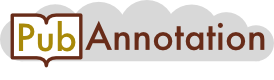
PMC:7252096 / 102220-126093
Annnotations
LitCovid-PD-FMA-UBERON
{"project":"LitCovid-PD-FMA-UBERON","denotations":[{"id":"T21","span":{"begin":59,"end":63},"obj":"Body_part"},{"id":"T22","span":{"begin":68,"end":73},"obj":"Body_part"},{"id":"T23","span":{"begin":131,"end":138},"obj":"Body_part"},{"id":"T24","span":{"begin":227,"end":233},"obj":"Body_part"},{"id":"T25","span":{"begin":669,"end":674},"obj":"Body_part"},{"id":"T26","span":{"begin":786,"end":791},"obj":"Body_part"},{"id":"T27","span":{"begin":856,"end":861},"obj":"Body_part"},{"id":"T28","span":{"begin":1080,"end":1084},"obj":"Body_part"},{"id":"T29","span":{"begin":1341,"end":1346},"obj":"Body_part"},{"id":"T30","span":{"begin":1589,"end":1593},"obj":"Body_part"},{"id":"T31","span":{"begin":1616,"end":1620},"obj":"Body_part"},{"id":"T32","span":{"begin":1856,"end":1861},"obj":"Body_part"},{"id":"T33","span":{"begin":1896,"end":1907},"obj":"Body_part"},{"id":"T34","span":{"begin":2019,"end":2035},"obj":"Body_part"},{"id":"T35","span":{"begin":2030,"end":2035},"obj":"Body_part"},{"id":"T36","span":{"begin":2040,"end":2051},"obj":"Body_part"},{"id":"T37","span":{"begin":2230,"end":2234},"obj":"Body_part"},{"id":"T38","span":{"begin":2235,"end":2241},"obj":"Body_part"},{"id":"T39","span":{"begin":2348,"end":2354},"obj":"Body_part"},{"id":"T40","span":{"begin":2783,"end":2787},"obj":"Body_part"},{"id":"T41","span":{"begin":2798,"end":2802},"obj":"Body_part"},{"id":"T42","span":{"begin":3145,"end":3149},"obj":"Body_part"},{"id":"T43","span":{"begin":3172,"end":3177},"obj":"Body_part"},{"id":"T44","span":{"begin":3250,"end":3255},"obj":"Body_part"},{"id":"T45","span":{"begin":3305,"end":3309},"obj":"Body_part"},{"id":"T46","span":{"begin":3404,"end":3408},"obj":"Body_part"},{"id":"T47","span":{"begin":3573,"end":3578},"obj":"Body_part"},{"id":"T48","span":{"begin":3594,"end":3598},"obj":"Body_part"},{"id":"T49","span":{"begin":3692,"end":3697},"obj":"Body_part"},{"id":"T50","span":{"begin":3714,"end":3719},"obj":"Body_part"},{"id":"T51","span":{"begin":3720,"end":3728},"obj":"Body_part"},{"id":"T52","span":{"begin":3739,"end":3744},"obj":"Body_part"},{"id":"T53","span":{"begin":3769,"end":3782},"obj":"Body_part"},{"id":"T54","span":{"begin":3777,"end":3782},"obj":"Body_part"},{"id":"T55","span":{"begin":3822,"end":3834},"obj":"Body_part"},{"id":"T56","span":{"begin":3822,"end":3826},"obj":"Body_part"},{"id":"T57","span":{"begin":3913,"end":3924},"obj":"Body_part"},{"id":"T58","span":{"begin":4065,"end":4081},"obj":"Body_part"},{"id":"T59","span":{"begin":4076,"end":4081},"obj":"Body_part"},{"id":"T60","span":{"begin":4260,"end":4265},"obj":"Body_part"},{"id":"T61","span":{"begin":4494,"end":4499},"obj":"Body_part"},{"id":"T62","span":{"begin":4624,"end":4628},"obj":"Body_part"},{"id":"T63","span":{"begin":4827,"end":4831},"obj":"Body_part"},{"id":"T64","span":{"begin":4939,"end":4943},"obj":"Body_part"},{"id":"T65","span":{"begin":5396,"end":5401},"obj":"Body_part"},{"id":"T66","span":{"begin":5538,"end":5542},"obj":"Body_part"},{"id":"T67","span":{"begin":5620,"end":5636},"obj":"Body_part"},{"id":"T68","span":{"begin":5631,"end":5636},"obj":"Body_part"},{"id":"T69","span":{"begin":5738,"end":5754},"obj":"Body_part"},{"id":"T70","span":{"begin":5749,"end":5754},"obj":"Body_part"},{"id":"T71","span":{"begin":5939,"end":5951},"obj":"Body_part"},{"id":"T72","span":{"begin":5945,"end":5951},"obj":"Body_part"},{"id":"T73","span":{"begin":6068,"end":6072},"obj":"Body_part"},{"id":"T74","span":{"begin":6123,"end":6125},"obj":"Body_part"},{"id":"T76","span":{"begin":6243,"end":6247},"obj":"Body_part"},{"id":"T77","span":{"begin":6251,"end":6255},"obj":"Body_part"},{"id":"T78","span":{"begin":6645,"end":6654},"obj":"Body_part"},{"id":"T79","span":{"begin":6678,"end":6694},"obj":"Body_part"},{"id":"T80","span":{"begin":6689,"end":6694},"obj":"Body_part"},{"id":"T81","span":{"begin":6720,"end":6724},"obj":"Body_part"},{"id":"T82","span":{"begin":6905,"end":6911},"obj":"Body_part"},{"id":"T83","span":{"begin":7016,"end":7023},"obj":"Body_part"},{"id":"T84","span":{"begin":7178,"end":7184},"obj":"Body_part"},{"id":"T85","span":{"begin":7337,"end":7341},"obj":"Body_part"},{"id":"T86","span":{"begin":7427,"end":7442},"obj":"Body_part"},{"id":"T87","span":{"begin":7438,"end":7442},"obj":"Body_part"},{"id":"T88","span":{"begin":7486,"end":7497},"obj":"Body_part"},{"id":"T89","span":{"begin":7510,"end":7521},"obj":"Body_part"},{"id":"T90","span":{"begin":7590,"end":7595},"obj":"Body_part"},{"id":"T91","span":{"begin":7606,"end":7611},"obj":"Body_part"},{"id":"T92","span":{"begin":7627,"end":7631},"obj":"Body_part"},{"id":"T93","span":{"begin":7657,"end":7662},"obj":"Body_part"},{"id":"T94","span":{"begin":7731,"end":7735},"obj":"Body_part"},{"id":"T95","span":{"begin":7825,"end":7841},"obj":"Body_part"},{"id":"T96","span":{"begin":7836,"end":7841},"obj":"Body_part"},{"id":"T97","span":{"begin":8014,"end":8024},"obj":"Body_part"},{"id":"T98","span":{"begin":8020,"end":8024},"obj":"Body_part"},{"id":"T99","span":{"begin":8025,"end":8033},"obj":"Body_part"},{"id":"T100","span":{"begin":8167,"end":8173},"obj":"Body_part"},{"id":"T101","span":{"begin":8924,"end":8936},"obj":"Body_part"},{"id":"T102","span":{"begin":8930,"end":8936},"obj":"Body_part"},{"id":"T103","span":{"begin":9132,"end":9138},"obj":"Body_part"},{"id":"T104","span":{"begin":9427,"end":9432},"obj":"Body_part"},{"id":"T105","span":{"begin":9603,"end":9608},"obj":"Body_part"},{"id":"T106","span":{"begin":9718,"end":9723},"obj":"Body_part"},{"id":"T107","span":{"begin":9843,"end":9848},"obj":"Body_part"},{"id":"T108","span":{"begin":10215,"end":10220},"obj":"Body_part"},{"id":"T109","span":{"begin":10467,"end":10472},"obj":"Body_part"},{"id":"T110","span":{"begin":10500,"end":10504},"obj":"Body_part"},{"id":"T111","span":{"begin":10751,"end":10756},"obj":"Body_part"},{"id":"T112","span":{"begin":11034,"end":11038},"obj":"Body_part"},{"id":"T113","span":{"begin":11150,"end":11156},"obj":"Body_part"},{"id":"T114","span":{"begin":11310,"end":11316},"obj":"Body_part"},{"id":"T115","span":{"begin":11351,"end":11356},"obj":"Body_part"},{"id":"T116","span":{"begin":11357,"end":11363},"obj":"Body_part"},{"id":"T117","span":{"begin":11918,"end":11921},"obj":"Body_part"},{"id":"T118","span":{"begin":12075,"end":12079},"obj":"Body_part"},{"id":"T119","span":{"begin":12084,"end":12088},"obj":"Body_part"},{"id":"T120","span":{"begin":12372,"end":12377},"obj":"Body_part"},{"id":"T121","span":{"begin":12549,"end":12560},"obj":"Body_part"},{"id":"T122","span":{"begin":12687,"end":12692},"obj":"Body_part"},{"id":"T123","span":{"begin":12694,"end":12699},"obj":"Body_part"},{"id":"T124","span":{"begin":12937,"end":12943},"obj":"Body_part"},{"id":"T125","span":{"begin":12968,"end":12975},"obj":"Body_part"},{"id":"T126","span":{"begin":13528,"end":13532},"obj":"Body_part"},{"id":"T127","span":{"begin":13583,"end":13587},"obj":"Body_part"},{"id":"T128","span":{"begin":13609,"end":13620},"obj":"Body_part"},{"id":"T129","span":{"begin":13680,"end":13684},"obj":"Body_part"},{"id":"T130","span":{"begin":13732,"end":13737},"obj":"Body_part"},{"id":"T131","span":{"begin":13749,"end":13753},"obj":"Body_part"},{"id":"T132","span":{"begin":13757,"end":13761},"obj":"Body_part"},{"id":"T133","span":{"begin":14023,"end":14028},"obj":"Body_part"},{"id":"T134","span":{"begin":14086,"end":14090},"obj":"Body_part"},{"id":"T135","span":{"begin":14142,"end":14146},"obj":"Body_part"},{"id":"T136","span":{"begin":14233,"end":14237},"obj":"Body_part"},{"id":"T137","span":{"begin":14600,"end":14616},"obj":"Body_part"},{"id":"T138","span":{"begin":14611,"end":14616},"obj":"Body_part"},{"id":"T139","span":{"begin":14618,"end":14629},"obj":"Body_part"},{"id":"T140","span":{"begin":14631,"end":14642},"obj":"Body_part"},{"id":"T141","span":{"begin":14647,"end":14657},"obj":"Body_part"},{"id":"T142","span":{"begin":14663,"end":14678},"obj":"Body_part"},{"id":"T143","span":{"begin":14674,"end":14678},"obj":"Body_part"},{"id":"T144","span":{"begin":14695,"end":14704},"obj":"Body_part"},{"id":"T145","span":{"begin":14838,"end":14853},"obj":"Body_part"},{"id":"T146","span":{"begin":14849,"end":14853},"obj":"Body_part"},{"id":"T147","span":{"begin":15043,"end":15058},"obj":"Body_part"},{"id":"T148","span":{"begin":15054,"end":15058},"obj":"Body_part"},{"id":"T149","span":{"begin":15102,"end":15106},"obj":"Body_part"},{"id":"T150","span":{"begin":15253,"end":15258},"obj":"Body_part"},{"id":"T151","span":{"begin":15346,"end":15350},"obj":"Body_part"},{"id":"T152","span":{"begin":15379,"end":15384},"obj":"Body_part"},{"id":"T153","span":{"begin":15810,"end":15815},"obj":"Body_part"},{"id":"T154","span":{"begin":15972,"end":15976},"obj":"Body_part"},{"id":"T155","span":{"begin":16329,"end":16333},"obj":"Body_part"},{"id":"T156","span":{"begin":16377,"end":16381},"obj":"Body_part"},{"id":"T157","span":{"begin":16396,"end":16400},"obj":"Body_part"},{"id":"T158","span":{"begin":16651,"end":16653},"obj":"Body_part"},{"id":"T160","span":{"begin":16753,"end":16757},"obj":"Body_part"},{"id":"T161","span":{"begin":16841,"end":16859},"obj":"Body_part"},{"id":"T162","span":{"begin":16905,"end":16907},"obj":"Body_part"},{"id":"T164","span":{"begin":17067,"end":17069},"obj":"Body_part"},{"id":"T166","span":{"begin":17155,"end":17159},"obj":"Body_part"},{"id":"T167","span":{"begin":17351,"end":17355},"obj":"Body_part"},{"id":"T168","span":{"begin":17370,"end":17374},"obj":"Body_part"},{"id":"T169","span":{"begin":17447,"end":17452},"obj":"Body_part"},{"id":"T170","span":{"begin":17453,"end":17458},"obj":"Body_part"},{"id":"T171","span":{"begin":17505,"end":17509},"obj":"Body_part"},{"id":"T172","span":{"begin":17571,"end":17575},"obj":"Body_part"},{"id":"T173","span":{"begin":17590,"end":17595},"obj":"Body_part"},{"id":"T174","span":{"begin":17733,"end":17737},"obj":"Body_part"},{"id":"T175","span":{"begin":17771,"end":17775},"obj":"Body_part"},{"id":"T176","span":{"begin":17926,"end":17930},"obj":"Body_part"},{"id":"T177","span":{"begin":18087,"end":18092},"obj":"Body_part"},{"id":"T178","span":{"begin":18161,"end":18165},"obj":"Body_part"},{"id":"T179","span":{"begin":18407,"end":18412},"obj":"Body_part"},{"id":"T180","span":{"begin":18657,"end":18661},"obj":"Body_part"},{"id":"T181","span":{"begin":18723,"end":18730},"obj":"Body_part"},{"id":"T182","span":{"begin":18750,"end":18755},"obj":"Body_part"},{"id":"T183","span":{"begin":18760,"end":18764},"obj":"Body_part"},{"id":"T184","span":{"begin":18905,"end":18910},"obj":"Body_part"},{"id":"T185","span":{"begin":18927,"end":18931},"obj":"Body_part"},{"id":"T186","span":{"begin":19017,"end":19022},"obj":"Body_part"},{"id":"T187","span":{"begin":19106,"end":19110},"obj":"Body_part"},{"id":"T188","span":{"begin":19176,"end":19180},"obj":"Body_part"},{"id":"T189","span":{"begin":19339,"end":19345},"obj":"Body_part"},{"id":"T190","span":{"begin":19640,"end":19644},"obj":"Body_part"},{"id":"T191","span":{"begin":19776,"end":19782},"obj":"Body_part"},{"id":"T192","span":{"begin":20045,"end":20050},"obj":"Body_part"},{"id":"T193","span":{"begin":20059,"end":20063},"obj":"Body_part"},{"id":"T194","span":{"begin":20121,"end":20125},"obj":"Body_part"},{"id":"T195","span":{"begin":20134,"end":20138},"obj":"Body_part"},{"id":"T196","span":{"begin":20219,"end":20224},"obj":"Body_part"},{"id":"T197","span":{"begin":20295,"end":20300},"obj":"Body_part"},{"id":"T198","span":{"begin":20370,"end":20375},"obj":"Body_part"},{"id":"T199","span":{"begin":20428,"end":20433},"obj":"Body_part"},{"id":"T200","span":{"begin":20521,"end":20526},"obj":"Body_part"},{"id":"T201","span":{"begin":20527,"end":20539},"obj":"Body_part"},{"id":"T202","span":{"begin":20527,"end":20531},"obj":"Body_part"},{"id":"T203","span":{"begin":20573,"end":20578},"obj":"Body_part"},{"id":"T204","span":{"begin":20618,"end":20623},"obj":"Body_part"},{"id":"T205","span":{"begin":20642,"end":20646},"obj":"Body_part"},{"id":"T206","span":{"begin":20647,"end":20659},"obj":"Body_part"},{"id":"T207","span":{"begin":20647,"end":20651},"obj":"Body_part"},{"id":"T208","span":{"begin":20693,"end":20698},"obj":"Body_part"},{"id":"T209","span":{"begin":20833,"end":20838},"obj":"Body_part"},{"id":"T210","span":{"begin":20848,"end":20853},"obj":"Body_part"},{"id":"T211","span":{"begin":20869,"end":20875},"obj":"Body_part"},{"id":"T212","span":{"begin":21017,"end":21021},"obj":"Body_part"},{"id":"T213","span":{"begin":21022,"end":21027},"obj":"Body_part"},{"id":"T214","span":{"begin":21073,"end":21077},"obj":"Body_part"},{"id":"T215","span":{"begin":21078,"end":21083},"obj":"Body_part"},{"id":"T216","span":{"begin":21129,"end":21134},"obj":"Body_part"},{"id":"T217","span":{"begin":21135,"end":21140},"obj":"Body_part"},{"id":"T218","span":{"begin":21186,"end":21191},"obj":"Body_part"},{"id":"T219","span":{"begin":21192,"end":21197},"obj":"Body_part"},{"id":"T220","span":{"begin":21233,"end":21237},"obj":"Body_part"},{"id":"T221","span":{"begin":21256,"end":21261},"obj":"Body_part"},{"id":"T222","span":{"begin":21280,"end":21285},"obj":"Body_part"},{"id":"T223","span":{"begin":21298,"end":21302},"obj":"Body_part"},{"id":"T224","span":{"begin":21587,"end":21591},"obj":"Body_part"},{"id":"T225","span":{"begin":21632,"end":21637},"obj":"Body_part"},{"id":"T226","span":{"begin":21735,"end":21746},"obj":"Body_part"},{"id":"T227","span":{"begin":21871,"end":21876},"obj":"Body_part"},{"id":"T228","span":{"begin":22080,"end":22085},"obj":"Body_part"},{"id":"T229","span":{"begin":22139,"end":22144},"obj":"Body_part"},{"id":"T230","span":{"begin":22191,"end":22196},"obj":"Body_part"},{"id":"T231","span":{"begin":22372,"end":22377},"obj":"Body_part"},{"id":"T232","span":{"begin":22431,"end":22436},"obj":"Body_part"},{"id":"T233","span":{"begin":22571,"end":22576},"obj":"Body_part"},{"id":"T234","span":{"begin":22810,"end":22815},"obj":"Body_part"},{"id":"T235","span":{"begin":22890,"end":22895},"obj":"Body_part"},{"id":"T236","span":{"begin":22972,"end":22977},"obj":"Body_part"},{"id":"T237","span":{"begin":23157,"end":23161},"obj":"Body_part"},{"id":"T238","span":{"begin":23780,"end":23784},"obj":"Body_part"}],"attributes":[{"id":"A21","pred":"fma_id","subj":"T21","obj":"http://purl.org/sig/ont/fma/fma7195"},{"id":"A22","pred":"fma_id","subj":"T22","obj":"http://purl.org/sig/ont/fma/fma7208"},{"id":"A23","pred":"fma_id","subj":"T23","obj":"http://purl.org/sig/ont/fma/fma9637"},{"id":"A24","pred":"fma_id","subj":"T24","obj":"http://purl.org/sig/ont/fma/fma84116"},{"id":"A25","pred":"fma_id","subj":"T25","obj":"http://purl.org/sig/ont/fma/fma68646"},{"id":"A26","pred":"fma_id","subj":"T26","obj":"http://purl.org/sig/ont/fma/fma68646"},{"id":"A27","pred":"fma_id","subj":"T27","obj":"http://purl.org/sig/ont/fma/fma68646"},{"id":"A28","pred":"fma_id","subj":"T28","obj":"http://purl.org/sig/ont/fma/fma68646"},{"id":"A29","pred":"fma_id","subj":"T29","obj":"http://purl.org/sig/ont/fma/fma68646"},{"id":"A30","pred":"fma_id","subj":"T30","obj":"http://purl.org/sig/ont/fma/fma68646"},{"id":"A31","pred":"fma_id","subj":"T31","obj":"http://purl.org/sig/ont/fma/fma68646"},{"id":"A32","pred":"fma_id","subj":"T32","obj":"http://purl.org/sig/ont/fma/fma68646"},{"id":"A33","pred":"fma_id","subj":"T33","obj":"http://purl.org/sig/ont/fma/fma62499"},{"id":"A34","pred":"fma_id","subj":"T34","obj":"http://purl.org/sig/ont/fma/fma66768"},{"id":"A35","pred":"fma_id","subj":"T35","obj":"http://purl.org/sig/ont/fma/fma68646"},{"id":"A36","pred":"fma_id","subj":"T36","obj":"http://purl.org/sig/ont/fma/fma62122"},{"id":"A37","pred":"fma_id","subj":"T37","obj":"http://purl.org/sig/ont/fma/fma7195"},{"id":"A38","pred":"fma_id","subj":"T38","obj":"http://purl.org/sig/ont/fma/fma9637"},{"id":"A39","pred":"fma_id","subj":"T39","obj":"http://purl.org/sig/ont/fma/fma84116"},{"id":"A40","pred":"fma_id","subj":"T40","obj":"http://purl.org/sig/ont/fma/fma68646"},{"id":"A41","pred":"fma_id","subj":"T41","obj":"http://purl.org/sig/ont/fma/fma74402"},{"id":"A42","pred":"fma_id","subj":"T42","obj":"http://purl.org/sig/ont/fma/fma68646"},{"id":"A43","pred":"fma_id","subj":"T43","obj":"http://purl.org/sig/ont/fma/fma68646"},{"id":"A44","pred":"fma_id","subj":"T44","obj":"http://purl.org/sig/ont/fma/fma68646"},{"id":"A45","pred":"fma_id","subj":"T45","obj":"http://purl.org/sig/ont/fma/fma68646"},{"id":"A46","pred":"fma_id","subj":"T46","obj":"http://purl.org/sig/ont/fma/fma74402"},{"id":"A47","pred":"fma_id","subj":"T47","obj":"http://purl.org/sig/ont/fma/fma68646"},{"id":"A48","pred":"fma_id","subj":"T48","obj":"http://purl.org/sig/ont/fma/fma7195"},{"id":"A49","pred":"fma_id","subj":"T49","obj":"http://purl.org/sig/ont/fma/fma68646"},{"id":"A50","pred":"fma_id","subj":"T50","obj":"http://purl.org/sig/ont/fma/fma68646"},{"id":"A51","pred":"fma_id","subj":"T51","obj":"http://purl.org/sig/ont/fma/fma62871"},{"id":"A52","pred":"fma_id","subj":"T52","obj":"http://purl.org/sig/ont/fma/fma68646"},{"id":"A53","pred":"fma_id","subj":"T53","obj":"http://purl.org/sig/ont/fma/fma70339"},{"id":"A54","pred":"fma_id","subj":"T54","obj":"http://purl.org/sig/ont/fma/fma68646"},{"id":"A55","pred":"fma_id","subj":"T55","obj":"http://purl.org/sig/ont/fma/fma62807"},{"id":"A56","pred":"fma_id","subj":"T56","obj":"http://purl.org/sig/ont/fma/fma68646"},{"id":"A57","pred":"fma_id","subj":"T57","obj":"http://purl.org/sig/ont/fma/fma62499"},{"id":"A58","pred":"fma_id","subj":"T58","obj":"http://purl.org/sig/ont/fma/fma66768"},{"id":"A59","pred":"fma_id","subj":"T59","obj":"http://purl.org/sig/ont/fma/fma68646"},{"id":"A60","pred":"fma_id","subj":"T60","obj":"http://purl.org/sig/ont/fma/fma7208"},{"id":"A61","pred":"fma_id","subj":"T61","obj":"http://purl.org/sig/ont/fma/fma68646"},{"id":"A62","pred":"fma_id","subj":"T62","obj":"http://purl.org/sig/ont/fma/fma68646"},{"id":"A63","pred":"fma_id","subj":"T63","obj":"http://purl.org/sig/ont/fma/fma74402"},{"id":"A64","pred":"fma_id","subj":"T64","obj":"http://purl.org/sig/ont/fma/fma68646"},{"id":"A65","pred":"fma_id","subj":"T65","obj":"http://purl.org/sig/ont/fma/fma68646"},{"id":"A66","pred":"fma_id","subj":"T66","obj":"http://purl.org/sig/ont/fma/fma68646"},{"id":"A67","pred":"fma_id","subj":"T67","obj":"http://purl.org/sig/ont/fma/fma66768"},{"id":"A68","pred":"fma_id","subj":"T68","obj":"http://purl.org/sig/ont/fma/fma68646"},{"id":"A69","pred":"fma_id","subj":"T69","obj":"http://purl.org/sig/ont/fma/fma66768"},{"id":"A70","pred":"fma_id","subj":"T70","obj":"http://purl.org/sig/ont/fma/fma68646"},{"id":"A71","pred":"fma_id","subj":"T71","obj":"http://purl.org/sig/ont/fma/fma59684"},{"id":"A72","pred":"fma_id","subj":"T72","obj":"http://purl.org/sig/ont/fma/fma85355"},{"id":"A73","pred":"fma_id","subj":"T73","obj":"http://purl.org/sig/ont/fma/fma68646"},{"id":"A74","pred":"fma_id","subj":"T74","obj":"http://purl.org/sig/ont/fma/fma13444"},{"id":"A75","pred":"fma_id","subj":"T74","obj":"http://purl.org/sig/ont/fma/fma68614"},{"id":"A76","pred":"fma_id","subj":"T76","obj":"http://purl.org/sig/ont/fma/fma68646"},{"id":"A77","pred":"fma_id","subj":"T77","obj":"http://purl.org/sig/ont/fma/fma74402"},{"id":"A78","pred":"fma_id","subj":"T78","obj":"http://purl.org/sig/ont/fma/fma84050"},{"id":"A79","pred":"fma_id","subj":"T79","obj":"http://purl.org/sig/ont/fma/fma66768"},{"id":"A80","pred":"fma_id","subj":"T80","obj":"http://purl.org/sig/ont/fma/fma68646"},{"id":"A81","pred":"fma_id","subj":"T81","obj":"http://purl.org/sig/ont/fma/fma74402"},{"id":"A82","pred":"fma_id","subj":"T82","obj":"http://purl.org/sig/ont/fma/fma9637"},{"id":"A83","pred":"fma_id","subj":"T83","obj":"http://purl.org/sig/ont/fma/fma9637"},{"id":"A84","pred":"fma_id","subj":"T84","obj":"http://purl.org/sig/ont/fma/fma84116"},{"id":"A85","pred":"fma_id","subj":"T85","obj":"http://purl.org/sig/ont/fma/fma68646"},{"id":"A86","pred":"fma_id","subj":"T86","obj":"http://purl.org/sig/ont/fma/fma66768"},{"id":"A87","pred":"fma_id","subj":"T87","obj":"http://purl.org/sig/ont/fma/fma68646"},{"id":"A88","pred":"fma_id","subj":"T88","obj":"http://purl.org/sig/ont/fma/fma62499"},{"id":"A89","pred":"fma_id","subj":"T89","obj":"http://purl.org/sig/ont/fma/fma62499"},{"id":"A90","pred":"fma_id","subj":"T90","obj":"http://purl.org/sig/ont/fma/fma68646"},{"id":"A91","pred":"fma_id","subj":"T91","obj":"http://purl.org/sig/ont/fma/fma68646"},{"id":"A92","pred":"fma_id","subj":"T92","obj":"http://purl.org/sig/ont/fma/fma68646"},{"id":"A93","pred":"fma_id","subj":"T93","obj":"http://purl.org/sig/ont/fma/fma68646"},{"id":"A94","pred":"fma_id","subj":"T94","obj":"http://purl.org/sig/ont/fma/fma68646"},{"id":"A95","pred":"fma_id","subj":"T95","obj":"http://purl.org/sig/ont/fma/fma66768"},{"id":"A96","pred":"fma_id","subj":"T96","obj":"http://purl.org/sig/ont/fma/fma68646"},{"id":"A97","pred":"fma_id","subj":"T97","obj":"http://purl.org/sig/ont/fma/fma62516"},{"id":"A98","pred":"fma_id","subj":"T98","obj":"http://purl.org/sig/ont/fma/fma68646"},{"id":"A99","pred":"fma_id","subj":"T99","obj":"http://purl.org/sig/ont/fma/fma84050"},{"id":"A100","pred":"fma_id","subj":"T100","obj":"http://purl.org/sig/ont/fma/fma84116"},{"id":"A101","pred":"fma_id","subj":"T101","obj":"http://purl.org/sig/ont/fma/fma59684"},{"id":"A102","pred":"fma_id","subj":"T102","obj":"http://purl.org/sig/ont/fma/fma85355"},{"id":"A103","pred":"fma_id","subj":"T103","obj":"http://purl.org/sig/ont/fma/fma84116"},{"id":"A104","pred":"fma_id","subj":"T104","obj":"http://purl.org/sig/ont/fma/fma68646"},{"id":"A105","pred":"fma_id","subj":"T105","obj":"http://purl.org/sig/ont/fma/fma68646"},{"id":"A106","pred":"fma_id","subj":"T106","obj":"http://purl.org/sig/ont/fma/fma68646"},{"id":"A107","pred":"fma_id","subj":"T107","obj":"http://purl.org/sig/ont/fma/fma24901"},{"id":"A108","pred":"fma_id","subj":"T108","obj":"http://purl.org/sig/ont/fma/fma68646"},{"id":"A109","pred":"fma_id","subj":"T109","obj":"http://purl.org/sig/ont/fma/fma68646"},{"id":"A110","pred":"fma_id","subj":"T110","obj":"http://purl.org/sig/ont/fma/fma68646"},{"id":"A111","pred":"fma_id","subj":"T111","obj":"http://purl.org/sig/ont/fma/fma68646"},{"id":"A112","pred":"fma_id","subj":"T112","obj":"http://purl.org/sig/ont/fma/fma7195"},{"id":"A113","pred":"fma_id","subj":"T113","obj":"http://purl.org/sig/ont/fma/fma84116"},{"id":"A114","pred":"fma_id","subj":"T114","obj":"http://purl.org/sig/ont/fma/fma84116"},{"id":"A115","pred":"fma_id","subj":"T115","obj":"http://purl.org/sig/ont/fma/fma7490"},{"id":"A116","pred":"fma_id","subj":"T116","obj":"http://purl.org/sig/ont/fma/fma84116"},{"id":"A117","pred":"fma_id","subj":"T117","obj":"http://purl.org/sig/ont/fma/fma67095"},{"id":"A118","pred":"fma_id","subj":"T118","obj":"http://purl.org/sig/ont/fma/fma68646"},{"id":"A119","pred":"fma_id","subj":"T119","obj":"http://purl.org/sig/ont/fma/fma68646"},{"id":"A120","pred":"fma_id","subj":"T120","obj":"http://purl.org/sig/ont/fma/fma68646"},{"id":"A121","pred":"fma_id","subj":"T121","obj":"http://purl.org/sig/ont/fma/fma62499"},{"id":"A122","pred":"fma_id","subj":"T122","obj":"http://purl.org/sig/ont/fma/fma68646"},{"id":"A123","pred":"fma_id","subj":"T123","obj":"http://purl.org/sig/ont/fma/fma68646"},{"id":"A124","pred":"fma_id","subj":"T124","obj":"http://purl.org/sig/ont/fma/fma84116"},{"id":"A125","pred":"fma_id","subj":"T125","obj":"http://purl.org/sig/ont/fma/fma84116"},{"id":"A126","pred":"fma_id","subj":"T126","obj":"http://purl.org/sig/ont/fma/fma68646"},{"id":"A127","pred":"fma_id","subj":"T127","obj":"http://purl.org/sig/ont/fma/fma68646"},{"id":"A128","pred":"fma_id","subj":"T128","obj":"http://purl.org/sig/ont/fma/fma62845"},{"id":"A129","pred":"fma_id","subj":"T129","obj":"http://purl.org/sig/ont/fma/fma68646"},{"id":"A130","pred":"fma_id","subj":"T130","obj":"http://purl.org/sig/ont/fma/fma68646"},{"id":"A131","pred":"fma_id","subj":"T131","obj":"http://purl.org/sig/ont/fma/fma74402"},{"id":"A132","pred":"fma_id","subj":"T132","obj":"http://purl.org/sig/ont/fma/fma68646"},{"id":"A133","pred":"fma_id","subj":"T133","obj":"http://purl.org/sig/ont/fma/fma68646"},{"id":"A134","pred":"fma_id","subj":"T134","obj":"http://purl.org/sig/ont/fma/fma68646"},{"id":"A135","pred":"fma_id","subj":"T135","obj":"http://purl.org/sig/ont/fma/fma74402"},{"id":"A136","pred":"fma_id","subj":"T136","obj":"http://purl.org/sig/ont/fma/fma68646"},{"id":"A137","pred":"fma_id","subj":"T137","obj":"http://purl.org/sig/ont/fma/fma66768"},{"id":"A138","pred":"fma_id","subj":"T138","obj":"http://purl.org/sig/ont/fma/fma68646"},{"id":"A139","pred":"fma_id","subj":"T139","obj":"http://purl.org/sig/ont/fma/fma62860"},{"id":"A140","pred":"fma_id","subj":"T140","obj":"http://purl.org/sig/ont/fma/fma63261"},{"id":"A141","pred":"fma_id","subj":"T141","obj":"http://purl.org/sig/ont/fma/fma62852"},{"id":"A142","pred":"fma_id","subj":"T142","obj":"http://purl.org/sig/ont/fma/fma66768"},{"id":"A143","pred":"fma_id","subj":"T143","obj":"http://purl.org/sig/ont/fma/fma68646"},{"id":"A144","pred":"fma_id","subj":"T144","obj":"http://purl.org/sig/ont/fma/fma62852"},{"id":"A145","pred":"fma_id","subj":"T145","obj":"http://purl.org/sig/ont/fma/fma66768"},{"id":"A146","pred":"fma_id","subj":"T146","obj":"http://purl.org/sig/ont/fma/fma68646"},{"id":"A147","pred":"fma_id","subj":"T147","obj":"http://purl.org/sig/ont/fma/fma66768"},{"id":"A148","pred":"fma_id","subj":"T148","obj":"http://purl.org/sig/ont/fma/fma68646"},{"id":"A149","pred":"fma_id","subj":"T149","obj":"http://purl.org/sig/ont/fma/fma74402"},{"id":"A150","pred":"fma_id","subj":"T150","obj":"http://purl.org/sig/ont/fma/fma68646"},{"id":"A151","pred":"fma_id","subj":"T151","obj":"http://purl.org/sig/ont/fma/fma67122"},{"id":"A152","pred":"fma_id","subj":"T152","obj":"http://purl.org/sig/ont/fma/fma68646"},{"id":"A153","pred":"fma_id","subj":"T153","obj":"http://purl.org/sig/ont/fma/fma68646"},{"id":"A154","pred":"fma_id","subj":"T154","obj":"http://purl.org/sig/ont/fma/fma68646"},{"id":"A155","pred":"fma_id","subj":"T155","obj":"http://purl.org/sig/ont/fma/fma67122"},{"id":"A156","pred":"fma_id","subj":"T156","obj":"http://purl.org/sig/ont/fma/fma67122"},{"id":"A157","pred":"fma_id","subj":"T157","obj":"http://purl.org/sig/ont/fma/fma68646"},{"id":"A158","pred":"fma_id","subj":"T158","obj":"http://purl.org/sig/ont/fma/fma13444"},{"id":"A159","pred":"fma_id","subj":"T158","obj":"http://purl.org/sig/ont/fma/fma68614"},{"id":"A160","pred":"fma_id","subj":"T160","obj":"http://purl.org/sig/ont/fma/fma74402"},{"id":"A161","pred":"fma_id","subj":"T161","obj":"http://purl.org/sig/ont/fma/fma54736"},{"id":"A162","pred":"fma_id","subj":"T162","obj":"http://purl.org/sig/ont/fma/fma13444"},{"id":"A163","pred":"fma_id","subj":"T162","obj":"http://purl.org/sig/ont/fma/fma68614"},{"id":"A164","pred":"fma_id","subj":"T164","obj":"http://purl.org/sig/ont/fma/fma13444"},{"id":"A165","pred":"fma_id","subj":"T164","obj":"http://purl.org/sig/ont/fma/fma68614"},{"id":"A166","pred":"fma_id","subj":"T166","obj":"http://purl.org/sig/ont/fma/fma68646"},{"id":"A167","pred":"fma_id","subj":"T167","obj":"http://purl.org/sig/ont/fma/fma67122"},{"id":"A168","pred":"fma_id","subj":"T168","obj":"http://purl.org/sig/ont/fma/fma68646"},{"id":"A169","pred":"fma_id","subj":"T169","obj":"http://purl.org/sig/ont/fma/fma7208"},{"id":"A170","pred":"fma_id","subj":"T170","obj":"http://purl.org/sig/ont/fma/fma68646"},{"id":"A171","pred":"fma_id","subj":"T171","obj":"http://purl.org/sig/ont/fma/fma68646"},{"id":"A172","pred":"fma_id","subj":"T172","obj":"http://purl.org/sig/ont/fma/fma68646"},{"id":"A173","pred":"fma_id","subj":"T173","obj":"http://purl.org/sig/ont/fma/fma68646"},{"id":"A174","pred":"fma_id","subj":"T174","obj":"http://purl.org/sig/ont/fma/fma67122"},{"id":"A175","pred":"fma_id","subj":"T175","obj":"http://purl.org/sig/ont/fma/fma68646"},{"id":"A176","pred":"fma_id","subj":"T176","obj":"http://purl.org/sig/ont/fma/fma68646"},{"id":"A177","pred":"fma_id","subj":"T177","obj":"http://purl.org/sig/ont/fma/fma68646"},{"id":"A178","pred":"fma_id","subj":"T178","obj":"http://purl.org/sig/ont/fma/fma68646"},{"id":"A179","pred":"fma_id","subj":"T179","obj":"http://purl.org/sig/ont/fma/fma68646"},{"id":"A180","pred":"fma_id","subj":"T180","obj":"http://purl.org/sig/ont/fma/fma68646"},{"id":"A181","pred":"fma_id","subj":"T181","obj":"http://purl.org/sig/ont/fma/fma9637"},{"id":"A182","pred":"fma_id","subj":"T182","obj":"http://purl.org/sig/ont/fma/fma7208"},{"id":"A183","pred":"fma_id","subj":"T183","obj":"http://purl.org/sig/ont/fma/fma7195"},{"id":"A184","pred":"fma_id","subj":"T184","obj":"http://purl.org/sig/ont/fma/fma68646"},{"id":"A185","pred":"fma_id","subj":"T185","obj":"http://purl.org/sig/ont/fma/fma68646"},{"id":"A186","pred":"fma_id","subj":"T186","obj":"http://purl.org/sig/ont/fma/fma68646"},{"id":"A187","pred":"fma_id","subj":"T187","obj":"http://purl.org/sig/ont/fma/fma68646"},{"id":"A188","pred":"fma_id","subj":"T188","obj":"http://purl.org/sig/ont/fma/fma68646"},{"id":"A189","pred":"fma_id","subj":"T189","obj":"http://purl.org/sig/ont/fma/fma68646"},{"id":"A190","pred":"fma_id","subj":"T190","obj":"http://purl.org/sig/ont/fma/fma68646"},{"id":"A191","pred":"fma_id","subj":"T191","obj":"http://purl.org/sig/ont/fma/fma9637"},{"id":"A192","pred":"fma_id","subj":"T192","obj":"http://purl.org/sig/ont/fma/fma7208"},{"id":"A193","pred":"fma_id","subj":"T193","obj":"http://purl.org/sig/ont/fma/fma68646"},{"id":"A194","pred":"fma_id","subj":"T194","obj":"http://purl.org/sig/ont/fma/fma7195"},{"id":"A195","pred":"fma_id","subj":"T195","obj":"http://purl.org/sig/ont/fma/fma68646"},{"id":"A196","pred":"fma_id","subj":"T196","obj":"http://purl.org/sig/ont/fma/fma68646"},{"id":"A197","pred":"fma_id","subj":"T197","obj":"http://purl.org/sig/ont/fma/fma68646"},{"id":"A198","pred":"fma_id","subj":"T198","obj":"http://purl.org/sig/ont/fma/fma68646"},{"id":"A199","pred":"fma_id","subj":"T199","obj":"http://purl.org/sig/ont/fma/fma68646"},{"id":"A200","pred":"fma_id","subj":"T200","obj":"http://purl.org/sig/ont/fma/fma7208"},{"id":"A201","pred":"fma_id","subj":"T201","obj":"http://purl.org/sig/ont/fma/fma62807"},{"id":"A202","pred":"fma_id","subj":"T202","obj":"http://purl.org/sig/ont/fma/fma68646"},{"id":"A203","pred":"fma_id","subj":"T203","obj":"http://purl.org/sig/ont/fma/fma68646"},{"id":"A204","pred":"fma_id","subj":"T204","obj":"http://purl.org/sig/ont/fma/fma7208"},{"id":"A205","pred":"fma_id","subj":"T205","obj":"http://purl.org/sig/ont/fma/fma7195"},{"id":"A206","pred":"fma_id","subj":"T206","obj":"http://purl.org/sig/ont/fma/fma62807"},{"id":"A207","pred":"fma_id","subj":"T207","obj":"http://purl.org/sig/ont/fma/fma68646"},{"id":"A208","pred":"fma_id","subj":"T208","obj":"http://purl.org/sig/ont/fma/fma68646"},{"id":"A209","pred":"fma_id","subj":"T209","obj":"http://purl.org/sig/ont/fma/fma68646"},{"id":"A210","pred":"fma_id","subj":"T210","obj":"http://purl.org/sig/ont/fma/fma68646"},{"id":"A211","pred":"fma_id","subj":"T211","obj":"http://purl.org/sig/ont/fma/fma9637"},{"id":"A212","pred":"fma_id","subj":"T212","obj":"http://purl.org/sig/ont/fma/fma7195"},{"id":"A213","pred":"fma_id","subj":"T213","obj":"http://purl.org/sig/ont/fma/fma68646"},{"id":"A214","pred":"fma_id","subj":"T214","obj":"http://purl.org/sig/ont/fma/fma7195"},{"id":"A215","pred":"fma_id","subj":"T215","obj":"http://purl.org/sig/ont/fma/fma68646"},{"id":"A216","pred":"fma_id","subj":"T216","obj":"http://purl.org/sig/ont/fma/fma7208"},{"id":"A217","pred":"fma_id","subj":"T217","obj":"http://purl.org/sig/ont/fma/fma68646"},{"id":"A218","pred":"fma_id","subj":"T218","obj":"http://purl.org/sig/ont/fma/fma7208"},{"id":"A219","pred":"fma_id","subj":"T219","obj":"http://purl.org/sig/ont/fma/fma68646"},{"id":"A220","pred":"fma_id","subj":"T220","obj":"http://purl.org/sig/ont/fma/fma68646"},{"id":"A221","pred":"fma_id","subj":"T221","obj":"http://purl.org/sig/ont/fma/fma68646"},{"id":"A222","pred":"fma_id","subj":"T222","obj":"http://purl.org/sig/ont/fma/fma68646"},{"id":"A223","pred":"fma_id","subj":"T223","obj":"http://purl.org/sig/ont/fma/fma68646"},{"id":"A224","pred":"fma_id","subj":"T224","obj":"http://purl.org/sig/ont/fma/fma68646"},{"id":"A225","pred":"fma_id","subj":"T225","obj":"http://purl.org/sig/ont/fma/fma68646"},{"id":"A226","pred":"fma_id","subj":"T226","obj":"http://purl.org/sig/ont/fma/fma62499"},{"id":"A227","pred":"fma_id","subj":"T227","obj":"http://purl.org/sig/ont/fma/fma68646"},{"id":"A228","pred":"fma_id","subj":"T228","obj":"http://purl.org/sig/ont/fma/fma68646"},{"id":"A229","pred":"fma_id","subj":"T229","obj":"http://purl.org/sig/ont/fma/fma68646"},{"id":"A230","pred":"fma_id","subj":"T230","obj":"http://purl.org/sig/ont/fma/fma68646"},{"id":"A231","pred":"fma_id","subj":"T231","obj":"http://purl.org/sig/ont/fma/fma68646"},{"id":"A232","pred":"fma_id","subj":"T232","obj":"http://purl.org/sig/ont/fma/fma68646"},{"id":"A233","pred":"fma_id","subj":"T233","obj":"http://purl.org/sig/ont/fma/fma68646"},{"id":"A234","pred":"fma_id","subj":"T234","obj":"http://purl.org/sig/ont/fma/fma68646"},{"id":"A235","pred":"fma_id","subj":"T235","obj":"http://purl.org/sig/ont/fma/fma68646"},{"id":"A236","pred":"fma_id","subj":"T236","obj":"http://purl.org/sig/ont/fma/fma68646"},{"id":"A237","pred":"fma_id","subj":"T237","obj":"http://purl.org/sig/ont/fma/fma68646"},{"id":"A238","pred":"fma_id","subj":"T238","obj":"http://purl.org/sig/ont/fma/fma74402"}],"text":"Quantification and Statistical Analysis\n\nNon-Human Primate Lung and Ileum\nLibraries corresponding to 7 animals (variable number of tissues per animal) were sequenced using Illumina NextSeq. Reads were aligned to the M. mulatta genome assembly 8.0.1 annotation version 102 and processed according to the Drop-Seq Computational Protocol v2.0 (https://github.com/broadinstitute/Drop-seq). Data was normalized and scaled using the Seurat R package v2.3.4 (https://satijalab.org/seurat/): transforming the data to loge(UMI+1) and applying a scale factor of 10,000. To identify major axes of variation within our data, we first examined only highly variable genes across all cells, yielding approximately 1,000-3,000 variable genes with average expression \u003e 0.1 log-normalized UMI across all cells. An approximate principal component analysis was applied to the cells to generate 100 principal components (PCs). Using the JackStraw function within Seurat, we identified significant PCs to be used for subsequent clustering and further dimensionality reduction. For 2D visualization and cell type clustering, we used a Uniform Manifold Approximation and Projection (UMAP) dimensionality reduction technique (https://github.com/lmcinnes/umap) with “min_dist” set to 0.5 and “n_neighbors” set to 30. To identify clusters of transcriptionally similar cells, we employed unsupervised clustering as described above using the FindClusters tool within the Seurat R package with default parameters and k.param set to 10 and resolution set to 0.5. Each cluster was sub-clustered to identify more granular cell types, requiring each cell type to express \u003e 25 significantly upregulated genes by differential expression test (FindMarkers implemented in Seurat, setting “test.use” to “bimod,” Bonferroni-adjusted p value cutoff \u003c 0.001). Differential expression tests between cells from ACE2 + versus ACE2 - Type II Pneumocytes were conducted using the SCDE R package with default parameters (Kharchenko et al., 2014). Expression data for epithelial cells and enterocytes included in this dataset can be visualized and downloaded here: https://singlecell.broadinstitute.org/single_cell/study/SCP807?scpbr=the-alexandria-project#study-summary.\n\nHuman Lung Tissue\nLibraries corresponding to 8 donors were sequenced using Illumina NextSeq. Reads were aligned to the hg19 genome assembly and processed according to the Drop-Seq Computational Protocol v2.0 (https://github.com/broadinstitute/Drop-seq). Data was normalized and scaled using the Seurat R package v3.1.0 (https://satijalab.org/seurat/), transforming the data to loge(UMI+1) and applying a scale factor of 10,000. For each array, we assessed the quality of constructed libraries by examining the distribution of reads, genes and transcripts per cell. Variable gene selection, principal components analysis, and selection of significant principal components was performed as above. We visualized our results in a two-dimensional space using UMAP (https://github.com/lmcinnes/umap), and annotated each cluster based on the identification of highly expressed genes. To further characterize substructure within cell types (for example, T cells), we performed dimensionality reduction (PCA) and clustering over those cells alone. Sub-clusters (i.e., clusters within broad cell type classifications) were annotated by cross-referencing cluster-defining genes with curated gene lists and online databases SaVanT (http://newpathways.mcdb.ucla.edu/savant-dev/) and GSEA/MsigDB (https://www.gsea-msigdb.org/gsea/msigdb/index.jsp). Proliferating cells from the human lung (Figure 2C) express high levels of mitotic markers, such as MKI67, and represent primarily T cells (CD3D, CD3E), B cells/antibody-secreting cells (IGJ, MZB1, IGHG1), and myeloid cells (CD14, APOE) and represent a composite cell cluster. Differential expression analysis between ACE2+ TMPRSS2+ and negative type II pneumocytes was performed in Seurat using a likelihood-ratio test (FindMarkers implemented in Seurat, setting “test.use” to bimod). Expression data for epithelial cells included in this dataset can be visualized and downloaded here: https://singlecell.broadinstitute.org/single_cell/study/SCP814?scpbr=the-alexandria-project#study-summary.