and May 6, 2020. All participants in the Australian sample were subject to a national “shelter-in-place” order issued by the federal government. However, issuance of orders in the USA was devolved to state governments resulting in some variations. The vast majority of participants in the U.S. sample (n = 610, 87.0%) were subject to “shelter-in-place” orders for the duration of the study. However, some states did not impose “shelter-in-place” orders at all (Arkansas, Iowa, Nebraska, North Dakota, South Dakota, Utah, and Wyoming), so a minority of participants in the U.S. sample (n = 37, 5.3%) were never subject to an order. Furthermore, in some cases in the U.S. sample (n = 47, 6.7%), “shelter-in-place” orders had been lifted prior to follow-up data collection. However, among the states in the USA that did not have “shelter-in-place” orders, or lifted their orders during the study, all issued social distancing guidelines and encouraged the population to follow those guidelines. Baseline sample characteristics are presented in Table 1.\nTable 1. Sample characteristics and descriptive statistics for study variables at baseline and at 1 week follow-up\nVariable Australia sample U.S. sample\nBaseline Follow-up Baseline Follow-up\nParticipants 495 365 701 440\nAge, M years (SD) 47.09 (17.11) 49.78 (16.89) 45.55 (17.40) 51.77 (16.26)\nGender, n (%)a\n Female 252 (51.1) 182 (50.1) 352 (48.9) 205 (46.6)\n Male 241 (48.9) 181 (49.9) 341 (50.5) 231 (52.5)\n Not specified/prefer not to answer 0 (0.0) 0 (0.0) 4 (0.6) 4 (0.9)\nEmployment status, n (%)b\n Currently unemployed/full-time caregiver 231 (46.7) 180 (49.3) 330 (47.3) 216 (49.5)\n Part-time/casual employed 97 (19.6) 65 (17.8) 106 (15.2) 60 (13.8)\n Currently employed full time 140 (28.3) 104 (28.5) 233 (33.4) 147 (33.7)\n Leave without pay/furloughed 27 (5.5) 16 (4.4) 28 (4.0) 13 (3.0)\nMarital status, n (%)c\n Married 184 (37.2) 146 (40.0) 300 (43.0) 224 (51.4)\n Widowed 8 (1.6) 7 (1.9) 22 (3.2) 18 (4.1)\n Separated/divorced 53 (10.7) 39 (10.7) 69 (9.9) 47 (10.8)\n Never married 160 (32.3) 103 (28.2) 255 (36.6) 126(28.9)\n Married de facto 90 (18.2) 70 (19.2) 51 (7.3) 21 (4.8)\nEthnicity, n (%)d\n Black 3 (0.6) 1 (0.3) 52 (7.5) 26 (6.0)\n Caucasian/White 392 (79.2) 304 (83.3) 566 (81.2) 376 (86.2)\n Asian (South-East Asia/South Asia) 71 (14.3) 43 (11.8) 39 (5.6) 24 (5.5)\n Middle-Eastern 6 (1.2) 3 (0.8) 1 (0.1) 0 (0.0)\n Other 13 (2.6) 6 (1.6) 27 (3.9) 8 (1.8)\n Prefer not to answer 10 (2.0) 8 (2.2) 12 (1.7) 2 (0.5)\nIncome, n (%)e\n Zero income 8 (1.7) 4 (1.2) 31 (4.4) 19 (4.4)\n $1–$199 ($1–$10,399) 9 (2.0) 6 (1.8) 40 (5.7) 24 (5.5)\n $200–$299 ($10,400–$15,599) 12 (2.6) 8 (2.4) 34 (4.9) 23 (5.3)\n $300–$399 ($15,600–$20,799) 19 (4.1) 12 (3.6) 38 (5.5) 23 (5.3)\n $400–$599 ($20,800–$31,199) 42 (9.2) 33 (9.9) 62 (8.9) 33 (7.6)\n $600–$799 ($31,200–$41,599) 57 (12.4) 42 (12.6) 61 (8.8) 39 (8.9)\n $800–$999 ($41,600–$51,999) 45 (9.8) 31 (9.3) 68 (9.8) 46 (10.6)\n $1,000–$1,249 ($52,000–$64,999) 39 (8.5) 32 (9.6) 48 (6.9) 38 (8.7)\n $1,250–$1,499 ($65,000–$77,999) 28 (6.1) 22 (6.6) 59 (8.5) 41 (9.4)\n $1,500–$1,999 ($78,000–$103,999) 72 (15.7) 50 (15.0) 72 (10.3) 48 (11.0)\n $2,000 or more ($104,000 or more) 81 (17.6) 62 (18.6) 108 (15.5) 74 (17.0)\n Prefer not to answer 47 (10.2) 32 (9.6) 76 (10.9) 28 (6.4)\nEducation level, n (%)\n Completed junior/lower/primary school 18 (3.6) 17 (4.7) 6 (0.9) 0 (0.0)\n Completed senior/high/secondary school 133 (26.9) 98 (26.8) 265 (37.8) 132 (30.0)\n Postschool vocational qualification/diploma 147 (29.7) 111 (30.4) 138 (19.7) 94 (21.4)\n Undergraduate university degree 131 (26.5) 93 (25.5) 214 (30.5) 159 (36.1)\n Postgraduate university degree 66 (13.3) 46 (12.6) 78 (11.1) 55 (12.5)\nSD standard deviation.\naTwo participants in the Australian sample did not report their gender and four participants in the U.S. sample did not report their gender.\nbFour participants in the U.S. sample did not report their employment status.\ncFour participants in the U.S. sample did not report their marital status.\ndFour participants in the U.S. sample did not report their ethnicity.\neThirty-one participants in the Australian sample did not report their income and four participants in the U.S. sample did not report their income.