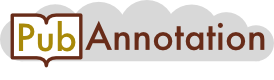
PMC:7252096 / 111157-113145
Annnotations
LitCovid-PD-FMA-UBERON
{"project":"LitCovid-PD-FMA-UBERON","denotations":[{"id":"T103","span":{"begin":195,"end":201},"obj":"Body_part"},{"id":"T104","span":{"begin":490,"end":495},"obj":"Body_part"},{"id":"T105","span":{"begin":666,"end":671},"obj":"Body_part"},{"id":"T106","span":{"begin":781,"end":786},"obj":"Body_part"},{"id":"T107","span":{"begin":906,"end":911},"obj":"Body_part"},{"id":"T108","span":{"begin":1278,"end":1283},"obj":"Body_part"},{"id":"T109","span":{"begin":1530,"end":1535},"obj":"Body_part"},{"id":"T110","span":{"begin":1563,"end":1567},"obj":"Body_part"},{"id":"T111","span":{"begin":1814,"end":1819},"obj":"Body_part"}],"attributes":[{"id":"A103","pred":"fma_id","subj":"T103","obj":"http://purl.org/sig/ont/fma/fma84116"},{"id":"A104","pred":"fma_id","subj":"T104","obj":"http://purl.org/sig/ont/fma/fma68646"},{"id":"A105","pred":"fma_id","subj":"T105","obj":"http://purl.org/sig/ont/fma/fma68646"},{"id":"A106","pred":"fma_id","subj":"T106","obj":"http://purl.org/sig/ont/fma/fma68646"},{"id":"A107","pred":"fma_id","subj":"T107","obj":"http://purl.org/sig/ont/fma/fma24901"},{"id":"A108","pred":"fma_id","subj":"T108","obj":"http://purl.org/sig/ont/fma/fma68646"},{"id":"A109","pred":"fma_id","subj":"T109","obj":"http://purl.org/sig/ont/fma/fma68646"},{"id":"A110","pred":"fma_id","subj":"T110","obj":"http://purl.org/sig/ont/fma/fma68646"},{"id":"A111","pred":"fma_id","subj":"T111","obj":"http://purl.org/sig/ont/fma/fma68646"}],"text":"Libraries corresponding to 4 mice, with 2 Seq-Well arrays per mouse were sequenced using Illumina NextSeq as described (Gierahn et al., 2017, Hughes et al., 2019). Reads were aligned to the mm10 genome and processed according to the Drop-Seq Computational Protocol v2.0 (https://github.com/broadinstitute/Drop-seq). Data was normalized and scaled using the Seurat R package v2.3.4 (https://satijalab.org/seurat/): transforming the data to loge(UMI+1) and applying a scale factor of 10,000. Cells with fewer than 1000 UMIs and 500 unique genes were removed. To identify major axes of variation within our data, we first examined only highly variable genes across all cells, yielding approximately 5,000 variable genes. An approximate principal component analysis was applied to the cells to generate 200 principal components (PCs). Using a combination of the Jackstraw function in Seurat and observing the “elbow” of the standard deviations of PCs, we chose the top 70 PCs for subsequent clustering and visualization. For 2D visualization, we used a Uniform Manifold Approximation and Projection (UMAP) dimensionality reduction technique (https://github.com/lmcinnes/umap) with “min_dist” set to 0.3 and “n_neighbors” set to 50. To identify clusters of transcriptionally similar cells, we employed unsupervised clustering as described above using the FindClusters tool within the Seurat R package with default parameters and k.param set to 10. Resolution was chosen based on maximization of the average silhouette width across all cells. Clusters were merged if a cell type expressed fewer than 25 significantly upregulated genes by differential expression test (FindAllMarkers implemented in Seurat, setting “test.use” to “bimod,” Bonferroni-adjusted p value cutoff \u003c 0.001). Differential expression tests between cells from saline-treated or IFNa-treated mice were assessed using the FindMarkers function with “test.use” set to “bimod. This dataset can be visualized and downloaded here:"}
LitCovid-PD-UBERON