\n\nHuman Ileum\nLibraries corresponding to 13 donors were sequenced using Illumina NovaSeq S2 with a Read 1 26bp, Read 2 91bp, Index 1 8bp configuration before reads were aligned to GRCh38. Each sample was filtered individually for low quality cells and genes by analyzing distributions of reads, transcripts, percent reads mapped to mitochondrial genes, and complexity per cell, then merged as an outer join to create a single dataset. Clustering and differential expression tests were processed using Seurat v3.1.0 (https://satijalab.org/seurat/). Normalization and variable gene selection was processed with SCTransform (https://github.com/ChristophH/sctransform). Clustering for major cell types was performed using Louvain clustering on dimensionally reduced PCA space with resolution set via grid search optimizing for maximum average silhouette score. Due to the scale of the dataset, a randomized subsampling from across the dataset was used to calculate the silhouette score. We annotated clusters based on highly expressed genes, then sub-clusters were characterized by performing PCA dimensionality reduction and clustering over those cells alone, and annotated based on highly expressed genes found via one-versus-rest differential expression test (Wilcoxon) within the major cell type. Differential expression analysis between ACE2 + TMPRSS2 + and negative epithelial cells was performed in Seurat using a Wilcoxon test and Bonferroni p value correction. Expression data for epithelial cells included in this dataset can be visualized and downloaded here: https://singlecell.broadinstitute.org/single_cell/study/SCP812?scpbr=the-alexandria-project#study-summary.\n\nHuman Adult Nasal Mucosa\nSample processing, sequencing, and analysis was performed as in (Ordovas-Montanes et al., 2018). Briefly, scRNA-seq cell suspensions were freshly processed using Seq-Well v1 and Seurat v2.3.4 was utilized for computational analyses presented here (Butler et al., 2018, Satija et al., 2015). Cell by gene matrix and R code for initialization of object available to download as Supplemental Data and Supplementary Tables here https://www.nature.com/articles/s41586-018-0449-8 and here:\nhttp://shaleklab.com/resource/mapping-allergic-inflammation/? and visualized here: https://singlecell.broadinstitute.org/single_cell/study/SCP253?scpbr=the-alexandria-project#study-summary. Scores for various cytokines acting on human airway epithelial cells were calculated based on gene lists derived for (Ordovas-Montanes et al., 2018), calculated using AddModuleScore function Seurat, and effect size calculated by Cohen’s d, as previously reported.\n\nGranulomatous Tissue from Mycobacterium Tuberculosis Infected NHPs\nLibraries corresponding to 10 animals (variable number of tissues/animal) were sequenced using Illumina NovaSeq S2. Data was aligned using the Dropseq-tools pipeline on Terra (app.terra.bio) to M. fascicularis reference genome assembly 5, annotation version 101. Clustering was performed using Leiden clustering in the Scanpy (scanpy.readthedocs.io) package (Wolf et al., 2018). Cell type labels were assigned using known marker genes. In this analysis, we include all epithelial cell subsets (secretory, multiciliated, type II pneumocytes, and type I pneumocytes) from all samples. Differential expression between ACE2 + TMPRSS2 + cells and other cells of the matched cell subtype (e.g., Secretory Cells) were performed using the “bimod” likelihood-ratio test within each cell subtype and filtered on Benjamini-Hochberg-corrected p value \u003c 0.05. Expression data for epithelial cells included in this dataset can be visualized and downloaded here:\nhttps://singlecell.broadinstitute.org/single_cell/study/SCP806?scpbr=the-alexandria-project#study-summary.\n\nBasal Cell Cytokine Stimulation\nLibraries corresponding to 279 populations were sequenced using Illumina NextSeq. Reads were aligned to the hg19 or mm10 genome assembly using the cumulus platform https://cumulus-doc.readthedocs.io/en/0.12.0/smart_seq_2.html and output as TPM using RSEM v1.3.2. Populations were transformed to transcripts per 10K reads and log2(1+TP10K) transformed. ACE2 expression by stimulation condition and dose were assessed using one-way ANOVA with post hoc testing using a Bonferroni correction. Plots were generated using ggplot2, and transcriptome-wide differential expression was calculated using the Seurat R package v3.1.0 (https://satijalab.org/seurat), function FindMarkers with test.use = ”bimod.” Expression data can be visualized and downloaded here:\nhttps://singlecell.broadinstitute.org/single_cell/study/SCP822?scpbr=the-alexandria-project.\n\nInterferon Treatment of Mouse Nasal Mucosa\nLibraries corresponding to 4 mice, with 2 Seq-Well arrays per mouse were sequenced using Illumina NextSeq as described (Gierahn et al., 2017, Hughes et al., 2019). Reads were aligned to the mm10 genome and processed according to the Drop-Seq Computational Protocol v2.0 (https://github.com/broadinstitute/Drop-seq). Data was normalized and scaled using the Seurat R package v2.3.4 (https://satijalab.org/seurat/): transforming the data to loge(UMI+1) and applying a scale factor of 10,000. Cells with fewer than 1000 UMIs and 500 unique genes were removed. To identify major axes of variation within our data, we first examined only highly variable genes across all cells, yielding approximately 5,000 variable genes. An approximate principal component analysis was applied to the cells to generate 200 principal components (PCs). Using a combination of the Jackstraw function in Seurat and observing the “elbow” of the standard deviations of PCs, we chose the top 70 PCs for subsequent clustering and visualization. For 2D visualization, we used a Uniform Manifold Approximation and Projection (UMAP) dimensionality reduction technique (https://github.com/lmcinnes/umap) with “min_dist” set to 0.3 and “n_neighbors” set to 50. To identify clusters of transcriptionally similar cells, we employed unsupervised clustering as described above using the FindClusters tool within the Seurat R package with default parameters and k.param set to 10. Resolution was chosen based on maximization of the average silhouette width across all cells. Clusters were merged if a cell type expressed fewer than 25 significantly upregulated genes by differential expression test (FindAllMarkers implemented in Seurat, setting “test.use” to “bimod,” Bonferroni-adjusted p value cutoff \u003c 0.001). Differential expression tests between cells from saline-treated or IFNa-treated mice were assessed using the FindMarkers function with “test.use” set to “bimod. This dataset can be visualized and downloaded here:\nhttps://singlecell.broadinstitute.org/single_cell/study/SCP832?scpbr=the-alexandria-project#study-summary.\n\nLung from MHV68-Infected WT and IFNγR KO Mice\nLibraries corresponding to 14 mice were aligned to a custom reference genome encompassing both murine (mm10) and herpes virus genes: 84 known genes from MHV68 were retrieved from NCBI (NCBI: txid33708) and added to the mm10 mouse genome. Reads were aligned to the custom joint genome and processed according to the Drop-Seq Computational Protocol v2.0 (https://github.com/broadinstitute/Drop-seq). Barcodes with \u003c 200 unique genes, \u003e 20,000 UMI counts, and \u003e 30% of transcript counts derived from mitochondrially encoded genes were discarded. Data analysis was performed using the Scanpy Package following the common procedure, the expression matrices were normalized using scran’s size factor based approach and log transformed via scanpy’s pp.log1p() function (Lun et al., 2016, Wolf et al., 2018). SoupX was utilized to reduce ambient RNA bias, using default parameters with pCut set to 0.3, and was applied to each sample before merging the count matrices (Young and Behjati, 2020). UMI per cell and cell cycle were regressed out. Highly variable genes were selected by running pp.highly_variable_genes() for each sample separately, returning the top 4,000 variable genes per sample, and genes identified in variable in \u003e 5 samples were retained, yielding 14,305 genes. Next, only Epcam+ cells were considered, principal components (PCs) were calculated using only the selected variable genes, and 6 PCs were used to perform unsupervised Louvain clustering. Type I Pneumocytes were excluded from this analysis based on uniformly negative expression of Ace2, resulting in a final dataset subset of 5,558 cells. Cells were identified as infected if at least one viral read was detected.\n\nNasal Washes during Influenza Infection\nSample processing, sequencing, and analysis was performed as in (Cao et al., 2020). Reads were aligned to the GRCh37 reference genome combined with influenza genomes. Mapped reads from each sample were then corrected for Drop-seq barcode synthesis error using the Drop-seq core computational tools developed by the McCarroll Lab (Macosko et al., 2015). Genes were quantified using End Sequence Analysis Toolkit (ESAT, github/garber-lab/ESAT) with parameters -wlen 100 -wOlap 50 -wExt 0 -scPrep (Derr et al., 2016). Finally, UMIs that likely result from sequencing errors were corrected by merging any UMIs that were observed only once and have 1 hamming distance from a UMI detected by two or more aligned reads. Only cell barcodes with more than 1,000 UMIs were analyzed. Cell barcodes with mostly erythrocyte genes (HBA, HBB) were removed. From here on, the remaining cell barcodes in the matrix would be referred to as cells. The final gene by cell matrix was normalized using the scran package v3.10 (Lun et al., 2016). The normalized matrix was used for dimensionality reduction by first selecting variable genes that had a high coefficient of variance (CV) and were expressed (\u003e = 1 UMI) by more than three cells. Influenza viral genes, interferon stimulated genes, and cell cycle related genes were removed from the variable gene list in order to minimize the impact of viral responses and mitosis on clustering and cell type identification. This resulted in the selection of 2484 variable genes. t-distributed stochastic neighbor embedding (tSNE) was applied to the first ten principal components (PCs), which explained 95% of the total data variance. Density clustering (Rodriguez and Laio, 2014) was performed on the resulting tSNE coordinates and identified four major clusters: epithelial cells, neutrophils, macrophages and leukocytes. The epithelial cell cluster and the leukocyte cluster were then re-clustered independently, as described above, to identify populations within each metacluster. Specifically, the epithelial cell cluster was re-embedded using 2629 variable genes selected by the same criteria mentioned in the previous section and 13 PCs that explained 95% of the variance. Density clustering over the epithelial cell subset revealed ten clusters. Differential gene expression analysis using edgeR (Robinson et al., 2010) was performed to identify marker genes for each cluster. Influenza-infected and bystander cells were identified after correcting for sample-specific distribution of ambient influenza mRNA contamination and predicted cells most likely to be infected identified using a hurdle zero inflated negative binomial (ZINB) model and a support vector machine (SVM) classifier.\n\nPower Calculations for Detection of Rare Transcripts\nWe conducted the following statistical analysis to estimate the effects of various factors on our ability to make confident claims regarding the presence/absence of transcripts of interest (e.g., ACE2), both within individual cells and clusters (Figure S6). Specifically, we investigated the roles of capture/reverse transcription efficiency, ACE2 expression level, sequencing depth, and cell numbers. Taken together, the results of this power analysis are in agreement with other efforts to model biological and technical sources of zero-inflation within scRNA-seq data (e.g., https://satijalab.org/howmanycells and Kharchenko et al., 2014, Svensson, 2020).\nWe began by quantifying how likely we are to capture and transcribe at least one ACE2 mRNA molecule, as a function of the number ACE2 mRNA molecules per cell and a protocol’s efficiency (Figure S6A). Drop-Seq has a capture/transcription efficiency of ∼10% (as estimated using ERCC spike ins; see (Macosko et al., 2015), and the experimental platforms used in this study are either equivalent (e.g., Seq-Well v1, (Gierahn et al., 2017) or superior (e.g., 10-fold better unique molecule detection, 5-fold better gene detection using Seq-Well S3;(Hughes et al., 2019)). Most relevant to this context, inferior turbinate scrapings were processed using both Seq-Well v1 and Seq-Well S3 (Figure S3B). Importantly, Seq-Well S3 provided \u003e two-fold increase in the detection frequency of rare ACE2 transcripts (i.e., ACE2+: 4.7% for v1 versus 9.8% for S3), making it reasonable to expect that such improvements in single-cell experimental technologies have yielded corresponding improvements in capture and transcription efficiency. Based on Drop-Seq’s 10% efficiency, even if ACE2 is expressed at the low level of 5 mRNA molecules per cell (a reasonable order-of-magnitude estimate, given that non-human primate ileum cells had a maximum of 10 ACE2 unique molecules per cell observed via sequencing and an average of 1.93 molecules per cell in expressing cells, see Figures 3B and 3C), our experimental platforms have a minimum likelihood of 41% to capture and reverse transcribe at least one ACE2 mRNA molecule in any given individual cell. This likelihood rapidly increases if we estimate higher efficiencies for improved scRNA-seq technologies (e.g., 67% likelihood within any individual cell at 20% capture/transcription efficiency, 76% likelihood at 25% efficiency, Figure S6A). Thus, while transcript drop-out may reduce the fraction of positive cells, with the capture and transcription efficiencies of improved single-cell technologies, the impact is likely to be minor (reads are likely underestimated by up to a factor of ∼2.5x), given a sufficient depth of sequencing (see below). We note that this impacts both clusters deemed to contain and not contain ACE2+ cells, and suggests our percentages are likely lower bounds for true expression (within a factor of ∼2.5x).\nNext, we examined the probability of sequencing an ACE2 transcript as a function of read depth and ACE2’s fractional abundance in each single cell within our sequencing libraries. First, across two different tissues (non-human primate ileum and lung, representing a high expresser of ACE2 and low expresser, respectively), we calculated the proportion of unique ACE2 molecules in our ACE2+ cells (defined as any cell with at least 1 UMI aligning to ACE2) as a fraction of total reads within individual cells to provide an order-of-magnitude estimate for average ACE2 abundance in our single-cell sequencing libraries (i.e., the probability that a read within a cell corresponds to a unique molecule of ACE2, Figure S6B). We highlight that by calculating probabilities based on ACE2 unique molecules divided by an individual cell’s total reads, we are providing a conservative estimate for the probability of observing ACE2 as a function of sequencing depth (e.g., as compared to basing these probabilities on ACE2 non-UMI-collapsed reads divided by total reads). Next, we obtained information on the number of reads in these cell populations to provide estimates of average sequencing depths (Figure S6C). Using the mean fractional abundances of ACE2 from each tissue (Figure S6B) and the mean read depths for all genes (Figure S6C), we calculated the probability of detecting at least 1 ACE2 molecule (i.e., P(detecting \u003e 0 ACE2 molecules) = 1 - (1 - ACE2 fractional abundance)Read depth). This results in a 93.7% probability in ileum-derived cell libraries that contain ACE2, and a 76.0% probability for lung-derived cell libraries, indicating that our sequencing depths are sufficient to detect ACE2+ cells (Figure S6D).\nTo further evaluate whether our ability to detect ACE2+ cells was an artifact of sequencing depth, we compared the number of ACE2+ cells in a cluster to the mean number of reads across all cells in that same cluster (Figure S6E). We did not observe any significant correlation: the ileum cell cluster with the highest number of ACE2+ cells had the lowest sequencing depth of all ileum clusters, and the lung cell cluster with the highest number of ACE2+ cells was approximately average in its read depth (on a log-log scale, Pearson’s r = −0.31, non-significant). Further, when comparing ACE2+ cells to ACE2- cells within a given tissue, we did not observe a positive correlation between read depth and ACE2 status (i.e., mean ± standard error of the mean, SEM, reads among all lung cells = 28,512 ± 344; mean ± SEM reads among ACE2+ lung cells = 28,553 ± 2,988; mean ± SEM reads among all ileum cells = 14,864 ± 288; mean ± SEM reads among ACE2+ ileum cells = 10,591 ± 441, full statistics on cell depth among ACE2+ cells compared to ACE2- cells of the same cell type can be found in Table S9). Thus, we can be confident that the observed differences in ACE2+ proportions across clusters are not driven by differences in sequencing depth.\nFinally, we investigated how observed differences in ACE2+ proportions across clusters might be affected by cell sampling. Using the proportion of ACE2+ cells in a “typical” cluster annotated as being ACE2 positive (i.e., 6.8% in non-human primate type II pneumocytes, Figure 1), we calculated the cluster sizes needed to be confident that the probability of observing zero to a few positive cells is unlikely to have arisen by random chance (probabilities calculated under a negative binomial distribution with parameter p = 0.068, Figure S6E). We found that as cluster sizes approach and exceed 100 cells, the probability of observing zero to a few positive cells rapidly approaches zero, if we assume 6.8% of cells are positive. Further, to examine our confidence in estimating an approximate upper bound (ignoring the impact of protocol inefficiencies discussed above) for the fraction of cells positive in a cluster as a function of the number of cells in that cluster, we also calculated the probability of observing zero (and its complement, probability of observing at least 1) ACE2+ cells as a function of cluster size across true positive proportions ranging from 0.1% to 10% (probabilities calculated under a negative binomial distribution with parameter p = 0.001 to 0.1, representing hypothetical proportions of ACE2+ cells Figure S6F). Given our typical cluster sizes (on the order of hundreds of cells, exact values provided in Table S9), we find that for us to observe 0 ACE2+ cells in a cluster due to sampling artifacts, the fraction of true positives must be ∼1% or less. Thus, these complementary approaches demonstrate that our observed variations in ACE2+ cell proportions across clusters likely reflect underlying biological differences, rather than random chance.\n\nStatistical Testing\nParameters such as sample size, number of replicates, number of independent experiments, measures of center, dispersion, and precision (mean ± SEM) and statistical significances are reported in Figures and Figure Legends. A p value less than 0.05 was considered significant. Where appropriate, a Bonferroni or FDR correction was used to account for multiple tests, alternative correction methods are noted in the figure legends or Methods. All statistical tests corresponding to differential gene expression are described above and completed using R language for Statistical Computing."}
LitCovid-PD-UBERON
{"project":"LitCovid-PD-UBERON","denotations":[{"id":"T237","span":{"begin":59,"end":63},"obj":"Body_part"},{"id":"T238","span":{"begin":68,"end":73},"obj":"Body_part"},{"id":"T239","span":{"begin":536,"end":541},"obj":"Body_part"},{"id":"T240","span":{"begin":2230,"end":2234},"obj":"Body_part"},{"id":"T241","span":{"begin":2235,"end":2241},"obj":"Body_part"},{"id":"T242","span":{"begin":2628,"end":2633},"obj":"Body_part"},{"id":"T243","span":{"begin":3594,"end":3598},"obj":"Body_part"},{"id":"T244","span":{"begin":3822,"end":3834},"obj":"Body_part"},{"id":"T245","span":{"begin":4260,"end":4265},"obj":"Body_part"},{"id":"T246","span":{"begin":5120,"end":5125},"obj":"Body_part"},{"id":"T247","span":{"begin":5945,"end":5951},"obj":"Body_part"},{"id":"T248","span":{"begin":6905,"end":6911},"obj":"Body_part"},{"id":"T249","span":{"begin":8930,"end":8936},"obj":"Body_part"},{"id":"T250","span":{"begin":9403,"end":9408},"obj":"Body_part"},{"id":"T251","span":{"begin":9843,"end":9848},"obj":"Body_part"},{"id":"T252","span":{"begin":11034,"end":11038},"obj":"Body_part"},{"id":"T253","span":{"begin":14674,"end":14686},"obj":"Body_part"},{"id":"T254","span":{"begin":14849,"end":14861},"obj":"Body_part"},{"id":"T255","span":{"begin":17447,"end":17452},"obj":"Body_part"},{"id":"T256","span":{"begin":18750,"end":18755},"obj":"Body_part"},{"id":"T257","span":{"begin":18760,"end":18764},"obj":"Body_part"},{"id":"T258","span":{"begin":19776,"end":19782},"obj":"Body_part"},{"id":"T259","span":{"begin":20045,"end":20050},"obj":"Body_part"},{"id":"T260","span":{"begin":20121,"end":20125},"obj":"Body_part"},{"id":"T261","span":{"begin":20521,"end":20526},"obj":"Body_part"},{"id":"T262","span":{"begin":20527,"end":20539},"obj":"Body_part"},{"id":"T263","span":{"begin":20618,"end":20623},"obj":"Body_part"},{"id":"T264","span":{"begin":20642,"end":20646},"obj":"Body_part"},{"id":"T265","span":{"begin":20647,"end":20659},"obj":"Body_part"},{"id":"T266","span":{"begin":20757,"end":20762},"obj":"Body_part"},{"id":"T267","span":{"begin":20869,"end":20875},"obj":"Body_part"},{"id":"T268","span":{"begin":21017,"end":21021},"obj":"Body_part"},{"id":"T269","span":{"begin":21073,"end":21077},"obj":"Body_part"},{"id":"T270","span":{"begin":21129,"end":21134},"obj":"Body_part"},{"id":"T271","span":{"begin":21186,"end":21191},"obj":"Body_part"}],"attributes":[{"id":"A237","pred":"uberon_id","subj":"T237","obj":"http://purl.obolibrary.org/obo/UBERON_0002048"},{"id":"A238","pred":"uberon_id","subj":"T238","obj":"http://purl.obolibrary.org/obo/UBERON_0002116"},{"id":"A239","pred":"uberon_id","subj":"T239","obj":"http://purl.obolibrary.org/obo/UBERON_0002542"},{"id":"A240","pred":"uberon_id","subj":"T240","obj":"http://purl.obolibrary.org/obo/UBERON_0002048"},{"id":"A241","pred":"uberon_id","subj":"T241","obj":"http://purl.obolibrary.org/obo/UBERON_0000479"},{"id":"A242","pred":"uberon_id","subj":"T242","obj":"http://purl.obolibrary.org/obo/UBERON_0002542"},{"id":"A243","pred":"uberon_id","subj":"T243","obj":"http://purl.obolibrary.org/obo/UBERON_0002048"},{"id":"A244","pred":"uberon_id","subj":"T244","obj":"http://purl.obolibrary.org/obo/UBERON_0034922"},{"id":"A245","pred":"uberon_id","subj":"T245","obj":"http://purl.obolibrary.org/obo/UBERON_0002116"},{"id":"A246","pred":"uberon_id","subj":"T246","obj":"http://purl.obolibrary.org/obo/UBERON_0002542"},{"id":"A247","pred":"uberon_id","subj":"T247","obj":"http://purl.obolibrary.org/obo/UBERON_0000344"},{"id":"A248","pred":"uberon_id","subj":"T248","obj":"http://purl.obolibrary.org/obo/UBERON_0000479"},{"id":"A249","pred":"uberon_id","subj":"T249","obj":"http://purl.obolibrary.org/obo/UBERON_0000344"},{"id":"A250","pred":"uberon_id","subj":"T250","obj":"http://purl.obolibrary.org/obo/UBERON_0002542"},{"id":"A251","pred":"uberon_id","subj":"T251","obj":"http://purl.obolibrary.org/obo/UBERON_0001461"},{"id":"A252","pred":"uberon_id","subj":"T252","obj":"http://purl.obolibrary.org/obo/UBERON_0002048"},{"id":"A253","pred":"uberon_id","subj":"T253","obj":"http://purl.obolibrary.org/obo/UBERON_0034922"},{"id":"A254","pred":"uberon_id","subj":"T254","obj":"http://purl.obolibrary.org/obo/UBERON_0034922"},{"id":"A255","pred":"uberon_id","subj":"T255","obj":"http://purl.obolibrary.org/obo/UBERON_0002116"},{"id":"A256","pred":"uberon_id","subj":"T256","obj":"http://purl.obolibrary.org/obo/UBERON_0002116"},{"id":"A257","pred":"uberon_id","subj":"T257","obj":"http://purl.obolibrary.org/obo/UBERON_0002048"},{"id":"A258","pred":"uberon_id","subj":"T258","obj":"http://purl.obolibrary.org/obo/UBERON_0000479"},{"id":"A259","pred":"uberon_id","subj":"T259","obj":"http://purl.obolibrary.org/obo/UBERON_0002116"},{"id":"A260","pred":"uberon_id","subj":"T260","obj":"http://purl.obolibrary.org/obo/UBERON_0002048"},{"id":"A261","pred":"uberon_id","subj":"T261","obj":"http://purl.obolibrary.org/obo/UBERON_0002116"},{"id":"A262","pred":"uberon_id","subj":"T262","obj":"http://purl.obolibrary.org/obo/UBERON_0034922"},{"id":"A263","pred":"uberon_id","subj":"T263","obj":"http://purl.obolibrary.org/obo/UBERON_0002116"},{"id":"A264","pred":"uberon_id","subj":"T264","obj":"http://purl.obolibrary.org/obo/UBERON_0002048"},{"id":"A265","pred":"uberon_id","subj":"T265","obj":"http://purl.obolibrary.org/obo/UBERON_0034922"},{"id":"A266","pred":"uberon_id","subj":"T266","obj":"http://purl.obolibrary.org/obo/UBERON_0002542"},{"id":"A267","pred":"uberon_id","subj":"T267","obj":"http://purl.obolibrary.org/obo/UBERON_0000479"},{"id":"A268","pred":"uberon_id","subj":"T268","obj":"http://purl.obolibrary.org/obo/UBERON_0002048"},{"id":"A269","pred":"uberon_id","subj":"T269","obj":"http://purl.obolibrary.org/obo/UBERON_0002048"},{"id":"A270","pred":"uberon_id","subj":"T270","obj":"http://purl.obolibrary.org/obo/UBERON_0002116"},{"id":"A271","pred":"uberon_id","subj":"T271","obj":"http://purl.obolibrary.org/obo/UBERON_0002116"}],"text":"Quantification and Statistical Analysis\n\nNon-Human Primate Lung and Ileum\nLibraries corresponding to 7 animals (variable number of tissues per animal) were sequenced using Illumina NextSeq. Reads were aligned to the M. mulatta genome assembly 8.0.1 annotation version 102 and processed according to the Drop-Seq Computational Protocol v2.0 (https://github.com/broadinstitute/Drop-seq). Data was normalized and scaled using the Seurat R package v2.3.4 (https://satijalab.org/seurat/): transforming the data to loge(UMI+1) and applying a scale factor of 10,000. To identify major axes of variation within our data, we first examined only highly variable genes across all cells, yielding approximately 1,000-3,000 variable genes with average expression \u003e 0.1 log-normalized UMI across all cells. An approximate principal component analysis was applied to the cells to generate 100 principal components (PCs). Using the JackStraw function within Seurat, we identified significant PCs to be used for subsequent clustering and further dimensionality reduction. For 2D visualization and cell type clustering, we used a Uniform Manifold Approximation and Projection (UMAP) dimensionality reduction technique (https://github.com/lmcinnes/umap) with “min_dist” set to 0.5 and “n_neighbors” set to 30. To identify clusters of transcriptionally similar cells, we employed unsupervised clustering as described above using the FindClusters tool within the Seurat R package with default parameters and k.param set to 10 and resolution set to 0.5. Each cluster was sub-clustered to identify more granular cell types, requiring each cell type to express \u003e 25 significantly upregulated genes by differential expression test (FindMarkers implemented in Seurat, setting “test.use” to “bimod,” Bonferroni-adjusted p value cutoff \u003c 0.001). Differential expression tests between cells from ACE2 + versus ACE2 - Type II Pneumocytes were conducted using the SCDE R package with default parameters (Kharchenko et al., 2014). Expression data for epithelial cells and enterocytes included in this dataset can be visualized and downloaded here: https://singlecell.broadinstitute.org/single_cell/study/SCP807?scpbr=the-alexandria-project#study-summary.\n\nHuman Lung Tissue\nLibraries corresponding to 8 donors were sequenced using Illumina NextSeq. Reads were aligned to the hg19 genome assembly and processed according to the Drop-Seq Computational Protocol v2.0 (https://github.com/broadinstitute/Drop-seq). Data was normalized and scaled using the Seurat R package v3.1.0 (https://satijalab.org/seurat/), transforming the data to loge(UMI+1) and applying a scale factor of 10,000. For each array, we assessed the quality of constructed libraries by examining the distribution of reads, genes and transcripts per cell. Variable gene selection, principal components analysis, and selection of significant principal components was performed as above. We visualized our results in a two-dimensional space using UMAP (https://github.com/lmcinnes/umap), and annotated each cluster based on the identification of highly expressed genes. To further characterize substructure within cell types (for example, T cells), we performed dimensionality reduction (PCA) and clustering over those cells alone. Sub-clusters (i.e., clusters within broad cell type classifications) were annotated by cross-referencing cluster-defining genes with curated gene lists and online databases SaVanT (http://newpathways.mcdb.ucla.edu/savant-dev/) and GSEA/MsigDB (https://www.gsea-msigdb.org/gsea/msigdb/index.jsp). Proliferating cells from the human lung (Figure 2C) express high levels of mitotic markers, such as MKI67, and represent primarily T cells (CD3D, CD3E), B cells/antibody-secreting cells (IGJ, MZB1, IGHG1), and myeloid cells (CD14, APOE) and represent a composite cell cluster. Differential expression analysis between ACE2+ TMPRSS2+ and negative type II pneumocytes was performed in Seurat using a likelihood-ratio test (FindMarkers implemented in Seurat, setting “test.use” to bimod). Expression data for epithelial cells included in this dataset can be visualized and downloaded here: https://singlecell.broadinstitute.org/single_cell/study/SCP814?scpbr=the-alexandria-project#study-summary.\n\nHuman Ileum\nLibraries corresponding to 13 donors were sequenced using Illumina NovaSeq S2 with a Read 1 26bp, Read 2 91bp, Index 1 8bp configuration before reads were aligned to GRCh38. Each sample was filtered individually for low quality cells and genes by analyzing distributions of reads, transcripts, percent reads mapped to mitochondrial genes, and complexity per cell, then merged as an outer join to create a single dataset. Clustering and differential expression tests were processed using Seurat v3.1.0 (https://satijalab.org/seurat/). Normalization and variable gene selection was processed with SCTransform (https://github.com/ChristophH/sctransform). Clustering for major cell types was performed using Louvain clustering on dimensionally reduced PCA space with resolution set via grid search optimizing for maximum average silhouette score. Due to the scale of the dataset, a randomized subsampling from across the dataset was used to calculate the silhouette score. We annotated clusters based on highly expressed genes, then sub-clusters were characterized by performing PCA dimensionality reduction and clustering over those cells alone, and annotated based on highly expressed genes found via one-versus-rest differential expression test (Wilcoxon) within the major cell type. Differential expression analysis between ACE2 + TMPRSS2 + and negative epithelial cells was performed in Seurat using a Wilcoxon test and Bonferroni p value correction. Expression data for epithelial cells included in this dataset can be visualized and downloaded here: https://singlecell.broadinstitute.org/single_cell/study/SCP812?scpbr=the-alexandria-project#study-summary.\n\nHuman Adult Nasal Mucosa\nSample processing, sequencing, and analysis was performed as in (Ordovas-Montanes et al., 2018). Briefly, scRNA-seq cell suspensions were freshly processed using Seq-Well v1 and Seurat v2.3.4 was utilized for computational analyses presented here (Butler et al., 2018, Satija et al., 2015). Cell by gene matrix and R code for initialization of object available to download as Supplemental Data and Supplementary Tables here https://www.nature.com/articles/s41586-018-0449-8 and here:\nhttp://shaleklab.com/resource/mapping-allergic-inflammation/? and visualized here: https://singlecell.broadinstitute.org/single_cell/study/SCP253?scpbr=the-alexandria-project#study-summary. Scores for various cytokines acting on human airway epithelial cells were calculated based on gene lists derived for (Ordovas-Montanes et al., 2018), calculated using AddModuleScore function Seurat, and effect size calculated by Cohen’s d, as previously reported.\n\nGranulomatous Tissue from Mycobacterium Tuberculosis Infected NHPs\nLibraries corresponding to 10 animals (variable number of tissues/animal) were sequenced using Illumina NovaSeq S2. Data was aligned using the Dropseq-tools pipeline on Terra (app.terra.bio) to M. fascicularis reference genome assembly 5, annotation version 101. Clustering was performed using Leiden clustering in the Scanpy (scanpy.readthedocs.io) package (Wolf et al., 2018). Cell type labels were assigned using known marker genes. In this analysis, we include all epithelial cell subsets (secretory, multiciliated, type II pneumocytes, and type I pneumocytes) from all samples. Differential expression between ACE2 + TMPRSS2 + cells and other cells of the matched cell subtype (e.g., Secretory Cells) were performed using the “bimod” likelihood-ratio test within each cell subtype and filtered on Benjamini-Hochberg-corrected p value \u003c 0.05. Expression data for epithelial cells included in this dataset can be visualized and downloaded here:\nhttps://singlecell.broadinstitute.org/single_cell/study/SCP806?scpbr=the-alexandria-project#study-summary.\n\nBasal Cell Cytokine Stimulation\nLibraries corresponding to 279 populations were sequenced using Illumina NextSeq. Reads were aligned to the hg19 or mm10 genome assembly using the cumulus platform https://cumulus-doc.readthedocs.io/en/0.12.0/smart_seq_2.html and output as TPM using RSEM v1.3.2. Populations were transformed to transcripts per 10K reads and log2(1+TP10K) transformed. ACE2 expression by stimulation condition and dose were assessed using one-way ANOVA with post hoc testing using a Bonferroni correction. Plots were generated using ggplot2, and transcriptome-wide differential expression was calculated using the Seurat R package v3.1.0 (https://satijalab.org/seurat), function FindMarkers with test.use = ”bimod.” Expression data can be visualized and downloaded here:\nhttps://singlecell.broadinstitute.org/single_cell/study/SCP822?scpbr=the-alexandria-project.\n\nInterferon Treatment of Mouse Nasal Mucosa\nLibraries corresponding to 4 mice, with 2 Seq-Well arrays per mouse were sequenced using Illumina NextSeq as described (Gierahn et al., 2017, Hughes et al., 2019). Reads were aligned to the mm10 genome and processed according to the Drop-Seq Computational Protocol v2.0 (https://github.com/broadinstitute/Drop-seq). Data was normalized and scaled using the Seurat R package v2.3.4 (https://satijalab.org/seurat/): transforming the data to loge(UMI+1) and applying a scale factor of 10,000. Cells with fewer than 1000 UMIs and 500 unique genes were removed. To identify major axes of variation within our data, we first examined only highly variable genes across all cells, yielding approximately 5,000 variable genes. An approximate principal component analysis was applied to the cells to generate 200 principal components (PCs). Using a combination of the Jackstraw function in Seurat and observing the “elbow” of the standard deviations of PCs, we chose the top 70 PCs for subsequent clustering and visualization. For 2D visualization, we used a Uniform Manifold Approximation and Projection (UMAP) dimensionality reduction technique (https://github.com/lmcinnes/umap) with “min_dist” set to 0.3 and “n_neighbors” set to 50. To identify clusters of transcriptionally similar cells, we employed unsupervised clustering as described above using the FindClusters tool within the Seurat R package with default parameters and k.param set to 10. Resolution was chosen based on maximization of the average silhouette width across all cells. Clusters were merged if a cell type expressed fewer than 25 significantly upregulated genes by differential expression test (FindAllMarkers implemented in Seurat, setting “test.use” to “bimod,” Bonferroni-adjusted p value cutoff \u003c 0.001). Differential expression tests between cells from saline-treated or IFNa-treated mice were assessed using the FindMarkers function with “test.use” set to “bimod. This dataset can be visualized and downloaded here:\nhttps://singlecell.broadinstitute.org/single_cell/study/SCP832?scpbr=the-alexandria-project#study-summary.\n\nLung from MHV68-Infected WT and IFNγR KO Mice\nLibraries corresponding to 14 mice were aligned to a custom reference genome encompassing both murine (mm10) and herpes virus genes: 84 known genes from MHV68 were retrieved from NCBI (NCBI: txid33708) and added to the mm10 mouse genome. Reads were aligned to the custom joint genome and processed according to the Drop-Seq Computational Protocol v2.0 (https://github.com/broadinstitute/Drop-seq). Barcodes with \u003c 200 unique genes, \u003e 20,000 UMI counts, and \u003e 30% of transcript counts derived from mitochondrially encoded genes were discarded. Data analysis was performed using the Scanpy Package following the common procedure, the expression matrices were normalized using scran’s size factor based approach and log transformed via scanpy’s pp.log1p() function (Lun et al., 2016, Wolf et al., 2018). SoupX was utilized to reduce ambient RNA bias, using default parameters with pCut set to 0.3, and was applied to each sample before merging the count matrices (Young and Behjati, 2020). UMI per cell and cell cycle were regressed out. Highly variable genes were selected by running pp.highly_variable_genes() for each sample separately, returning the top 4,000 variable genes per sample, and genes identified in variable in \u003e 5 samples were retained, yielding 14,305 genes. Next, only Epcam+ cells were considered, principal components (PCs) were calculated using only the selected variable genes, and 6 PCs were used to perform unsupervised Louvain clustering. Type I Pneumocytes were excluded from this analysis based on uniformly negative expression of Ace2, resulting in a final dataset subset of 5,558 cells. Cells were identified as infected if at least one viral read was detected.