\n\nDesign and Procedure\nThe study adopted a prospective correlational design with self-report measures of social cognition constructs from the proposed integrated model, intention, and past social distancing behavior administered at an initial data collection occasion in a survey administered using the Qualtrics online survey tool. Social cognition measures included the theory of planned behavior (attitude, subjective norm, and PBC), moral norm, anticipated regret, action planning, and habit constructs. Participants were informed that they were participating in a survey on their social distancing behavior and provided with information outlining study requirements. They were required to provide informed consent before proceeding with the survey. Participants were also provided with instructions on how to complete study measures and a definition of the target behavior: “The following survey will ask about your beliefs and attitudes about ‘social distancing’. What do we mean by social distancing? Social distancing (also known as ‘physical distancing’) is deliberately increasing the physical space between people to avoid spreading illness. The World Health Organization and other world leading health authorities suggest that you should maintain at least a 1–2 meter (3–6 feet) distance from other people to lessen the chances of getting infected with COVID-19. When answering the questions in this survey, think about your social distancing behavior (i.e., maintaining at least a 1–2 m (3–6 ft) distance from other people).” One week later, participants were re-contacted by the panel company and asked to self-report their habit and social distancing behavior over the previous week using the same measures used at the initial data collection occasion. Participants received a fixed sum of money for their participation based on expected completion time consistent with the panel company’s published rates. Approval for study procedures was granted prior to data collection from the Griffith University Human Research Ethics Committee.\n\nMeasures\nStudy measures were multi-item self-report measures of constructs based on published guidelines and measures used in previous studies [13, 29, 30]. Participants provided their responses on scales with seven-point response options. Complete study measures are provided in Supplementary Appendix A.\n\nSocial cognition constructs\nMulti-item measures of attitude, subjective norm, PBC, moral norm, anticipated regret, and action planning were developed according to published guidelines [13, 29]. Each measure made explicit reference to the target behavior of social distancing, and participants were reminded of the definition of social distancing before completing the measures.\n\nIntention\nParticipants’ intention to participate in social distancing behavior over the next week was measured using a scale developed according to published guidelines [31].\n\nHabit\nHabit was measured at both time points using the behavioral automaticity items of Verplanken and Orbell’s self-report habit index [25]. The measure measures individuals’ reflections on the extent to which the behavior is experienced as automatic and enacted without thought.\n\nPast behavior and behavior\nParticipants self-reported their participation in social distancing behavior to minimize the transmission of the SARS-CoV-2 virus that causes COVID-19. The measure comprised two items prompting participants to report their frequency of social distancing behavior in the previous week. This is based on previously used self-report behavioral measures that have demonstrated concurrent validity with non-self-report measures in other behavioral contexts [32].\n\nDemographic variables\nParticipants self-reported their age in years, gender, employment status (currently unemployed/full-time caregiver, currently full-time employed, part-time employed, or on leave without pay/furloughed), marital status (married, widowed, separated/divorced, never married, or in a de factor relationship), annual household income stratified by 11 income levels based on Australian and U.S. national averages, highest level of formal education (completed junior/lower/primary school, completed senior/high/secondary school, postschool vocational qualification/diploma, further education diploma, undergraduate university degree, or postgraduate university degree), and ethnicity (Black, Caucasian/White, Asian, or Middle-eastern). Binary income (low income vs. middle/high income), highest education level (completed school education only vs. completed postschool education), and ethnicity (Caucasian/White vs. non-White) variables were computed for use in subsequent analyses.