{"project":"LitCovid-PD-UBERON","denotations":[{"id":"T250","span":{"begin":466,"end":471},"obj":"Body_part"},{"id":"T251","span":{"begin":906,"end":911},"obj":"Body_part"}],"attributes":[{"id":"A250","pred":"uberon_id","subj":"T250","obj":"http://purl.obolibrary.org/obo/UBERON_0002542"},{"id":"A251","pred":"uberon_id","subj":"T251","obj":"http://purl.obolibrary.org/obo/UBERON_0001461"}],"text":"Libraries corresponding to 4 mice, with 2 Seq-Well arrays per mouse were sequenced using Illumina NextSeq as described (Gierahn et al., 2017, Hughes et al., 2019). Reads were aligned to the mm10 genome and processed according to the Drop-Seq Computational Protocol v2.0 (https://github.com/broadinstitute/Drop-seq). Data was normalized and scaled using the Seurat R package v2.3.4 (https://satijalab.org/seurat/): transforming the data to loge(UMI+1) and applying a scale factor of 10,000. Cells with fewer than 1000 UMIs and 500 unique genes were removed. To identify major axes of variation within our data, we first examined only highly variable genes across all cells, yielding approximately 5,000 variable genes. An approximate principal component analysis was applied to the cells to generate 200 principal components (PCs). Using a combination of the Jackstraw function in Seurat and observing the “elbow” of the standard deviations of PCs, we chose the top 70 PCs for subsequent clustering and visualization. For 2D visualization, we used a Uniform Manifold Approximation and Projection (UMAP) dimensionality reduction technique (https://github.com/lmcinnes/umap) with “min_dist” set to 0.3 and “n_neighbors” set to 50. To identify clusters of transcriptionally similar cells, we employed unsupervised clustering as described above using the FindClusters tool within the Seurat R package with default parameters and k.param set to 10. Resolution was chosen based on maximization of the average silhouette width across all cells. Clusters were merged if a cell type expressed fewer than 25 significantly upregulated genes by differential expression test (FindAllMarkers implemented in Seurat, setting “test.use” to “bimod,” Bonferroni-adjusted p value cutoff \u003c 0.001). Differential expression tests between cells from saline-treated or IFNa-treated mice were assessed using the FindMarkers function with “test.use” set to “bimod. This dataset can be visualized and downloaded here:"}
LitCovid-PD-CLO
{"project":"LitCovid-PD-CLO","denotations":[{"id":"T154","span":{"begin":62,"end":67},"obj":"http://purl.obolibrary.org/obo/CLO_0007836"},{"id":"T155","span":{"begin":464,"end":465},"obj":"http://purl.obolibrary.org/obo/CLO_0001020"},{"id":"T156","span":{"begin":490,"end":495},"obj":"http://purl.obolibrary.org/obo/GO_0005623"},{"id":"T157","span":{"begin":537,"end":542},"obj":"http://purl.obolibrary.org/obo/OGG_0000000002"},{"id":"T158","span":{"begin":649,"end":654},"obj":"http://purl.obolibrary.org/obo/OGG_0000000002"},{"id":"T159","span":{"begin":666,"end":671},"obj":"http://purl.obolibrary.org/obo/GO_0005623"},{"id":"T160","span":{"begin":711,"end":716},"obj":"http://purl.obolibrary.org/obo/OGG_0000000002"},{"id":"T161","span":{"begin":781,"end":786},"obj":"http://purl.obolibrary.org/obo/GO_0005623"},{"id":"T162","span":{"begin":837,"end":838},"obj":"http://purl.obolibrary.org/obo/CLO_0001020"},{"id":"T163","span":{"begin":1047,"end":1048},"obj":"http://purl.obolibrary.org/obo/CLO_0001020"},{"id":"T164","span":{"begin":1278,"end":1283},"obj":"http://purl.obolibrary.org/obo/GO_0005623"},{"id":"T165","span":{"begin":1530,"end":1535},"obj":"http://purl.obolibrary.org/obo/GO_0005623"},{"id":"T166","span":{"begin":1561,"end":1562},"obj":"http://purl.obolibrary.org/obo/CLO_0001020"},{"id":"T167","span":{"begin":1563,"end":1572},"obj":"http://purl.obolibrary.org/obo/CL_0000000"},{"id":"T168","span":{"begin":1623,"end":1628},"obj":"http://purl.obolibrary.org/obo/OGG_0000000002"},{"id":"T169","span":{"begin":1656,"end":1660},"obj":"http://purl.obolibrary.org/obo/UBERON_0000473"},{"id":"T170","span":{"begin":1800,"end":1805},"obj":"http://purl.obolibrary.org/obo/UBERON_0000473"},{"id":"T171","span":{"begin":1814,"end":1819},"obj":"http://purl.obolibrary.org/obo/GO_0005623"}],"text":"Libraries corresponding to 4 mice, with 2 Seq-Well arrays per mouse were sequenced using Illumina NextSeq as described (Gierahn et al., 2017, Hughes et al., 2019). Reads were aligned to the