\n\nNasal Washes during Influenza Infection\nSample processing, sequencing, and analysis was performed as in (Cao et al., 2020). Reads were aligned to the GRCh37 reference genome combined with influenza genomes. Mapped reads from each sample were then corrected for Drop-seq barcode synthesis error using the Drop-seq core computational tools developed by the McCarroll Lab (Macosko et al., 2015). Genes were quantified using End Sequence Analysis Toolkit (ESAT, github/garber-lab/ESAT) with parameters -wlen 100 -wOlap 50 -wExt 0 -scPrep (Derr et al., 2016). Finally, UMIs that likely result from sequencing errors were corrected by merging any UMIs that were observed only once and have 1 hamming distance from a UMI detected by two or more aligned reads. Only cell barcodes with more than 1,000 UMIs were analyzed. Cell barcodes with mostly erythrocyte genes (HBA, HBB) were removed. From here on, the remaining cell barcodes in the matrix would be referred to as cells. The final gene by cell matrix was normalized using the scran package v3.10 (Lun et al., 2016). The normalized matrix was used for dimensionality reduction by first selecting variable genes that had a high coefficient of variance (CV) and were expressed (\u003e = 1 UMI) by more than three cells. Influenza viral genes, interferon stimulated genes, and cell cycle related genes were removed from the variable gene list in order to minimize the impact of viral responses and mitosis on clustering and cell type identification. This resulted in the selection of 2484 variable genes. t-distributed stochastic neighbor embedding (tSNE) was applied to the first ten principal components (PCs), which explained 95% of the total data variance. Density clustering (Rodriguez and Laio, 2014) was performed on the resulting tSNE coordinates and identified four major clusters: epithelial cells, neutrophils, macrophages and leukocytes. The epithelial cell cluster and the leukocyte cluster were then re-clustered independently, as described above, to identify populations within each metacluster. Specifically, the epithelial cell cluster was re-embedded using 2629 variable genes selected by the same criteria mentioned in the previous section and 13 PCs that explained 95% of the variance. Density clustering over the epithelial cell subset revealed ten clusters. Differential gene expression analysis using edgeR (Robinson et al., 2010) was performed to identify marker genes for each cluster. Influenza-infected and bystander cells were identified after correcting for sample-specific distribution of ambient influenza mRNA contamination and predicted cells most likely to be infected identified using a hurdle zero inflated negative binomial (ZINB) model and a support vector machine (SVM) classifier.\n\nPower Calculations for Detection of Rare Transcripts\nWe conducted the following statistical analysis to estimate the effects of various factors on our ability to make confident claims regarding the presence/absence of transcripts of interest (e.g., ACE2), both within individual cells and clusters (Figure S6). Specifically, we investigated the roles of capture/reverse transcription efficiency, ACE2 expression level, sequencing depth, and cell numbers. Taken together, the results of this power analysis are in agreement with other efforts to model biological and technical sources of zero-inflation within scRNA-seq data (e.g., https://satijalab.org/howmanycells and Kharchenko et al., 2014, Svensson, 2020).\nWe began by quantifying how likely we are to capture and transcribe at least one ACE2 mRNA molecule, as a function of the number ACE2 mRNA molecules per cell and a protocol’s efficiency (Figure S6A). Drop-Seq has a capture/transcription efficiency of ∼10% (as estimated using ERCC spike ins; see (Macosko et al., 2015), and the experimental platforms used in this study are either equivalent (e.g., Seq-Well v1, (Gierahn et al., 2017) or superior (e.g., 10-fold better unique molecule detection, 5-fold better gene detection using Seq-Well S3;(Hughes et al., 2019)). Most relevant to this context, inferior turbinate scrapings were processed using both Seq-Well v1 and Seq-Well S3 (Figure S3B). Importantly, Seq-Well S3 provided \u003e two-fold increase in the detection frequency of rare ACE2 transcripts (i.e., ACE2+: 4.7% for v1 versus 9.8% for S3), making it reasonable to expect that such improvements in single-cell experimental technologies have yielded corresponding improvements in capture and transcription efficiency. Based on Drop-Seq’s 10% efficiency, even if ACE2 is expressed at the low level of 5 mRNA molecules per cell (a reasonable order-of-magnitude estimate, given that non-human primate ileum cells had a maximum of 10 ACE2 unique molecules per cell observed via sequencing and an average of 1.93 molecules per cell in expressing cells, see Figures 3B and 3C), our experimental platforms have a minimum likelihood of 41% to capture and reverse transcribe at least one ACE2 mRNA molecule in any given individual cell. This likelihood rapidly increases if we estimate higher efficiencies for improved scRNA-seq technologies (e.g., 67% likelihood within any individual cell at 20% capture/transcription efficiency, 76% likelihood at 25% efficiency, Figure S6A). Thus, while transcript drop-out may reduce the fraction of positive cells, with the capture and transcription efficiencies of improved single-cell technologies, the impact is likely to be minor (reads are likely underestimated by up to a factor of ∼2.5x), given a sufficient depth of sequencing (see below). We note that this impacts both clusters deemed to contain and not contain ACE2+ cells, and suggests our percentages are likely lower bounds for true expression (within a factor of ∼2.5x).\nNext, we examined the probability of sequencing an ACE2 transcript as a function of read depth and ACE2’s fractional abundance in each single cell within our sequencing libraries. First, across two different tissues (non-human primate ileum and lung, representing a high expresser of ACE2 and low expresser, respectively), we calculated the proportion of unique ACE2 molecules in our ACE2+ cells (defined as any cell with at least 1 UMI aligning to ACE2) as a fraction of total reads within individual cells to provide an order-of-magnitude estimate for average ACE2 abundance in our single-cell sequencing libraries (i.e., the probability that a read within a cell corresponds to a unique molecule of ACE2, Figure S6B). We highlight that by calculating probabilities based on ACE2 unique molecules divided by an individual cell’s total reads, we are providing a conservative estimate for the probability of observing ACE2 as a function of sequencing depth (e.g., as compared to basing these probabilities on ACE2 non-UMI-collapsed reads divided by total reads). Next, we obtained information on the number of reads in these cell populations to provide estimates of average sequencing depths (Figure S6C). Using the mean fractional abundances of ACE2 from each tissue (Figure S6B) and the mean read depths for all genes (Figure S6C), we calculated the probability of detecting at least 1 ACE2 molecule (i.e., P(detecting \u003e 0 ACE2 molecules) = 1 - (1 - ACE2 fractional abundance)Read depth). This results in a 93.7% probability in ileum-derived cell libraries that contain ACE2, and a 76.0% probability for lung-derived cell libraries, indicating that our sequencing depths are sufficient to detect ACE2+ cells (Figure S6D).\nTo further evaluate whether our ability to detect ACE2+ cells was an artifact of sequencing depth, we compared the number of ACE2+ cells in a cluster to the mean number of reads across all cells in that same cluster (Figure S6E). We did not observe any significant correlation: the ileum cell cluster with the highest number of ACE2+ cells had the lowest sequencing depth of all ileum clusters, and the lung cell cluster with the highest number of ACE2+ cells was approximately average in its read depth (on a log-log scale, Pearson’s r = −0.31, non-significant). Further, when comparing ACE2+ cells to ACE2- cells within a given tissue, we did not observe a positive correlation between read depth and ACE2 status (i.e., mean ± standard error of the mean, SEM, reads among all lung cells = 28,512 ± 344; mean ± SEM reads among ACE2+ lung cells = 28,553 ± 2,988; mean ± SEM reads among all ileum cells = 14,864 ± 288; mean ± SEM reads among ACE2+ ileum cells = 10,591 ± 441, full statistics on cell depth among ACE2+ cells compared to ACE2- cells of the same cell type can be found in Table S9). Thus, we can be confident that the observed differences in ACE2+ proportions across clusters are not driven by differences in sequencing depth.\nFinally, we investigated how observed differences in ACE2+ proportions across clusters might be affected by cell sampling. Using the proportion of ACE2+ cells in a “typical” cluster annotated as being ACE2 positive (i.e., 6.8% in non-human primate type II pneumocytes, Figure 1), we calculated the cluster sizes needed to be confident that the probability of observing zero to a few positive cells is unlikely to have arisen by random chance (probabilities calculated under a negative binomial distribution with parameter p = 0.068, Figure S6E). We found that as cluster sizes approach and exceed 100 cells, the probability of observing zero to a few positive cells rapidly approaches zero, if we assume 6.8% of cells are positive. Further, to examine our confidence in estimating an approximate upper bound (ignoring the impact of protocol inefficiencies discussed above) for the fraction of cells positive in a cluster as a function of the number of cells in that cluster, we also calculated the probability of observing zero (and its complement, probability of observing at least 1) ACE2+ cells as a function of cluster size across true positive proportions ranging from 0.1% to 10% (probabilities calculated under a negative binomial distribution with parameter p = 0.001 to 0.1, representing hypothetical proportions of ACE2+ cells Figure S6F). Given our typical cluster sizes (on the order of hundreds of cells, exact values provided in Table S9), we find that for us to observe 0 ACE2+ cells in a cluster due to sampling artifacts, the fraction of true positives must be ∼1% or less. Thus, these complementary approaches demonstrate that our observed variations in ACE2+ cell proportions across clusters likely reflect underlying biological differences, rather than random chance.\n\nStatistical Testing\nParameters such as sample size, number of replicates, number of independent experiments, measures of center, dispersion, and precision (mean ± SEM) and statistical significances are reported in Figures and Figure Legends. A p value less than 0.05 was considered significant. Where appropriate, a Bonferroni or FDR correction was used to account for multiple tests, alternative correction methods are noted in the figure legends or Methods. All statistical tests corresponding to differential gene expression are described above and completed using R language for Statistical Computing."}
LitCovid-PD-MONDO
{"project":"LitCovid-PD-MONDO","denotations":[{"id":"T372","span":{"begin":3699,"end":3703},"obj":"Disease"},{"id":"T373","span":{"begin":6474,"end":6482},"obj":"Disease"},{"id":"T374","span":{"begin":6483,"end":6495},"obj":"Disease"},{"id":"T375","span":{"begin":6931,"end":6943},"obj":"Disease"},{"id":"T376","span":{"begin":12790,"end":12809},"obj":"Disease"},{"id":"T377","span":{"begin":12958,"end":12967},"obj":"Disease"},{"id":"T378","span":{"begin":14030,"end":14039},"obj":"Disease"},{"id":"T379","span":{"begin":15220,"end":15229},"obj":"Disease"},{"id":"T380","span":{"begin":15336,"end":15345},"obj":"Disease"}],"attributes":[{"id":"A372","pred":"mondo_id","subj":"T372","obj":"http://purl.obolibrary.org/obo/MONDO_0014280"},{"id":"A373","pred":"mondo_id","subj":"T373","obj":"http://purl.obolibrary.org/obo/MONDO_0004980"},{"id":"A374","pred":"mondo_id","subj":"T374","obj":"http://purl.obolibrary.org/obo/MONDO_0021166"},{"id":"A375","pred":"mondo_id","subj":"T375","obj":"http://purl.obolibrary.org/obo/MONDO_0018076"},{"id":"A376","pred":"mondo_id","subj":"T376","obj":"http://purl.obolibrary.org/obo/MONDO_0005812"},{"id":"A377","pred":"mondo_id","subj":"T377","obj":"http://purl.obolibrary.org/obo/MONDO_0005812"},{"id":"A378","pred":"mondo_id","subj":"T378","obj":"http://purl.obolibrary.org/obo/MONDO_0005812"},{"id":"A379","pred":"mondo_id","subj":"T379","obj":"http://purl.obolibrary.org/obo/MONDO_0005812"},{"id":"A380","pred":"mondo_id","subj":"T380","obj":"http://purl.obolibrary.org/obo/MONDO_0005812"}],"text":"Quantification and Statistical Analysis\n\nNon-Human Primate Lung and Ileum\nLibraries corresponding to 7 animals (variable number of tissues per animal) were sequenced using Illumina NextSeq. Reads were aligned to the M. mulatta genome assembly 8.0.1 annotation version 102 and processed according to the Drop-Seq Computational Protocol v2.0 (https://github.com/broadinstitute/Drop-seq). Data was normalized and scaled using the Seurat R package v2.3.4 (https://satijalab.org/seurat/): transforming the data to loge(UMI+1) and applying a scale factor of 10,000. To identify major axes of variation within our data, we first examined only highly variable genes across all cells, yielding approximately 1,000-3,000 variable genes with average expression \u003e 0.1 log-normalized UMI across all cells. An approximate principal component analysis was applied to the cells to generate 100 principal components (PCs). Using the JackStraw function within Seurat, we identified significant PCs to be used for subsequent clustering and further dimensionality reduction. For 2D visualization and cell type clustering, we used a Uniform Manifold Approximation and Projection (UMAP) dimensionality reduction technique (https://github.com/lmcinnes/umap) with “min_dist” set to 0.5 and “n_neighbors” set to 30. To identify clusters of transcriptionally similar cells, we employed unsupervised clustering as described above using the FindClusters tool within the Seurat R package with default parameters and k.param set to 10 and resolution set to 0.5. Each cluster was sub-clustered to identify more granular cell types, requiring each cell type to express \u003e 25 significantly upregulated genes by differential expression test (FindMarkers implemented in Seurat, setting “test.use” to “bimod,” Bonferroni-adjusted p value cutoff \u003c 0.001). Differential expression tests between cells from ACE2 + versus ACE2 - Type II Pneumocytes were conducted using the SCDE R package with default parameters (Kharchenko et al., 2014). Expression data for epithelial cells and enterocytes included in this dataset can be visualized and downloaded here: https://singlecell.broadinstitute.org/single_cell/study/SCP807?scpbr=the-alexandria-project#study-summary.\n\nHuman Lung Tissue\nLibraries corresponding to 8 donors were sequenced using Illumina NextSeq. Reads were aligned to the hg19 genome assembly and processed according to the Drop-Seq Computational Protocol v2.0 (https://github.com/broadinstitute/Drop-seq). Data was normalized and scaled using the Seurat R package v3.1.0 (https://satijalab.org/seurat/), transforming the data to loge(UMI+1) and applying a scale factor of 10,000. For each array, we assessed the quality of constructed libraries by examining the distribution of reads, genes and transcripts per cell. Variable gene selection, principal components analysis, and selection of significant principal components was performed as above. We visualized our results in a two-dimensional space using UMAP (https://github.com/lmcinnes/umap), and annotated each cluster based on the identification of highly expressed genes. To further characterize substructure within cell types (for example, T cells), we performed dimensionality reduction (PCA) and clustering over those cells alone. Sub-clusters (i.e., clusters within broad cell type classifications) were annotated by cross-referencing cluster-defining genes with curated gene lists and online databases SaVanT (http://newpathways.mcdb.ucla.edu/savant-dev/) and GSEA/MsigDB (https://www.gsea-msigdb.org/gsea/msigdb/index.jsp). Proliferating cells from the human lung (Figure 2C) express high levels of mitotic markers, such as MKI67, and represent primarily T cells (CD3D, CD3E), B cells/antibody-secreting cells (IGJ, MZB1, IGHG1), and myeloid cells (CD14, APOE) and represent a composite cell cluster. Differential expression analysis between ACE2+ TMPRSS2+ and negative type II pneumocytes was performed in Seurat using a likelihood-ratio test (FindMarkers implemented in Seurat, setting “test.use” to bimod). Expression data for epithelial cells included in this dataset can be visualized and downloaded here: https://singlecell.broadinstitute.org/single_cell/study/SCP814?scpbr=the-alexandria-project#study-summary.\n\nHuman Ileum\nLibraries corresponding to 13 donors were sequenced using Illumina NovaSeq S2 with a Read 1 26bp, Read 2 91bp, Index 1 8bp configuration before reads were aligned to GRCh38. Each sample was filtered individually for low quality cells and genes by analyzing distributions of reads, transcripts, percent reads mapped to mitochondrial genes, and complexity per cell, then merged as an outer join to create a single dataset. Clustering and differential expression tests were processed using Seurat v3.1.0 (https://satijalab.org/seurat/). Normalization and variable gene selection was processed with SCTransform (https://github.com/ChristophH/sctransform). Clustering for major cell types was performed using Louvain clustering on dimensionally reduced PCA space with resolution set via grid search optimizing for maximum average silhouette score. Due to the scale of the dataset, a randomized subsampling from across the dataset was used to calculate the silhouette score. We annotated clusters based on highly expressed genes, then sub-clusters were characterized by performing PCA dimensionality reduction and clustering over those cells alone, and annotated based on highly expressed genes found via one-versus-rest differential expression test (Wilcoxon) within the major cell type. Differential expression analysis between ACE2 + TMPRSS2 + and negative epithelial cells was performed in Seurat using a Wilcoxon test and Bonferroni p value correction. Expression data for epithelial cells included in this dataset can be visualized and downloaded here: https://singlecell.broadinstitute.org/single_cell/study/SCP812?scpbr=the-alexandria-project#study-summary.\n\nHuman Adult Nasal Mucosa\nSample processing, sequencing, and analysis was performed as in (Ordovas-Montanes et al., 2018). Briefly, scRNA-seq cell suspensions were freshly processed using Seq-Well v1 and Seurat v2.3.4 was utilized for computational analyses presented here (Butler et al., 2018, Satija et al., 2015). Cell by gene matrix and R code for initialization of object available to download as Supplemental Data and Supplementary Tables here https://www.nature.com/articles/s41586-018-0449-8 and here:\nhttp://shaleklab.com/resource/mapping-allergic-inflammation/? and visualized here: https://singlecell.broadinstitute.org/single_cell/study/SCP253?scpbr=the-alexandria-project#study-summary. Scores for various cytokines acting on human airway epithelial cells were calculated based on gene lists derived for (Ordovas-Montanes et al., 2018), calculated using AddModuleScore function Seurat, and effect size calculated by Cohen’s d, as previously reported.\n\nGranulomatous Tissue from Mycobacterium Tuberculosis Infected NHPs\nLibraries corresponding to 10 animals (variable number of tissues/animal) were sequenced using Illumina NovaSeq S2. Data was aligned using the Dropseq-tools pipeline on Terra (app.terra.bio) to M. fascicularis reference genome assembly 5, annotation version 101. Clustering was performed using Leiden clustering in the Scanpy (scanpy.readthedocs.io) package (Wolf et al., 2018). Cell type labels were assigned using known marker genes. In this analysis, we include all epithelial cell subsets (secretory, multiciliated, type II pneumocytes, and type I pneumocytes) from all samples. Differential expression between ACE2 + TMPRSS2 + cells and other cells of the matched cell subtype (e.g., Secretory Cells) were performed using the “bimod” likelihood-ratio test within each cell subtype and filtered on Benjamini-Hochberg-corrected p value \u003c 0.05. Expression data for epithelial cells included in this dataset can be visualized and downloaded here:\nhttps://singlecell.broadinstitute.org/single_cell/study/SCP806?scpbr=the-alexandria-project#study-summary.\n\nBasal Cell Cytokine Stimulation\nLibraries corresponding to 279 populations were sequenced using Illumina NextSeq. Reads were aligned to the hg19 or mm10 genome assembly using the cumulus platform https://cumulus-doc.readthedocs.io/en/0.12.0/smart_seq_2.html and output as TPM using RSEM v1.3.2. Populations were transformed to transcripts per 10K reads and log2(1+TP10K) transformed. ACE2 expression by stimulation condition and dose were assessed using one-way ANOVA with post hoc testing using a Bonferroni correction. Plots were generated using ggplot2, and transcriptome-wide differential expression was calculated using the Seurat R package v3.1.0 (https://satijalab.org/seurat), function FindMarkers with test.use = ”bimod.” Expression data can be visualized and downloaded here:\nhttps://singlecell.broadinstitute.org/single_cell/study/SCP822?scpbr=the-alexandria-project.\n\nInterferon Treatment of Mouse Nasal Mucosa\nLibraries corresponding to 4 mice, with 2 Seq-Well arrays per mouse were sequenced using Illumina NextSeq as described (Gierahn et al., 2017, Hughes et al., 2019). Reads were aligned to the mm10 genome and processed according to the Drop-Seq Computational Protocol v2.0 (https://github.com/broadinstitute/Drop-seq). Data was normalized and scaled using the Seurat R package v2.3.4 (https://satijalab.org/seurat/): transforming the data to loge(UMI+1) and applying a scale factor of 10,000. Cells with fewer than 1000 UMIs and 500 unique genes were removed. To identify major axes of variation within our data, we first examined only highly variable genes across all cells, yielding approximately 5,000 variable genes. An approximate principal component analysis was applied to the cells to generate 200 principal components (PCs). Using a combination of the Jackstraw function in Seurat and observing the “elbow” of the standard deviations of PCs, we chose the top 70 PCs for subsequent clustering and visualization. For 2D visualization, we used a Uniform Manifold Approximation and Projection (UMAP) dimensionality reduction technique (https://github.com/lmcinnes/umap) with “min_dist” set to 0.3 and “n_neighbors” set to 50. To identify clusters of transcriptionally similar cells, we employed unsupervised clustering as described above using the FindClusters tool within the Seurat R package with default parameters and k.param set to 10. Resolution was chosen based on maximization of the average silhouette width across all cells. Clusters were merged if a cell type expressed fewer than 25 significantly upregulated genes by differential expression test (FindAllMarkers implemented in Seurat, setting “test.use” to “bimod,” Bonferroni-adjusted p value cutoff \u003c 0.001). Differential expression tests between cells from saline-treated or IFNa-treated mice were assessed using the FindMarkers function with “test.use” set to “bimod. This dataset can be visualized and downloaded here:\nhttps://singlecell.broadinstitute.org/single_cell/study/SCP832?scpbr=the-alexandria-project#study-summary.\n\nLung from MHV68-Infected WT and IFNγR KO Mice\nLibraries corresponding to 14 mice were aligned to a custom reference genome encompassing both murine (mm10) and herpes virus genes: 84 known genes from MHV68 were retrieved from NCBI (NCBI: txid33708) and added to the mm10 mouse genome. Reads were aligned to the custom joint genome and processed according to the Drop-Seq Computational Protocol v2.0 (https://github.com/broadinstitute/Drop-seq). Barcodes with \u003c 200 unique genes, \u003e 20,000 UMI counts, and \u003e 30% of transcript counts derived from mitochondrially encoded genes were discarded. Data analysis was performed using the Scanpy Package following the common procedure, the expression matrices were normalized using scran’s size factor based approach and log transformed via scanpy’s pp.log1p() function (Lun et al., 2016, Wolf et al., 2018). SoupX was utilized to reduce ambient RNA bias, using default parameters with pCut set to 0.3, and was applied to each sample before merging the count matrices (Young and Behjati, 2020). UMI per cell and cell cycle were regressed out. Highly variable genes were selected by running pp.highly_variable_genes() for each sample separately, returning the top 4,000 variable genes per sample, and genes identified in variable in \u003e 5 samples were retained, yielding 14,305 genes. Next, only Epcam+ cells were considered, principal components (PCs) were calculated using only the selected variable genes, and 6 PCs were used to perform unsupervised Louvain clustering. Type I Pneumocytes were excluded from this analysis based on uniformly negative expression of Ace2, resulting in a final dataset subset of 5,558 cells. Cells were identified as infected if at least one viral read was detected.\n\nNasal Washes during Influenza Infection\nSample processing, sequencing, and analysis was performed as in (Cao et al., 2020). Reads were aligned to the GRCh37 reference genome combined with influenza genomes. Mapped reads from each sample were then corrected for Drop-seq barcode synthesis error using the Drop-seq core computational tools developed by the McCarroll Lab (Macosko et al., 2015). Genes were quantified using End Sequence Analysis Toolkit (ESAT, github/garber-lab/ESAT) with parameters -wlen 100 -wOlap 50 -wExt 0 -scPrep (Derr et al., 2016). Finally, UMIs that likely result from sequencing errors were corrected by merging any UMIs that were observed only once and have 1 hamming distance from a UMI detected by two or more aligned reads. Only cell barcodes with more than 1,000 UMIs were analyzed. Cell barcodes with mostly erythrocyte genes (HBA, HBB) were removed. From here on, the remaining cell barcodes in the matrix would be referred to as cells. The final gene by cell matrix was normalized using the scran package v3.10 (Lun et al., 2016). The normalized matrix was used for dimensionality reduction by first selecting variable genes that had a high coefficient of variance (CV) and were expressed (\u003e = 1 UMI) by more than three cells. Influenza viral genes, interferon stimulated genes, and cell cycle related genes were removed from the variable gene list in order to minimize the impact of viral responses and mitosis on clustering and cell type identification. This resulted in the selection of 2484 variable genes. t-distributed stochastic neighbor embedding (tSNE) was applied to the first ten principal components (PCs), which explained 95% of the total data variance. Density clustering (Rodriguez and Laio, 2014) was performed on the resulting tSNE coordinates and identified four major clusters: epithelial cells, neutrophils, macrophages and leukocytes. The epithelial cell cluster and the leukocyte cluster were then re-clustered independently, as described above, to identify populations within each metacluster. Specifically, the epithelial cell cluster was re-embedded using 2629 variable genes selected by the same criteria mentioned in the previous section and 13 PCs that explained 95% of the variance. Density clustering over the epithelial cell subset revealed ten clusters. Differential gene expression analysis using edgeR (Robinson et al., 2010) was performed to identify marker genes for each cluster. Influenza-infected and bystander cells were identified after correcting for sample-specific distribution of ambient influenza mRNA contamination and predicted cells most likely to be infected identified using a hurdle zero inflated negative binomial (ZINB) model and a support vector machine (SVM) classifier.\n\nPower Calculations for Detection of Rare Transcripts\nWe conducted the following statistical analysis to estimate the effects of various factors on our ability to make confident claims regarding the presence/absence of transcripts of interest (e.g., ACE2), both within individual cells and clusters (Figure S6). Specifically, we investigated the roles of capture/reverse transcription efficiency, ACE2 expression level, sequencing depth, and cell numbers. Taken together, the results of this power analysis are in agreement with other efforts to model biological and technical sources of zero-inflation within scRNA-seq data (e.g., https://satijalab.org/howmanycells and Kharchenko et al., 2014, Svensson, 2020).\nWe began by quantifying how likely we are to capture and transcribe at least one ACE2 mRNA molecule, as a function of the number ACE2 mRNA molecules per cell and a protocol’s efficiency (Figure S6A). Drop-Seq has a capture/transcription efficiency of ∼10% (as estimated using ERCC spike ins; see (Macosko et al., 2015), and the experimental platforms used in this study are either equivalent (e.g., Seq-Well v1, (Gierahn et al., 2017) or superior (e.g., 10-fold better unique molecule detection, 5-fold better gene detection using Seq-Well S3;(Hughes et al., 2019)). Most relevant to this context, inferior turbinate scrapings were processed using both Seq-Well v1 and Seq-Well S3 (Figure S3B). Importantly, Seq-Well S3 provided \u003e two-fold increase in the detection frequency of rare ACE2 transcripts (i.e., ACE2+: 4.7% for v1 versus 9.8% for S3), making it reasonable to expect that such improvements in single-cell experimental technologies have yielded corresponding improvements in capture and transcription efficiency. Based on Drop-Seq’s 10% efficiency, even if ACE2 is expressed at the low level of 5 mRNA molecules per cell (a reasonable order-of-magnitude estimate, given that non-human primate ileum cells had a maximum of 10 ACE2 unique molecules per cell observed via sequencing and an average of 1.93 molecules per cell in expressing cells, see Figures 3B and 3C), our experimental platforms have a minimum likelihood of 41% to capture and reverse transcribe at least one ACE2 mRNA molecule in any given individual cell. This likelihood rapidly increases if we estimate higher efficiencies for improved scRNA-seq technologies (e.g., 67% likelihood within any individual cell at 20% capture/transcription efficiency, 76% likelihood at 25% efficiency, Figure S6A). Thus, while transcript drop-out may reduce the fraction of positive cells, with the capture and transcription efficiencies of improved single-cell technologies, the impact is likely to be minor (reads are likely underestimated by up to a factor of ∼2.5x), given a sufficient depth of sequencing (see below). We note that this impacts both clusters deemed to contain and not contain ACE2+ cells, and suggests our percentages are likely lower bounds for true expression (within a factor of ∼2.5x).\nNext, we examined the probability of sequencing an ACE2 transcript as a function of read depth and ACE2’s fractional abundance in each single cell within our sequencing libraries. First, across two different tissues (non-human primate ileum and lung, representing a high expresser of ACE2 and low expresser, respectively), we calculated the proportion of unique ACE2 molecules in our ACE2+ cells (defined as any cell with at least 1 UMI aligning to ACE2) as a fraction of total reads within individual cells to provide an order-of-magnitude estimate for average ACE2 abundance in our single-cell sequencing libraries (i.e., the probability that a read within a cell corresponds to a unique molecule of ACE2, Figure S6B). We highlight that by calculating probabilities based on ACE2 unique molecules divided by an individual cell’s total reads, we are providing a conservative estimate for the probability of observing ACE2 as a function of sequencing depth (e.g., as compared to basing these probabilities on ACE2 non-UMI-collapsed reads divided by total reads). Next, we obtained information on the number of reads in these cell populations to provide estimates of average sequencing depths (Figure S6C). Using the mean fractional abundances of ACE2 from each tissue (Figure S6B) and the mean read depths for all genes (Figure S6C), we calculated the probability of detecting at least 1 ACE2 molecule (i.e., P(detecting \u003e 0 ACE2 molecules) = 1 - (1 - ACE2 fractional abundance)Read depth). This results in a 93.7% probability in ileum-derived cell libraries that contain ACE2, and a 76.0% probability for lung-derived cell libraries, indicating that our sequencing depths are sufficient to detect ACE2+ cells (Figure S6D).\nTo further evaluate whether our ability to detect ACE2+ cells was an artifact of sequencing depth, we compared the number of ACE2+ cells in a cluster to the mean number of reads across all cells in that same cluster (Figure S6E). We did not observe any significant correlation: the ileum cell cluster with the highest number of ACE2+ cells had the lowest sequencing depth of all ileum clusters, and the lung cell cluster with the highest number of ACE2+ cells was approximately average in its read depth (on a log-log scale, Pearson’s r = −0.31, non-significant). Further, when comparing ACE2+ cells to ACE2- cells within a given tissue, we did not observe a positive correlation between read depth and ACE2 status (i.e., mean ± standard error of the mean, SEM, reads among all lung cells = 28,512 ± 344; mean ± SEM reads among ACE2+ lung cells = 28,553 ± 2,988; mean ± SEM reads among all ileum cells = 14,864 ± 288; mean ± SEM reads among ACE2+ ileum cells = 10,591 ± 441, full statistics on cell depth among ACE2+ cells compared to ACE2- cells of the same cell type can be found in Table S9). Thus, we can be confident that the observed differences in ACE2+ proportions across clusters are not driven by differences in sequencing depth.\nFinally, we investigated how observed differences in ACE2+ proportions across clusters might be affected by cell sampling. Using the proportion of ACE2+ cells in a “typical” cluster annotated as being ACE2 positive (i.e., 6.8% in non-human primate type II pneumocytes, Figure 1), we calculated the cluster sizes needed to be confident that the probability of observing zero to a few positive cells is unlikely to have arisen by random chance (probabilities calculated under a negative binomial distribution with parameter p = 0.068, Figure S6E). We found that as cluster sizes approach and exceed 100 cells, the probability of observing zero to a few positive cells rapidly approaches zero, if we assume 6.8% of cells are positive. Further, to examine our confidence in estimating an approximate upper bound (ignoring the impact of protocol inefficiencies discussed above) for the fraction of cells positive in a cluster as a function of the number of cells in that cluster, we also calculated the probability of observing zero (and its complement, probability of observing at least 1) ACE2+ cells as a function of cluster size across true positive proportions ranging from 0.1% to 10% (probabilities calculated under a negative binomial distribution with parameter p = 0.001 to 0.1, representing hypothetical proportions of ACE2+ cells Figure S6F). Given our typical cluster sizes (on the order of hundreds of cells, exact values provided in Table S9), we find that for us to observe 0 ACE2+ cells in a cluster due to sampling artifacts, the fraction of true positives must be ∼1% or less. Thus, these complementary approaches demonstrate that our observed variations in ACE2+ cell proportions across clusters likely reflect underlying biological differences, rather than random chance.\n\nStatistical Testing\nParameters such as sample size, number of replicates, number of independent experiments, measures of center, dispersion, and precision (mean ± SEM) and statistical significances are reported in Figures and Figure Legends. A p value less than 0.05 was considered significant. Where appropriate, a Bonferroni or FDR correction was used to account for multiple tests, alternative correction methods are noted in the figure legends or Methods. All statistical tests corresponding to differential gene expression are described above and completed using R language for Statistical Computing."}
LitCovid-PD-CLO