\n\nData Analysis\nHypothesized relations among the integrated model constructs were tested in the Australian and U.S. samples separately using variance-based structural equation modeling implemented in the WARP 7.0 analysis package [33]. Model parameters and standard errors (SEs) were computed using the “Stable3” estimation method, which has been shown to provide the most precise parameter estimates in complex structural models in smaller samples and outperforms bootstrapping methods in simulation studies [33]. Simulation studies have also shown this method to provide more consistent and precise estimates in data containing outliers, which may inflate SEs and lead to abnormally high p-values [33]. Two models were estimated in each sample: a model testing predictions of the proposed integrated model with the binary demographic variables also included as covariates (Model 1; Fig. 1, upper panel) and a model that included effects of past social distancing behavior (Model 2; Fig. 1, lower panel). All constructs were latent variables indicated by single or multiple items. There were no missing data for the social cognition and self-reported behavioral variables. There were a few instances of missing data for the demographic variables ranging from 0.5% to 8.8% in the Australia sample, and 0.9% to 6.4% in the U.S. sample. Missing data are reported in Supplementary Appendix B. Missing data were imputed using stochastic hierarchical regression [33].\nThe analysis afforded a number of analyses to evaluate the adequacy of measures used to indicate the latent variables in the model. Construct validity of the latent factors for the social cognition, intention, and behavioral variables was established using the normalized factor pattern loadings after oblique rotation and Kaiser normalization [33] and the average variance extracted (AVE), which should approach or exceed .700 and .500, respectively. Internal consistency of the factors was estimated using omega reliability coefficients (ω) and composite reliability coefficients (ρ), which should exceed .700 and ideally approach .900. We also conducted tests of the discriminant validity of the constructs in the model. Discriminant validity was supported when the square root of the AVE for each latent variable exceeded its correlation with other latent variables.\nAdequacy of the proposed model in describing the data was established using the goodness-of-fit (GoF) index with values of .100, .250, and .360 corresponding to small, medium, and large effect sizes. Further information on model quality was provided by the average path coefficient and average R2 coefficient. These indices summarize the average parameter estimates of relations in the model and the amount of variance explained in each dependent variable, respectively, and should be statistically significant for a good-quality model. In addition, an overall GoF index is provided by the average block variance inflation factor for model parameters and the average full collinearity variance inflation factor, which should be equal to or lower than 3.3 for well-fitting models. These indices indicate the extent to which latent variables in the model overlap and contribute to model multicollinearity. They, therefore, provide an indication as to the uniqueness of the existing latent variables in the model. Four further indices were also used to evaluate model quality: the Simpson’s paradox ratio (SPR), R2 contribution ratio (R2CR), the statistical suppression ratio (SSR), and the nonlinear bivariate causality direction ratio (NLBCDR). The SPR indicates whether the model is free from incidences of Simpson’s paradox (i.e., when the path coefficient and the correlation associated with a latent variable have opposite signs), indicating a causality problem. The SPR should exceed .700 and ideally approach 1.000. The R2CR and SSR provide indication of the extent to which models are free from instances of negative R2 contributions and statistical suppression. The R2CR and SSR should exceed .900 and .700, respectively. The NLBCDR provides an estimate of the extent to which the proposed “causal” associations in the proposed model are more tenable than those in the opposite direction and provide an initial indicator of support for the hypothesized directions of the causal links in the proposed model compared to if the proposed direction were reversed. The NLBCDR should exceed .700 for high-quality models. Kock [33] provides further technical details on model fit and quality indices.