mm10 genome and processed according to the Drop-Seq Computational Protocol v2.0 (https://github.com/broadinstitute/Drop-seq). Data was normalized and scaled using the Seurat R package v2.3.4 (https://satijalab.org/seurat/): transforming the data to loge(UMI+1) and applying a scale factor of 10,000. Cells with fewer than 1000 UMIs and 500 unique genes were removed. To identify major axes of variation within our data, we first examined only highly variable genes across all cells, yielding approximately 5,000 variable genes. An approximate principal component analysis was applied to the cells to generate 200 principal components (PCs). Using a combination of the Jackstraw function in Seurat and observing the “elbow” of the standard deviations of PCs, we chose the top 70 PCs for subsequent clustering and visualization. For 2D visualization, we used a Uniform Manifold Approximation and Projection (UMAP) dimensionality reduction technique (https://github.com/lmcinnes/umap) with “min_dist” set to 0.3 and “n_neighbors” set to 50. To identify clusters of transcriptionally similar cells, we employed unsupervised clustering as described above using the FindClusters tool within the Seurat R package with default parameters and k.param set to 10. Resolution was chosen based on maximization of the average silhouette width across all cells. Clusters were merged if a cell type expressed fewer than 25 significantly upregulated genes by differential expression test (FindAllMarkers implemented in Seurat, setting “test.use” to “bimod,” Bonferroni-adjusted p value cutoff \u003c 0.001). Differential expression tests between cells from saline-treated or IFNa-treated mice were assessed using the FindMarkers function with “test.use” set to “bimod. This dataset can be visualized and downloaded here:"}
LitCovid-PD-GO-BP
{"project":"LitCovid-PD-GO-BP","denotations":[{"id":"T6","span":{"begin":1252,"end":1269},"obj":"http://purl.obolibrary.org/obo/GO_0006351"}],"text":"Libraries corresponding to 4 mice, with 2 Seq-Well arrays per mouse were sequenced using Illumina NextSeq as described (Gierahn et al., 2017, Hughes et al., 2019). Reads were aligned to the mm10 genome and processed according to the Drop-Seq Computational Protocol v2.0 (https://github.com/broadinstitute/Drop-seq). Data was normalized and scaled using the Seurat R package v2.3.4 (https://satijalab.org/seurat/): transforming the data to loge(UMI+1) and applying a scale factor of 10,000. Cells with fewer than 1000 UMIs and 500 unique genes were removed. To identify major axes of variation within our data, we first examined only highly variable genes across all cells, yielding approximately 5,000 variable genes. An approximate principal component analysis was applied to the cells to generate 200 principal components (PCs). Using a combination of the Jackstraw function in Seurat and observing the “elbow” of the standard deviations of PCs, we chose the top 70 PCs for subsequent clustering and visualization. For 2D visualization, we used a Uniform Manifold Approximation and Projection (UMAP) dimensionality reduction technique (https://github.com/lmcinnes/umap) with “min_dist” set to 0.3 and “n_neighbors” set to 50. To identify clusters of transcriptionally similar cells, we employed unsupervised clustering as described above using the FindClusters tool within the Seurat R package with default parameters and k.param set to 10. Resolution was chosen based on maximization of the average silhouette width across all cells. Clusters were merged if a cell type expressed fewer than 25 significantly upregulated genes by differential expression test (FindAllMarkers implemented in Seurat, setting “test.use” to “bimod,” Bonferroni-adjusted p value cutoff \u003c 0.001). Differential expression tests between cells from saline-treated or IFNa-treated mice were assessed using the FindMarkers function with “test.use” set to “bimod. This dataset can be visualized and downloaded here:"}
LitCovid-sentences