{"project":"LitCovid-PD-CLO","denotations":[{"id":"T37","span":{"begin":45,"end":50},"obj":"http://purl.obolibrary.org/obo/NCBITaxon_9606"},{"id":"T38","span":{"begin":51,"end":58},"obj":"http://purl.obolibrary.org/obo/NCBITaxon_9443"},{"id":"T39","span":{"begin":59,"end":63},"obj":"http://purl.obolibrary.org/obo/UBERON_0002048"},{"id":"T40","span":{"begin":59,"end":63},"obj":"http://www.ebi.ac.uk/efo/EFO_0000934"},{"id":"T41","span":{"begin":68,"end":73},"obj":"http://purl.obolibrary.org/obo/UBERON_0002116"},{"id":"T42","span":{"begin":103,"end":110},"obj":"http://purl.obolibrary.org/obo/NCBITaxon_33208"},{"id":"T43","span":{"begin":143,"end":149},"obj":"http://purl.obolibrary.org/obo/NCBITaxon_33208"},{"id":"T44","span":{"begin":268,"end":271},"obj":"http://purl.obolibrary.org/obo/CLO_0054060"},{"id":"T45","span":{"begin":534,"end":535},"obj":"http://purl.obolibrary.org/obo/CLO_0001020"},{"id":"T46","span":{"begin":652,"end":657},"obj":"http://purl.obolibrary.org/obo/OGG_0000000002"},{"id":"T47","span":{"begin":669,"end":674},"obj":"http://purl.obolibrary.org/obo/GO_0005623"},{"id":"T48","span":{"begin":720,"end":725},"obj":"http://purl.obolibrary.org/obo/OGG_0000000002"},{"id":"T49","span":{"begin":786,"end":791},"obj":"http://purl.obolibrary.org/obo/GO_0005623"},{"id":"T50","span":{"begin":856,"end":861},"obj":"http://purl.obolibrary.org/obo/GO_0005623"},{"id":"T51","span":{"begin":1080,"end":1089},"obj":"http://purl.obolibrary.org/obo/CL_0000000"},{"id":"T52","span":{"begin":1110,"end":1111},"obj":"http://purl.obolibrary.org/obo/CLO_0001020"},{"id":"T53","span":{"begin":1341,"end":1346},"obj":"http://purl.obolibrary.org/obo/GO_0005623"},{"id":"T54","span":{"begin":1589,"end":1599},"obj":"http://purl.obolibrary.org/obo/CL_0000000"},{"id":"T55","span":{"begin":1616,"end":1625},"obj":"http://purl.obolibrary.org/obo/CL_0000000"},{"id":"T56","span":{"begin":1668,"end":1673},"obj":"http://purl.obolibrary.org/obo/OGG_0000000002"},{"id":"T57","span":{"begin":1701,"end":1705},"obj":"http://purl.obolibrary.org/obo/UBERON_0000473"},{"id":"T58","span":{"begin":1842,"end":1847},"obj":"http://purl.obolibrary.org/obo/UBERON_0000473"},{"id":"T59","span":{"begin":1856,"end":1861},"obj":"http://purl.obolibrary.org/obo/GO_0005623"},{"id":"T60","span":{"begin":2019,"end":2029},"obj":"http://purl.obolibrary.org/obo/CL_0000066"},{"id":"T61","span":{"begin":2030,"end":2035},"obj":"http://purl.obolibrary.org/obo/GO_0005623"},{"id":"T62","span":{"begin":2224,"end":2229},"obj":"http://purl.obolibrary.org/obo/NCBITaxon_9606"},{"id":"T63","span":{"begin":2230,"end":2234},"obj":"http://purl.obolibrary.org/obo/UBERON_0002048"},{"id":"T64","span":{"begin":2230,"end":2234},"obj":"http://www.ebi.ac.uk/efo/EFO_0000934"},{"id":"T65","span":{"begin":2626,"end":2627},"obj":"http://purl.obolibrary.org/obo/CLO_0001020"},{"id":"T66","span":{"begin":2757,"end":2762},"obj":"http://purl.obolibrary.org/obo/OGG_0000000002"},{"id":"T67","span":{"begin":2779,"end":2787},"obj":"http://purl.obolibrary.org/obo/CLO_0008190"},{"id":"T68","span":{"begin":2798,"end":2802},"obj":"http://purl.obolibrary.org/obo/OGG_0000000002"},{"id":"T69","span":{"begin":2948,"end":2949},"obj":"http://purl.obolibrary.org/obo/CLO_0001020"},{"id":"T70","span":{"begin":3094,"end":3099},"obj":"http://purl.obolibrary.org/obo/OGG_0000000002"},{"id":"T71","span":{"begin":3145,"end":3155},"obj":"http://purl.obolibrary.org/obo/CL_0000000"},{"id":"T72","span":{"begin":3170,"end":3177},"obj":"http://purl.obolibrary.org/obo/CL_0000084"},{"id":"T73","span":{"begin":3250,"end":3255},"obj":"http://purl.obolibrary.org/obo/GO_0005623"},{"id":"T74","span":{"begin":3305,"end":3314},"obj":"http://purl.obolibrary.org/obo/CL_0000000"},{"id":"T75","span":{"begin":3385,"end":3390},"obj":"http://purl.obolibrary.org/obo/OGG_0000000002"},{"id":"T76","span":{"begin":3404,"end":3408},"obj":"http://purl.obolibrary.org/obo/OGG_0000000002"},{"id":"T77","span":{"begin":3573,"end":3578},"obj":"http://purl.obolibrary.org/obo/GO_0005623"},{"id":"T78","span":{"begin":3588,"end":3593},"obj":"http://purl.obolibrary.org/obo/NCBITaxon_9606"},{"id":"T79","span":{"begin":3594,"end":3598},"obj":"http://purl.obolibrary.org/obo/UBERON_0002048"},{"id":"T80","span":{"begin":3594,"end":3598},"obj":"http://www.ebi.ac.uk/efo/EFO_0000934"},{"id":"T81","span":{"begin":3690,"end":3697},"obj":"http://purl.obolibrary.org/obo/CL_0000084"},{"id":"T82","span":{"begin":3712,"end":3719},"obj":"http://purl.obolibrary.org/obo/CL_0000236"},{"id":"T83","span":{"begin":3739,"end":3744},"obj":"http://purl.obolibrary.org/obo/GO_0005623"},{"id":"T84","span":{"begin":3777,"end":3782},"obj":"http://purl.obolibrary.org/obo/GO_0005623"},{"id":"T85","span":{"begin":3810,"end":3811},"obj":"http://purl.obolibrary.org/obo/CLO_0001020"},{"id":"T86","span":{"begin":3822,"end":3834},"obj":"http://purl.obolibrary.org/obo/UBERON_0034922"},{"id":"T87","span":{"begin":3955,"end":3956},"obj":"http://purl.obolibrary.org/obo/CLO_0001020"},{"id":"T88","span":{"begin":3974,"end":3978},"obj":"http://purl.obolibrary.org/obo/UBERON_0000473"},{"id":"T89","span":{"begin":4065,"end":4075},"obj":"http://purl.obolibrary.org/obo/CL_0000066"},{"id":"T90","span":{"begin":4076,"end":4081},"obj":"http://purl.obolibrary.org/obo/GO_0005623"},{"id":"T91","span":{"begin":4254,"end":4259},"obj":"http://purl.obolibrary.org/obo/NCBITaxon_9606"},{"id":"T92","span":{"begin":4260,"end":4265},"obj":"http://purl.obolibrary.org/obo/UBERON_0002116"},{"id":"T93","span":{"begin":4341,"end":4343},"obj":"http://purl.obolibrary.org/obo/CLO_0008922"},{"id":"T94","span":{"begin":4341,"end":4343},"obj":"http://purl.obolibrary.org/obo/CLO_0050052"},{"id":"T95","span":{"begin":4349,"end":4350},"obj":"http://purl.obolibrary.org/obo/CLO_0001020"},{"id":"T96","span":{"begin":4494,"end":4499},"obj":"http://purl.obolibrary.org/obo/GO_0005623"},{"id":"T97","span":{"begin":4504,"end":4509},"obj":"http://purl.obolibrary.org/obo/OGG_0000000002"},{"id":"T98","span":{"begin":4598,"end":4603},"obj":"http://purl.obolibrary.org/obo/OGG_0000000002"},{"id":"T99","span":{"begin":4620,"end":4628},"obj":"http://purl.obolibrary.org/obo/CLO_0008190"},{"id":"T100","span":{"begin":4669,"end":4670},"obj":"http://purl.obolibrary.org/obo/CLO_0001020"},{"id":"T101","span":{"begin":4726,"end":4731},"obj":"http://purl.obolibrary.org/obo/UBERON_0000473"},{"id":"T102","span":{"begin":4827,"end":4831},"obj":"http://purl.obolibrary.org/obo/OGG_0000000002"},{"id":"T103","span":{"begin":4939,"end":4949},"obj":"http://purl.obolibrary.org/obo/CL_0000000"},{"id":"T104","span":{"begin":5142,"end":5143},"obj":"http://purl.obolibrary.org/obo/CLO_0001020"},{"id":"T105","span":{"begin":5283,"end":5288},"obj":"http://purl.obolibrary.org/obo/OGG_0000000002"},{"id":"T106","span":{"begin":5396,"end":5401},"obj":"http://purl.obolibrary.org/obo/GO_0005623"},{"id":"T107","span":{"begin":5449,"end":5454},"obj":"http://purl.obolibrary.org/obo/OGG_0000000002"},{"id":"T108","span":{"begin":5505,"end":5509},"obj":"http://purl.obolibrary.org/obo/UBERON_0000473"},{"id":"T109","span":{"begin":5538,"end":5547},"obj":"http://purl.obolibrary.org/obo/CL_0000000"},{"id":"T110","span":{"begin":5620,"end":5630},"obj":"http://purl.obolibrary.org/obo/CL_0000066"},{"id":"T111","span":{"begin":5631,"end":5636},"obj":"http://purl.obolibrary.org/obo/GO_0005623"},{"id":"T112","span":{"begin":5667,"end":5668},"obj":"http://purl.obolibrary.org/obo/CLO_0001020"},{"id":"T113","span":{"begin":5678,"end":5682},"obj":"http://purl.obolibrary.org/obo/UBERON_0000473"},{"id":"T114","span":{"begin":5738,"end":5748},"obj":"http://purl.obolibrary.org/obo/CL_0000066"},{"id":"T115","span":{"begin":5749,"end":5754},"obj":"http://purl.obolibrary.org/obo/GO_0005623"},{"id":"T116","span":{"begin":5927,"end":5932},"obj":"http://purl.obolibrary.org/obo/NCBITaxon_9606"},{"id":"T117","span":{"begin":5945,"end":5951},"obj":"http://purl.obolibrary.org/obo/UBERON_0000344"},{"id":"T118","span":{"begin":6042,"end":6046},"obj":"http://purl.obolibrary.org/obo/CLO_0001185"},{"id":"T119","span":{"begin":6068,"end":6072},"obj":"http://purl.obolibrary.org/obo/GO_0005623"},{"id":"T120","span":{"begin":6215,"end":6219},"obj":"http://purl.obolibrary.org/obo/CLO_0001185"},{"id":"T121","span":{"begin":6243,"end":6247},"obj":"http://purl.obolibrary.org/obo/GO_0005623"},{"id":"T122","span":{"begin":6251,"end":6255},"obj":"http://purl.obolibrary.org/obo/OGG_0000000002"},{"id":"T123","span":{"begin":6296,"end":6302},"obj":"http://purl.obolibrary.org/obo/BFO_0000030"},{"id":"T124","span":{"begin":6665,"end":6670},"obj":"http://purl.obolibrary.org/obo/NCBITaxon_9606"},{"id":"T125","span":{"begin":6671,"end":6677},"obj":"http://purl.obolibrary.org/obo/UBERON_0001005"},{"id":"T126","span":{"begin":6678,"end":6688},"obj":"http://purl.obolibrary.org/obo/CL_0000066"},{"id":"T127","span":{"begin":6689,"end":6694},"obj":"http://purl.obolibrary.org/obo/GO_0005623"},{"id":"T128","span":{"begin":6720,"end":6724},"obj":"http://purl.obolibrary.org/obo/OGG_0000000002"},{"id":"T129","span":{"begin":6769,"end":6773},"obj":"http://purl.obolibrary.org/obo/CLO_0001185"},{"id":"T130","span":{"begin":6988,"end":6995},"obj":"http://purl.obolibrary.org/obo/NCBITaxon_33208"},{"id":"T131","span":{"begin":7024,"end":7030},"obj":"http://purl.obolibrary.org/obo/NCBITaxon_33208"},{"id":"T132","span":{"begin":7070,"end":7072},"obj":"http://purl.obolibrary.org/obo/CLO_0008922"},{"id":"T133","span":{"begin":7070,"end":7072},"obj":"http://purl.obolibrary.org/obo/CLO_0050052"},{"id":"T134","span":{"begin":7330,"end":7334},"obj":"http://purl.obolibrary.org/obo/CLO_0001185"},{"id":"T135","span":{"begin":7337,"end":7346},"obj":"http://purl.obolibrary.org/obo/CL_0000000"},{"id":"T136","span":{"begin":7347,"end":7353},"obj":"http://purl.obolibrary.org/obo/CLO_0007225"},{"id":"T137","span":{"begin":7387,"end":7392},"obj":"http://purl.obolibrary.org/obo/OGG_0000000002"},{"id":"T138","span":{"begin":7427,"end":7437},"obj":"http://purl.obolibrary.org/obo/CL_0000066"},{"id":"T139","span":{"begin":7438,"end":7442},"obj":"http://purl.obolibrary.org/obo/GO_0005623"},{"id":"T140","span":{"begin":7590,"end":7595},"obj":"http://purl.obolibrary.org/obo/GO_0005623"},{"id":"T141","span":{"begin":7606,"end":7611},"obj":"http://purl.obolibrary.org/obo/GO_0005623"},{"id":"T142","span":{"begin":7627,"end":7631},"obj":"http://purl.obolibrary.org/obo/GO_0005623"},{"id":"T143","span":{"begin":7657,"end":7662},"obj":"http://purl.obolibrary.org/obo/GO_0005623"},{"id":"T144","span":{"begin":7714,"end":7718},"obj":"http://purl.obolibrary.org/obo/UBERON_0000473"},{"id":"T145","span":{"begin":7731,"end":7735},"obj":"http://purl.obolibrary.org/obo/GO_0005623"},{"id":"T146","span":{"begin":7825,"end":7835},"obj":"http://purl.obolibrary.org/obo/CL_0000066"},{"id":"T147","span":{"begin":7836,"end":7841},"obj":"http://purl.obolibrary.org/obo/GO_0005623"},{"id":"T148","span":{"begin":8014,"end":8024},"obj":"http://purl.obolibrary.org/obo/CL_0000646"},{"id":"T149","span":{"begin":8245,"end":8247},"obj":"http://purl.obolibrary.org/obo/CLO_0037161"},{"id":"T150","span":{"begin":8496,"end":8503},"obj":"http://purl.obolibrary.org/obo/UBERON_0000473"},{"id":"T151","span":{"begin":8510,"end":8511},"obj":"http://purl.obolibrary.org/obo/CLO_0001020"},{"id":"T152","span":{"begin":8918,"end":8923},"obj":"http://purl.obolibrary.org/obo/CLO_0007836"},{"id":"T153","span":{"begin":8930,"end":8936},"obj":"http://purl.obolibrary.org/obo/UBERON_0000344"},{"id":"T154","span":{"begin":8999,"end":9004},"obj":"http://purl.obolibrary.org/obo/CLO_0007836"},{"id":"T155","span":{"begin":9401,"end":9402},"obj":"http://purl.obolibrary.org/obo/CLO_0001020"},{"id":"T156","span":{"begin":9427,"end":9432},"obj":"http://purl.obolibrary.org/obo/GO_0005623"},{"id":"T157","span":{"begin":9474,"end":9479},"obj":"http://purl.obolibrary.org/obo/OGG_0000000002"},{"id":"T158","span":{"begin":9586,"end":9591},"obj":"http://purl.obolibrary.org/obo/OGG_0000000002"},{"id":"T159","span":{"begin":9603,"end":9608},"obj":"http://purl.obolibrary.org/obo/GO_0005623"},{"id":"T160","span":{"begin":9648,"end":9653},"obj":"http://purl.obolibrary.org/obo/OGG_0000000002"},{"id":"T161","span":{"begin":9718,"end":9723},"obj":"http://purl.obolibrary.org/obo/GO_0005623"},{"id":"T162","span":{"begin":9774,"end":9775},"obj":"http://purl.obolibrary.org/obo/CLO_0001020"},{"id":"T163","span":{"begin":9984,"end":9985},"obj":"http://purl.obolibrary.org/obo/CLO_0001020"},{"id":"T164","span":{"begin":10215,"end":10220},"obj":"http://purl.obolibrary.org/obo/GO_0005623"},{"id":"T165","span":{"begin":10467,"end":10472},"obj":"http://purl.obolibrary.org/obo/GO_0005623"},{"id":"T166","span":{"begin":10498,"end":10499},"obj":"http://purl.obolibrary.org/obo/CLO_0001020"},{"id":"T167","span":{"begin":10500,"end":10509},"obj":"http://purl.obolibrary.org/obo/CL_0000000"},{"id":"T168","span":{"begin":10560,"end":10565},"obj":"http://purl.obolibrary.org/obo/OGG_0000000002"},{"id":"T169","span":{"begin":10593,"end":10597},"obj":"http://purl.obolibrary.org/obo/UBERON_0000473"},{"id":"T170","span":{"begin":10737,"end":10742},"obj":"http://purl.obolibrary.org/obo/UBERON_0000473"},{"id":"T171","span":{"begin":10751,"end":10756},"obj":"http://purl.obolibrary.org/obo/GO_0005623"},{"id":"T172","span":{"begin":11034,"end":11038},"obj":"http://purl.obolibrary.org/obo/UBERON_0002048"},{"id":"T173","span":{"begin":11034,"end":11038},"obj":"http://www.ebi.ac.uk/efo/EFO_0000934"},{"id":"T174","span":{"begin":11070,"end":11074},"obj":"http://purl.obolibrary.org/obo/CLO_0008825"},{"id":"T175","span":{"begin":11131,"end":11132},"obj":"http://purl.obolibrary.org/obo/CLO_0001020"},{"id":"T176","span":{"begin":11200,"end":11205},"obj":"http://purl.obolibrary.org/obo/NCBITaxon_10239"},{"id":"T177","span":{"begin":11206,"end":11211},"obj":"http://purl.obolibrary.org/obo/OGG_0000000002"},{"id":"T178","span":{"begin":11222,"end":11227},"obj":"http://purl.obolibrary.org/obo/OGG_0000000002"},{"id":"T179","span":{"begin":11304,"end":11309},"obj":"http://purl.obolibrary.org/obo/CLO_0007836"},{"id":"T180","span":{"begin":11351,"end":11356},"obj":"http://purl.obolibrary.org/obo/UBERON_0000982"},{"id":"T181","span":{"begin":11351,"end":11356},"obj":"http://purl.obolibrary.org/obo/UBERON_0004905"},{"id":"T182","span":{"begin":11505,"end":11510},"obj":"http://purl.obolibrary.org/obo/OGG_0000000002"},{"id":"T183","span":{"begin":11601,"end":11606},"obj":"http://purl.obolibrary.org/obo/OGG_0000000002"},{"id":"T184","span":{"begin":11874,"end":11878},"obj":"http://purl.obolibrary.org/obo/CLO_0001185"},{"id":"T185","span":{"begin":12071,"end":12079},"obj":"http://purl.obolibrary.org/obo/CLO_0008190"},{"id":"T186","span":{"begin":12084,"end":12088},"obj":"http://purl.obolibrary.org/obo/GO_0005623"},{"id":"T187","span":{"begin":12131,"end":12136},"obj":"http://purl.obolibrary.org/obo/OGG_0000000002"},{"id":"T188","span":{"begin":12250,"end":12255},"obj":"http://purl.obolibrary.org/obo/OGG_0000000002"},{"id":"T189","span":{"begin":12272,"end":12277},"obj":"http://purl.obolibrary.org/obo/OGG_0000000002"},{"id":"T190","span":{"begin":12347,"end":12352},"obj":"http://purl.obolibrary.org/obo/OGG_0000000002"},{"id":"T191","span":{"begin":12372,"end":12377},"obj":"http://purl.obolibrary.org/obo/GO_0005623"},{"id":"T192","span":{"begin":12471,"end":12476},"obj":"http://purl.obolibrary.org/obo/OGG_0000000002"},{"id":"T193","span":{"begin":12655,"end":12656},"obj":"http://purl.obolibrary.org/obo/CLO_0001020"},{"id":"T194","span":{"begin":12687,"end":12692},"obj":"http://purl.obolibrary.org/obo/GO_0005623"},{"id":"T195","span":{"begin":12694,"end":12699},"obj":"http://purl.obolibrary.org/obo/GO_0005623"},{"id":"T196","span":{"begin":13163,"end":13168},"obj":"http://purl.obolibrary.org/obo/OGG_0000000002"},{"id":"T197","span":{"begin":13478,"end":13479},"obj":"http://purl.obolibrary.org/obo/CLO_0001020"},{"id":"T198","span":{"begin":13528,"end":13532},"obj":"http://purl.obolibrary.org/obo/GO_0005623"},{"id":"T199","span":{"begin":13583,"end":13587},"obj":"http://purl.obolibrary.org/obo/GO_0005623"},{"id":"T200","span":{"begin":13609,"end":13620},"obj":"http://purl.obolibrary.org/obo/CL_0000232"},{"id":"T201","span":{"begin":13621,"end":13626},"obj":"http://purl.obolibrary.org/obo/OGG_0000000002"},{"id":"T202","span":{"begin":13680,"end":13684},"obj":"http://purl.obolibrary.org/obo/GO_0005623"},{"id":"T203","span":{"begin":13732,"end":13737},"obj":"http://purl.obolibrary.org/obo/GO_0005623"},{"id":"T204","span":{"begin":13749,"end":13753},"obj":"http://purl.obolibrary.org/obo/OGG_0000000002"},{"id":"T205","span":{"begin":13757,"end":13761},"obj":"http://purl.obolibrary.org/obo/GO_0005623"},{"id":"T206","span":{"begin":13922,"end":13927},"obj":"http://purl.obolibrary.org/obo/OGG_0000000002"},{"id":"T207","span":{"begin":13937,"end":13938},"obj":"http://purl.obolibrary.org/obo/CLO_0001020"},{"id":"T208","span":{"begin":14023,"end":14028},"obj":"http://purl.obolibrary.org/obo/GO_0005623"},{"id":"T209","span":{"begin":14046,"end":14051},"obj":"http://purl.obolibrary.org/obo/OGG_0000000002"},{"id":"T210","span":{"begin":14075,"end":14080},"obj":"http://purl.obolibrary.org/obo/OGG_0000000002"},{"id":"T211","span":{"begin":14086,"end":14090},"obj":"http://purl.obolibrary.org/obo/GO_0005623"},{"id":"T212","span":{"begin":14105,"end":14110},"obj":"http://purl.obolibrary.org/obo/OGG_0000000002"},{"id":"T213","span":{"begin":14142,"end":14146},"obj":"http://purl.obolibrary.org/obo/OGG_0000000002"},{"id":"T214","span":{"begin":14233,"end":14242},"obj":"http://purl.obolibrary.org/obo/CL_0000000"},{"id":"T215","span":{"begin":14307,"end":14312},"obj":"http://purl.obolibrary.org/obo/OGG_0000000002"},{"id":"T216","span":{"begin":14390,"end":14393},"obj":"http://purl.obolibrary.org/obo/CLO_0050884"},{"id":"T217","span":{"begin":14600,"end":14610},"obj":"http://purl.obolibrary.org/obo/CL_0000066"},{"id":"T218","span":{"begin":14611,"end":14616},"obj":"http://purl.obolibrary.org/obo/GO_0005623"},{"id":"T219","span":{"begin":14663,"end":14673},"obj":"http://purl.obolibrary.org/obo/CL_0000066"},{"id":"T220","span":{"begin":14674,"end":14686},"obj":"http://purl.obolibrary.org/obo/UBERON_0034922"},{"id":"T221","span":{"begin":14838,"end":14848},"obj":"http://purl.obolibrary.org/obo/CL_0000066"},{"id":"T222","span":{"begin":14849,"end":14861},"obj":"http://purl.obolibrary.org/obo/UBERON_0034922"},{"id":"T223","span":{"begin":14898,"end":14903},"obj":"http://purl.obolibrary.org/obo/OGG_0000000002"},{"id":"T224","span":{"begin":15043,"end":15053},"obj":"http://purl.obolibrary.org/obo/CL_0000066"},{"id":"T225","span":{"begin":15054,"end":15058},"obj":"http://purl.obolibrary.org/obo/GO_0005623"},{"id":"T226","span":{"begin":15075,"end":15078},"obj":"http://purl.obolibrary.org/obo/CLO_0050884"},{"id":"T227","span":{"begin":15102,"end":15106},"obj":"http://purl.obolibrary.org/obo/OGG_0000000002"},{"id":"T228","span":{"begin":15196,"end":15201},"obj":"http://purl.obolibrary.org/obo/OGG_0000000002"},{"id":"T229","span":{"begin":15253,"end":15258},"obj":"http://purl.obolibrary.org/obo/GO_0005623"},{"id":"T230","span":{"begin":15379,"end":15384},"obj":"http://purl.obolibrary.org/obo/GO_0005623"},{"id":"T231","span":{"begin":15429,"end":15430},"obj":"http://purl.obolibrary.org/obo/CLO_0001020"},{"id":"T232","span":{"begin":15487,"end":15488},"obj":"http://purl.obolibrary.org/obo/CLO_0001020"},{"id":"T233","span":{"begin":15810,"end":15828},"obj":"http://purl.obolibrary.org/obo/UBERON_0034922"},{"id":"T234","span":{"begin":15972,"end":15976},"obj":"http://purl.obolibrary.org/obo/GO_0005623"},{"id":"T235","span":{"begin":16347,"end":16348},"obj":"http://purl.obolibrary.org/obo/CLO_0001020"},{"id":"T236","span":{"begin":16392,"end":16400},"obj":"http://purl.obolibrary.org/obo/CLO_0008190"},{"id":"T237","span":{"begin":16405,"end":16406},"obj":"http://purl.obolibrary.org/obo/CLO_0001020"},{"id":"T238","span":{"begin":16452,"end":16455},"obj":"http://purl.obolibrary.org/obo/CLO_0051582"},{"id":"T239","span":{"begin":16456,"end":16457},"obj":"http://purl.obolibrary.org/obo/CLO_0001020"},{"id":"T240","span":{"begin":16753,"end":16757},"obj":"http://purl.obolibrary.org/obo/OGG_0000000002"},{"id":"T241","span":{"begin":17155,"end":17159},"obj":"http://purl.obolibrary.org/obo/GO_0005623"},{"id":"T242","span":{"begin":17366,"end":17374},"obj":"http://purl.obolibrary.org/obo/CLO_0008190"},{"id":"T243","span":{"begin":17376,"end":17377},"obj":"http://purl.obolibrary.org/obo/CLO_0001020"},{"id":"T244","span":{"begin":17433,"end":17438},"obj":"http://purl.obolibrary.org/obo/NCBITaxon_9606"},{"id":"T245","span":{"begin":17439,"end":17446},"obj":"http://purl.obolibrary.org/obo/NCBITaxon_9443"},{"id":"T246","span":{"begin":17447,"end":17452},"obj":"http://purl.obolibrary.org/obo/UBERON_0002116"},{"id":"T247","span":{"begin":17453,"end":17458},"obj":"http://purl.obolibrary.org/obo/GO_0005623"},{"id":"T248","span":{"begin":17463,"end":17464},"obj":"http://purl.obolibrary.org/obo/CLO_0001020"},{"id":"T249","span":{"begin":17501,"end":17509},"obj":"http://purl.obolibrary.org/obo/CLO_0008190"},{"id":"T250","span":{"begin":17567,"end":17575},"obj":"http://purl.obolibrary.org/obo/CLO_0008190"},{"id":"T251","span":{"begin":17590,"end":17595},"obj":"http://purl.obolibrary.org/obo/GO_0005623"},{"id":"T252","span":{"begin":17653,"end":17654},"obj":"http://purl.obolibrary.org/obo/CLO_0001020"},{"id":"T253","span":{"begin":17677,"end":17679},"obj":"http://purl.obolibrary.org/obo/CLO_0053794"},{"id":"T254","span":{"begin":17771,"end":17775},"obj":"http://purl.obolibrary.org/obo/GO_0005623"},{"id":"T255","span":{"begin":17926,"end":17930},"obj":"http://purl.obolibrary.org/obo/GO_0005623"},{"id":"T256","span":{"begin":18087,"end":18092},"obj":"http://purl.obolibrary.org/obo/GO_0005623"},{"id":"T257","span":{"begin":18161,"end":18165},"obj":"http://purl.obolibrary.org/obo/GO_0005623"},{"id":"T258","span":{"begin":18255,"end":18256},"obj":"http://purl.obolibrary.org/obo/CLO_0001020"},{"id":"T259","span":{"begin":18281,"end":18282},"obj":"http://purl.obolibrary.org/obo/CLO_0001020"},{"id":"T260","span":{"begin":18407,"end":18412},"obj":"http://purl.obolibrary.org/obo/GO_0005623"},{"id":"T261","span":{"begin":18495,"end":18496},"obj":"http://purl.obolibrary.org/obo/CLO_0001020"},{"id":"T262","span":{"begin":18585,"end":18586},"obj":"http://purl.obolibrary.org/obo/CLO_0001020"},{"id":"T263","span":{"begin":18657,"end":18661},"obj":"http://purl.obolibrary.org/obo/GO_0005623"},{"id":"T264","span":{"begin":18736,"end":18741},"obj":"http://purl.obolibrary.org/obo/NCBITaxon_9606"},{"id":"T265","span":{"begin":18742,"end":18749},"obj":"http://purl.obolibrary.org/obo/NCBITaxon_9443"},{"id":"T266","span":{"begin":18750,"end":18755},"obj":"http://purl.obolibrary.org/obo/UBERON_0002116"},{"id":"T267","span":{"begin":18760,"end":18764},"obj":"http://purl.obolibrary.org/obo/UBERON_0002048"},{"id":"T268","span":{"begin":18760,"end":18764},"obj":"http://www.ebi.ac.uk/efo/EFO_0000934"},{"id":"T269","span":{"begin":18779,"end":18780},"obj":"http://purl.obolibrary.org/obo/CLO_0001020"},{"id":"T270","span":{"begin":18905,"end":18910},"obj":"http://purl.obolibrary.org/obo/GO_0005623"},{"id":"T271","span":{"begin":18927,"end":18931},"obj":"http://purl.obolibrary.org/obo/GO_0005623"},{"id":"T272","span":{"begin":18973,"end":18974},"obj":"http://purl.obolibrary.org/obo/CLO_0001020"},{"id":"T273","span":{"begin":19017,"end":19022},"obj":"http://purl.obolibrary.org/obo/GO_0005623"},{"id":"T274","span":{"begin":19106,"end":19110},"obj":"http://purl.obolibrary.org/obo/GO_0005623"},{"id":"T275","span":{"begin":19160,"end":19161},"obj":"http://purl.obolibrary.org/obo/CLO_0001020"},{"id":"T276","span":{"begin":19174,"end":19180},"obj":"http://purl.obolibrary.org/obo/CLO_0001020"},{"id":"T277","span":{"begin":19196,"end":19197},"obj":"http://purl.obolibrary.org/obo/CLO_0001020"},{"id":"T278","span":{"begin":19339,"end":19345},"obj":"http://purl.obolibrary.org/obo/GO_0005623"},{"id":"T279","span":{"begin":19376,"end":19377},"obj":"http://purl.obolibrary.org/obo/CLO_0001020"},{"id":"T280","span":{"begin":19441,"end":19442},"obj":"http://purl.obolibrary.org/obo/CLO_0001020"},{"id":"T281","span":{"begin":19640,"end":19644},"obj":"http://purl.obolibrary.org/obo/GO_0005623"},{"id":"T282","span":{"begin":19829,"end":19834},"obj":"http://purl.obolibrary.org/obo/OGG_0000000002"},{"id":"T283","span":{"begin":20022,"end":20023},"obj":"http://purl.obolibrary.org/obo/CLO_0001020"},{"id":"T284","span":{"begin":20045,"end":20050},"obj":"http://purl.obolibrary.org/obo/UBERON_0002116"},{"id":"T285","span":{"begin":20059,"end":20063},"obj":"http://purl.obolibrary.org/obo/GO_0005623"},{"id":"T286","span":{"begin":20097,"end":20098},"obj":"http://purl.obolibrary.org/obo/CLO_0001020"},{"id":"T287","span":{"begin":20121,"end":20125},"obj":"http://purl.obolibrary.org/obo/UBERON_0002048"},{"id":"T288","span":{"begin":20121,"end":20125},"obj":"http://www.ebi.ac.uk/efo/EFO_0000934"},{"id":"T289","span":{"begin":20134,"end":20138},"obj":"http://purl.obolibrary.org/obo/GO_0005623"},{"id":"T290","span":{"begin":20219,"end":20224},"obj":"http://purl.obolibrary.org/obo/GO_0005623"},{"id":"T291","span":{"begin":20295,"end":20300},"obj":"http://purl.obolibrary.org/obo/GO_0005623"},{"id":"T292","span":{"begin":20370,"end":20375},"obj":"http://purl.obolibrary.org/obo/GO_0005623"},{"id":"T293","span":{"begin":20379,"end":20380},"obj":"http://purl.obolibrary.org/obo/CLO_0001020"},{"id":"T294","span":{"begin":20428,"end":20433},"obj":"http://purl.obolibrary.org/obo/GO_0005623"},{"id":"T295","span":{"begin":20521,"end":20526},"obj":"http://purl.obolibrary.org/obo/UBERON_0002116"},{"id":"T296","span":{"begin":20527,"end":20539},"obj":"http://purl.obolibrary.org/obo/UBERON_0034922"},{"id":"T297","span":{"begin":20573,"end":20578},"obj":"http://purl.obolibrary.org/obo/GO_0005623"},{"id":"T298","span":{"begin":20618,"end":20623},"obj":"http://purl.obolibrary.org/obo/UBERON_0002116"},{"id":"T299","span":{"begin":20642,"end":20646},"obj":"http://purl.obolibrary.org/obo/UBERON_0002048"},{"id":"T300","span":{"begin":20642,"end":20646},"obj":"http://www.ebi.ac.uk/efo/EFO_0000934"},{"id":"T301","span":{"begin":20647,"end":20659},"obj":"http://purl.obolibrary.org/obo/UBERON_0034922"},{"id":"T302","span":{"begin":20693,"end":20698},"obj":"http://purl.obolibrary.org/obo/GO_0005623"},{"id":"T303","span":{"begin":20747,"end":20748},"obj":"http://purl.obolibrary.org/obo/CLO_0001020"},{"id":"T304","span":{"begin":20833,"end":20838},"obj":"http://purl.obolibrary.org/obo/GO_0005623"},{"id":"T305","span":{"begin":20848,"end":20853},"obj":"http://purl.obolibrary.org/obo/GO_0005623"},{"id":"T306","span":{"begin":20861,"end":20862},"obj":"http://purl.obolibrary.org/obo/CLO_0001020"},{"id":"T307","span":{"begin":20896,"end":20897},"obj":"http://purl.obolibrary.org/obo/CLO_0001020"},{"id":"T308","span":{"begin":21017,"end":21021},"obj":"http://purl.obolibrary.org/obo/UBERON_0002048"},{"id":"T309","span":{"begin":21017,"end":21021},"obj":"http://www.ebi.ac.uk/efo/EFO_0000934"},{"id":"T310","span":{"begin":21022,"end":21027},"obj":"http://purl.obolibrary.org/obo/GO_0005623"},{"id":"T311","span":{"begin":21073,"end":21077},"obj":"http://purl.obolibrary.org/obo/UBERON_0002048"},{"id":"T312","span":{"begin":21073,"end":21077},"obj":"http://www.ebi.ac.uk/efo/EFO_0000934"},{"id":"T313","span":{"begin":21078,"end":21083},"obj":"http://purl.obolibrary.org/obo/GO_0005623"},{"id":"T314","span":{"begin":21129,"end":21134},"obj":"http://purl.obolibrary.org/obo/UBERON_0002116"},{"id":"T315","span":{"begin":21135,"end":21140},"obj":"http://purl.obolibrary.org/obo/GO_0005623"},{"id":"T316","span":{"begin":21186,"end":21191},"obj":"http://purl.obolibrary.org/obo/UBERON_0002116"},{"id":"T317","span":{"begin":21192,"end":21197},"obj":"http://purl.obolibrary.org/obo/GO_0005623"},{"id":"T318","span":{"begin":21233,"end":21237},"obj":"http://purl.obolibrary.org/obo/GO_0005623"},{"id":"T319","span":{"begin":21256,"end":21261},"obj":"http://purl.obolibrary.org/obo/GO_0005623"},{"id":"T320","span":{"begin":21280,"end":21285},"obj":"http://purl.obolibrary.org/obo/GO_0005623"},{"id":"T321","span":{"begin":21298,"end":21307},"obj":"http://purl.obolibrary.org/obo/CL_0000000"},{"id":"T322","span":{"begin":21330,"end":21332},"obj":"http://purl.obolibrary.org/obo/CLO_0008935"},{"id":"T323","span":{"begin":21587,"end":21591},"obj":"http://purl.obolibrary.org/obo/GO_0005623"},{"id":"T324","span":{"begin":21632,"end":21637},"obj":"http://purl.obolibrary.org/obo/GO_0005623"},{"id":"T325","span":{"begin":21641,"end":21642},"obj":"http://purl.obolibrary.org/obo/CLO_0001020"},{"id":"T326","span":{"begin":21713,"end":21718},"obj":"http://purl.obolibrary.org/obo/NCBITaxon_9606"},{"id":"T327","span":{"begin":21719,"end":21726},"obj":"http://purl.obolibrary.org/obo/NCBITaxon_9443"},{"id":"T328","span":{"begin":21856,"end":21857},"obj":"http://purl.obolibrary.org/obo/CLO_0001020"},{"id":"T329","span":{"begin":21871,"end":21876},"obj":"http://purl.obolibrary.org/obo/GO_0005623"},{"id":"T330","span":{"begin":21953,"end":21954},"obj":"http://purl.obolibrary.org/obo/CLO_0001020"},{"id":"T331","span":{"begin":22080,"end":22085},"obj":"http://purl.obolibrary.org/obo/GO_0005623"},{"id":"T332","span":{"begin":22124,"end":22125},"obj":"http://purl.obolibrary.org/obo/CLO_0001020"},{"id":"T333","span":{"begin":22139,"end":22144},"obj":"http://purl.obolibrary.org/obo/GO_0005623"},{"id":"T334","span":{"begin":22191,"end":22196},"obj":"http://purl.obolibrary.org/obo/GO_0005623"},{"id":"T335","span":{"begin":22372,"end":22377},"obj":"http://purl.obolibrary.org/obo/GO_0005623"},{"id":"T336","span":{"begin":22390,"end":22391},"obj":"http://purl.obolibrary.org/obo/CLO_0001020"},{"id":"T337","span":{"begin":22403,"end":22404},"obj":"http://purl.obolibrary.org/obo/CLO_0001020"},{"id":"T338","span":{"begin":22431,"end":22452},"obj":"http://purl.obolibrary.org/obo/UBERON_0034922"},{"id":"T339","span":{"begin":22571,"end":22576},"obj":"http://purl.obolibrary.org/obo/GO_0005623"},{"id":"T340","span":{"begin":22580,"end":22581},"obj":"http://purl.obolibrary.org/obo/CLO_0001020"},{"id":"T341","span":{"begin":22697,"end":22698},"obj":"http://purl.obolibrary.org/obo/CLO_0001020"},{"id":"T342","span":{"begin":22810,"end":22815},"obj":"http://purl.obolibrary.org/obo/GO_0005623"},{"id":"T343","span":{"begin":22890,"end":22895},"obj":"http://purl.obolibrary.org/obo/GO_0005623"},{"id":"T344","span":{"begin":22928,"end":22930},"obj":"http://purl.obolibrary.org/obo/CLO_0008935"},{"id":"T345","span":{"begin":22972,"end":22977},"obj":"http://purl.obolibrary.org/obo/GO_0005623"},{"id":"T346","span":{"begin":22981,"end":22982},"obj":"http://purl.obolibrary.org/obo/CLO_0001020"},{"id":"T347","span":{"begin":23157,"end":23161},"obj":"http://purl.obolibrary.org/obo/GO_0005623"},{"id":"T348","span":{"begin":23280,"end":23287},"obj":"http://purl.obolibrary.org/obo/UBERON_0000473"},{"id":"T349","span":{"begin":23510,"end":23513},"obj":"http://purl.obolibrary.org/obo/CLO_0001547"},{"id":"T350","span":{"begin":23582,"end":23583},"obj":"http://purl.obolibrary.org/obo/CLO_0001020"},{"id":"T351","span":{"begin":23646,"end":23651},"obj":"http://purl.obolibrary.org/obo/UBERON_0000473"},{"id":"T352","span":{"begin":23744,"end":23749},"obj":"http://purl.obolibrary.org/obo/UBERON_0000473"},{"id":"T353","span":{"begin":23780,"end":23784},"obj":"http://purl.obolibrary.org/obo/OGG_0000000002"}],"text":"Quantification and Statistical Analysis\n\nNon-Human Primate Lung and Ileum\nLibraries corresponding to 7 animals (variable number of tissues per animal) were sequenced using Illumina NextSeq. Reads were aligned to the M. mulatta genome assembly 8.0.1 annotation version 102 and processed according to the Drop-Seq Computational Protocol v2.0 (https://github.com/broadinstitute/Drop-seq). Data was normalized and scaled using the Seurat R package v2.3.4 (https://satijalab.org/seurat/): transforming the data to loge(UMI+1) and applying a scale factor of 10,000. To identify major axes of variation within our data, we first examined only highly variable genes across all cells, yielding approximately 1,000-3,000 variable genes with average expression \u003e 0.1 log-normalized UMI across all cells. An approximate principal component analysis was applied to the cells to generate 100 principal components (PCs). Using the JackStraw function within Seurat, we identified significant PCs to be used for subsequent clustering and further dimensionality reduction. For 2D visualization and cell type clustering, we used a Uniform Manifold Approximation and Projection (UMAP) dimensionality reduction technique (https://github.com/lmcinnes/umap) with “min_dist” set to 0.5 and “n_neighbors” set to 30. To identify clusters of transcriptionally similar cells, we employed unsupervised clustering as described above using the FindClusters tool within the Seurat R package with default parameters and k.param set to 10 and resolution set to 0.5. Each cluster was sub-clustered to identify more granular cell types, requiring each cell type to express \u003e 25 significantly upregulated genes by differential expression test (FindMarkers implemented in Seurat, setting “test.use” to “bimod,” Bonferroni-adjusted p value cutoff \u003c 0.001). Differential expression tests between cells from ACE2 + versus ACE2 - Type II Pneumocytes were conducted using the SCDE R package with default parameters (Kharchenko et al., 2014). Expression data for epithelial cells and enterocytes included in this dataset can be visualized and downloaded here: https://singlecell.broadinstitute.org/single_cell/study/SCP807?scpbr=the-alexandria-project#study-summary.\n\nHuman Lung Tissue\nLibraries corresponding to 8 donors were sequenced using Illumina NextSeq. Reads were aligned to the hg19 genome assembly and processed according to the Drop-Seq Computational Protocol v2.0 (https://github.com/broadinstitute/Drop-seq). Data was normalized and scaled using the Seurat R package v3.1.0 (https://satijalab.org/seurat/), transforming the data to loge(UMI+1) and applying a scale factor of 10,000. For each array, we assessed the quality of constructed libraries by examining the distribution of reads, genes and transcripts per cell. Variable gene selection, principal components analysis, and selection of significant principal components was performed as above. We visualized our results in a two-dimensional space using UMAP (https://github.com/lmcinnes/umap), and annotated each cluster based on the identification of highly expressed genes. To further characterize substructure within cell types (for example, T cells), we performed dimensionality reduction (PCA) and clustering over those cells alone. Sub-clusters (i.e., clusters within broad cell type classifications) were annotated by cross-referencing cluster-defining genes with curated gene lists and online databases SaVanT (http://newpathways.mcdb.ucla.edu/savant-dev/) and GSEA/MsigDB (https://www.gsea-msigdb.org/gsea/msigdb/index.jsp). Proliferating cells from the human lung (Figure 2C) express high levels of mitotic markers, such as MKI67, and represent primarily T cells (CD3D, CD3E), B cells/antibody-secreting cells (IGJ, MZB1, IGHG1), and myeloid cells (CD14, APOE) and represent a composite cell cluster. Differential expression analysis between ACE2+ TMPRSS2+ and negative type II pneumocytes was performed in Seurat using a likelihood-ratio test (FindMarkers implemented in Seurat, setting “test.use” to bimod). Expression data for epithelial cells included in this dataset can be visualized and downloaded here: https://singlecell.broadinstitute.org/single_cell/study/SCP814?scpbr=the-alexandria-project#study-summary.\n\nHuman Ileum\nLibraries corresponding to 13 donors were sequenced using Illumina NovaSeq S2 with a Read 1 26bp, Read 2 91bp, Index 1 8bp configuration before reads were aligned to GRCh38. Each sample was filtered individually for low quality cells and genes by analyzing distributions of reads, transcripts, percent reads mapped to mitochondrial genes, and complexity per cell, then merged as an outer join to create a single dataset. Clustering and differential expression tests were processed using Seurat v3.1.0 (https://satijalab.org/seurat/). Normalization and variable gene selection was processed with SCTransform (https://github.com/ChristophH/sctransform). Clustering for major cell types was performed using Louvain clustering on dimensionally reduced PCA space with resolution set via grid search optimizing for maximum average silhouette score. Due to the scale of the dataset, a randomized subsampling from across the dataset was used to calculate the silhouette score. We annotated clusters based on highly expressed genes, then sub-clusters were characterized by performing PCA dimensionality reduction and clustering over those cells alone, and annotated based on highly expressed genes found via one-versus-rest differential expression test (Wilcoxon) within the major cell type. Differential expression analysis between ACE2 + TMPRSS2 + and negative epithelial cells was performed in Seurat using a Wilcoxon test and Bonferroni p value correction. Expression data for epithelial cells included in this dataset can be visualized and downloaded here: https://singlecell.broadinstitute.org/single_cell/study/SCP812?scpbr=the-alexandria-project#study-summary.\n\nHuman Adult Nasal Mucosa\nSample processing, sequencing, and analysis was performed as in (Ordovas-Montanes et al., 2018). Briefly, scRNA-seq cell suspensions were freshly processed using Seq-Well v1 and Seurat v2.3.4 was utilized for computational analyses presented here (Butler et al., 2018, Satija et al., 2015). Cell by gene matrix and R code for initialization of object available to download as Supplemental Data and Supplementary Tables here https://www.nature.com/articles/s41586-018-0449-8 and here:\nhttp://shaleklab.com/resource/mapping-allergic-inflammation/? and visualized here: https://singlecell.broadinstitute.org/single_cell/study/SCP253?scpbr=the-alexandria-project#study-summary. Scores for various cytokines acting on human airway epithelial cells were calculated based on gene lists derived for (Ordovas-Montanes et al., 2018), calculated using AddModuleScore function Seurat, and effect size calculated by Cohen’s d, as previously reported.\n\nGranulomatous Tissue from Mycobacterium Tuberculosis Infected NHPs\nLibraries corresponding to 10 animals (variable number of tissues/animal) were sequenced using Illumina NovaSeq S2. Data was aligned using the Dropseq-tools pipeline on Terra (app.terra.bio) to M. fascicularis reference genome assembly 5, annotation version 101. Clustering was performed using Leiden clustering in the Scanpy (scanpy.readthedocs.io) package (Wolf et al., 2018). Cell type labels were assigned using known marker genes. In this analysis, we include all epithelial cell subsets (secretory, multiciliated, type II pneumocytes, and type I pneumocytes) from all samples. Differential expression between ACE2 + TMPRSS2 + cells and other cells of the matched cell subtype (e.g., Secretory Cells) were performed using the “bimod” likelihood-ratio test within each cell subtype and filtered on Benjamini-Hochberg-corrected p value \u003c 0.05. Expression data for epithelial cells included in this dataset can be visualized and downloaded here:\nhttps://singlecell.broadinstitute.org/single_cell/study/SCP806?scpbr=the-alexandria-project#study-summary.\n\nBasal Cell Cytokine Stimulation\nLibraries corresponding to 279 populations were sequenced using Illumina NextSeq. Reads were aligned to the hg19 or mm10 genome assembly using the cumulus platform https://cumulus-doc.readthedocs.io/en/0.12.0/smart_seq_2.html and output as TPM using RSEM v1.3.2. Populations were transformed to transcripts per 10K reads and log2(1+TP10K) transformed. ACE2 expression by stimulation condition and dose were assessed using one-way ANOVA with post hoc testing using a Bonferroni correction. Plots were generated using ggplot2, and transcriptome-wide differential expression was calculated using the Seurat R package v3.1.0 (https://satijalab.org/seurat), function FindMarkers with test.use = ”bimod.” Expression data can be visualized and downloaded here:\nhttps://singlecell.broadinstitute.org/single_cell/study/SCP822?scpbr=the-alexandria-project.