\nModel effects were estimated using standardized path coefficients with confidence intervals (CIs) and test statistics. Effect sizes were estimated using a variant of Cohen’s f-square coefficient and represent the individual contribution of the predictor variable to the R2 coefficients of the criterion latent variable. Values of .02, .15, and .35 represent small, medium, and large effect sizes, respectively. Differences in the path coefficients in the models across the samples were tested using multiple-group analysis using the Satterthwaite method with two-tailed significance tests.\nWe also tested whether the inclusion of participants that were never under a “shelter-in-place” order, or had the “shelter-in-place” order lifted during the study, affected predicted relations in the models. The small numbers of participants that were, at some point, not subjected to “shelter-in-place” orders meant we could not conduct a formal moderator analysis, so we conducted a sensitivity analysis testing whether effects in the models differed if data from these participants were excluded. Models excluding and including past behavior were estimated in samples excluding participants who were never subject to a “shelter-in place” order, and in the sample that were never subject to an order, or who had the order lifted at some stage during the study. Formal comparisons of parameter estimates in these models with those from the full sample were made using the Satterthwaite method. Data files, analysis scripts, and output files for all analyses are available online: https://osf.io/x9tms/."}
2_test
{"project":"2_test","denotations":[{"id":"32914831-14715114-2620056","span":{"begin":7151,"end":7153},"obj":"14715114"},{"id":"32914831-22935297-2620057","span":{"begin":7155,"end":7157},"obj":"22935297"},{"id":"32914831-14715114-2620058","span":{"begin":7499,"end":7501},"obj":"14715114"}],"text":"Method\n\nParticipants and Recruitment\nSamples of Australian (N = 495, 50.1% female) and U.S. (N = 701, 48.9% female) residents were recruited via an online research panel company. To be eligible for inclusion, participants needed to be aged 18 years or older and not subject to formal quarantine for COVID-19. Participants were also screened for age, gender, and geographical region and quotas imposed during recruitment to ensure that the final samples closely matched the national distributions for these characteristics in each country. Data were collected between April 1 and May 6, 2020. All participants in the Australian sample were subject to a national “shelter-in-place” order issued by the federal government. However, issuance of orders in the USA was devolved to state governments resulting in some variations. The vast majority of participants in the U.S. sample (n = 610, 87.0%) were subject to “shelter-in-place” orders for the duration of the study. However, some states did not impose “shelter-in-place” orders at all (Arkansas, Iowa, Nebraska, North Dakota, South Dakota, Utah, and Wyoming), so a minority of participants in the U.S. sample (n = 37, 5.3%) were never subject to an order. Furthermore, in some cases in the U.S. sample (n = 47, 6.7%), “shelter-in-place” orders had been lifted prior to follow-up data collection. However, among the states in the USA that did not have “shelter-in-place” orders, or lifted their orders during the study, all issued social distancing guidelines and encouraged the population to follow those guidelines. Baseline sample characteristics are presented in Table 1.\nTable 1. Sample characteristics and descriptive statistics for study variables at baseline and at 1 week follow-up\nVariable Australia sample U.S. sample\nBaseline Follow-up Baseline Follow-up\nParticipants 495 365 701 440\nAge, M years (SD) 47.09 (17.11) 49.78 (16.89) 45.55 (17.40) 51.77 (16.26)\nGender, n (%)a\n Female 252 (51.1) 182 (50.1) 352 (48.9) 205 (46.6)\n Male 241 (48.9) 181 (49.9) 341 (50.5) 231 (52.5)\n Not specified/prefer not to answer 0 (0.0) 0 (0.0) 4 (0.6) 4 (0.9)\nEmployment status, n (%)b\n Currently unemployed/full-time caregiver 231 (46.7) 180 (49.3) 330 (47.3) 216 (49.5)\n Part-time/casual employed 97 (19.6) 65 (17.8) 106 (15.2) 60 (13.8)\n Currently employed full time 140 (28.3) 104 (28.5) 233 (33.4) 147 (33.7)\n Leave without pay/furloughed 27 (5.5) 16 (4.4) 28 (4.0) 13 (3.0)\nMarital status, n (%)c\n Married 184 (37.2) 146 (40.0) 300 (43.0) 224 (51.4)\n Widowed 8 (1.6) 7 (1.9) 22 (3.2) 18 (4.1)\n Separated/divorced 53 (10.7) 39 (10.7) 69 (9.9) 47 (10.8)\n Never married 160 (32.3) 103 (28.2) 255 (36.6) 126(28.9)\n Married de facto 90 (18.2) 70 (19.2) 51 (7.3) 21 (4.8)\nEthnicity, n (%)d\n Black 3 (0.6) 1 (0.3) 52 (7.5) 26 (6.0)\n Caucasian/White 392 (79.2) 304 (83.3) 566 (81.2) 376 (86.2)\n Asian (South-East Asia/South Asia) 71 (14.3) 43 (11.8) 39 (5.6) 24 (5.5)\n Middle-Eastern 6 (1.2) 3 (0.8) 1 (0.1) 0 (0.0)\n Other 13 (2.6) 6 (1.6) 27 (3.9) 8 (1.8)\n Prefer not to answer 10 (2.0) 8 (2.2) 12 (1.7) 2 (0.5)\nIncome, n (%)e\n Zero income 8 (1.7) 4 (1.2) 31 (4.4) 19 (4.4)\n $1–$199 ($1–$10,399) 9 (2.0) 6 (1.8) 40 (5.7) 24 (5.5)\n $200–$299 ($10,400–$15,599) 12 (2.6) 8 (2.4) 34 (4.9) 23 (5.3)\n $300–$399 ($15,600–$20,799) 19 (4.1) 12 (3.6) 38 (5.5) 23 (5.3)\n $400–$599 ($20,800–$31,199) 42 (9.2) 33 (9.9) 62 (8.9) 33 (7.6)\n $600–$799 ($31,200–$41,599) 57 (12.4) 42 (12.6) 61 (8.8) 39 (8.9)\n $800–$999 ($41,600–$51,999) 45 (9.8) 31 (9.3) 68 (9.8) 46 (10.6)\n $1,000–$1,249 ($52,000–$64,999) 39 (8.5) 32 (9.6) 48 (6.9) 38 (8.7)\n $1,250–$1,499 ($65,000–$77,999) 28 (6.1) 22 (6.6) 59 (8.5) 41 (9.4)\n $1,500–$1,999 ($78,000–$103,999) 72 (15.7) 50 (15.0) 72 (10.3) 48 (11.0)\n $2,000 or more ($104,000 or more) 81 (17.6) 62 (18.6) 108 (15.5) 74 (17.0)\n Prefer not to answer 47 (10.2) 32 (9.6) 76 (10.9) 28 (6.4)\nEducation level, n (%)\n Completed junior/lower/primary school 18 (3.6) 17 (4.7) 6 (0.9) 0 (0.0)\n Completed senior/high/secondary school 133 (26.9) 98 (26.8) 265 (37.8) 132 (30.0)\n Postschool vocational qualification/diploma 147 (29.7) 111 (30.4) 138 (19.7) 94 (21.4)\n Undergraduate university degree 131 (26.5) 93 (25.5) 214 (30.5) 159 (36.1)\n Postgraduate university degree 66 (13.3) 46 (12.6) 78 (11.1) 55 (12.5)\nSD standard deviation.\naTwo participants in the Australian sample did not report their gender and four participants in the U.S. sample did not report their gender.\nbFour participants in the U.S. sample did not report their employment status.\ncFour participants in the U.S. sample did not report their marital status.\ndFour participants in the U.S. sample did not report their ethnicity.\neThirty-one participants in the Australian sample did not report their income and four participants in the U.S. sample did not report their income.\n\nDesign and Procedure\nThe study adopted a prospective correlational design with self-report measures of social cognition constructs from the proposed integrated model, intention, and past social distancing behavior administered at an initial data collection occasion in a survey administered using the Qualtrics online survey tool. Social cognition measures included the theory of planned behavior (attitude, subjective norm, and PBC), moral norm, anticipated regret, action planning, and habit constructs. Participants were informed that they were participating in a survey on their social distancing behavior and provided with information outlining study requirements. They were required to provide informed consent before proceeding with the survey. Participants were also provided with instructions on how to complete study measures and a definition of the target behavior: “The following survey will ask about your beliefs and attitudes about ‘social distancing’. What do we mean by social distancing? Social distancing (also known as ‘physical distancing’) is deliberately increasing the physical space between people to avoid spreading illness. The World Health Organization and other world leading health authorities suggest that you should maintain at least a 1–2 meter (3–6 feet) distance from other people to lessen the chances of getting infected with COVID-19. When answering the questions in this survey, think about your social distancing behavior (i.e., maintaining at least a 1–2 m (3–6 ft) distance from other people).” One week later, participants were re-contacted by the panel company and asked to self-report their habit and social distancing behavior over the previous week using the same measures used at the initial data collection occasion. Participants received a fixed sum of money for their participation based on expected completion time consistent with the panel company’s published rates. Approval for study procedures was granted prior to data collection from the Griffith University Human Research Ethics Committee.