{"project":"LitCovid-sentences","denotations":[{"id":"T797","span":{"begin":0,"end":163},"obj":"Sentence"},{"id":"T798","span":{"begin":164,"end":315},"obj":"Sentence"},{"id":"T799","span":{"begin":316,"end":489},"obj":"Sentence"},{"id":"T800","span":{"begin":490,"end":556},"obj":"Sentence"},{"id":"T801","span":{"begin":557,"end":717},"obj":"Sentence"},{"id":"T802","span":{"begin":718,"end":830},"obj":"Sentence"},{"id":"T803","span":{"begin":831,"end":1016},"obj":"Sentence"},{"id":"T804","span":{"begin":1017,"end":1227},"obj":"Sentence"},{"id":"T805","span":{"begin":1228,"end":1442},"obj":"Sentence"},{"id":"T806","span":{"begin":1443,"end":1536},"obj":"Sentence"},{"id":"T807","span":{"begin":1537,"end":1775},"obj":"Sentence"},{"id":"T808","span":{"begin":1776,"end":1936},"obj":"Sentence"},{"id":"T809","span":{"begin":1937,"end":1988},"obj":"Sentence"}],"namespaces":[{"prefix":"_base","uri":"http://pubannotation.org/ontology/tao.owl#"}],"text":"Libraries corresponding to 4 mice, with 2 Seq-Well arrays per mouse were sequenced using Illumina NextSeq as described (Gierahn et al., 2017, Hughes et al., 2019). Reads were aligned to the mm10 genome and processed according to the Drop-Seq Computational Protocol v2.0 (https://github.com/broadinstitute/Drop-seq). Data was normalized and scaled using the Seurat R package v2.3.4 (https://satijalab.org/seurat/): transforming the data to loge(UMI+1) and applying a scale factor of 10,000. Cells with fewer than 1000 UMIs and 500 unique genes were removed. To identify major axes of variation within our data, we first examined only highly variable genes across all cells, yielding approximately 5,000 variable genes. An approximate principal component analysis was applied to the cells to generate 200 principal components (PCs). Using a combination of the Jackstraw function in Seurat and observing the “elbow” of the standard deviations of PCs, we chose the top 70 PCs for subsequent clustering and visualization. For 2D visualization, we used a Uniform Manifold Approximation and Projection (UMAP) dimensionality reduction technique (https://github.com/lmcinnes/umap) with “min_dist” set to 0.3 and “n_neighbors” set to 50. To identify clusters of transcriptionally similar cells, we employed unsupervised clustering as described above using the FindClusters tool within the Seurat R package with default parameters and k.param set to 10. Resolution was chosen based on maximization of the average silhouette width across all cells. Clusters were merged if a cell type expressed fewer than 25 significantly upregulated genes by differential expression test (FindAllMarkers implemented in Seurat, setting “test.use” to “bimod,” Bonferroni-adjusted p value cutoff \u003c 0.001). Differential expression tests between cells from saline-treated or IFNa-treated mice were assessed using the FindMarkers function with “test.use” set to “bimod. This dataset can be visualized and downloaded here:"}
LitCovid-PubTator
{"project":"LitCovid-PubTator","denotations":[{"id":"2768","span":{"begin":1843,"end":1847},"obj":"Gene"},{"id":"2769","span":{"begin":29,"end":33},"obj":"Species"},{"id":"2770","span":{"begin":62,"end":67},"obj":"Species"},{"id":"2771","span":{"begin":1856,"end":1860},"obj":"Species"}],"attributes":[{"id":"A2768","pred":"tao:has_database_id","subj":"2768","obj":"Gene:111654"},{"id":"A2769","pred":"tao:has_database_id","subj":"2769","obj":"Tax:10090"},{"id":"A2770","pred":"tao:has_database_id","subj":"2770","obj":"Tax:10090"},{"id":"A2771","pred":"tao:has_database_id","subj":"2771","obj":"Tax:10090"}],"namespaces":[{"prefix":"Tax","uri":"https://www.ncbi.nlm.nih.gov/taxonomy/"},{"prefix":"MESH","uri":"https://id.nlm.nih.gov/mesh/"},{"prefix":"Gene","uri":"https://www.ncbi.nlm.nih.gov/gene/"},{"prefix":"CVCL","uri":"https://web.expasy.org/cellosaurus/CVCL_"}],"text":"Libraries corresponding to 4 mice, with 2 Seq-Well arrays per mouse were sequenced using Illumina NextSeq as described (Gierahn et al., 