\n\nInterferon Treatment of Mouse Nasal Mucosa\nLibraries corresponding to 4 mice, with 2 Seq-Well arrays per mouse were sequenced using Illumina NextSeq as described (Gierahn et al., 2017, Hughes et al., 2019). Reads were aligned to the mm10 genome and processed according to the Drop-Seq Computational Protocol v2.0 (https://github.com/broadinstitute/Drop-seq). Data was normalized and scaled using the Seurat R package v2.3.4 (https://satijalab.org/seurat/): transforming the data to loge(UMI+1) and applying a scale factor of 10,000. Cells with fewer than 1000 UMIs and 500 unique genes were removed. To identify major axes of variation within our data, we first examined only highly variable genes across all cells, yielding approximately 5,000 variable genes. An approximate principal component analysis was applied to the cells to generate 200 principal components (PCs). Using a combination of the Jackstraw function in Seurat and observing the “elbow” of the standard deviations of PCs, we chose the top 70 PCs for subsequent clustering and visualization. For 2D visualization, we used a Uniform Manifold Approximation and Projection (UMAP) dimensionality reduction technique (https://github.com/lmcinnes/umap) with “min_dist” set to 0.3 and “n_neighbors” set to 50. To identify clusters of transcriptionally similar cells, we employed unsupervised clustering as described above using the FindClusters tool within the Seurat R package with default parameters and k.param set to 10. Resolution was chosen based on maximization of the average silhouette width across all cells. Clusters were merged if a cell type expressed fewer than 25 significantly upregulated genes by differential expression test (FindAllMarkers implemented in Seurat, setting “test.use” to “bimod,” Bonferroni-adjusted p value cutoff \u003c 0.001). Differential expression tests between cells from saline-treated or IFNa-treated mice were assessed using the FindMarkers function with “test.use” set to “bimod. This dataset can be visualized and downloaded here:\nhttps://singlecell.broadinstitute.org/single_cell/study/SCP832?scpbr=the-alexandria-project#study-summary.\n\nLung from MHV68-Infected WT and IFNγR KO Mice\nLibraries corresponding to 14 mice were aligned to a custom reference genome encompassing both murine (mm10) and herpes virus genes: 84 known genes from MHV68 were retrieved from NCBI (NCBI: txid33708) and added to the mm10 mouse genome. Reads were aligned to the custom joint genome and processed according to the Drop-Seq Computational Protocol v2.0 (https://github.com/broadinstitute/Drop-seq). Barcodes with \u003c 200 unique genes, \u003e 20,000 UMI counts, and \u003e 30% of transcript counts derived from mitochondrially encoded genes were discarded. Data analysis was performed using the Scanpy Package following the common procedure, the expression matrices were normalized using scran’s size factor based approach and log transformed via scanpy’s pp.log1p() function (Lun et al., 2016, Wolf et al., 2018). SoupX was utilized to reduce ambient RNA bias, using default parameters with pCut set to 0.3, and was applied to each sample before merging the count matrices (Young and Behjati, 2020). UMI per cell and cell cycle were regressed out. Highly variable genes were selected by running pp.highly_variable_genes() for each sample separately, returning the top 4,000 variable genes per sample, and genes identified in variable in \u003e 5 samples were retained, yielding 14,305 genes. Next, only Epcam+ cells were considered, principal components (PCs) were calculated using only the selected variable genes, and 6 PCs were used to perform unsupervised Louvain clustering. Type I Pneumocytes were excluded from this analysis based on uniformly negative expression of Ace2, resulting in a final dataset subset of 5,558 cells. Cells were identified as infected if at least one viral read was detected.\n\nNasal Washes during Influenza Infection\nSample processing, sequencing, and analysis was performed as in (Cao et al., 2020). Reads were aligned to the GRCh37 reference genome combined with influenza genomes. Mapped reads from each sample were then corrected for Drop-seq barcode synthesis error using the Drop-seq core computational tools developed by the McCarroll Lab (Macosko et al., 2015). Genes were quantified using End Sequence Analysis Toolkit (ESAT, github/garber-lab/ESAT) with parameters -wlen 100 -wOlap 50 -wExt 0 -scPrep (Derr et al., 2016). Finally, UMIs that likely result from sequencing errors were corrected by merging any UMIs that were observed only once and have 1 hamming distance from a UMI detected by two or more aligned reads. Only cell barcodes with more than 1,000 UMIs were analyzed. Cell barcodes with mostly erythrocyte genes (HBA, HBB) were removed. From here on, the remaining cell barcodes in the matrix would be referred to as cells. The final gene by cell matrix was normalized using the scran package v3.10 (Lun et al., 2016). The normalized matrix was used for dimensionality reduction by first selecting variable genes that had a high coefficient of variance (CV) and were expressed (\u003e = 1 UMI) by more than three cells. Influenza viral genes, interferon stimulated genes, and cell cycle related genes were removed from the variable gene list in order to minimize the impact of viral responses and mitosis on clustering and cell type identification. This resulted in the selection of 2484 variable genes. t-distributed stochastic neighbor embedding (tSNE) was applied to the first ten principal components (PCs), which explained 95% of the total data variance. Density clustering (Rodriguez and Laio, 2014) was performed on the resulting tSNE coordinates and identified four major clusters: epithelial cells, neutrophils, macrophages and leukocytes. The epithelial cell cluster and the leukocyte cluster were then re-clustered independently, as described above, to identify populations within each metacluster. Specifically, the epithelial cell cluster was re-embedded using 2629 variable genes selected by the same criteria mentioned in the previous section and 13 PCs that explained 95% of the variance. Density clustering over the epithelial cell subset revealed ten clusters. Differential gene expression analysis using edgeR (Robinson et al., 2010) was performed to identify marker genes for each cluster. Influenza-infected and bystander cells were identified after correcting for sample-specific distribution of ambient influenza mRNA contamination and predicted cells most likely to be infected identified using a hurdle zero inflated negative binomial (ZINB) model and a support vector machine (SVM) classifier.\n\nPower Calculations for Detection of Rare Transcripts\nWe conducted the following statistical analysis to estimate the effects of various factors on our ability to make confident claims regarding the presence/absence of transcripts of interest (e.g., ACE2), both within individual cells and clusters (Figure S6). Specifically, we investigated the roles of capture/reverse transcription efficiency, ACE2 expression level, sequencing depth, and cell numbers. Taken together, the results of this power analysis are in agreement with other efforts to model biological and technical sources of zero-inflation within scRNA-seq data (e.g., https://satijalab.org/howmanycells and Kharchenko et al., 2014, Svensson, 2020).\nWe began by quantifying how likely we are to capture and transcribe at least one ACE2 mRNA molecule, as a function of the number ACE2 mRNA molecules per cell and a protocol’s efficiency (Figure S6A). Drop-Seq has a capture/transcription efficiency of ∼10% (as estimated using ERCC spike ins; see (Macosko et al., 2015), and the experimental platforms used in this study are either equivalent (e.g., Seq-Well v1, (Gierahn et al., 2017) or superior (e.g., 10-fold better unique molecule detection, 5-fold better gene detection using Seq-Well S3;(Hughes et al., 2019)). Most relevant to this context, inferior turbinate scrapings were processed using both Seq-Well v1 and Seq-Well S3 (Figure S3B). Importantly, Seq-Well S3 provided \u003e two-fold increase in the detection frequency of rare ACE2 transcripts (i.e., ACE2+: 4.7% for v1 versus 9.8% for S3), making it reasonable to expect that such improvements in single-cell experimental technologies have yielded corresponding improvements in capture and transcription efficiency. Based on Drop-Seq’s 10% efficiency, even if ACE2 is expressed at the low level of 5 mRNA molecules per cell (a reasonable order-of-magnitude estimate, given that non-human primate ileum cells had a maximum of 10 ACE2 unique molecules per cell observed via sequencing and an average of 1.93 molecules per cell in expressing cells, see Figures 3B and 3C), our experimental platforms have a minimum likelihood of 41% to capture and reverse transcribe at least one ACE2 mRNA molecule in any given individual cell. This likelihood rapidly increases if we estimate higher efficiencies for improved scRNA-seq technologies (e.g., 67% likelihood within any individual cell at 20% capture/transcription efficiency, 76% likelihood at 25% efficiency, Figure S6A). Thus, while transcript drop-out may reduce the fraction of positive cells, with the capture and transcription efficiencies of improved single-cell technologies, the impact is likely to be minor (reads are likely underestimated by up to a factor of ∼2.5x), given a sufficient depth of sequencing (see below). We note that this impacts both clusters deemed to contain and not contain ACE2+ cells, and suggests our percentages are likely lower bounds for true expression (within a factor of ∼2.5x).\nNext, we examined the probability of sequencing an ACE2 transcript as a function of read depth and ACE2’s fractional abundance in each single cell within our sequencing libraries. First, across two different tissues (non-human primate ileum and lung, representing a high expresser of ACE2 and low expresser, respectively), we calculated the proportion of unique ACE2 molecules in our ACE2+ cells (defined as any cell with at least 1 UMI aligning to ACE2) as a fraction of total reads within individual cells to provide an order-of-magnitude estimate for average ACE2 abundance in our single-cell sequencing libraries (i.e., the probability that a read within a cell corresponds to a unique molecule of ACE2, Figure S6B). We highlight that by calculating probabilities based on ACE2 unique molecules divided by an individual cell’s total reads, we are providing a conservative estimate for the probability of observing ACE2 as a function of sequencing depth (e.g., as compared to basing these probabilities on ACE2 non-UMI-collapsed reads divided by total reads). Next, we obtained information on the number of reads in these cell populations to provide estimates of average sequencing depths (Figure S6C). Using the mean fractional abundances of ACE2 from each tissue (Figure S6B) and the mean read depths for all genes (Figure S6C), we calculated the probability of detecting at least 1 ACE2 molecule (i.e., P(detecting \u003e 0 ACE2 molecules) = 1 - (1 - ACE2 fractional abundance)Read depth). This results in a 93.7% probability in ileum-derived cell libraries that contain ACE2, and a 76.0% probability for lung-derived cell libraries, indicating that our sequencing depths are sufficient to detect ACE2+ cells (Figure S6D).\nTo further evaluate whether our ability to detect ACE2+ cells was an artifact of sequencing depth, we compared the number of ACE2+ cells in a cluster to the mean number of reads across all cells in that same cluster (Figure S6E). We did not observe any significant correlation: the ileum cell cluster with the highest number of ACE2+ cells had the lowest sequencing depth of all ileum clusters, and the lung cell cluster with the highest number of ACE2+ cells was approximately average in its read depth (on a log-log scale, Pearson’s r = −0.31, non-significant). Further, when comparing ACE2+ cells to ACE2- cells within a given tissue, we did not observe a positive correlation between read depth and ACE2 status (i.e., mean ± standard error of the mean, SEM, reads among all lung cells = 28,512 ± 344; mean ± SEM reads among ACE2+ lung cells = 28,553 ± 2,988; mean ± SEM reads among all ileum cells = 14,864 ± 288; mean ± SEM reads among ACE2+ ileum cells = 10,591 ± 441, full statistics on cell depth among ACE2+ cells compared to ACE2- cells of the same cell type can be found in Table S9). Thus, we can be confident that the observed differences in ACE2+ proportions across clusters are not driven by differences in sequencing depth.\nFinally, we investigated how observed differences in ACE2+ proportions across clusters might be affected by cell sampling. Using the proportion of ACE2+ cells in a “typical” cluster annotated as being ACE2 positive (i.e., 6.8% in non-human primate type II pneumocytes, Figure 1), we calculated the cluster sizes needed to be confident that the probability of observing zero to a few positive cells is unlikely to have arisen by random chance (probabilities calculated under a negative binomial distribution with parameter p = 0.068, Figure S6E). We found that as cluster sizes approach and exceed 100 cells, the probability of observing zero to a few positive cells rapidly approaches zero, if we assume 6.8% of cells are positive. Further, to examine our confidence in estimating an approximate upper bound (ignoring the impact of protocol inefficiencies discussed above) for the fraction of cells positive in a cluster as a function of the number of cells in that cluster, we also calculated the probability of observing zero (and its complement, probability of observing at least 1) ACE2+ cells as a function of cluster size across true positive proportions ranging from 0.1% to 10% (probabilities calculated under a negative binomial distribution with parameter p = 0.001 to 0.1, representing hypothetical proportions of ACE2+ cells Figure S6F). Given our typical cluster sizes (on the order of hundreds of cells, exact values provided in Table S9), we find that for us to observe 0 ACE2+ cells in a cluster due to sampling artifacts, the fraction of true positives must be ∼1% or less. Thus, these complementary approaches demonstrate that our observed variations in ACE2+ cell proportions across clusters likely reflect underlying biological differences, rather than random chance.\n\nStatistical Testing\nParameters such as sample size, number of replicates, number of independent experiments, measures of center, dispersion, and precision (mean ± SEM) and statistical significances are reported in Figures and Figure Legends. A p value less than 0.05 was considered significant. Where appropriate, a Bonferroni or FDR correction was used to account for multiple tests, alternative correction methods are noted in the figure legends or Methods. All statistical tests corresponding to differential gene expression are described above and completed using R language for Statistical Computing."}
LitCovid-PD-CHEBI
{"project":"LitCovid-PD-CHEBI","denotations":[{"id":"T73454","span":{"begin":1893,"end":1895},"obj":"Chemical"},{"id":"T1289","span":{"begin":3219,"end":3222},"obj":"Chemical"},{"id":"T10711","span":{"begin":3910,"end":3912},"obj":"Chemical"},{"id":"T62284","span":{"begin":4341,"end":4343},"obj":"Chemical"},{"id":"T54385","span":{"begin":5014,"end":5017},"obj":"Chemical"},{"id":"T25822","span":{"begin":5341,"end":5344},"obj":"Chemical"},{"id":"T27564","span":{"begin":7070,"end":7072},"obj":"Chemical"},{"id":"T67203","span":{"begin":7483,"end":7485},"obj":"Chemical"},{"id":"T91028","span":{"begin":8245,"end":8247},"obj":"Chemical"},{"id":"T39198","span":{"begin":8286,"end":8289},"obj":"Chemical"},{"id":"T21041","span":{"begin":11059,"end":11061},"obj":"Chemical"},{"id":"T49594","span":{"begin":13628,"end":13631},"obj":"Chemical"},{"id":"T75445","span":{"begin":14053,"end":14063},"obj":"Chemical"},{"id":"T40879","span":{"begin":16334,"end":16342},"obj":"Chemical"},{"id":"T78056","span":{"begin":16382,"end":16391},"obj":"Chemical"},{"id":"T35798","span":{"begin":16719,"end":16727},"obj":"Chemical"},{"id":"T3501","span":{"begin":16783,"end":16785},"obj":"Chemical"},{"id":"T914","span":{"begin":16921,"end":16923},"obj":"Chemical"},{"id":"T21728","span":{"begin":16960,"end":16962},"obj":"Chemical"},{"id":"T55329","span":{"begin":17086,"end":17088},"obj":"Chemical"},{"id":"T49729","span":{"begin":17356,"end":17365},"obj":"Chemical"},{"id":"T70596","span":{"begin":17491,"end":17500},"obj":"Chemical"},{"id":"T14582","span":{"begin":17557,"end":17566},"obj":"Chemical"},{"id":"T92850","span":{"begin":17738,"end":17746},"obj":"Chemical"},{"id":"T18549","span":{"begin":18882,"end":18891},"obj":"Chemical"},{"id":"T76325","span":{"begin":19205,"end":19213},"obj":"Chemical"},{"id":"T9827","span":{"begin":19304,"end":19313},"obj":"Chemical"},{"id":"T1128","span":{"begin":19908,"end":19916},"obj":"Chemical"},{"id":"T15833","span":{"begin":19945,"end":19954},"obj":"Chemical"},{"id":"T17094","span":{"begin":21732,"end":21734},"obj":"Chemical"}],"attributes":[{"id":"A1201","pred":"chebi_id","subj":"T73454","obj":"http://purl.obolibrary.org/obo/CHEBI_74067"},{"id":"A91055","pred":"chebi_id","subj":"T1289","obj":"http://purl.obolibrary.org/obo/CHEBI_36751"},{"id":"A35420","pred":"chebi_id","subj":"T1289","obj":"http://purl.obolibrary.org/obo/CHEBI_62248"},{"id":"A69900","pred":"chebi_id","subj":"T10711","obj":"http://purl.obolibrary.org/obo/CHEBI_74067"},{"id":"A78683","pred":"chebi_id","subj":"T62284","obj":"http://purl.obolibrary.org/obo/CHEBI_29387"},{"id":"A67569","pred":"chebi_id","subj":"T54385","obj":"http://purl.obolibrary.org/obo/CHEBI_36751"},{"id":"A31444","pred":"chebi_id","subj":"T54385","obj":"http://purl.obolibrary.org/obo/CHEBI_62248"},{"id":"A7361","pred":"chebi_id","subj":"T25822","obj":"http://purl.obolibrary.org/obo/CHEBI_36751"},{"id":"A14788","pred":"chebi_id","subj":"T25822","obj":"http://purl.obolibrary.org/obo/CHEBI_62248"},{"id":"A8564","pred":"chebi_id","subj":"T27564","obj":"http://purl.obolibrary.org/obo/CHEBI_29387"},{"id":"A57541","pred":"chebi_id","subj":"T67203","obj":"http://purl.obolibrary.org/obo/CHEBI_74067"},{"id":"A36781","pred":"chebi_id","subj":"T91028","obj":"http://purl.obolibrary.org/obo/CHEBI_30347"},{"id":"A41610","pred":"chebi_id","subj":"T39198","obj":"http://purl.obolibrary.org/obo/CHEBI_63631"},{"id":"A10184","pred":"chebi_id","subj":"T21041","obj":"http://purl.obolibrary.org/obo/CHEBI_141450"},{"id":"A35549","pred":"chebi_id","subj":"T49594","obj":"http://purl.obolibrary.org/obo/CHEBI_31585"},{"id":"A41959","pred":"chebi_id","subj":"T49594","obj":"http://purl.obolibrary.org/obo/CHEBI_5588"},{"id":"A3877","pred":"chebi_id","subj":"T75445","obj":"http://purl.obolibrary.org/obo/CHEBI_52999"},{"id":"A80708","pred":"chebi_id","subj":"T40879","obj":"http://purl.obolibrary.org/obo/CHEBI_25367"},{"id":"A53698","pred":"chebi_id","subj":"T78056","obj":"http://purl.obolibrary.org/obo/CHEBI_25367"},{"id":"A36411","pred":"chebi_id","subj":"T35798","obj":"http://purl.obolibrary.org/obo/CHEBI_25367"},{"id":"A38912","pred":"chebi_id","subj":"T3501","obj":"http://purl.obolibrary.org/obo/CHEBI_29388"},{"id":"A79159","pred":"chebi_id","subj":"T914","obj":"http://purl.obolibrary.org/obo/CHEBI_29388"},{"id":"A48354","pred":"chebi_id","subj":"T21728","obj":"http://purl.obolibrary.org/obo/CHEBI_29388"},{"id":"A81710","pred":"chebi_id","subj":"T55329","obj":"http://purl.obolibrary.org/obo/CHEBI_29388"},{"id":"A36787","pred":"chebi_id","subj":"T49729","obj":"http://purl.obolibrary.org/obo/CHEBI_25367"},{"id":"A38983","pred":"chebi_id","subj":"T70596","obj":"http://purl.obolibrary.org/obo/CHEBI_25367"},{"id":"A20087","pred":"chebi_id","subj":"T14582","obj":"http://purl.obolibrary.org/obo/CHEBI_25367"},{"id":"A10197","pred":"chebi_id","subj":"T92850","obj":"http://purl.obolibrary.org/obo/CHEBI_25367"},{"id":"A10665","pred":"chebi_id","subj":"T18549","obj":"http://purl.obolibrary.org/obo/CHEBI_25367"},{"id":"A52167","pred":"chebi_id","subj":"T76325","obj":"http://purl.obolibrary.org/obo/CHEBI_25367"},{"id":"A95015","pred":"chebi_id","subj":"T9827","obj":"http://purl.obolibrary.org/obo/CHEBI_25367"},{"id":"A23614","pred":"chebi_id","subj":"T1128","obj":"http://purl.obolibrary.org/obo/CHEBI_25367"},{"id":"A82135","pred":"chebi_id","subj":"T15833","obj":"http://purl.obolibrary.org/obo/CHEBI_25367"},{"id":"A1329","pred":"chebi_id","subj":"T17094","obj":"http://purl.obolibrary.org/obo/CHEBI_74067"}],"text":"Quantification and Statistical Analysis\n\nNon-Human Primate Lung and Ileum\nLibraries corresponding to 7 animals (variable number of tissues per animal) were sequenced using Illumina NextSeq. Reads were aligned to the M. mulatta genome assembly 8.0.1 annotation version 102 and processed according to the Drop-Seq Computational Protocol v2.0 (https://github.com/broadinstitute/Drop-seq). Data was normalized and scaled using the Seurat R package v2.3.4 (https://satijalab.org/seurat/): transforming the data to loge(UMI+1) and applying a scale factor of 10,000. To identify major axes of variation within our data, we first examined only highly variable genes across all cells, yielding approximately 1,000-3,000 variable genes with average expression \u003e 0.1 log-normalized UMI across all cells. An approximate principal component analysis was applied to the cells to generate 100 principal components (PCs). Using the JackStraw function within Seurat, we identified significant PCs to be used for subsequent clustering and further dimensionality reduction. For 2D visualization and cell type clustering, we used a Uniform Manifold Approximation and Projection (UMAP) dimensionality reduction technique (https://github.com/lmcinnes/umap) with “min_dist” set to 0.5 and “n_neighbors” set to 30. To identify clusters of transcriptionally similar cells, we employed unsupervised clustering as described above using the FindClusters tool within the Seurat R package with default parameters and k.param set to 10 and resolution set to 0.5. Each cluster was sub-clustered to identify more granular cell types, requiring each cell type to express \u003e 25 significantly upregulated genes by differential expression test (FindMarkers implemented in Seurat, setting “test.use” to “bimod,” Bonferroni-adjusted p value cutoff \u003c 0.001). Differential expression tests between cells from ACE2 + versus ACE2 - Type II Pneumocytes were conducted using the SCDE R package with default parameters (Kharchenko et al., 2014). Expression data for epithelial cells and enterocytes included in this dataset can be visualized and downloaded here: https://singlecell.broadinstitute.org/single_cell/study/SCP807?scpbr=the-alexandria-project#study-summary.\n\nHuman Lung Tissue\nLibraries corresponding to 8 donors were sequenced using Illumina NextSeq. Reads were aligned to the hg19 genome assembly and processed according to the Drop-Seq Computational Protocol v2.0 (https://github.com/broadinstitute/Drop-seq). Data was normalized and scaled using the Seurat R package v3.1.0 (https://satijalab.org/seurat/), transforming the data to loge(UMI+1) and applying a scale factor of 10,000. For each array, we assessed the quality of constructed libraries by examining the distribution of reads, genes and transcripts per cell. Variable gene selection, principal components analysis, and selection of significant principal components was performed as above. We visualized our results in a two-dimensional space using UMAP (https://github.com/lmcinnes/umap), and annotated each cluster based on the identification of highly expressed genes. To further characterize substructure within cell types (for example, T cells), we performed dimensionality reduction (PCA) and clustering over those cells alone. Sub-clusters (i.e., clusters within broad cell type classifications) were annotated by cross-referencing cluster-defining genes with curated gene lists and online databases SaVanT (http://newpathways.mcdb.ucla.edu/savant-dev/) and GSEA/MsigDB (https://www.gsea-msigdb.org/gsea/msigdb/index.jsp). Proliferating cells from the human lung (Figure 2C) express high levels of mitotic markers, such as MKI67, and represent primarily T cells (CD3D, CD3E), B cells/antibody-secreting cells (IGJ, MZB1, IGHG1), and myeloid cells (CD14, APOE) and represent a composite cell cluster. Differential expression analysis between ACE2+ TMPRSS2+ and negative type II pneumocytes was performed in Seurat using a likelihood-ratio test (FindMarkers implemented in Seurat, setting “test.use” to bimod). Expression data for epithelial cells included in this dataset can be visualized and downloaded here: https://singlecell.broadinstitute.org/single_cell/study/SCP814?scpbr=the-alexandria-project#study-summary.\n\nHuman Ileum\nLibraries corresponding to 13 donors were sequenced using Illumina NovaSeq S2 with a Read 1 26bp, Read 2 91bp, Index 1 8bp configuration before reads were aligned to GRCh38. Each sample was filtered individually for low quality cells and genes by analyzing distributions of reads, transcripts, percent reads mapped to mitochondrial genes, and complexity per cell, then merged as an outer join to create a single dataset. Clustering and differential expression tests were processed using Seurat v3.1.0 (https://satijalab.org/seurat/). Normalization and variable gene selection was processed with SCTransform (https://github.com/ChristophH/sctransform). Clustering for major cell types was performed using Louvain clustering on dimensionally reduced PCA space with resolution set via grid search optimizing for maximum average silhouette score. Due to the scale of the dataset, a randomized subsampling from across the dataset was used to calculate the silhouette score. We annotated clusters based on highly expressed genes, then sub-clusters were characterized by performing PCA dimensionality reduction and clustering over those cells alone, and annotated based on highly expressed genes found via one-versus-rest differential expression test (Wilcoxon) within the major cell type. Differential expression analysis between ACE2 + TMPRSS2 + and negative epithelial cells was performed in Seurat using a Wilcoxon test and Bonferroni p value correction. Expression data for epithelial cells included in this dataset can be visualized and downloaded here: https://singlecell.broadinstitute.org/single_cell/study/SCP812?scpbr=the-alexandria-project#study-summary.\n\nHuman Adult Nasal Mucosa\nSample processing, sequencing, and analysis was performed as in (Ordovas-Montanes et al., 2018). Briefly, scRNA-seq cell suspensions were freshly processed using Seq-Well v1 and Seurat v2.3.4 was utilized for computational analyses presented here (Butler et al., 2018, Satija et al., 2015). Cell by gene matrix and R code for initialization of object available to download as Supplemental Data and Supplementary Tables here https://www.nature.com/articles/s41586-018-0449-8 and here:\nhttp://shaleklab.com/resource/mapping-allergic-inflammation/? and visualized here: https://singlecell.broadinstitute.org/single_cell/study/SCP253?scpbr=the-alexandria-project#study-summary. Scores for various cytokines acting on human airway epithelial cells were calculated based on gene lists derived for (Ordovas-Montanes et al., 2018), calculated using AddModuleScore function Seurat, and effect size calculated by Cohen’s d, as previously reported.\n\nGranulomatous Tissue from Mycobacterium Tuberculosis Infected NHPs\nLibraries corresponding to 10 animals (variable number of tissues/animal) were sequenced using Illumina NovaSeq S2. Data was aligned using the Dropseq-tools pipeline on Terra (app.terra.bio) to M. fascicularis reference genome assembly 5, annotation version 101. Clustering was performed using Leiden clustering in the Scanpy (scanpy.readthedocs.io) package (Wolf et al., 2018). Cell type labels were assigned using known marker genes. In this analysis, we include all epithelial cell subsets (secretory, multiciliated, type II pneumocytes, and type I pneumocytes) from all samples. Differential expression between ACE2 + TMPRSS2 + cells and other cells of the matched cell subtype (e.g., Secretory Cells) were performed using the “bimod” likelihood-ratio test within each cell subtype and filtered on Benjamini-Hochberg-corrected p value \u003c 0.05. Expression data for epithelial cells included in this dataset can be visualized and downloaded here:\nhttps://singlecell.broadinstitute.org/single_cell/study/SCP806?scpbr=the-alexandria-project#study-summary.\n\nBasal Cell Cytokine Stimulation\nLibraries corresponding to 279 populations were sequenced using Illumina NextSeq. Reads were aligned to the hg19 or mm10 genome assembly using the cumulus platform https://cumulus-doc.readthedocs.io/en/0.12.0/smart_seq_2.html and output as TPM using RSEM v1.3.2. Populations were transformed to transcripts per 10K reads and log2(1+TP10K) transformed. ACE2 expression by stimulation condition and dose were assessed using one-way ANOVA with post hoc testing using a Bonferroni correction. Plots were generated using ggplot2, and transcriptome-wide differential expression was calculated using the Seurat R package v3.1.0 (https://satijalab.org/seurat), function FindMarkers with test.use = ”bimod.” Expression data can be visualized and downloaded here:\nhttps://singlecell.broadinstitute.org/single_cell/study/SCP822?scpbr=the-alexandria-project.\n\nInterferon Treatment of Mouse Nasal Mucosa\nLibraries corresponding to 4 mice, with 2 Seq-Well arrays per mouse were sequenced using Illumina NextSeq as described (Gierahn et al., 2017, Hughes et al., 2019). Reads were aligned to the mm10 genome and processed according to the Drop-Seq Computational Protocol v2.0 (https://github.com/broadinstitute/Drop-seq). Data was normalized and scaled using the Seurat R package v2.3.4 (https://satijalab.org/seurat/): transforming the data to loge(UMI+1) and applying a scale factor of 10,000. Cells with fewer than 1000 UMIs and 500 unique genes were removed. To identify major axes of variation within our data, we first examined only highly variable genes across all cells, yielding approximately 5,000 variable genes. An approximate principal component analysis was applied to the cells to generate 200 principal components (PCs). Using a combination of the Jackstraw function in Seurat and observing the “elbow” of the standard deviations of PCs, we chose the top 70 PCs for subsequent clustering and visualization. For 2D visualization, we used a Uniform Manifold Approximation and Projection (UMAP) dimensionality reduction technique (https://github.com/lmcinnes/umap) with “min_dist” set to 0.3 and “n_neighbors” set to 50. To identify clusters of transcriptionally similar cells, we employed unsupervised clustering as described above using the FindClusters tool within the Seurat R package with default parameters and k.param set to 10. Resolution was chosen based on maximization of the average silhouette width across all cells. Clusters were merged if a cell type expressed fewer than 25 significantly upregulated genes by differential expression test (FindAllMarkers implemented in Seurat, setting “test.use” to “bimod,” Bonferroni-adjusted p value cutoff \u003c 0.001). Differential expression tests between cells from saline-treated or IFNa-treated mice were assessed using the FindMarkers function with “test.use” set to “bimod. This dataset can be visualized and downloaded here:\nhttps://singlecell.broadinstitute.org/single_cell/study/SCP832?scpbr=the-alexandria-project#study-summary.\n\nLung from MHV68-Infected WT and IFNγR KO Mice\nLibraries corresponding to 14 mice were aligned to a custom reference genome encompassing both murine (mm10) and herpes virus genes: 84 known genes from MHV68 were retrieved from NCBI (NCBI: txid33708) and added to the mm10 mouse genome. Reads were aligned to the custom joint genome and processed according to the Drop-Seq Computational Protocol v2.0 (https://github.com/broadinstitute/Drop-seq). Barcodes with \u003c 200 unique genes, \u003e 20,000 UMI counts, and \u003e 30% of transcript counts derived from mitochondrially encoded genes were discarded. Data analysis was performed using the Scanpy Package following the common procedure, the expression matrices were normalized using scran’s size factor based approach and log transformed via scanpy’s pp.log1p() function (Lun et al., 2016, Wolf et al., 2018). SoupX was utilized to reduce ambient RNA bias, using default parameters with pCut set to 0.3, and was applied to each sample before merging the count matrices (Young and Behjati, 2020). UMI per cell and cell cycle were regressed out. Highly variable genes were selected by running pp.highly_variable_genes() for each sample separately, returning the top 4,000 variable genes per sample, and genes identified in variable in \u003e 5 samples were retained, yielding 14,305 genes. Next, only Epcam+ cells were considered, principal components (PCs) were calculated using only the selected variable genes, and 6 PCs were used to perform unsupervised Louvain clustering. Type I Pneumocytes were excluded from this analysis based on uniformly negative expression of Ace2, resulting in a final dataset subset of 5,558 cells. Cells were identified as infected if at least one viral read was detected.\n\nNasal Washes during Influenza Infection\nSample processing, sequencing, and analysis was performed as in (Cao et al., 2020). Reads were aligned to the GRCh37 reference genome combined with influenza genomes. Mapped reads from each sample were then corrected for Drop-seq barcode synthesis error using the Drop-seq core computational tools developed by the McCarroll Lab (Macosko et al., 2015). Genes were quantified using End Sequence Analysis Toolkit (ESAT, github/garber-lab/ESAT) with parameters -wlen 100 -wOlap 50 -wExt 0 -scPrep (Derr et al., 2016). Finally, UMIs that likely result from sequencing errors were corrected by merging any UMIs that were observed only once and have 1 hamming distance from a UMI detected by two or more aligned reads. Only cell barcodes with more than 1,000 UMIs were analyzed. Cell barcodes with mostly erythrocyte genes (HBA, HBB) were removed. From here on, the remaining cell barcodes in the matrix would be referred to as cells. The final gene by cell matrix was normalized using the scran package v3.10 (Lun et al., 2016). The normalized matrix was used for dimensionality reduction by first selecting variable genes that had a high coefficient of variance (CV) and were expressed (\u003e = 1 UMI) by more than three cells. Influenza viral genes, interferon stimulated genes, and cell cycle related genes were removed from the variable gene list in order to minimize the impact of viral responses and mitosis on clustering and cell type identification. This resulted in the selection of 2484 variable genes. t-distributed stochastic neighbor embedding (tSNE) was applied to the first ten principal components (PCs), which explained 95% of the total data variance. Density clustering (Rodriguez and Laio, 2014) was performed on the resulting tSNE coordinates and identified four major clusters: epithelial cells, neutrophils, macrophages and leukocytes. The epithelial cell cluster and the leukocyte cluster were then re-clustered independently, as described above, to identify populations within each metacluster. Specifically, the epithelial cell cluster was re-embedded using 2629 variable genes selected by the same criteria mentioned in the previous section and 13 PCs that explained 95% of the variance. Density clustering over the epithelial cell subset revealed ten clusters. Differential gene expression analysis using edgeR (Robinson et al., 2010) was performed to identify marker genes for each cluster. Influenza-infected and bystander cells were identified after correcting for sample-specific distribution of ambient influenza mRNA contamination and predicted cells most likely to be infected identified using a hurdle zero inflated negative binomial (ZINB) model and a support vector machine (SVM) classifier.\n\nPower Calculations for Detection of Rare Transcripts\nWe conducted the following statistical analysis to estimate the effects of various factors on our ability to make confident claims regarding the presence/absence of transcripts of interest (e.g., ACE2), both within individual cells and clusters (Figure S6). Specifically, we investigated the roles of capture/reverse transcription efficiency, ACE2 expression level, sequencing depth, and cell numbers. Taken together, the results of this power analysis are in agreement with other efforts to model biological and technical sources of zero-inflation within scRNA-seq data (e.g., https://satijalab.org/howmanycells and Kharchenko et al., 2014, Svensson, 2020).\nWe began by quantifying how likely we are to capture and transcribe at least one ACE2 mRNA molecule, as a function of the number ACE2 mRNA molecules per cell and a protocol’s efficiency (Figure S6A). Drop-Seq has a capture/transcription efficiency of ∼10% (as estimated using ERCC spike ins; see (Macosko et al., 2015), and the experimental platforms used in this study are either equivalent (e.g., Seq-Well v1, (Gierahn et al., 2017) or superior (e.g., 10-fold better unique molecule detection, 5-fold better gene detection using Seq-Well S3;(Hughes et al., 2019)). Most relevant to this context, inferior turbinate scrapings were processed using both Seq-Well v1 and Seq-Well S3 (Figure S3B). Importantly, Seq-Well S3 provided \u003e two-fold increase in the detection frequency of rare ACE2 transcripts (i.e., ACE2+: 4.7% for v1 versus 9.8% for S3), making it reasonable to expect that such improvements in single-cell experimental technologies have yielded corresponding improvements in capture and transcription efficiency. Based on Drop-Seq’s 10% efficiency, even if ACE2 is expressed at the low level of 5 mRNA molecules per cell (a reasonable order-of-magnitude estimate, given that non-human primate ileum cells had a maximum of 10 ACE2 unique molecules per cell observed via sequencing and an average of 1.93 molecules per cell in expressing cells, see Figures 3B and 3C), our experimental platforms have a minimum likelihood of 41% to capture and reverse transcribe at least one ACE2 mRNA molecule in any given individual cell. This likelihood rapidly increases if we estimate higher efficiencies for improved scRNA-seq technologies (e.g., 67% likelihood within any individual cell at 20% capture/transcription efficiency, 76% likelihood at 25% efficiency, Figure S6A). Thus, while transcript drop-out may reduce the fraction of positive cells, with the capture and transcription efficiencies of improved single-cell technologies, the impact is likely to be minor (reads are likely underestimated by up to a factor of ∼2.5x), given a sufficient depth of sequencing (see below). We note that this impacts both clusters deemed to contain and not contain ACE2+ cells, and suggests our percentages are likely lower bounds for true expression (within a factor of ∼2.5x).