\n\nMeasures\nStudy measures were multi-item self-report measures of constructs based on published guidelines and measures used in previous studies [13, 29, 30]. Participants provided their responses on scales with seven-point response options. Complete study measures are provided in Supplementary Appendix A.\n\nSocial cognition constructs\nMulti-item measures of attitude, subjective norm, PBC, moral norm, anticipated regret, and action planning were developed according to published guidelines [13, 29]. Each measure made explicit reference to the target behavior of social distancing, and participants were reminded of the definition of social distancing before completing the measures.\n\nIntention\nParticipants’ intention to participate in social distancing behavior over the next week was measured using a scale developed according to published guidelines [31].\n\nHabit\nHabit was measured at both time points using the behavioral automaticity items of Verplanken and Orbell’s self-report habit index [25]. The measure measures individuals’ reflections on the extent to which the behavior is experienced as automatic and enacted without thought.\n\nPast behavior and behavior\nParticipants self-reported their participation in social distancing behavior to minimize the transmission of the SARS-CoV-2 virus that causes COVID-19. The measure comprised two items prompting participants to report their frequency of social distancing behavior in the previous week. This is based on previously used self-report behavioral measures that have demonstrated concurrent validity with non-self-report measures in other behavioral contexts [32].\n\nDemographic variables\nParticipants self-reported their age in years, gender, employment status (currently unemployed/full-time caregiver, currently full-time employed, part-time employed, or on leave without pay/furloughed), marital status (married, widowed, separated/divorced, never married, or in a de factor relationship), annual household income stratified by 11 income levels based on Australian and U.S. national averages, highest level of formal education (completed junior/lower/primary school, completed senior/high/secondary school, postschool vocational qualification/diploma, further education diploma, undergraduate university degree, or postgraduate university degree), and ethnicity (Black, Caucasian/White, Asian, or Middle-eastern). Binary income (low income vs. middle/high income), highest education level (completed school education only vs. completed postschool education), and ethnicity (Caucasian/White vs. non-White) variables were computed for use in subsequent analyses.\n\nData Analysis\nHypothesized relations among the integrated model constructs were tested in the Australian and U.S. samples separately using variance-based structural equation modeling implemented in the WARP 7.0 analysis package [33]. Model parameters and standard errors (SEs) were computed using the “Stable3” estimation method, which has been shown to provide the most precise parameter estimates in complex structural models in smaller samples and outperforms bootstrapping methods in simulation studies [33]. Simulation studies have also shown this method to provide more consistent and precise estimates in data containing outliers, which may inflate SEs and lead to abnormally high p-values [33]. Two models were estimated in each sample: a model testing predictions of the proposed integrated model with the binary demographic variables also included as covariates (Model 1; Fig. 1, upper panel) and a model that included effects of past social distancing behavior (Model 2; Fig. 1, lower panel). All constructs were latent variables indicated by single or multiple items. There were no missing data for the social cognition and self-reported behavioral variables. There were a few instances of missing data for the demographic variables ranging from 0.5% to 8.8% in the Australia sample, and 0.9% to 6.4% in the U.S. sample. Missing data are reported in Supplementary Appendix B. Missing data were imputed using stochastic hierarchical regression [33].