2017, Hughes et al., 2019). Reads were aligned to the mm10 genome and processed according to the Drop-Seq Computational Protocol v2.0 (https://github.com/broadinstitute/Drop-seq). Data was normalized and scaled using the Seurat R package v2.3.4 (https://satijalab.org/seurat/): transforming the data to loge(UMI+1) and applying a scale factor of 10,000. Cells with fewer than 1000 UMIs and 500 unique genes were removed. To identify major axes of variation within our data, we first examined only highly variable genes across all cells, yielding approximately 5,000 variable genes. An approximate principal component analysis was applied to the cells to generate 200 principal components (PCs). Using a combination of the Jackstraw function in Seurat and observing the “elbow” of the standard deviations of PCs, we chose the top 70 PCs for subsequent clustering and visualization. For 2D visualization, we used a Uniform Manifold Approximation and Projection (UMAP) dimensionality reduction technique (https://github.com/lmcinnes/umap) with “min_dist” set to 0.3 and “n_neighbors” set to 50. To identify clusters of transcriptionally similar cells, we employed unsupervised clustering as described above using the FindClusters tool within the Seurat R package with default parameters and k.param set to 10. Resolution was chosen based on maximization of the average silhouette width across all cells. Clusters were merged if a cell type expressed fewer than 25 significantly upregulated genes by differential expression test (FindAllMarkers implemented in Seurat, setting “test.use” to “bimod,” Bonferroni-adjusted p value cutoff \u003c 0.001). Differential expression tests between cells from saline-treated or IFNa-treated mice were assessed using the FindMarkers function with “test.use” set to “bimod. This dataset can be visualized and downloaded here:"}
2_test
{"project":"2_test","denotations":[{"id":"32413319-28192419-20790582","span":{"begin":136,"end":140},"obj":"28192419"}],"text":"Libraries corresponding to 4 mice, with 2 Seq-Well arrays per mouse were sequenced using Illumina NextSeq as described (Gierahn et al., 2017, Hughes et al., 2019). Reads were aligned to the mm10 genome and processed according to the Drop-Seq Computational Protocol v2.0 (https://github.com/broadinstitute/Drop-seq). Data was normalized and scaled using the Seurat R package v2.3.4 (https://satijalab.org/seurat/): transforming the data to loge(UMI+1) and applying a scale factor of 10,000. Cells with fewer than 1000 UMIs and 500 unique genes were removed. To identify major axes of variation within our data, we first examined only highly variable genes across all cells, yielding approximately 5,000 variable genes. An approximate principal component analysis was applied to the cells to generate 200 principal components (PCs). Using a combination of the Jackstraw function in Seurat and observing the “elbow” of the standard deviations of PCs, we chose the top 70 PCs for subsequent clustering and visualization. For 2D visualization, we used a Uniform Manifold Approximation and Projection (UMAP) dimensionality reduction technique (https://github.com/lmcinnes/umap) with “min_dist” set to 0.3 and “n_neighbors” set to 50. To identify clusters of transcriptionally similar cells, we employed unsupervised clustering as described above using the FindClusters tool within the Seurat R package with default parameters and k.param set to 10. Resolution was chosen based on maximization of the average silhouette width across all cells. Clusters were merged if a cell type expressed fewer than 25 significantly upregulated genes by differential expression test (FindAllMarkers implemented in Seurat, setting “test.use” to “bimod,” Bonferroni-adjusted p value cutoff \u003c 0.001). Differential expression tests between cells from saline-treated or IFNa-treated mice were assessed using the FindMarkers function with “test.use” set to “bimod. This dataset can be visualized and downloaded here:"}