\nNext, we examined the probability of sequencing an ACE2 transcript as a function of read depth and ACE2’s fractional abundance in each single cell within our sequencing libraries. First, across two different tissues (non-human primate ileum and lung, representing a high expresser of ACE2 and low expresser, respectively), we calculated the proportion of unique ACE2 molecules in our ACE2+ cells (defined as any cell with at least 1 UMI aligning to ACE2) as a fraction of total reads within individual cells to provide an order-of-magnitude estimate for average ACE2 abundance in our single-cell sequencing libraries (i.e., the probability that a read within a cell corresponds to a unique molecule of ACE2, Figure S6B). We highlight that by calculating probabilities based on ACE2 unique molecules divided by an individual cell’s total reads, we are providing a conservative estimate for the probability of observing ACE2 as a function of sequencing depth (e.g., as compared to basing these probabilities on ACE2 non-UMI-collapsed reads divided by total reads). Next, we obtained information on the number of reads in these cell populations to provide estimates of average sequencing depths (Figure S6C). Using the mean fractional abundances of ACE2 from each tissue (Figure S6B) and the mean read depths for all genes (Figure S6C), we calculated the probability of detecting at least 1 ACE2 molecule (i.e., P(detecting \u003e 0 ACE2 molecules) = 1 - (1 - ACE2 fractional abundance)Read depth). This results in a 93.7% probability in ileum-derived cell libraries that contain ACE2, and a 76.0% probability for lung-derived cell libraries, indicating that our sequencing depths are sufficient to detect ACE2+ cells (Figure S6D).\nTo further evaluate whether our ability to detect ACE2+ cells was an artifact of sequencing depth, we compared the number of ACE2+ cells in a cluster to the mean number of reads across all cells in that same cluster (Figure S6E). We did not observe any significant correlation: the ileum cell cluster with the highest number of ACE2+ cells had the lowest sequencing depth of all ileum clusters, and the lung cell cluster with the highest number of ACE2+ cells was approximately average in its read depth (on a log-log scale, Pearson’s r = −0.31, non-significant). Further, when comparing ACE2+ cells to ACE2- cells within a given tissue, we did not observe a positive correlation between read depth and ACE2 status (i.e., mean ± standard error of the mean, SEM, reads among all lung cells = 28,512 ± 344; mean ± SEM reads among ACE2+ lung cells = 28,553 ± 2,988; mean ± SEM reads among all ileum cells = 14,864 ± 288; mean ± SEM reads among ACE2+ ileum cells = 10,591 ± 441, full statistics on cell depth among ACE2+ cells compared to ACE2- cells of the same cell type can be found in Table S9). Thus, we can be confident that the observed differences in ACE2+ proportions across clusters are not driven by differences in sequencing depth.\nFinally, we investigated how observed differences in ACE2+ proportions across clusters might be affected by cell sampling. Using the proportion of ACE2+ cells in a “typical” cluster annotated as being ACE2 positive (i.e., 6.8% in non-human primate type II pneumocytes, Figure 1), we calculated the cluster sizes needed to be confident that the probability of observing zero to a few positive cells is unlikely to have arisen by random chance (probabilities calculated under a negative binomial distribution with parameter p = 0.068, Figure S6E). We found that as cluster sizes approach and exceed 100 cells, the probability of observing zero to a few positive cells rapidly approaches zero, if we assume 6.8% of cells are positive. Further, to examine our confidence in estimating an approximate upper bound (ignoring the impact of protocol inefficiencies discussed above) for the fraction of cells positive in a cluster as a function of the number of cells in that cluster, we also calculated the probability of observing zero (and its complement, probability of observing at least 1) ACE2+ cells as a function of cluster size across true positive proportions ranging from 0.1% to 10% (probabilities calculated under a negative binomial distribution with parameter p = 0.001 to 0.1, representing hypothetical proportions of ACE2+ cells Figure S6F). Given our typical cluster sizes (on the order of hundreds of cells, exact values provided in Table S9), we find that for us to observe 0 ACE2+ cells in a cluster due to sampling artifacts, the fraction of true positives must be ∼1% or less. Thus, these complementary approaches demonstrate that our observed variations in ACE2+ cell proportions across clusters likely reflect underlying biological differences, rather than random chance.\n\nStatistical Testing\nParameters such as sample size, number of replicates, number of independent experiments, measures of center, dispersion, and precision (mean ± SEM) and statistical significances are reported in Figures and Figure Legends. A p value less than 0.05 was considered significant. Where appropriate, a Bonferroni or FDR correction was used to account for multiple tests, alternative correction methods are noted in the figure legends or Methods. All statistical tests corresponding to differential gene expression are described above and completed using R language for Statistical Computing."}
LitCovid-PD-GO-BP
{"project":"LitCovid-PD-GO-BP","denotations":[{"id":"T4","span":{"begin":1315,"end":1332},"obj":"http://purl.obolibrary.org/obo/GO_0006351"},{"id":"T5","span":{"begin":6483,"end":6495},"obj":"http://purl.obolibrary.org/obo/GO_0006954"},{"id":"T6","span":{"begin":10189,"end":10206},"obj":"http://purl.obolibrary.org/obo/GO_0006351"},{"id":"T7","span":{"begin":11546,"end":11556},"obj":"http://purl.obolibrary.org/obo/GO_0006351"},{"id":"T8","span":{"begin":12084,"end":12094},"obj":"http://purl.obolibrary.org/obo/GO_0007049"},{"id":"T9","span":{"begin":13048,"end":13057},"obj":"http://purl.obolibrary.org/obo/GO_0009058"},{"id":"T10","span":{"begin":14086,"end":14096},"obj":"http://purl.obolibrary.org/obo/GO_0007049"},{"id":"T11","span":{"begin":14207,"end":14214},"obj":"http://purl.obolibrary.org/obo/GO_0140014"},{"id":"T12","span":{"begin":14207,"end":14214},"obj":"http://purl.obolibrary.org/obo/GO_0000278"},{"id":"T13","span":{"begin":15102,"end":15117},"obj":"http://purl.obolibrary.org/obo/GO_0010467"},{"id":"T14","span":{"begin":15893,"end":15914},"obj":"http://purl.obolibrary.org/obo/GO_0001171"},{"id":"T15","span":{"begin":15901,"end":15914},"obj":"http://purl.obolibrary.org/obo/GO_0006351"},{"id":"T16","span":{"begin":16466,"end":16479},"obj":"http://purl.obolibrary.org/obo/GO_0006351"},{"id":"T17","span":{"begin":17241,"end":17254},"obj":"http://purl.obolibrary.org/obo/GO_0006351"},{"id":"T18","span":{"begin":17946,"end":17959},"obj":"http://purl.obolibrary.org/obo/GO_0006351"},{"id":"T19","span":{"begin":18031,"end":18041},"obj":"http://purl.obolibrary.org/obo/GO_0006351"},{"id":"T20","span":{"begin":18115,"end":18128},"obj":"http://purl.obolibrary.org/obo/GO_0006351"},{"id":"T21","span":{"begin":18571,"end":18581},"obj":"http://purl.obolibrary.org/obo/GO_0006351"},{"id":"T22","span":{"begin":23780,"end":23795},"obj":"http://purl.obolibrary.org/obo/GO_0010467"}],"text":"Quantification and Statistical Analysis\n\nNon-Human Primate Lung and Ileum\nLibraries corresponding to 7 animals (variable number of tissues per animal) were sequenced using Illumina NextSeq. Reads were aligned to the M. mulatta genome assembly 8.0.1 annotation version 102 and processed according to the Drop-Seq Computational Protocol v2.0 (https://github.com/broadinstitute/Drop-seq). Data was normalized and scaled using the Seurat R package v2.3.4 (https://satijalab.org/seurat/): transforming the data to loge(UMI+1) and applying a scale factor of 10,000. To identify major axes of variation within our data, we first examined only highly variable genes across all cells, yielding approximately 1,000-3,000 variable genes with average expression \u003e 0.1 log-normalized UMI across all cells. An approximate principal component analysis was applied to the cells to generate 100 principal components (PCs). Using the JackStraw function within Seurat, we identified significant PCs to be used for subsequent clustering and further dimensionality reduction. For 2D visualization and cell type clustering, we used a Uniform Manifold Approximation and Projection (UMAP) dimensionality reduction technique (https://github.com/lmcinnes/umap) with “min_dist” set to 0.5 and “n_neighbors” set to 30. To identify clusters of transcriptionally similar cells, we employed unsupervised clustering as described above using the FindClusters tool within the Seurat R package with default parameters and k.param set to 10 and resolution set to 0.5. Each cluster was sub-clustered to identify more granular cell types, requiring each cell type to express \u003e 25 significantly upregulated genes by differential expression test (FindMarkers implemented in Seurat, setting “test.use” to “bimod,” Bonferroni-adjusted p value cutoff \u003c 0.001). Differential expression tests between cells from ACE2 + versus ACE2 - Type II Pneumocytes were conducted using the SCDE R package with default parameters (Kharchenko et al., 2014). Expression data for epithelial cells and enterocytes included in this dataset can be visualized and downloaded here: https://singlecell.broadinstitute.org/single_cell/study/SCP807?scpbr=the-alexandria-project#study-summary.\n\nHuman Lung Tissue\nLibraries corresponding to 8 donors were sequenced using Illumina NextSeq. Reads were aligned to the hg19 genome assembly and processed according to the Drop-Seq Computational Protocol v2.0 (https://github.com/broadinstitute/Drop-seq). Data was normalized and scaled using the Seurat R package v3.1.0 (https://satijalab.org/seurat/), transforming the data to loge(UMI+1) and applying a scale factor of 10,000. For each array, we assessed the quality of constructed libraries by examining the distribution of reads, genes and transcripts per cell. Variable gene selection, principal components analysis, and selection of significant principal components was performed as above. We visualized our results in a two-dimensional space using UMAP (https://github.com/lmcinnes/umap), and annotated each cluster based on the identification of highly expressed genes. To further characterize substructure within cell types (for example, T cells), we performed dimensionality reduction (PCA) and clustering over those cells alone. Sub-clusters (i.e., clusters within broad cell type classifications) were annotated by cross-referencing cluster-defining genes with curated gene lists and online databases SaVanT (http://newpathways.mcdb.ucla.edu/savant-dev/) and GSEA/MsigDB (https://www.gsea-msigdb.org/gsea/msigdb/index.jsp). Proliferating cells from the human lung (Figure 2C) express high levels of mitotic markers, such as MKI67, and represent primarily T cells (CD3D, CD3E), B cells/antibody-secreting cells (IGJ, MZB1, IGHG1), and myeloid cells (CD14, APOE) and represent a composite cell cluster. Differential expression analysis between ACE2+ TMPRSS2+ and negative type II pneumocytes was performed in Seurat using a likelihood-ratio test (FindMarkers implemented in Seurat, setting “test.use” to bimod). Expression data for epithelial cells included in this dataset can be visualized and downloaded here: https://singlecell.broadinstitute.org/single_cell/study/SCP814?scpbr=the-alexandria-project#study-summary.\n\nHuman Ileum\nLibraries corresponding to 13 donors were sequenced using Illumina NovaSeq S2 with a Read 1 26bp, Read 2 91bp, Index 1 8bp configuration before reads were aligned to GRCh38. Each sample was filtered individually for low quality cells and genes by analyzing distributions of reads, transcripts, percent reads mapped to mitochondrial genes, and complexity per cell, then merged as an outer join to create a single dataset. Clustering and differential expression tests were processed using Seurat v3.1.0 (https://satijalab.org/seurat/). Normalization and variable gene selection was processed with SCTransform (https://github.com/ChristophH/sctransform). Clustering for major cell types was performed using Louvain clustering on dimensionally reduced PCA space with resolution set via grid search optimizing for maximum average silhouette score. Due to the scale of the dataset, a randomized subsampling from across the dataset was used to calculate the silhouette score. We annotated clusters based on highly expressed genes, then sub-clusters were characterized by performing PCA dimensionality reduction and clustering over those cells alone, and annotated based on highly expressed genes found via one-versus-rest differential expression test (Wilcoxon) within the major cell type. Differential expression analysis between ACE2 + TMPRSS2 + and negative epithelial cells was performed in Seurat using a Wilcoxon test and Bonferroni p value correction. Expression data for epithelial cells included in this dataset can be visualized and downloaded here: https://singlecell.broadinstitute.org/single_cell/study/SCP812?scpbr=the-alexandria-project#study-summary.\n\nHuman Adult Nasal Mucosa\nSample processing, sequencing, and analysis was performed as in (Ordovas-Montanes et al., 2018). Briefly, scRNA-seq cell suspensions were freshly processed using Seq-Well v1 and Seurat v2.3.4 was utilized for computational analyses presented here (Butler et al., 2018, Satija et al., 2015). Cell by gene matrix and R code for initialization of object available to download as Supplemental Data and Supplementary Tables here https://www.nature.com/articles/s41586-018-0449-8 and here:\nhttp://shaleklab.com/resource/mapping-allergic-inflammation/? and visualized here: https://singlecell.broadinstitute.org/single_cell/study/SCP253?scpbr=the-alexandria-project#study-summary. Scores for various cytokines acting on human airway epithelial cells were calculated based on gene lists derived for (Ordovas-Montanes et al., 2018), calculated using AddModuleScore function Seurat, and effect size calculated by Cohen’s d, as previously reported.\n\nGranulomatous Tissue from Mycobacterium Tuberculosis Infected NHPs\nLibraries corresponding to 10 animals (variable number of tissues/animal) were sequenced using Illumina NovaSeq S2. Data was aligned using the Dropseq-tools pipeline on Terra (app.terra.bio) to M. fascicularis reference genome assembly 5, annotation version 101. Clustering was performed using Leiden clustering in the Scanpy (scanpy.readthedocs.io) package (Wolf et al., 2018). Cell type labels were assigned using known marker genes. In this analysis, we include all epithelial cell subsets (secretory, multiciliated, type II pneumocytes, and type I pneumocytes) from all samples. Differential expression between ACE2 + TMPRSS2 + cells and other cells of the matched cell subtype (e.g., Secretory Cells) were performed using the “bimod” likelihood-ratio test within each cell subtype and filtered on Benjamini-Hochberg-corrected p value \u003c 0.05. Expression data for epithelial cells included in this dataset can be visualized and downloaded here:\nhttps://singlecell.broadinstitute.org/single_cell/study/SCP806?scpbr=the-alexandria-project#study-summary.\n\nBasal Cell Cytokine Stimulation\nLibraries corresponding to 279 populations were sequenced using Illumina NextSeq. Reads were aligned to the hg19 or mm10 genome assembly using the cumulus platform https://cumulus-doc.readthedocs.io/en/0.12.0/smart_seq_2.html and output as TPM using RSEM v1.3.2. Populations were transformed to transcripts per 10K reads and log2(1+TP10K) transformed. ACE2 expression by stimulation condition and dose were assessed using one-way ANOVA with post hoc testing using a Bonferroni correction. Plots were generated using ggplot2, and transcriptome-wide differential expression was calculated using the Seurat R package v3.1.0 (https://satijalab.org/seurat), function FindMarkers with test.use = ”bimod.” Expression data can be visualized and downloaded here:\nhttps://singlecell.broadinstitute.org/single_cell/study/SCP822?scpbr=the-alexandria-project.\n\nInterferon Treatment of Mouse Nasal Mucosa\nLibraries corresponding to 4 mice, with 2 Seq-Well arrays per mouse were sequenced using Illumina NextSeq as described (Gierahn et al., 2017, Hughes et al., 2019). Reads were aligned to the mm10 genome and processed according to the Drop-Seq Computational Protocol v2.0 (https://github.com/broadinstitute/Drop-seq). Data was normalized and scaled using the Seurat R package v2.3.4 (https://satijalab.org/seurat/): transforming the data to loge(UMI+1) and applying a scale factor of 10,000. Cells with fewer than 1000 UMIs and 500 unique genes were removed. To identify major axes of variation within our data, we first examined only highly variable genes across all cells, yielding approximately 5,000 variable genes. An approximate principal component analysis was applied to the cells to generate 200 principal components (PCs). Using a combination of the Jackstraw function in Seurat and observing the “elbow” of the standard deviations of PCs, we chose the top 70 PCs for subsequent clustering and visualization. For 2D visualization, we used a Uniform Manifold Approximation and Projection (UMAP) dimensionality reduction technique (https://github.com/lmcinnes/umap) with “min_dist” set to 0.3 and “n_neighbors” set to 50. To identify clusters of transcriptionally similar cells, we employed unsupervised clustering as described above using the FindClusters tool within the Seurat R package with default parameters and k.param set to 10. Resolution was chosen based on maximization of the average silhouette width across all cells. Clusters were merged if a cell type expressed fewer than 25 significantly upregulated genes by differential expression test (FindAllMarkers implemented in Seurat, setting “test.use” to “bimod,” Bonferroni-adjusted p value cutoff \u003c 0.001). Differential expression tests between cells from saline-treated or IFNa-treated mice were assessed using the FindMarkers function with “test.use” set to “bimod. This dataset can be visualized and downloaded here:\nhttps://singlecell.broadinstitute.org/single_cell/study/SCP832?scpbr=the-alexandria-project#study-summary.\n\nLung from MHV68-Infected WT and IFNγR KO Mice\nLibraries corresponding to 14 mice were aligned to a custom reference genome encompassing both murine (mm10) and herpes virus genes: 84 known genes from MHV68 were retrieved from NCBI (NCBI: txid33708) and added to the mm10 mouse genome. Reads were aligned to the custom joint genome and processed according to the Drop-Seq Computational Protocol v2.0 (https://github.com/broadinstitute/Drop-seq). Barcodes with \u003c 200 unique genes, \u003e 20,000 UMI counts, and \u003e 30% of transcript counts derived from mitochondrially encoded genes were discarded. Data analysis was performed using the Scanpy Package following the common procedure, the expression matrices were normalized using scran’s size factor based approach and log transformed via scanpy’s pp.log1p() function (Lun et al., 2016, Wolf et al., 2018). SoupX was utilized to reduce ambient RNA bias, using default parameters with pCut set to 0.3, and was applied to each sample before merging the count matrices (Young and Behjati, 2020). UMI per cell and cell cycle were regressed out. Highly variable genes were selected by running pp.highly_variable_genes() for each sample separately, returning the top 4,000 variable genes per sample, and genes identified in variable in \u003e 5 samples were retained, yielding 14,305 genes. Next, only Epcam+ cells were considered, principal components (PCs) were calculated using only the selected variable genes, and 6 PCs were used to perform unsupervised Louvain clustering. Type I Pneumocytes were excluded from this analysis based on uniformly negative expression of Ace2, resulting in a final dataset subset of 5,558 cells. Cells were identified as infected if at least one viral read was detected.\n\nNasal Washes during Influenza Infection\nSample processing, sequencing, and analysis was performed as in (Cao et al., 2020). Reads were aligned to the GRCh37 reference genome combined with influenza genomes. Mapped reads from each sample were then corrected for Drop-seq barcode synthesis error using the Drop-seq core computational tools developed by the McCarroll Lab (Macosko et al., 2015). Genes were quantified using End Sequence Analysis Toolkit (ESAT, github/garber-lab/ESAT) with parameters -wlen 100 -wOlap 50 -wExt 0 -scPrep (Derr et al., 2016). Finally, UMIs that likely result from sequencing errors were corrected by merging any UMIs that were observed only once and have 1 hamming distance from a UMI detected by two or more aligned reads. Only cell barcodes with more than 1,000 UMIs were analyzed. Cell barcodes with mostly erythrocyte genes (HBA, HBB) were removed. From here on, the remaining cell barcodes in the matrix would be referred to as cells. The final gene by cell matrix was normalized using the scran package v3.10 (Lun et al., 2016). The normalized matrix was used for dimensionality reduction by first selecting variable genes that had a high coefficient of variance (CV) and were expressed (\u003e = 1 UMI) by more than three cells. Influenza viral genes, interferon stimulated genes, and cell cycle related genes were removed from the variable gene list in order to minimize the impact of viral responses and mitosis on clustering and cell type identification. This resulted in the selection of 2484 variable genes. t-distributed stochastic neighbor embedding (tSNE) was applied to the first ten principal components (PCs), which explained 95% of the total data variance. Density clustering (Rodriguez and Laio, 2014) was performed on the resulting tSNE coordinates and identified four major clusters: epithelial cells, neutrophils, macrophages and leukocytes. The epithelial cell cluster and the leukocyte cluster were then re-clustered independently, as described above, to identify populations within each metacluster. Specifically, the epithelial cell cluster was re-embedded using 2629 variable genes selected by the same criteria mentioned in the previous section and 13 PCs that explained 95% of the variance. Density clustering over the epithelial cell subset revealed ten clusters. Differential gene expression analysis using edgeR (Robinson et al., 2010) was performed to identify marker genes for each cluster. Influenza-infected and bystander cells were identified after correcting for sample-specific distribution of ambient influenza mRNA contamination and predicted cells most likely to be infected identified using a hurdle zero inflated negative binomial (ZINB) model and a support vector machine (SVM) classifier.\n\nPower Calculations for Detection of Rare Transcripts\nWe conducted the following statistical analysis to estimate the effects of various factors on our ability to make confident claims regarding the presence/absence of transcripts of interest (e.g., ACE2), both within individual cells and clusters (Figure S6). Specifically, we investigated the roles of capture/reverse transcription efficiency, ACE2 expression level, sequencing depth, and cell numbers. Taken together, the results of this power analysis are in agreement with other efforts to model biological and technical sources of zero-inflation within scRNA-seq data (e.g., https://satijalab.org/howmanycells and Kharchenko et al., 2014, Svensson, 2020).\nWe began by quantifying how likely we are to capture and transcribe at least one ACE2 mRNA molecule, as a function of the number ACE2 mRNA molecules per cell and a protocol’s efficiency (Figure S6A). Drop-Seq has a capture/transcription efficiency of ∼10% (as estimated using ERCC spike ins; see (Macosko et al., 2015), and the experimental platforms used in this study are either equivalent (e.g., Seq-Well v1, (Gierahn et al., 2017) or superior (e.g., 10-fold better unique molecule detection, 5-fold better gene detection using Seq-Well S3;(Hughes et al., 2019)). Most relevant to this context, inferior turbinate scrapings were processed using both Seq-Well v1 and Seq-Well S3 (Figure S3B). Importantly, Seq-Well S3 provided \u003e two-fold increase in the detection frequency of rare ACE2 transcripts (i.e., ACE2+: 4.7% for v1 versus 9.8% for S3), making it reasonable to expect that such improvements in single-cell experimental technologies have yielded corresponding improvements in capture and transcription efficiency. Based on Drop-Seq’s 10% efficiency, even if ACE2 is expressed at the low level of 5 mRNA molecules per cell (a reasonable order-of-magnitude estimate, given that non-human primate ileum cells had a maximum of 10 ACE2 unique molecules per cell observed via sequencing and an average of 1.93 molecules per cell in expressing cells, see Figures 3B and 3C), our experimental platforms have a minimum likelihood of 41% to capture and reverse transcribe at least one ACE2 mRNA molecule in any given individual cell. This likelihood rapidly increases if we estimate higher efficiencies for improved scRNA-seq technologies (e.g., 67% likelihood within any individual cell at 20% capture/transcription efficiency, 76% likelihood at 25% efficiency, Figure S6A). Thus, while transcript drop-out may reduce the fraction of positive cells, with the capture and transcription efficiencies of improved single-cell technologies, the impact is likely to be minor (reads are likely underestimated by up to a factor of ∼2.5x), given a sufficient depth of sequencing (see below). We note that this impacts both clusters deemed to contain and not contain ACE2+ cells, and suggests our percentages are likely lower bounds for true expression (within a factor of ∼2.5x).\nNext, we examined the probability of sequencing an ACE2 transcript as a function of read depth and ACE2’s fractional abundance in each single cell within our sequencing libraries. First, across two different tissues (non-human primate ileum and lung, representing a high expresser of ACE2 and low expresser, respectively), we calculated the proportion of unique ACE2 molecules in our ACE2+ cells (defined as any cell with at least 1 UMI aligning to ACE2) as a fraction of total reads within individual cells to provide an order-of-magnitude estimate for average ACE2 abundance in our single-cell sequencing libraries (i.e., the probability that a read within a cell corresponds to a unique molecule of ACE2, Figure S6B). We highlight that by calculating probabilities based on ACE2 unique molecules divided by an individual cell’s total reads, we are providing a conservative estimate for the probability of observing ACE2 as a function of sequencing depth (e.g., as compared to basing these probabilities on ACE2 non-UMI-collapsed reads divided by total reads). Next, we obtained information on the number of reads in these cell populations to provide estimates of average sequencing depths (Figure S6C). Using the mean fractional abundances of ACE2 from each tissue (Figure S6B) and the mean read depths for all genes (Figure S6C), we calculated the probability of detecting at least 1 ACE2 molecule (i.e., P(detecting \u003e 0 ACE2 molecules) = 1 - (1 - ACE2 fractional abundance)Read depth). This results in a 93.7% probability in ileum-derived cell libraries that contain ACE2, and a 76.0% probability for lung-derived cell libraries, indicating that our sequencing depths are sufficient to detect ACE2+ cells (Figure S6D).\nTo further evaluate whether our ability to detect ACE2+ cells was an artifact of sequencing depth, we compared the number of ACE2+ cells in a cluster to the mean number of reads across all cells in that same cluster (Figure S6E). We did not observe any significant correlation: the ileum cell cluster with the highest number of ACE2+ cells had the lowest sequencing depth of all ileum clusters, and the lung cell cluster with the highest number of ACE2+ cells was approximately average in its read depth (on a log-log scale, Pearson’s r = −0.31, non-significant). Further, when comparing ACE2+ cells to ACE2- cells within a given tissue, we did not observe a positive correlation between read depth and ACE2 status (i.e., mean ± standard error of the mean, SEM, reads among all lung cells = 28,512 ± 344; mean ± SEM reads among ACE2+ lung cells = 28,553 ± 2,988; mean ± SEM reads among all ileum cells = 14,864 ± 288; mean ± SEM reads among ACE2+ ileum cells = 10,591 ± 441, full statistics on cell depth among ACE2+ cells compared to ACE2- cells of the same cell type can be found in Table S9). Thus, we can be confident that the observed differences in ACE2+ proportions across clusters are not driven by differences in sequencing depth.\nFinally, we investigated how observed differences in ACE2+ proportions across clusters might be affected by cell sampling. Using the proportion of ACE2+ cells in a “typical” cluster annotated as being ACE2 positive (i.e., 6.8% in non-human primate type II pneumocytes, Figure 1), we calculated the cluster sizes needed to be confident that the probability of observing zero to a few positive cells is unlikely to have arisen by random chance (probabilities calculated under a negative binomial distribution with parameter p = 0.068, Figure S6E). We found that as cluster sizes approach and exceed 100 cells, the probability of observing zero to a few positive cells rapidly approaches zero, if we assume 6.8% of cells are positive. Further, to examine our confidence in estimating an approximate upper bound (ignoring the impact of protocol inefficiencies discussed above) for the fraction of cells positive in a cluster as a function of the number of cells in that cluster, we also calculated the probability of observing zero (and its complement, probability of observing at least 1) ACE2+ cells as a function of cluster size across true positive proportions ranging from 0.1% to 10% (probabilities calculated under a negative binomial distribution with parameter p = 0.001 to 0.1, representing hypothetical proportions of ACE2+ cells Figure S6F). Given our typical cluster sizes (on the order of hundreds of cells, exact values provided in Table S9), we find that for us to observe 0 ACE2+ cells in a cluster due to sampling artifacts, the fraction of true positives must be ∼1% or less. Thus, these complementary approaches demonstrate that our observed variations in ACE2+ cell proportions across clusters likely reflect underlying biological differences, rather than random chance.\n\nStatistical Testing\nParameters such as sample size, number of replicates, number of independent experiments, measures of center, dispersion, and precision (mean ± SEM) and statistical significances are reported in Figures and Figure Legends. A p value less than 0.05 was considered significant. Where appropriate, a Bonferroni or FDR correction was used to account for multiple tests, alternative correction methods are noted in the figure legends or Methods. All statistical tests corresponding to differential gene expression are described above and completed using R language for Statistical Computing."}
LitCovid-sentences
{"project":"LitCovid-sentences","denotations":[{"id":"T739","span":{"begin":0,"end":39},"obj":"Sentence"},{"id":"T740","span":{"begin":41,"end":73},"obj":"Sentence"},{"id":"T741","span":{"begin":74,"end":189},"obj":"Sentence"},{"id":"T742","span":{"begin":190,"end":385},"obj":"Sentence"},{"id":"T743","span":{"begin":386,"end":559},"obj":"Sentence"},{"id":"T744","span":{"begin":560,"end":792},"obj":"Sentence"},{"id":"T745","span":{"begin":793,"end":905},"obj":"Sentence"},{"id":"T746","span":{"begin":906,"end":1054},"obj":"Sentence"},{"id":"T747","span":{"begin":1055,"end":1290},"obj":"Sentence"},{"id":"T748","span":{"begin":1291,"end":1531},"obj":"Sentence"},{"id":"T749","span":{"begin":1532,"end":1817},"obj":"Sentence"},{"id":"T750","span":{"begin":1818,"end":1998},"obj":"Sentence"},{"id":"T751","span":{"begin":1999,"end":2222},"obj":"Sentence"},{"id":"T752","span":{"begin":2224,"end":2241},"obj":"Sentence"},{"id":"T753","span":{"begin":2242,"end":2316},"obj":"Sentence"},{"id":"T754","span":{"begin":2317,"end":2477},"obj":"Sentence"},{"id":"T755","span":{"begin":2478,"end":2651},"obj":"Sentence"},{"id":"T756","span":{"begin":2652,"end":2788},"obj":"Sentence"},{"id":"T757","span":{"begin":2789,"end":2918},"obj":"Sentence"},{"id":"T758","span":{"begin":2919,"end":3100},"obj":"Sentence"},{"id":"T759","span":{"begin":3101,"end":3262},"obj":"Sentence"},{"id":"T760","span":{"begin":3263,"end":3558},"obj":"Sentence"},{"id":"T761","span":{"begin":3559,"end":3835},"obj":"Sentence"},{"id":"T762","span":{"begin":3836,"end":4044},"obj":"Sentence"},{"id":"T763","span":{"begin":4045,"end":4252},"obj":"Sentence"},{"id":"T764","span":{"begin":4254,"end":4265},"obj":"Sentence"},{"id":"T765","span":{"begin":4266,"end":4439},"obj":"Sentence"},{"id":"T766","span":{"begin":4440,"end":4686},"obj":"Sentence"},{"id":"T767","span":{"begin":4687,"end":4799},"obj":"Sentence"},{"id":"T768","span":{"begin":4800,"end":4917},"obj":"Sentence"},{"id":"T769","span":{"begin":4918,"end":5108},"obj":"Sentence"},{"id":"T770","span":{"begin":5109,"end":5234},"obj":"Sentence"},{"id":"T771","span":{"begin":5235,"end":5548},"obj":"Sentence"},{"id":"T772","span":{"begin":5549,"end":5717},"obj":"Sentence"},{"id":"T773","span":{"begin":5718,"end":5925},"obj":"Sentence"},{"id":"T774","span":{"begin":5927,"end":5951},"obj":"Sentence"},{"id":"T775","span":{"begin":5952,"end":6048},"obj":"Sentence"},{"id":"T776","span":{"begin":6049,"end":6242},"obj":"Sentence"},{"id":"T777","span":{"begin":6243,"end":6435},"obj":"Sentence"},{"id":"T778","span":{"begin":6436,"end":6625},"obj":"Sentence"},{"id":"T779","span":{"begin":6626,"end":6889},"obj":"Sentence"},{"id":"T780","span":{"begin":6891,"end":6957},"obj":"Sentence"},{"id":"T781","span":{"begin":6958,"end":7073},"obj":"Sentence"},{"id":"T782","span":{"begin":7074,"end":7220},"obj":"Sentence"},{"id":"T783","span":{"begin":7221,"end":7336},"obj":"Sentence"},{"id":"T784","span":{"begin":7337,"end":7393},"obj":"Sentence"},{"id":"T785","span":{"begin":7394,"end":7540},"obj":"Sentence"},{"id":"T786","span":{"begin":7541,"end":7804},"obj":"Sentence"},{"id":"T787","span":{"begin":7805,"end":7905},"obj":"Sentence"},{"id":"T788","span":{"begin":7906,"end":8012},"obj":"Sentence"},{"id":"T789","span":{"begin":8014,"end":8045},"obj":"Sentence"},{"id":"T790","span":{"begin":8046,"end":8127},"obj":"Sentence"},{"id":"T791","span":{"begin":8128,"end":8308},"obj":"Sentence"},{"id":"T792","span":{"begin":8309,"end":8397},"obj":"Sentence"},{"id":"T793","span":{"begin":8398,"end":8534},"obj":"Sentence"},{"id":"T794","span":{"begin":8535,"end":8799},"obj":"Sentence"},{"id":"T795","span":{"begin":8800,"end":8892},"obj":"Sentence"},{"id":"T796","span":{"begin":8894,"end":8936},"obj":"Sentence"},{"id":"T797","span":{"begin":8937,"end":9100},"obj":"Sentence"},{"id":"T798","span":{"begin":9101,"end":9252},"obj":"Sentence"},{"id":"T799","span":{"begin":9253,"end":9426},"obj":"Sentence"},{"id":"T800","span":{"begin":9427,"end":9493},"obj":"Sentence"},{"id":"T801","span":{"begin":9494,"end":9654},"obj":"Sentence"},{"id":"T802","span":{"begin":9655,"end":9767},"obj":"Sentence"},{"id":"T803","span":{"begin":9768,"end":9953},"obj":"Sentence"},{"id":"T804","span":{"begin":9954,"end":10164},"obj":"Sentence"},{"id":"T805","span":{"begin":10165,"end":10379},"obj":"Sentence"},{"id":"T806","span":{"begin":10380,"end":10473},"obj":"Sentence"},{"id":"T807","span":{"begin":10474,"end":10712},"obj":"Sentence"},{"id":"T808","span":{"begin":10713,"end":10873},"obj":"Sentence"},{"id":"T809","span":{"begin":10874,"end":10925},"obj":"Sentence"},{"id":"T810","span":{"begin":10926,"end":11032},"obj":"Sentence"},{"id":"T811","span":{"begin":11034,"end":11079},"obj":"Sentence"},{"id":"T812","span":{"begin":11080,"end":11212},"obj":"Sentence"},{"id":"T813","span":{"begin":11213,"end":11317},"obj":"Sentence"},{"id":"T814","span":{"begin":11318,"end":11477},"obj":"Sentence"},{"id":"T815","span":{"begin":11478,"end":11622},"obj":"Sentence"},{"id":"T816","span":{"begin":11623,"end":11880},"obj":"Sentence"},{"id":"T817","span":{"begin":11881,"end":12066},"obj":"Sentence"},{"id":"T818","span":{"begin":12067,"end":12114},"obj":"Sentence"},{"id":"T819","span":{"begin":12115,"end":12353},"obj":"Sentence"},{"id":"T820","span":{"begin":12354,"end":12541},"obj":"Sentence"},{"id":"T821","span":{"begin":12542,"end":12693},"obj":"Sentence"},{"id":"T822","span":{"begin":12694,"end":12768},"obj":"Sentence"},{"id":"T823","span":{"begin":12770,"end":12809},"obj":"Sentence"},{"id":"T824","span":{"begin":12810,"end":12893},"obj":"Sentence"},{"id":"T825","span":{"begin":12894,"end":12976},"obj":"Sentence"},{"id":"T826","span":{"begin":12977,"end":13162},"obj":"Sentence"},{"id":"T827","span":{"begin":13163,"end":13324},"obj":"Sentence"},{"id":"T828","span":{"begin":13325,"end":13522},"obj":"Sentence"},{"id":"T829","span":{"begin":13523,"end":13582},"obj":"Sentence"},{"id":"T830","span":{"begin":13583,"end":13651},"obj":"Sentence"},{"id":"T831","span":{"begin":13652,"end":13738},"obj":"Sentence"},{"id":"T832","span":{"begin":13739,"end":13833},"obj":"Sentence"},{"id":"T833","span":{"begin":13834,"end":14029},"obj":"Sentence"},{"id":"T834","span":{"begin":14030,"end":14258},"obj":"Sentence"},{"id":"T835","span":{"begin":14259,"end":14469},"obj":"Sentence"},{"id":"T836","span":{"begin":14470,"end":14658},"obj":"Sentence"},{"id":"T837","span":{"begin":14659,"end":14819},"obj":"Sentence"},{"id":"T838","span":{"begin":14820,"end":15014},"obj":"Sentence"},{"id":"T839","span":{"begin":15015,"end":15088},"obj":"Sentence"},{"id":"T840","span":{"begin":15089,"end":15219},"obj":"Sentence"},{"id":"T841","span":{"begin":15220,"end":15529},"obj":"Sentence"},{"id":"T842","span":{"begin":15531,"end":15583},"obj":"Sentence"},{"id":"T843","span":{"begin":15584,"end":15841},"obj":"Sentence"},{"id":"T844","span":{"begin":15842,"end":15985},"obj":"Sentence"},{"id":"T845","span":{"begin":15986,"end":16242},"obj":"Sentence"},{"id":"T846","span":{"begin":16243,"end":16442},"obj":"Sentence"},{"id":"T847","span":{"begin":16443,"end":16809},"obj":"Sentence"},{"id":"T848","span":{"begin":16810,"end":16937},"obj":"Sentence"},{"id":"T849","span":{"begin":16938,"end":17057},"obj":"Sentence"},{"id":"T850","span":{"begin":17058,"end":17266},"obj":"Sentence"},{"id":"T851","span":{"begin":17267,"end":17776},"obj":"Sentence"},{"id":"T852","span":{"begin":17777,"end":18018},"obj":"Sentence"},{"id":"T853","span":{"begin":18019,"end":18326},"obj":"Sentence"},{"id":"T854","span":{"begin":18327,"end":18514},"obj":"Sentence"},{"id":"T855","span":{"begin":18515,"end":18694},"obj":"Sentence"},{"id":"T856","span":{"begin":18695,"end":19235},"obj":"Sentence"},{"id":"T857","span":{"begin":19236,"end":19577},"obj":"Sentence"},{"id":"T858","span":{"begin":19578,"end":19720},"obj":"Sentence"},{"id":"T859","span":{"begin":19721,"end":20005},"obj":"Sentence"},{"id":"T860","span":{"begin":20006,"end":20238},"obj":"Sentence"},{"id":"T861","span":{"begin":20239,"end":20468},"obj":"Sentence"},{"id":"T862","span":{"begin":20469,"end":20802},"obj":"Sentence"},{"id":"T863","span":{"begin":20803,"end":21334},"obj":"Sentence"},{"id":"T864","span":{"begin":21335,"end":21478},"obj":"Sentence"},{"id":"T865","span":{"begin":21479,"end":21601},"obj":"Sentence"},{"id":"T866","span":{"begin":21602,"end":22024},"obj":"Sentence"},{"id":"T867","span":{"begin":22025,"end":22210},"obj":"Sentence"},{"id":"T868","span":{"begin":22211,"end":22828},"obj":"Sentence"},{"id":"T869","span":{"begin":22829,"end":23069},"obj":"Sentence"},{"id":"T870","span":{"begin":23070,"end":23266},"obj":"Sentence"},{"id":"T871","span":{"begin":23268,"end":23287},"obj":"Sentence"},{"id":"T872","span":{"begin":23288,"end":23509},"obj":"Sentence"},{"id":"T873","span":{"begin":23510,"end":23562},"obj":"Sentence"},{"id":"T874","span":{"begin":23563,"end":23727},"obj":"Sentence"},{"id":"T875","span":{"begin":23728,"end":23873},"obj":"Sentence"}],"namespaces":[{"prefix":"_base","uri":"http://pubannotation.org/ontology/tao.owl#"}],"text":"Quantification and Statistical Analysis\n\nNon-Human Primate Lung and Ileum\nLibraries corresponding to 7 animals (variable number of tissues per animal) were sequenced using Illumina NextSeq. Reads were aligned to the M. mulatta genome assembly 8.0.1 annotation version 102 and processed according to the Drop-Seq Computational Protocol v2.0 (https://github.com/broadinstitute/Drop-seq). Data was normalized and scaled using the Seurat R package v2.3.4 (https://satijalab.org/seurat/): transforming the data to loge(UMI+1) and applying a scale factor of 10,000. To identify major axes of variation within our data, we first examined only highly variable genes across all cells, yielding approximately 1,000-3,000 variable genes with average expression \u003e 0.1 log-normalized UMI across all cells. An approximate principal component analysis was applied to the cells to generate 100 principal components (PCs). Using the JackStraw function within Seurat, we identified significant PCs to be used for subsequent clustering and further dimensionality reduction. For 2D visualization and cell type clustering, we used a Uniform Manifold Approximation and Projection (UMAP) dimensionality reduction technique (https://github.com/lmcinnes/umap) with “min_dist” set to 0.5 and “n_neighbors” set to 30. To identify clusters of transcriptionally similar cells, we employed unsupervised clustering as described above using the FindClusters tool within the Seurat R package with default parameters and k.param set to 10 and resolution set to 0.5. Each cluster was sub-clustered to identify more granular cell types, requiring each cell type to express \u003e 25 significantly upregulated genes by differential expression test (FindMarkers implemented in Seurat, setting “test.use” to “bimod,” Bonferroni-adjusted p value cutoff \u003c 0.001). Differential expression tests between cells from ACE2 + versus ACE2 - Type II Pneumocytes were conducted using the SCDE R package with default parameters (Kharchenko et al., 2014). Expression data for epithelial cells and enterocytes included in this dataset can be visualized and downloaded here: https://singlecell.broadinstitute.org/single_cell/study/SCP807?scpbr=the-alexandria-project#study-summary.