\nThe analysis afforded a number of analyses to evaluate the adequacy of measures used to indicate the latent variables in the model. Construct validity of the latent factors for the social cognition, intention, and behavioral variables was established using the normalized factor pattern loadings after oblique rotation and Kaiser normalization [33] and the average variance extracted (AVE), which should approach or exceed .700 and .500, respectively. Internal consistency of the factors was estimated using omega reliability coefficients (ω) and composite reliability coefficients (ρ), which should exceed .700 and ideally approach .900. We also conducted tests of the discriminant validity of the constructs in the model. Discriminant validity was supported when the square root of the AVE for each latent variable exceeded its correlation with other latent variables.\nAdequacy of the proposed model in describing the data was established using the goodness-of-fit (GoF) index with values of .100, .250, and .360 corresponding to small, medium, and large effect sizes. Further information on model quality was provided by the average path coefficient and average R2 coefficient. These indices summarize the average parameter estimates of relations in the model and the amount of variance explained in each dependent variable, respectively, and should be statistically significant for a good-quality model. In addition, an overall GoF index is provided by the average block variance inflation factor for model parameters and the average full collinearity variance inflation factor, which should be equal to or lower than 3.3 for well-fitting models. These indices indicate the extent to which latent variables in the model overlap and contribute to model multicollinearity. They, therefore, provide an indication as to the uniqueness of the existing latent variables in the model. Four further indices were also used to evaluate model quality: the Simpson’s paradox ratio (SPR), R2 contribution ratio (R2CR), the statistical suppression ratio (SSR), and the nonlinear bivariate causality direction ratio (NLBCDR). The SPR indicates whether the model is free from incidences of Simpson’s paradox (i.e., when the path coefficient and the correlation associated with a latent variable have opposite signs), indicating a causality problem. The SPR should exceed .700 and ideally approach 1.000. The R2CR and SSR provide indication of the extent to which models are free from instances of negative R2 contributions and statistical suppression. The R2CR and SSR should exceed .900 and .700, respectively. The NLBCDR provides an estimate of the extent to which the proposed “causal” associations in the proposed model are more tenable than those in the opposite direction and provide an initial indicator of support for the hypothesized directions of the causal links in the proposed model compared to if the proposed direction were reversed. The NLBCDR should exceed .700 for high-quality models. Kock [33] provides further technical details on model fit and quality indices.\nModel effects were estimated using standardized path coefficients with confidence intervals (CIs) and test statistics. Effect sizes were estimated using a variant of Cohen’s f-square coefficient and represent the individual contribution of the predictor variable to the R2 coefficients of the criterion latent variable. Values of .02, .15, and .35 represent small, medium, and large effect sizes, respectively. Differences in the path coefficients in the models across the samples were tested using multiple-group analysis using the Satterthwaite method with two-tailed significance tests.\nWe also tested whether the inclusion of participants that were never under a “shelter-in-place” order, or had the “shelter-in-place” order lifted during the study, affected predicted relations in the models. The small numbers of participants that were, at some point, not subjected to “shelter-in-place” orders meant we could not conduct a formal moderator analysis, so we conducted a sensitivity analysis testing whether effects in the models differed if data from these participants were excluded. Models excluding and including past behavior were estimated in samples excluding participants who were never subject to a “shelter-in place” order, and in the sample that were never subject to an order, or who had the order lifted at some stage during the study. Formal comparisons of parameter estimates in these models with those from the full sample were made using the Satterthwaite method. Data files, analysis scripts, and output files for all analyses are available online: https://osf.io/x9tms/."}