\n\nHuman Lung Tissue\nLibraries corresponding to 8 donors were sequenced using Illumina NextSeq. Reads were aligned to the hg19 genome assembly and processed according to the Drop-Seq Computational Protocol v2.0 (https://github.com/broadinstitute/Drop-seq). Data was normalized and scaled using the Seurat R package v3.1.0 (https://satijalab.org/seurat/), transforming the data to loge(UMI+1) and applying a scale factor of 10,000. For each array, we assessed the quality of constructed libraries by examining the distribution of reads, genes and transcripts per cell. Variable gene selection, principal components analysis, and selection of significant principal components was performed as above. We visualized our results in a two-dimensional space using UMAP (https://github.com/lmcinnes/umap), and annotated each cluster based on the identification of highly expressed genes. To further characterize substructure within cell types (for example, T cells), we performed dimensionality reduction (PCA) and clustering over those cells alone. Sub-clusters (i.e., clusters within broad cell type classifications) were annotated by cross-referencing cluster-defining genes with curated gene lists and online databases SaVanT (http://newpathways.mcdb.ucla.edu/savant-dev/) and GSEA/MsigDB (https://www.gsea-msigdb.org/gsea/msigdb/index.jsp). Proliferating cells from the human lung (Figure 2C) express high levels of mitotic markers, such as MKI67, and represent primarily T cells (CD3D, CD3E), B cells/antibody-secreting cells (IGJ, MZB1, IGHG1), and myeloid cells (CD14, APOE) and represent a composite cell cluster. Differential expression analysis between ACE2+ TMPRSS2+ and negative type II pneumocytes was performed in Seurat using a likelihood-ratio test (FindMarkers implemented in Seurat, setting “test.use” to bimod). Expression data for epithelial cells included in this dataset can be visualized and downloaded here: https://singlecell.broadinstitute.org/single_cell/study/SCP814?scpbr=the-alexandria-project#study-summary.\n\nHuman Ileum\nLibraries corresponding to 13 donors were sequenced using Illumina NovaSeq S2 with a Read 1 26bp, Read 2 91bp, Index 1 8bp configuration before reads were aligned to GRCh38. Each sample was filtered individually for low quality cells and genes by analyzing distributions of reads, transcripts, percent reads mapped to mitochondrial genes, and complexity per cell, then merged as an outer join to create a single dataset. Clustering and differential expression tests were processed using Seurat v3.1.0 (https://satijalab.org/seurat/). Normalization and variable gene selection was processed with SCTransform (https://github.com/ChristophH/sctransform). Clustering for major cell types was performed using Louvain clustering on dimensionally reduced PCA space with resolution set via grid search optimizing for maximum average silhouette score. Due to the scale of the dataset, a randomized subsampling from across the dataset was used to calculate the silhouette score. We annotated clusters based on highly expressed genes, then sub-clusters were characterized by performing PCA dimensionality reduction and clustering over those cells alone, and annotated based on highly expressed genes found via one-versus-rest differential expression test (Wilcoxon) within the major cell type. Differential expression analysis between ACE2 + TMPRSS2 + and negative epithelial cells was performed in Seurat using a Wilcoxon test and Bonferroni p value correction. Expression data for epithelial cells included in this dataset can be visualized and downloaded here: https://singlecell.broadinstitute.org/single_cell/study/SCP812?scpbr=the-alexandria-project#study-summary.\n\nHuman Adult Nasal Mucosa\nSample processing, sequencing, and analysis was performed as in (Ordovas-Montanes et al., 2018). Briefly, scRNA-seq cell suspensions were freshly processed using Seq-Well v1 and Seurat v2.3.4 was utilized for computational analyses presented here (Butler et al., 2018, Satija et al., 2015). Cell by gene matrix and R code for initialization of object available to download as Supplemental Data and Supplementary Tables here https://www.nature.com/articles/s41586-018-0449-8 and here:\nhttp://shaleklab.com/resource/mapping-allergic-inflammation/? and visualized here: https://singlecell.broadinstitute.org/single_cell/study/SCP253?scpbr=the-alexandria-project#study-summary. Scores for various cytokines acting on human airway epithelial cells were calculated based on gene lists derived for (Ordovas-Montanes et al., 2018), calculated using AddModuleScore function Seurat, and effect size calculated by Cohen’s d, as previously reported.\n\nGranulomatous Tissue from Mycobacterium Tuberculosis Infected NHPs\nLibraries corresponding to 10 animals (variable number of tissues/animal) were sequenced using Illumina NovaSeq S2. Data was aligned using the Dropseq-tools pipeline on Terra (app.terra.bio) to M. fascicularis reference genome assembly 5, annotation version 101. Clustering was performed using Leiden clustering in the Scanpy (scanpy.readthedocs.io) package (Wolf et al., 2018). Cell type labels were assigned using known marker genes. In this analysis, we include all epithelial cell subsets (secretory, multiciliated, type II pneumocytes, and type I pneumocytes) from all samples. Differential expression between ACE2 + TMPRSS2 + cells and other cells of the matched cell subtype (e.g., Secretory Cells) were performed using the “bimod” likelihood-ratio test within each cell subtype and filtered on Benjamini-Hochberg-corrected p value \u003c 0.05. Expression data for epithelial cells included in this dataset can be visualized and downloaded here:\nhttps://singlecell.broadinstitute.org/single_cell/study/SCP806?scpbr=the-alexandria-project#study-summary.\n\nBasal Cell Cytokine Stimulation\nLibraries corresponding to 279 populations were sequenced using Illumina NextSeq. Reads were aligned to the hg19 or mm10 genome assembly using the cumulus platform https://cumulus-doc.readthedocs.io/en/0.12.0/smart_seq_2.html and output as TPM using RSEM v1.3.2. Populations were transformed to transcripts per 10K reads and log2(1+TP10K) transformed. ACE2 expression by stimulation condition and dose were assessed using one-way ANOVA with post hoc testing using a Bonferroni correction. Plots were generated using ggplot2, and transcriptome-wide differential expression was calculated using the Seurat R package v3.1.0 (https://satijalab.org/seurat), function FindMarkers with test.use = ”bimod.” Expression data can be visualized and downloaded here:\nhttps://singlecell.broadinstitute.org/single_cell/study/SCP822?scpbr=the-alexandria-project.\n\nInterferon Treatment of Mouse Nasal Mucosa\nLibraries corresponding to 4 mice, with 2 Seq-Well arrays per mouse were sequenced using Illumina NextSeq as described (Gierahn et al., 2017, Hughes et al., 2019). Reads were aligned to the mm10 genome and processed according to the Drop-Seq Computational Protocol v2.0 (https://github.com/broadinstitute/Drop-seq). Data was normalized and scaled using the Seurat R package v2.3.4 (https://satijalab.org/seurat/): transforming the data to loge(UMI+1) and applying a scale factor of 10,000. Cells with fewer than 1000 UMIs and 500 unique genes were removed. To identify major axes of variation within our data, we first examined only highly variable genes across all cells, yielding approximately 5,000 variable genes. An approximate principal component analysis was applied to the cells to generate 200 principal components (PCs). Using a combination of the Jackstraw function in Seurat and observing the “elbow” of the standard deviations of PCs, we chose the top 70 PCs for subsequent clustering and visualization. For 2D visualization, we used a Uniform Manifold Approximation and Projection (UMAP) dimensionality reduction technique (https://github.com/lmcinnes/umap) with “min_dist” set to 0.3 and “n_neighbors” set to 50. To identify clusters of transcriptionally similar cells, we employed unsupervised clustering as described above using the FindClusters tool within the Seurat R package with default parameters and k.param set to 10. Resolution was chosen based on maximization of the average silhouette width across all cells. Clusters were merged if a cell type expressed fewer than 25 significantly upregulated genes by differential expression test (FindAllMarkers implemented in Seurat, setting “test.use” to “bimod,” Bonferroni-adjusted p value cutoff \u003c 0.001). Differential expression tests between cells from saline-treated or IFNa-treated mice were assessed using the FindMarkers function with “test.use” set to “bimod. This dataset can be visualized and downloaded here:\nhttps://singlecell.broadinstitute.org/single_cell/study/SCP832?scpbr=the-alexandria-project#study-summary.\n\nLung from MHV68-Infected WT and IFNγR KO Mice\nLibraries corresponding to 14 mice were aligned to a custom reference genome encompassing both murine (mm10) and herpes virus genes: 84 known genes from MHV68 were retrieved from NCBI (NCBI: txid33708) and added to the mm10 mouse genome. Reads were aligned to the custom joint genome and processed according to the Drop-Seq Computational Protocol v2.0 (https://github.com/broadinstitute/Drop-seq). Barcodes with \u003c 200 unique genes, \u003e 20,000 UMI counts, and \u003e 30% of transcript counts derived from mitochondrially encoded genes were discarded. Data analysis was performed using the Scanpy Package following the common procedure, the expression matrices were normalized using scran’s size factor based approach and log transformed via scanpy’s pp.log1p() function (Lun et al., 2016, Wolf et al., 2018). SoupX was utilized to reduce ambient RNA bias, using default parameters with pCut set to 0.3, and was applied to each sample before merging the count matrices (Young and Behjati, 2020). UMI per cell and cell cycle were regressed out. Highly variable genes were selected by running pp.highly_variable_genes() for each sample separately, returning the top 4,000 variable genes per sample, and genes identified in variable in \u003e 5 samples were retained, yielding 14,305 genes. Next, only Epcam+ cells were considered, principal components (PCs) were calculated using only the selected variable genes, and 6 PCs were used to perform unsupervised Louvain clustering. Type I Pneumocytes were excluded from this analysis based on uniformly negative expression of Ace2, resulting in a final dataset subset of 5,558 cells. Cells were identified as infected if at least one viral read was detected.\n\nNasal Washes during Influenza Infection\nSample processing, sequencing, and analysis was performed as in (Cao et al., 2020). Reads were aligned to the GRCh37 reference genome combined with influenza genomes. Mapped reads from each sample were then corrected for Drop-seq barcode synthesis error using the Drop-seq core computational tools developed by the McCarroll Lab (Macosko et al., 2015). Genes were quantified using End Sequence Analysis Toolkit (ESAT, github/garber-lab/ESAT) with parameters -wlen 100 -wOlap 50 -wExt 0 -scPrep (Derr et al., 2016). Finally, UMIs that likely result from sequencing errors were corrected by merging any UMIs that were observed only once and have 1 hamming distance from a UMI detected by two or more aligned reads. Only cell barcodes with more than 1,000 UMIs were analyzed. Cell barcodes with mostly erythrocyte genes (HBA, HBB) were removed. From here on, the remaining cell barcodes in the matrix would be referred to as cells. The final gene by cell matrix was normalized using the scran package v3.10 (Lun et al., 2016). The normalized matrix was used for dimensionality reduction by first selecting variable genes that had a high coefficient of variance (CV) and were expressed (\u003e = 1 UMI) by more than three cells. Influenza viral genes, interferon stimulated genes, and cell cycle related genes were removed from the variable gene list in order to minimize the impact of viral responses and mitosis on clustering and cell type identification. This resulted in the selection of 2484 variable genes. t-distributed stochastic neighbor embedding (tSNE) was applied to the first ten principal components (PCs), which explained 95% of the total data variance. Density clustering (Rodriguez and Laio, 2014) was performed on the resulting tSNE coordinates and identified four major clusters: epithelial cells, neutrophils, macrophages and leukocytes. The epithelial cell cluster and the leukocyte cluster were then re-clustered independently, as described above, to identify populations within each metacluster. Specifically, the epithelial cell cluster was re-embedded using 2629 variable genes selected by the same criteria mentioned in the previous section and 13 PCs that explained 95% of the variance. Density clustering over the epithelial cell subset revealed ten clusters. Differential gene expression analysis using edgeR (Robinson et al., 2010) was performed to identify marker genes for each cluster. Influenza-infected and bystander cells were identified after correcting for sample-specific distribution of ambient influenza mRNA contamination and predicted cells most likely to be infected identified using a hurdle zero inflated negative binomial (ZINB) model and a support vector machine (SVM) classifier.\n\nPower Calculations for Detection of Rare Transcripts\nWe conducted the following statistical analysis to estimate the effects of various factors on our ability to make confident claims regarding the presence/absence of transcripts of interest (e.g., ACE2), both within individual cells and clusters (Figure S6). Specifically, we investigated the roles of capture/reverse transcription efficiency, ACE2 expression level, sequencing depth, and cell numbers. Taken together, the results of this power analysis are in agreement with other efforts to model biological and technical sources of zero-inflation within scRNA-seq data (e.g., https://satijalab.org/howmanycells and Kharchenko et al., 2014, Svensson, 2020).\nWe began by quantifying how likely we are to capture and transcribe at least one ACE2 mRNA molecule, as a function of the number ACE2 mRNA molecules per cell and a protocol’s efficiency (Figure S6A). Drop-Seq has a capture/transcription efficiency of ∼10% (as estimated using ERCC spike ins; see (Macosko et al., 2015), and the experimental platforms used in this study are either equivalent (e.g., Seq-Well v1, (Gierahn et al., 2017) or superior (e.g., 10-fold better unique molecule detection, 5-fold better gene detection using Seq-Well S3;(Hughes et al., 2019)). Most relevant to this context, inferior turbinate scrapings were processed using both Seq-Well v1 and Seq-Well S3 (Figure S3B). Importantly, Seq-Well S3 provided \u003e two-fold increase in the detection frequency of rare ACE2 transcripts (i.e., ACE2+: 4.7% for v1 versus 9.8% for S3), making it reasonable to expect that such improvements in single-cell experimental technologies have yielded corresponding improvements in capture and transcription efficiency. Based on Drop-Seq’s 10% efficiency, even if ACE2 is expressed at the low level of 5 mRNA molecules per cell (a reasonable order-of-magnitude estimate, given that non-human primate ileum cells had a maximum of 10 ACE2 unique molecules per cell observed via sequencing and an average of 1.93 molecules per cell in expressing cells, see Figures 3B and 3C), our experimental platforms have a minimum likelihood of 41% to capture and reverse transcribe at least one ACE2 mRNA molecule in any given individual cell. This likelihood rapidly increases if we estimate higher efficiencies for improved scRNA-seq technologies (e.g., 67% likelihood within any individual cell at 20% capture/transcription efficiency, 76% likelihood at 25% efficiency, Figure S6A). Thus, while transcript drop-out may reduce the fraction of positive cells, with the capture and transcription efficiencies of improved single-cell technologies, the impact is likely to be minor (reads are likely underestimated by up to a factor of ∼2.5x), given a sufficient depth of sequencing (see below). We note that this impacts both clusters deemed to contain and not contain ACE2+ cells, and suggests our percentages are likely lower bounds for true expression (within a factor of ∼2.5x).\nNext, we examined the probability of sequencing an ACE2 transcript as a function of read depth and ACE2’s fractional abundance in each single cell within our sequencing libraries. First, across two different tissues (non-human primate ileum and lung, representing a high expresser of ACE2 and low expresser, respectively), we calculated the proportion of unique ACE2 molecules in our ACE2+ cells (defined as any cell with at least 1 UMI aligning to ACE2) as a fraction of total reads within individual cells to provide an order-of-magnitude estimate for average ACE2 abundance in our single-cell sequencing libraries (i.e., the probability that a read within a cell corresponds to a unique molecule of ACE2, Figure S6B). We highlight that by calculating probabilities based on ACE2 unique molecules divided by an individual cell’s total reads, we are providing a conservative estimate for the probability of observing ACE2 as a function of sequencing depth (e.g., as compared to basing these probabilities on ACE2 non-UMI-collapsed reads divided by total reads). Next, we obtained information on the number of reads in these cell populations to provide estimates of average sequencing depths (Figure S6C). Using the mean fractional abundances of ACE2 from each tissue (Figure S6B) and the mean read depths for all genes (Figure S6C), we calculated the probability of detecting at least 1 ACE2 molecule (i.e., P(detecting \u003e 0 ACE2 molecules) = 1 - (1 - ACE2 fractional abundance)Read depth). This results in a 93.7% probability in ileum-derived cell libraries that contain ACE2, and a 76.0% probability for lung-derived cell libraries, indicating that our sequencing depths are sufficient to detect ACE2+ cells (Figure S6D).\nTo further evaluate whether our ability to detect ACE2+ cells was an artifact of sequencing depth, we compared the number of ACE2+ cells in a cluster to the mean number of reads across all cells in that same cluster (Figure S6E). We did not observe any significant correlation: the ileum cell cluster with the highest number of ACE2+ cells had the lowest sequencing depth of all ileum clusters, and the lung cell cluster with the highest number of ACE2+ cells was approximately average in its read depth (on a log-log scale, Pearson’s r = −0.31, non-significant). Further, when comparing ACE2+ cells to ACE2- cells within a given tissue, we did not observe a positive correlation between read depth and ACE2 status (i.e., mean ± standard error of the mean, SEM, reads among all lung cells = 28,512 ± 344; mean ± SEM reads among ACE2+ lung cells = 28,553 ± 2,988; mean ± SEM reads among all ileum cells = 14,864 ± 288; mean ± SEM reads among ACE2+ ileum cells = 10,591 ± 441, full statistics on cell depth among ACE2+ cells compared to ACE2- cells of the same cell type can be found in Table S9). Thus, we can be confident that the observed differences in ACE2+ proportions across clusters are not driven by differences in sequencing depth.\nFinally, we investigated how observed differences in ACE2+ proportions across clusters might be affected by cell sampling. Using the proportion of ACE2+ cells in a “typical” cluster annotated as being ACE2 positive (i.e., 6.8% in non-human primate type II pneumocytes, Figure 1), we calculated the cluster sizes needed to be confident that the probability of observing zero to a few positive cells is unlikely to have arisen by random chance (probabilities calculated under a negative binomial distribution with parameter p = 0.068, Figure S6E). We found that as cluster sizes approach and exceed 100 cells, the probability of observing zero to a few positive cells rapidly approaches zero, if we assume 6.8% of cells are positive. Further, to examine our confidence in estimating an approximate upper bound (ignoring the impact of protocol inefficiencies discussed above) for the fraction of cells positive in a cluster as a function of the number of cells in that cluster, we also calculated the probability of observing zero (and its complement, probability of observing at least 1) ACE2+ cells as a function of cluster size across true positive proportions ranging from 0.1% to 10% (probabilities calculated under a negative binomial distribution with parameter p = 0.001 to 0.1, representing hypothetical proportions of ACE2+ cells Figure S6F). Given our typical cluster sizes (on the order of hundreds of cells, exact values provided in Table S9), we find that for us to observe 0 ACE2+ cells in a cluster due to sampling artifacts, the fraction of true positives must be ∼1% or less. Thus, these complementary approaches demonstrate that our observed variations in ACE2+ cell proportions across clusters likely reflect underlying biological differences, rather than random chance.\n\nStatistical Testing\nParameters such as sample size, number of replicates, number of independent experiments, measures of center, dispersion, and precision (mean ± SEM) and statistical significances are reported in Figures and Figure Legends. A p value less than 0.05 was considered significant. Where appropriate, a Bonferroni or FDR correction was used to account for multiple tests, alternative correction methods are noted in the figure legends or Methods. All statistical tests corresponding to differential gene expression are described above and completed using R language for Statistical Computing."}
LitCovid-PubTator
{"project":"LitCovid-PubTator","denotations":[{"id":"2691","span":{"begin":45,"end":50},"obj":"Species"},{"id":"2695","span":{"begin":1867,"end":1871},"obj":"Gene"},{"id":"2696","span":{"begin":1881,"end":1885},"obj":"Gene"},{"id":"2697","span":{"begin":216,"end":226},"obj":"Species"},{"id":"2699","span":{"begin":2224,"end":2229},"obj":"Species"},{"id":"2715","span":{"begin":3659,"end":3664},"obj":"Gene"},{"id":"2716","span":{"begin":3699,"end":3703},"obj":"Gene"},{"id":"2717","span":{"begin":3705,"end":3709},"obj":"Gene"},{"id":"2718","span":{"begin":3746,"end":3749},"obj":"Gene"},{"id":"2719","span":{"begin":3751,"end":3755},"obj":"Gene"},{"id":"2720","span":{"begin":3757,"end":3762},"obj":"Gene"},{"id":"2721","span":{"begin":3790,"end":3794},"obj":"Gene"},{"id":"2722","span":{"begin":3877,"end":3881},"obj":"Gene"},{"id":"2723","span":{"begin":3883,"end":3890},"obj":"Gene"},{"id":"2724","span":{"begin":3588,"end":3593},"obj":"Species"},{"id":"2725","span":{"begin":3942,"end":3948},"obj":"Chemical"},{"id":"2726","span":{"begin":4007,"end":4013},"obj":"Chemical"},{"id":"2727","span":{"begin":4037,"end":4042},"obj":"Chemical"},{"id":"2728","span":{"begin":3499,"end":3505},"obj":"Disease"},{"id":"2729","span":{"begin":3519,"end":3546},"obj":"Disease"},{"id":"2731","span":{"begin":4254,"end":4259},"obj":"Species"},{"id":"2735","span":{"begin":5590,"end":5594},"obj":"Gene"},{"id":"2736","span":{"begin":5597,"end":5604},"obj":"Gene"},{"id":"2737","span":{"begin":4970,"end":4977},"obj":"Chemical"},{"id":"2739","span":{"begin":5927,"end":5932},"obj":"Species"},{"id":"2743","span":{"begin":6575,"end":6578},"obj":"Gene"},{"id":"2744","span":{"begin":6665,"end":6670},"obj":"Species"},{"id":"2745","span":{"begin":6474,"end":6495},"obj":"Disease"},{"id":"2747","span":{"begin":6931,"end":6957},"obj":"Disease"},{"id":"2751","span":{"begin":7573,"end":7577},"obj":"Gene"},{"id":"2752","span":{"begin":7580,"end":7587},"obj":"Gene"},{"id":"2753","span":{"begin":7152,"end":7167},"obj":"Species"},{"id":"2755","span":{"begin":7962,"end":7965},"obj":"Gene"},{"id":"2757","span":{"begin":8398,"end":8402},"obj":"Gene"},{"id":"2759","span":{"begin":8856,"end":8859},"obj":"Gene"},{"id":"2762","span":{"begin":8894,"end":8904},"obj":"Gene"},{"id":"2763","span":{"begin":8918,"end":8923},"obj":"Species"},{"id":"2768","span":{"begin":10780,"end":10784},"obj":"Gene"},{"id":"2769","span":{"begin":8966,"end":8970},"obj":"Species"},{"id":"2770","span":{"begin":8999,"end":9004},"obj":"Species"},{"id":"2771","span":{"begin":10793,"end":10797},"obj":"Species"},{"id":"2773","span":{"begin":10982,"end":10985},"obj":"Gene"},{"id":"2775","span":{"begin":11044,"end":11061},"obj":"Disease"},{"id":"2783","span":{"begin":12365,"end":12370},"obj":"Gene"},{"id":"2784","span":{"begin":12636,"end":12640},"obj":"Gene"},{"id":"2785","span":{"begin":11110,"end":11114},"obj":"Species"},{"id":"2786","span":{"begin":11175,"end":11181},"obj":"Species"},{"id":"2787","span":{"begin":11233,"end":11238},"obj":"Species"},{"id":"2788","span":{"begin":11304,"end":11309},"obj":"Species"},{"id":"2789","span":{"begin":12719,"end":12727},"obj":"Disease"},{"id":"2792","span":{"begin":12790,"end":12799},"obj":"Species"},{"id":"2793","span":{"begin":12800,"end":12809},"obj":"Disease"},{"id":"2802","span":{"begin":14053,"end":14063},"obj":"Gene"},{"id":"2803","span":{"begin":13633,"end":13636},"obj":"Gene"},{"id":"2804","span":{"begin":12958,"end":12967},"obj":"Species"},{"id":"2805","span":{"begin":14030,"end":14039},"obj":"Species"},{"id":"2806","span":{"begin":15220,"end":15229},"obj":"Species"},{"id":"2807","span":{"begin":15336,"end":15345},"obj":"Species"},{"id":"2808","span":{"begin":15230,"end":15238},"obj":"Disease"},{"id":"2809","span":{"begin":15403,"end":15411},"obj":"Disease"},{"id":"2812","span":{"begin":15780,"end":15784},"obj":"Gene"},{"id":"2813","span":{"begin":15927,"end":15931},"obj":"Gene"},{"id":"2824","span":{"begin":16324,"end":16328},"obj":"Gene"},{"id":"2825","span":{"begin":16372,"end":16376},"obj":"Gene"},{"id":"2826","span":{"begin":17027,"end":17031},"obj":"Gene"},{"id":"2827","span":{"begin":17051,"end":17055},"obj":"Gene"},{"id":"2828","span":{"begin":17311,"end":17315},"obj":"Gene"},{"id":"2829","span":{"begin":17479,"end":17483},"obj":"Gene"},{"id":"2830","span":{"begin":17728,"end":17732},"obj":"Gene"},{"id":"2831","span":{"begin":18401,"end":18405},"obj":"Gene"},{"id":"2832","span":{"begin":16524,"end":16529},"obj":"Gene"},{"id":"2833","span":{"begin":17433,"end":17438},"obj":"Species"},{"id":"2852","span":{"begin":18566,"end":18570},"obj":"Gene"},{"id":"2853","span":{"begin":18614,"end":18618},"obj":"Gene"},{"id":"2854","span":{"begin":18799,"end":18803},"obj":"Gene"},{"id":"2855","span":{"begin":18877,"end":18881},"obj":"Gene"},{"id":"2856","span":{"begin":18899,"end":18903},"obj":"Gene"},{"id":"2857","span":{"begin":18964,"end":18968},"obj":"Gene"},{"id":"2858","span":{"begin":19077,"end":19081},"obj":"Gene"},{"id":"2859","span":{"begin":19217,"end":19221},"obj":"Gene"},{"id":"2860","span":{"begin":19292,"end":19296},"obj":"Gene"},{"id":"2861","span":{"begin":19433,"end":19437},"obj":"Gene"},{"id":"2862","span":{"begin":19524,"end":19528},"obj":"Gene"},{"id":"2863","span":{"begin":19761,"end":19765},"obj":"Gene"},{"id":"2864","span":{"begin":19903,"end":19907},"obj":"Gene"},{"id":"2865","span":{"begin":19940,"end":19944},"obj":"Gene"},{"id":"2866","span":{"begin":19967,"end":19971},"obj":"Gene"},{"id":"2867","span":{"begin":20087,"end":20091},"obj":"Gene"},{"id":"2868","span":{"begin":20213,"end":20217},"obj":"Gene"},{"id":"2869","span":{"begin":18736,"end":18741},"obj":"Species"},{"id":"2882","span":{"begin":20289,"end":20293},"obj":"Gene"},{"id":"2883","span":{"begin":20364,"end":20368},"obj":"Gene"},{"id":"2884","span":{"begin":20567,"end":20571},"obj":"Gene"},{"id":"2885","span":{"begin":20687,"end":20691},"obj":"Gene"},{"id":"2886","span":{"begin":20827,"end":20831},"obj":"Gene"},{"id":"2887","span":{"begin":20842,"end":20846},"obj":"Gene"},{"id":"2888","span":{"begin":20942,"end":20946},"obj":"Gene"},{"id":"2889","span":{"begin":21067,"end":21071},"obj":"Gene"},{"id":"2890","span":{"begin":21180,"end":21184},"obj":"Gene"},{"id":"2891","span":{"begin":21250,"end":21254},"obj":"Gene"},{"id":"2892","span":{"begin":21274,"end":21278},"obj":"Gene"},{"id":"2893","span":{"begin":21394,"end":21398},"obj":"Gene"},{"id":"2902","span":{"begin":21532,"end":21536},"obj":"Gene"},{"id":"2903","span":{"begin":21626,"end":21630},"obj":"Gene"},{"id":"2904","span":{"begin":21680,"end":21684},"obj":"Gene"},{"id":"2905","span":{"begin":22565,"end":22569},"obj":"Gene"},{"id":"2906","span":{"begin":22804,"end":22808},"obj":"Gene"},{"id":"2907","span":{"begin":22966,"end":22970},"obj":"Gene"},{"id":"2908","span":{"begin":23151,"end":23155},"obj":"Gene"},{"id":"2909","span":{"begin":21713,"end":21718},"obj":"Species"}],"attributes":[{"id":"A2691","pred":"tao:has_database_id","subj":"2691","obj":"Tax:9606"},{"id":"A2695","pred":"tao:has_database_id","subj":"2695","obj":"Gene:59272"},{"id":"A2696","pred":"tao:has_database_id","subj":"2696","obj":"Gene:59272"},{"id":"A2697","pred":"tao:has_database_id","subj":"2697","obj":"Tax:9544"},{"id":"A2699","pred":"tao:has_database_id","subj":"2699","obj":"Tax:9606"},{"id":"A2715","pred":"tao:has_database_id","subj":"2715","obj":"Gene:4288"},{"id":"A2716","pred":"tao:has_database_id","subj":"2716","obj":"Gene:915"},{"id":"A2717","pred":"tao:has_database_id","subj":"2717","obj":"Gene:916"},{"id":"A2718","pred":"tao:has_database_id","subj":"2718","obj":"Gene:3512"},{"id":"A2719","pred":"tao:has_database_id","subj":"2719","obj":"Gene:51237"},{"id":"A2720","pred":"tao:has_database_id","subj":"2720","obj":"Gene:3500"},{"id":"A2721","pred":"tao:has_database_id","subj":"2721","obj":"Gene:348"},{"id":"A2722","pred":"tao:has_database_id","subj":"2722","obj":"Gene:59272"},{"id":"A2723","pred":"tao:has_database_id","subj":"2723","obj":"Gene:7113"},{"id":"A2724","pred":"tao:has_database_id","subj":"2724","obj":"Tax:9606"},{"id":"A2731","pred":"tao:has_database_id","subj":"2731","obj":"Tax:9606"},{"id":"A2735","pred":"tao:has_database_id","subj":"2735","obj":"Gene:59272"},{"id":"A2736","pred":"tao:has_database_id","subj":"2736","obj":"Gene:7113"},{"id":"A2739","pred":"tao:has_database_id","subj":"2739","obj":"Tax:9606"},{"id":"A2743","pred":"tao:has_database_id","subj":"2743","obj":"Gene:114131"},{"id":"A2744","pred":"tao:has_database_id","subj":"2744","obj":"Tax:9606"},{"id":"A2745","pred":"tao:has_database_id","subj":"2745","obj":"MESH:D007249"},{"id":"A2747","pred":"tao:has_database_id","subj":"2747","obj":"MESH:D007239"},{"id":"A2751","pred":"tao:has_database_id","subj":"2751","obj":"Gene:59272"},{"id":"A2752","pred":"tao:has_database_id","subj":"2752","obj":"Gene:7113"},{"id":"A2753","pred":"tao:has_database_id","subj":"2753","obj":"Tax:9541"},{"id":"A2755","pred":"tao:has_database_id","subj":"2755","obj":"Gene:114131"},{"id":"A2757","pred":"tao:has_database_id","subj":"2757","obj":"Gene:59272"},{"id":"A2759","pred":"tao:has_database_id","subj":"2759","obj":"Gene:114131"},{"id":"A2762","pred":"tao:has_database_id","subj":"2762","obj":"Gene:3439"},{"id":"A2763","pred":"tao:has_database_id","subj":"2763","obj":"Tax:10090"},{"id":"A2768","pred":"tao:has_database_id","subj":"2768","obj":"Gene:111654"},{"id":"A2769","pred":"tao:has_database_id","subj":"2769","obj":"Tax:10090"},{"id":"A2770","pred":"tao:has_database_id","subj":"2770","obj":"Tax:10090"},{"id":"A2771","pred":"tao:has_database_id","subj":"2771","obj":"Tax:10090"},{"id":"A2773","pred":"tao:has_database_id","subj":"2773","obj":"Gene:114131"},{"id":"A2775","pred":"tao:has_database_id","subj":"2775","obj":"MESH:D007239"},{"id":"A2783","pred":"tao:has_database_id","subj":"2783","obj":"Gene:4072"},{"id":"A2784","pred":"tao:has_database_id","subj":"2784","obj":"Gene:59272"},{"id":"A2785","pred":"tao:has_database_id","subj":"2785","obj":"Tax:10090"},{"id":"A2786","pred":"tao:has_database_id","subj":"2786","obj":"Tax:10090"},{"id":"A2787","pred":"tao:has_database_id","subj":"2787","obj":"Tax:1440122"},{"id":"A2788","pred":"tao:has_database_id","subj":"2788","obj":"Tax:10090"},{"id":"A2789","pred":"tao:has_database_id","subj":"2789","obj":"MESH:D007239"},{"id":"A2792","pred":"tao:has_database_id","subj":"2792","obj":"Tax:11520"},{"id":"A2793","pred":"tao:has_database_id","subj":"2793","obj":"MESH:D007239"},{"id":"A2802","pred":"tao:has_database_id","subj":"2802","obj":"Gene:3439"},{"id":"A2803","pred":"tao:has_database_id","subj":"2803","obj":"Gene:3043"},{"id":"A2804","pred":"tao:has_database_id","subj":"2804","obj":"Tax:11520"},{"id":"A2805","pred":"tao:has_database_id","subj":"2805","obj":"Tax:11520"},{"id":"A2806","pred":"tao:has_database_id","subj":"2806","obj":"Tax:11520"},{"id":"A2807","pred":"tao:has_database_id","subj":"2807","obj":"Tax:11520"},{"id":"A2808","pred":"tao:has_database_id","subj":"2808","obj":"MESH:D007239"},{"id":"A2809","pred":"tao:has_database_id","subj":"2809","obj":"MESH:D007239"},{"id":"A2812","pred":"tao:has_database_id","subj":"2812","obj":"Gene:59272"},{"id":"A2813","pred":"tao:has_database_id","subj":"2813","obj":"Gene:59272"},{"id":"A2824","pred":"tao:has_database_id","subj":"2824","obj":"Gene:59272"},{"id":"A2825","pred":"tao:has_database_id","subj":"2825","obj":"Gene:59272"},{"id":"A2826","pred":"tao:has_database_id","subj":"2826","obj":"Gene:59272"},{"id":"A2827","pred":"tao:has_database_id","subj":"2827","obj":"Gene:59272"},{"id":"A2828","pred":"tao:has_database_id","subj":"2828","obj":"Gene:59272"},{"id":"A2829","pred":"tao:has_database_id","subj":"2829","obj":"Gene:59272"},{"id":"A2830","pred":"tao:has_database_id","subj":"2830","obj":"Gene:59272"},{"id":"A2831","pred":"tao:has_database_id","subj":"2831","obj":"Gene:59272"},{"id":"A2832","pred":"tao:has_database_id","subj":"2832","obj":"Gene:43740568"},{"id":"A2833","pred":"tao:has_database_id","subj":"2833","obj":"Tax:9606"},{"id":"A2852","pred":"tao:has_database_id","subj":"2852","obj":"Gene:59272"},{"id":"A2853","pred":"tao:has_database_id","subj":"2853","obj":"Gene:59272"},{"id":"A2854","pred":"tao:has_database_id","subj":"2854","obj":"Gene:59272"},{"id":"A2855","pred":"tao:has_database_id","subj":"2855","obj":"Gene:59272"},{"id":"A2856","pred":"tao:has_database_id","subj":"2856","obj":"Gene:59272"},{"id":"A2857","pred":"tao:has_database_id","subj":"2857","obj":"Gene:59272"},{"id":"A2858","pred":"tao:has_database_id","subj":"2858","obj":"Gene:59272"},{"id":"A2859","pred":"tao:has_database_id","subj":"2859","obj":"Gene:59272"},{"id":"A2860","pred":"tao:has_database_id","subj":"2860","obj":"Gene:59272"},{"id":"A2861","pred":"tao:has_database_id","subj":"2861","obj":"Gene:59272"},{"id":"A2862","pred":"tao:has_database_id","subj":"2862","obj":"Gene:59272"},{"id":"A2863","pred":"tao:has_database_id","subj":"2863","obj":"Gene:59272"},{"id":"A2864","pred":"tao:has_database_id","subj":"2864","obj":"Gene:59272"},{"id":"A2865","pred":"tao:has_database_id","subj":"2865","obj":"Gene:59272"},{"id":"A2866","pred":"tao:has_database_id","subj":"2866","obj":"Gene:59272"},{"id":"A2867","pred":"tao:has_database_id","subj":"2867","obj":"Gene:59272"},{"id":"A2868","pred":"tao:has_database_id","subj":"2868","obj":"Gene:59272"},{"id":"A2869","pred":"tao:has_database_id","subj":"2869","obj":"Tax:9606"},{"id":"A2882","pred":"tao:has_database_id","subj":"2882","obj":"Gene:59272"},{"id":"A2883","pred":"tao:has_database_id","subj":"2883","obj":"Gene:59272"},{"id":"A2884","pred":"tao:has_database_id","subj":"2884","obj":"Gene:59272"},{"id":"A2885","pred":"tao:has_database_id","subj":"2885","obj":"Gene:59272"},{"id":"A2886","pred":"tao:has_database_id","subj":"2886","obj":"Gene:59272"},{"id":"A2887","pred":"tao:has_database_id","subj":"2887","obj":"Gene:59272"},{"id":"A2888","pred":"tao:has_database_id","subj":"2888","obj":"Gene:59272"},{"id":"A2889","pred":"tao:has_database_id","subj":"2889","obj":"Gene:59272"},{"id":"A2890","pred":"tao:has_database_id","subj":"2890","obj":"Gene:59272"},{"id":"A2891","pred":"tao:has_database_id","subj":"2891","obj":"Gene:59272"},{"id":"A2892","pred":"tao:has_database_id","subj":"2892","obj":"Gene:59272"},{"id":"A2893","pred":"tao:has_database_id","subj":"2893","obj":"Gene:59272"},{"id":"A2902","pred":"tao:has_database_id","subj":"2902","obj":"Gene:59272"},{"id":"A2903","pred":"tao:has_database_id","subj":"2903","obj":"Gene:59272"},{"id":"A2904","pred":"tao:has_database_id","subj":"2904","obj":"Gene:59272"},{"id":"A2905","pred":"tao:has_database_id","subj":"2905","obj":"Gene:59272"},{"id":"A2906","pred":"tao:has_database_id","subj":"2906","obj":"Gene:59272"},{"id":"A2907","pred":"tao:has_database_id","subj":"2907","obj":"Gene:59272"},{"id":"A2908","pred":"tao:has_database_id","subj":"2908","obj":"Gene:59272"},{"id":"A2909","pred":"tao:has_database_id","subj":"2909","obj":"Tax:9606"}],"namespaces":[{"prefix":"Tax","uri":"https://www.ncbi.nlm.nih.gov/taxonomy/"},{"prefix":"MESH","uri":"https://id.nlm.nih.gov/mesh/"},{"prefix":"Gene","uri":"https://www.ncbi.nlm.nih.gov/gene/"},{"prefix":"CVCL","uri":"https://web.expasy.org/cellosaurus/CVCL_"}],"text":"Quantification and Statistical Analysis\n\nNon-Human Primate Lung and Ileum\nLibraries corresponding to 7 animals (variable number of tissues per animal) were sequenced using Illumina NextSeq. Reads were aligned to the M. mulatta genome assembly 8.0.1 annotation version 102 and processed according to the Drop-Seq Computational Protocol v2.0 (https://github.com/broadinstitute/Drop-seq). Data was normalized and scaled using the Seurat R package v2.3.4 (https://satijalab.org/seurat/): transforming the data to loge(UMI+1) and applying a scale factor of 10,000. To identify major axes of variation within our data, we first examined only highly variable genes across all cells, yielding approximately 1,000-3,000 variable genes with average expression \u003e 0.1 log-normalized UMI across all cells. An approximate principal component analysis was applied to the cells to generate 100 principal components (PCs). Using the JackStraw function within Seurat, we identified significant PCs to be used for subsequent clustering and further dimensionality reduction. For 2D visualization and cell type clustering, we used a Uniform Manifold Approximation and Projection (UMAP) dimensionality reduction technique (https://github.com/lmcinnes/umap) with “min_dist” set to 0.5 and “n_neighbors” set to 30. To identify clusters of transcriptionally similar cells, we employed unsupervised clustering as described above using the FindClusters tool within the Seurat R package with default parameters and k.param set to 10 and resolution set to 0.5. Each cluster was sub-clustered to identify more granular cell types, requiring each cell type to express \u003e 25 significantly upregulated genes by differential expression test (FindMarkers implemented in Seurat, setting “test.use” to “bimod,” Bonferroni-adjusted p value cutoff \u003c 0.001). Differential expression tests between cells from ACE2 + versus ACE2 - Type II Pneumocytes were conducted using the SCDE R package with default parameters (Kharchenko et al., 2014). Expression data for epithelial cells and enterocytes included in this dataset can be visualized and downloaded here: https://singlecell.broadinstitute.org/single_cell/study/SCP807?scpbr=the-alexandria-project#study-summary.\n\nHuman Lung Tissue\nLibraries corresponding to 8 donors were sequenced using Illumina NextSeq. Reads were aligned to the hg19 genome assembly and processed according to the Drop-Seq Computational Protocol v2.0 (https://github.com/broadinstitute/Drop-seq). Data was normalized and scaled using the Seurat R package v3.1.0 (https://satijalab.org/seurat/), transforming the data to loge(UMI+1) and applying a scale factor of 10,000. For each array, we assessed the quality of constructed libraries by examining the distribution of reads, genes and transcripts per cell. Variable gene selection, principal components analysis, and selection of significant principal components was performed as above. We visualized our results in a two-dimensional space using UMAP (https://github.com/lmcinnes/umap), and annotated each cluster based on the identification of highly expressed genes. To further characterize substructure within cell types (for example, T cells), we performed dimensionality reduction (PCA) and clustering over those cells alone. Sub-clusters (i.e., clusters within broad cell type classifications) were annotated by cross-referencing cluster-defining genes with curated gene lists and online databases SaVanT (http://newpathways.mcdb.ucla.edu/savant-dev/) and GSEA/MsigDB (https://www.gsea-msigdb.org/gsea/msigdb/index.jsp). Proliferating cells from the human lung (Figure 2C) express high levels of mitotic markers, such as MKI67, and represent primarily T cells (CD3D, CD3E), B cells/antibody-secreting cells (IGJ, MZB1, IGHG1), and myeloid cells (CD14, APOE) and represent a composite cell cluster. Differential expression analysis between ACE2+ TMPRSS2+ and negative type II pneumocytes was performed in Seurat using a likelihood-ratio test (FindMarkers implemented in Seurat, setting “test.use” to bimod). Expression data for epithelial cells included in this dataset can be visualized and downloaded here: https://singlecell.broadinstitute.org/single_cell/study/SCP814?scpbr=the-alexandria-project#study-summary.\n\nHuman Ileum\nLibraries corresponding to 13 donors were sequenced using Illumina NovaSeq S2 with a Read 1 26bp, Read 2 91bp, Index 1 8bp configuration before reads were aligned to GRCh38. Each sample was filtered individually for low quality cells and genes by analyzing distributions of reads, transcripts, percent reads mapped to mitochondrial genes, and complexity per cell, then merged as an outer join to create a single dataset. Clustering and differential expression tests were processed using Seurat v3.1.0 (https://satijalab.org/seurat/). Normalization and variable gene selection was processed with SCTransform (https://github.com/ChristophH/sctransform). Clustering for major cell types was performed using Louvain clustering on dimensionally reduced PCA space with resolution set via grid search optimizing for maximum average silhouette score. Due to the scale of the dataset, a randomized subsampling from across the dataset was used to calculate the silhouette score. We annotated clusters based on highly expressed genes, then sub-clusters were characterized by performing PCA dimensionality reduction and clustering over those cells alone, and annotated based on highly expressed genes found via one-versus-rest differential expression test (Wilcoxon) within the major cell type. Differential expression analysis between ACE2 + TMPRSS2 + and negative epithelial cells was performed in Seurat using a Wilcoxon test and Bonferroni p value correction. Expression data for epithelial cells included in this dataset can be visualized and downloaded here: https://singlecell.broadinstitute.org/single_cell/study/SCP812?scpbr=the-alexandria-project#study-summary.\n\nHuman Adult Nasal Mucosa\nSample processing, sequencing, and analysis was performed as in (Ordovas-Montanes et al., 2018). Briefly, scRNA-seq cell suspensions were freshly processed using Seq-Well v1 and Seurat v2.3.4 was utilized for computational analyses presented here (Butler et al., 2018, Satija et al., 2015). Cell by gene matrix and R code for initialization of object available to download as Supplemental Data and Supplementary Tables here https://www.nature.com/articles/s41586-018-0449-8 and here:\nhttp://shaleklab.com/resource/mapping-allergic-inflammation/? and visualized here: https://singlecell.broadinstitute.org/single_cell/study/SCP253?scpbr=the-alexandria-project#study-summary. Scores for various cytokines acting on human airway epithelial cells were calculated based on gene lists derived for (Ordovas-Montanes et al., 2018), calculated using AddModuleScore function Seurat, and effect size calculated by Cohen’s d, as previously reported.\n\nGranulomatous Tissue from Mycobacterium Tuberculosis Infected NHPs\nLibraries corresponding to 10 animals (variable number of tissues/animal) were sequenced using Illumina NovaSeq S2. Data was aligned using the Dropseq-tools pipeline on Terra (app.terra.bio) to M. fascicularis reference genome assembly 5, annotation version 101. Clustering was performed using Leiden clustering in the Scanpy (scanpy.readthedocs.io) package (Wolf et al., 2018). Cell type labels were assigned using known marker genes. In this analysis, we include all epithelial cell subsets (secretory, multiciliated, type II pneumocytes, and type I pneumocytes) from all samples. Differential expression between ACE2 + TMPRSS2 + cells and other cells of the matched cell subtype (e.g., Secretory Cells) were performed using the “bimod” likelihood-ratio test within each cell subtype and filtered on Benjamini-Hochberg-corrected p value \u003c 0.05. Expression data for epithelial cells included in this dataset can be visualized and downloaded here:\nhttps://singlecell.broadinstitute.org/single_cell/study/SCP806?scpbr=the-alexandria-project#study-summary.\n\nBasal Cell Cytokine Stimulation\nLibraries corresponding to 279 populations were sequenced using Illumina NextSeq. Reads were aligned to the hg19 or mm10 genome assembly using the cumulus platform https://cumulus-doc.readthedocs.io/en/0.12.0/smart_seq_2.html and output as TPM using RSEM v1.3.2. Populations were transformed to transcripts per 10K reads and log2(1+TP10K) transformed. ACE2 expression by stimulation condition and dose were assessed using one-way ANOVA with post hoc testing using a Bonferroni correction. Plots were generated using ggplot2, and transcriptome-wide differential expression was calculated using the Seurat R package v3.1.0 (https://satijalab.org/seurat), function FindMarkers with test.use = ”bimod.” Expression data can be visualized and downloaded here:\nhttps://singlecell.broadinstitute.org/single_cell/study/SCP822?scpbr=the-alexandria-project.\n\nInterferon Treatment of Mouse Nasal Mucosa\nLibraries corresponding to 4 mice, with 2 Seq-Well arrays per mouse were sequenced using Illumina NextSeq as described (Gierahn et al., 2017, Hughes et al., 2019). Reads were aligned to the mm10 genome and processed according to the Drop-Seq Computational Protocol v2.0 (https://github.com/broadinstitute/Drop-seq). Data was normalized and scaled using the Seurat R package v2.3.4 (https://satijalab.org/seurat/): transforming the data to loge(UMI+1) and applying a scale factor of 10,000. Cells with fewer than 1000 UMIs and 500 unique genes were removed. To identify major axes of variation within our data, we first examined only highly variable genes across all cells, yielding approximately 5,000 variable genes. An approximate principal component analysis was applied to the cells to generate 200 principal components (PCs). Using a combination of the Jackstraw function in Seurat and observing the “elbow” of the standard deviations of PCs, we chose the top 70 PCs for subsequent clustering and visualization. For 2D visualization, we used a Uniform Manifold Approximation and Projection (UMAP) dimensionality reduction technique (https://github.com/lmcinnes/umap) with “min_dist” set to 0.3 and “n_neighbors” set to 50. To identify clusters of transcriptionally similar cells, we employed unsupervised clustering as described above using the FindClusters tool within the Seurat R package with default parameters and k.param set to 10. Resolution was chosen based on maximization of the average silhouette width across all cells. Clusters were merged if a cell type expressed fewer than 25 significantly upregulated genes by differential expression test (FindAllMarkers implemented in Seurat, setting “test.use” to “bimod,” Bonferroni-adjusted p value cutoff \u003c 0.001). Differential expression tests between cells from saline-treated or IFNa-treated mice were assessed using the FindMarkers function with “test.use” set to “bimod. This dataset can be visualized and downloaded here:\nhttps://singlecell.broadinstitute.org/single_cell/study/SCP832?scpbr=the-alexandria-project#study-summary.\n\nLung from MHV68-Infected WT and IFNγR KO Mice\nLibraries corresponding to 14 mice were aligned to a custom reference genome encompassing both murine (mm10) and herpes virus genes: 84 known genes from MHV68 were retrieved from NCBI (NCBI: txid33708) and added to the mm10 mouse genome. Reads were aligned to the custom joint genome and processed according to the Drop-Seq Computational Protocol v2.0 (https://github.com/broadinstitute/Drop-seq). Barcodes with \u003c 200 unique genes, \u003e 20,000 UMI counts, and \u003e 30% of transcript counts derived from mitochondrially encoded genes were discarded. Data analysis was performed using the Scanpy Package following the common procedure, the expression matrices were normalized using scran’s size factor based approach and log transformed via scanpy’s pp.log1p() function (Lun et al., 2016, Wolf et al., 2018). SoupX was utilized to reduce ambient RNA bias, using default parameters with pCut set to 0.3, and was applied to each sample before merging the count matrices (Young and Behjati, 2020). UMI per cell and cell cycle were regressed out. Highly variable genes were selected by running pp.highly_variable_genes() for each sample separately, returning the top 4,000 variable genes per sample, and genes identified in variable in \u003e 5 samples were retained, yielding 14,305 genes. Next, only Epcam+ cells were considered, principal components (PCs) were calculated using only the selected variable genes, and 6 PCs were used to perform unsupervised Louvain clustering. Type I Pneumocytes were excluded from this analysis based on uniformly negative expression of Ace2, resulting in a final dataset subset of 5,558 cells. Cells were identified as infected if at least one viral read was detected.\n\nNasal Washes during Influenza Infection\nSample processing, sequencing, and analysis was performed as in (Cao et al., 2020). Reads were aligned to the GRCh37 reference genome combined with influenza genomes. Mapped reads from each sample were then corrected for Drop-seq barcode synthesis error using the Drop-seq core computational tools developed by the McCarroll Lab (Macosko et al., 2015). Genes were quantified using End Sequence Analysis Toolkit (ESAT, github/garber-lab/ESAT) with parameters -wlen 100 -wOlap 50 -wExt 0 -scPrep (Derr et al., 2016). Finally, UMIs that likely result from sequencing errors were corrected by merging any UMIs that were observed only once and have 1 hamming distance from a UMI detected by two or more aligned reads. Only cell barcodes with more than 1,000 UMIs were analyzed. Cell barcodes with mostly erythrocyte genes (HBA, HBB) were removed. From here on, the remaining cell barcodes in the matrix would be referred to as cells. The final gene by cell matrix was normalized using the scran package v3.10 (Lun et al., 2016). The normalized matrix was used for dimensionality reduction by first selecting variable genes that had a high coefficient of variance (CV) and were expressed (\u003e = 1 UMI) by more than three cells. Influenza viral genes, interferon stimulated genes, and cell cycle related genes were removed from the variable gene list in order to minimize the impact of viral responses and mitosis on clustering and cell type identification. This resulted in the selection of 2484 variable genes. t-distributed stochastic neighbor embedding (tSNE) was applied to the first ten principal components (PCs), which explained 95% of the total data variance. Density clustering (Rodriguez and Laio, 2014) was performed on the resulting tSNE coordinates and identified four major clusters: epithelial cells, neutrophils, macrophages and leukocytes. The epithelial cell cluster and the leukocyte cluster were then re-clustered independently, as described above, to identify populations within each metacluster. Specifically, the epithelial cell cluster was re-embedded using 2629 variable genes selected by the same criteria mentioned in the previous section and 13 PCs that explained 95% of the variance. Density clustering over the epithelial cell subset revealed ten clusters. Differential gene expression analysis using edgeR (Robinson et al., 2010) was performed to identify marker genes for each cluster. Influenza-infected and bystander cells were identified after correcting for sample-specific distribution of ambient influenza mRNA contamination and predicted cells most likely to be infected identified using a hurdle zero inflated negative binomial (ZINB) model and a support vector machine (SVM) classifier.\n\nPower Calculations for Detection of Rare Transcripts\nWe conducted the following statistical analysis to estimate the effects of various factors on our ability to make confident claims regarding the presence/absence of transcripts of interest (e.g., ACE2), both within individual cells and clusters (Figure S6). Specifically, we investigated the roles of capture/reverse transcription efficiency, ACE2 expression level, sequencing depth, and cell numbers. Taken together, the results of this power analysis are in agreement with other efforts to model biological and technical sources of zero-inflation within scRNA-seq data (e.g., https://satijalab.org/howmanycells and Kharchenko et al., 2014, Svensson, 2020).\nWe began by quantifying how likely we are to capture and transcribe at least one ACE2 mRNA molecule, as a function of the number ACE2 mRNA molecules per cell and a protocol’s efficiency (Figure S6A). Drop-Seq has a capture/transcription efficiency of ∼10% (as estimated using ERCC spike ins; see (Macosko et al., 2015), and the experimental platforms used in this study are either equivalent (e.g., Seq-Well v1, (Gierahn et al., 2017) or superior (e.g., 10-fold better unique molecule detection, 5-fold better gene detection using Seq-Well S3;(Hughes et al., 2019)). Most relevant to this context, inferior turbinate scrapings were processed using both Seq-Well v1 and Seq-Well S3 (Figure S3B). Importantly, Seq-Well S3 provided \u003e two-fold increase in the detection frequency of rare ACE2 transcripts (i.e., ACE2+: 4.7% for v1 versus 9.8% for S3), making it reasonable to expect that such improvements in single-cell experimental technologies have yielded corresponding improvements in capture and transcription efficiency. Based on Drop-Seq’s 10% efficiency, even if ACE2 is expressed at the low level of 5 mRNA molecules per cell (a reasonable order-of-magnitude estimate, given that non-human primate ileum cells had a maximum of 10 ACE2 unique molecules per cell observed via sequencing and an average of 1.93 molecules per cell in expressing cells, see Figures 3B and 3C), our experimental platforms have a minimum likelihood of 41% to capture and reverse transcribe at least one ACE2 mRNA molecule in any given individual cell. This likelihood rapidly increases if we estimate higher efficiencies for improved scRNA-seq technologies (e.g., 67% likelihood within any individual cell at 20% capture/transcription efficiency, 76% likelihood at 25% efficiency, Figure S6A). Thus, while transcript drop-out may reduce the fraction of positive cells, with the capture and transcription efficiencies of improved single-cell technologies, the impact is likely to be minor (reads are likely underestimated by up to a factor of ∼2.5x), given a sufficient depth of sequencing (see below). We note that this impacts both clusters deemed to contain and not contain ACE2+ cells, and suggests our percentages are likely lower bounds for true expression (within a factor of ∼2.5x).\nNext, we examined the probability of sequencing an ACE2 transcript as a function of read depth and ACE2’s fractional abundance in each single cell within our sequencing libraries. First, across two different tissues (non-human primate ileum and lung, representing a high expresser of ACE2 and low expresser, respectively), we calculated the proportion of unique ACE2 molecules in our ACE2+ cells (defined as any cell with at least 1 UMI aligning to ACE2) as a fraction of total reads within individual cells to provide an order-of-magnitude estimate for average ACE2 abundance in our single-cell sequencing libraries (i.e., the probability that a read within a cell corresponds to a unique molecule of ACE2, Figure S6B). We highlight that by calculating probabilities based on ACE2 unique molecules divided by an individual cell’s total reads, we are providing a conservative estimate for the probability of observing ACE2 as a function of sequencing depth (e.g., as compared to basing these probabilities on ACE2 non-UMI-collapsed reads divided by total reads). Next, we obtained information on the number of reads in these cell populations to provide estimates of average sequencing depths (Figure S6C). Using the mean fractional abundances of ACE2 from each tissue (Figure S6B) and the mean read depths for all genes (Figure S6C), we calculated the probability of detecting at least 1 ACE2 molecule (i.e., P(detecting \u003e 0 ACE2 molecules) = 1 - (1 - ACE2 fractional abundance)Read depth). This results in a 93.7% probability in ileum-derived cell libraries that contain ACE2, and a 76.0% probability for lung-derived cell libraries, indicating that our sequencing depths are sufficient to detect ACE2+ cells (Figure S6D).\nTo further evaluate whether our ability to detect ACE2+ cells was an artifact of sequencing depth, we compared the number of ACE2+ cells in a cluster to the mean number of reads across all cells in that same cluster (Figure S6E). We did not observe any significant correlation: the ileum cell cluster with the highest number of ACE2+ cells had the lowest sequencing depth of all ileum clusters, and the lung cell cluster with the highest number of ACE2+ cells was approximately average in its read depth (on a log-log scale, Pearson’s r = −0.31, non-significant). Further, when comparing ACE2+ cells to ACE2- cells within a given tissue, we did not observe a positive correlation between read depth and ACE2 status (i.e., mean ± standard error of the mean, SEM, reads among all lung cells = 28,512 ± 344; mean ± SEM reads among ACE2+ lung cells = 28,553 ± 2,988; mean ± SEM reads among all ileum cells = 14,864 ± 288; mean ± SEM reads among ACE2+ ileum cells = 10,591 ± 441, full statistics on cell depth among ACE2+ cells compared to ACE2- cells of the same cell type can be found in Table S9). Thus, we can be confident that the observed differences in ACE2+ proportions across clusters are not driven by differences in sequencing depth.\nFinally, we investigated how observed differences in ACE2+ proportions across clusters might be affected by cell sampling. Using the proportion of ACE2+ cells in a “typical” cluster annotated as being ACE2 positive (i.e., 6.8% in non-human primate type II pneumocytes, Figure 1), we calculated the cluster sizes needed to be confident that the probability of observing zero to a few positive cells is unlikely to have arisen by random chance (probabilities calculated under a negative binomial distribution with parameter p = 0.068, Figure S6E). We found that as cluster sizes approach and exceed 100 cells, the probability of observing zero to a few positive cells rapidly approaches zero, if we assume 6.8% of cells are positive. Further, to examine our confidence in estimating an approximate upper bound (ignoring the impact of protocol inefficiencies discussed above) for the fraction of cells positive in a cluster as a function of the number of cells in that cluster, we also calculated the probability of observing zero (and its complement, probability of observing at least 1) ACE2+ cells as a function of cluster size across true positive proportions ranging from 0.1% to 10% (probabilities calculated under a negative binomial distribution with parameter p = 0.001 to 0.1, representing hypothetical proportions of ACE2+ cells Figure S6F). Given our typical cluster sizes (on the order of hundreds of cells, exact values provided in Table S9), we find that for us to observe 0 ACE2+ cells in a cluster due to sampling artifacts, the fraction of true positives must be ∼1% or less. Thus, these complementary approaches demonstrate that our observed variations in ACE2+ cell proportions across clusters likely reflect underlying biological differences, rather than random chance.\n\nStatistical Testing\nParameters such as sample size, number of replicates, number of independent experiments, measures of center, dispersion, and precision (mean ± SEM) and statistical significances are reported in Figures and Figure Legends. A p value less than 0.05 was considered significant. Where appropriate, a Bonferroni or FDR correction was used to account for multiple tests, alternative correction methods are noted in the figure legends or Methods. All statistical tests corresponding to differential gene expression are described above and completed using R language for Statistical Computing."}
2_test
{"project":"2_test","denotations":[{"id":"32413319-24836921-20790576","span":{"begin":1992,"end":1996},"obj":"24836921"},{"id":"32413319-30135581-20790577","span":{"begin":6042,"end":6046},"obj":"30135581"},{"id":"32413319-29608179-20790578","span":{"begin":6215,"end":6219},"obj":"29608179"},{"id":"32413319-25867923-20790579","span":{"begin":6236,"end":6240},"obj":"25867923"},{"id":"32413319-30135581-20790580","span":{"begin":6769,"end":6773},"obj":"30135581"},{"id":"32413319-29409532-20790581","span":{"begin":7330,"end":7334},"obj":"29409532"},{"id":"32413319-28192419-20790582","span":{"begin":9073,"end":9077},"obj":"28192419"},{"id":"32413319-27909575-20790583","span":{"begin":11855,"end":11859},"obj":"27909575"},{"id":"32413319-29409532-20790584","span":{"begin":11874,"end":11878},"obj":"29409532"},{"id":"32413319-26000488-20790585","span":{"begin":13156,"end":13160},"obj":"26000488"},{"id":"32413319-27470110-20790586","span":{"begin":13318,"end":13322},"obj":"27470110"},{"id":"32413319-27909575-20790587","span":{"begin":13827,"end":13831},"obj":"27909575"},{"id":"32413319-24970081-20790588","span":{"begin":14510,"end":14514},"obj":"24970081"},{"id":"32413319-19910308-20790589","span":{"begin":15157,"end":15161},"obj":"19910308"},{"id":"32413319-24836921-20790590","span":{"begin":16220,"end":16224},"obj":"24836921"},{"id":"32413319-31937974-20790591","span":{"begin":16236,"end":16240},"obj":"31937974"},{"id":"32413319-26000488-20790592","span":{"begin":16556,"end":16560},"obj":"26000488"},{"id":"32413319-28192419-20790593","span":{"begin":16672,"end":16676},"obj":"28192419"}],"text":"Quantification and Statistical Analysis\n\nNon-Human Primate Lung and Ileum\nLibraries corresponding to 7 animals (variable number of tissues per animal) were sequenced using Illumina NextSeq. Reads were aligned to the M. mulatta genome assembly 8.0.1 annotation version 102 and processed according to the Drop-Seq Computational Protocol v2.0 (https://github.com/broadinstitute/Drop-seq). Data was normalized and scaled using the Seurat R package v2.3.4 (https://satijalab.org/seurat/): transforming the data to loge(UMI+1) and applying a scale factor of 10,000. To identify major axes of variation within our data, we first examined only highly variable genes across all cells, yielding approximately 1,000-3,000 variable genes with average expression \u003e 0.1 log-normalized UMI across all cells. An approximate principal component analysis was applied to the cells to generate 100 principal components (PCs). Using the JackStraw function within Seurat, we identified significant PCs to be used for subsequent clustering and further dimensionality reduction. For 2D visualization and cell type clustering, we used a Uniform Manifold Approximation and Projection (UMAP) dimensionality reduction technique (https://github.com/lmcinnes/umap) with “min_dist” set to 0.5 and “n_neighbors” set to 30. To identify clusters of transcriptionally similar cells, we employed unsupervised clustering as described above using the FindClusters tool within the Seurat R package with default parameters and k.param set to 10 and resolution set to 0.5. Each cluster was sub-clustered to identify more granular cell types, requiring each cell type to express \u003e 25 significantly upregulated genes by differential expression test (FindMarkers implemented in Seurat, setting “test.use” to “bimod,” Bonferroni-adjusted p value cutoff \u003c 0.001). Differential expression tests between cells from ACE2 + versus ACE2 - Type II Pneumocytes were conducted using the SCDE R package with default parameters (Kharchenko et al., 2014). Expression data for epithelial cells and enterocytes included in this dataset can be visualized and downloaded here: https://singlecell.broadinstitute.org/single_cell/study/SCP807?scpbr=the-alexandria-project#study-summary.\n\nHuman Lung Tissue\nLibraries corresponding to 8 donors were sequenced using Illumina NextSeq. Reads were aligned to the hg19 genome assembly and processed according to the Drop-Seq Computational Protocol v2.0 (https://github.com/broadinstitute/Drop-seq). Data was normalized and scaled using the Seurat R package v3.1.0 (https://satijalab.org/seurat/), transforming the data to loge(UMI+1) and applying a scale factor of 10,000. For each array, we assessed the quality of constructed libraries by examining the distribution of reads, genes and transcripts per cell. Variable gene selection, principal components analysis, and selection of significant principal components was performed as above. We visualized our results in a two-dimensional space using UMAP (https://github.com/lmcinnes/umap), and annotated each cluster based on the identification of highly expressed genes. To further characterize substructure within cell types (for example, T cells), we performed dimensionality reduction (PCA) and clustering over those cells alone. Sub-clusters (i.e., clusters within broad cell type classifications) were annotated by cross-referencing cluster-defining genes with curated gene lists and online databases SaVanT (http://newpathways.mcdb.ucla.edu/savant-dev/) and GSEA/MsigDB (https://www.gsea-msigdb.org/gsea/msigdb/index.jsp). Proliferating cells from the human lung (Figure 2C) express high levels of mitotic markers, such as MKI67, and represent primarily T cells (CD3D, CD3E), B cells/antibody-secreting cells (IGJ, MZB1, IGHG1), and myeloid cells (CD14, APOE) and represent a composite cell cluster. Differential expression analysis between ACE2+ TMPRSS2+ and negative type II pneumocytes was performed in Seurat using a likelihood-ratio test (FindMarkers implemented in Seurat, setting “test.use” to bimod). Expression data for epithelial cells included in this dataset can be visualized and downloaded here: https://singlecell.broadinstitute.org/single_cell/study/SCP814?scpbr=the-alexandria-project#study-summary.\n\nHuman Ileum\nLibraries corresponding to 13 donors were sequenced using Illumina NovaSeq S2 with a Read 1 26bp, Read 2 91bp, Index 1 8bp configuration before reads were aligned to GRCh38. Each sample was filtered individually for low quality cells and genes by analyzing distributions of reads, transcripts, percent reads mapped to mitochondrial genes, and complexity per cell, then merged as an outer join to create a single dataset. Clustering and differential expression tests were processed using Seurat v3.1.0 (https://satijalab.org/seurat/). Normalization and variable gene selection was processed with SCTransform (https://github.com/ChristophH/sctransform). Clustering for major cell types was performed using Louvain clustering on dimensionally reduced PCA space with resolution set via grid search optimizing for maximum average silhouette score. Due to the scale of the dataset, a randomized subsampling from across the dataset was used to calculate the silhouette score. We annotated clusters based on highly expressed genes, then sub-clusters were characterized by performing PCA dimensionality reduction and clustering over those cells alone, and annotated based on highly expressed genes found via one-versus-rest differential expression test (Wilcoxon) within the major cell type. Differential expression analysis between ACE2 + TMPRSS2 + and negative epithelial cells was performed in Seurat using a Wilcoxon test and Bonferroni p value correction. Expression data for epithelial cells included in this dataset can be visualized and downloaded here: https://singlecell.broadinstitute.org/single_cell/study/SCP812?scpbr=the-alexandria-project#study-summary.\n\nHuman Adult Nasal Mucosa\nSample processing, sequencing, and analysis was performed as in (Ordovas-Montanes et al., 2018). Briefly, scRNA-seq cell suspensions were freshly processed using Seq-Well v1 and Seurat v2.3.4 was utilized for computational analyses presented here (Butler et al., 2018, Satija et al., 2015). Cell by gene matrix and R code for initialization of object available to download as Supplemental Data and Supplementary Tables here https://www.nature.com/articles/s41586-018-0449-8 and here:\nhttp://shaleklab.com/resource/mapping-allergic-inflammation/? and visualized here: https://singlecell.broadinstitute.org/single_cell/study/SCP253?scpbr=the-alexandria-project#study-summary. Scores for various cytokines acting on human airway epithelial cells were calculated based on gene lists derived for (Ordovas-Montanes et al., 2018), calculated using AddModuleScore function Seurat, and effect size calculated by Cohen’s d, as previously reported.\n\nGranulomatous Tissue from Mycobacterium Tuberculosis Infected NHPs\nLibraries corresponding to 10 animals (variable number of tissues/animal) were sequenced using Illumina NovaSeq S2. Data was aligned using the Dropseq-tools pipeline on Terra (app.terra.bio) to M. fascicularis reference genome assembly 5, annotation version 101. Clustering was performed using Leiden clustering in the Scanpy (scanpy.readthedocs.io) package (Wolf et al., 2018). Cell type labels were assigned using known marker genes. In this analysis, we include all epithelial cell subsets (secretory, multiciliated, type II pneumocytes, and type I pneumocytes) from all samples. Differential expression between ACE2 + TMPRSS2 + cells and other cells of the matched cell subtype (e.g., Secretory Cells) were performed using the “bimod” likelihood-ratio test within each cell subtype and filtered on Benjamini-Hochberg-corrected p value \u003c 0.05. Expression data for epithelial cells included in this dataset can be visualized and downloaded here:\nhttps://singlecell.broadinstitute.org/single_cell/study/SCP806?scpbr=the-alexandria-project#study-summary.\n\nBasal Cell Cytokine Stimulation\nLibraries corresponding to 279 populations were sequenced using Illumina NextSeq. Reads were aligned to the hg19 or mm10 genome assembly using the cumulus platform https://cumulus-doc.readthedocs.io/en/0.12.0/smart_seq_2.html and output as TPM using RSEM v1.3.2. Populations were transformed to transcripts per 10K reads and log2(1+TP10K) transformed. ACE2 expression by stimulation condition and dose were assessed using one-way ANOVA with post hoc testing using a Bonferroni correction. Plots were generated using ggplot2, and transcriptome-wide differential expression was calculated using the Seurat R package v3.1.0 (https://satijalab.org/seurat), function FindMarkers with test.use = ”bimod.” Expression data can be visualized and downloaded here:\nhttps://singlecell.broadinstitute.org/single_cell/study/SCP822?scpbr=the-alexandria-project.\n\nInterferon Treatment of Mouse Nasal Mucosa\nLibraries corresponding to 4 mice, with 2 Seq-Well arrays per mouse were sequenced using Illumina NextSeq as described (Gierahn et al., 2017, Hughes et al., 2019). Reads were aligned to the mm10 genome and processed according to the Drop-Seq Computational Protocol v2.0 (https://github.com/broadinstitute/Drop-seq). Data was normalized and scaled using the Seurat R package v2.3.4 (https://satijalab.org/seurat/): transforming the data to loge(UMI+1) and applying a scale factor of 10,000. Cells with fewer than 1000 UMIs and 500 unique genes were removed. To identify major axes of variation within our data, we first examined only highly variable genes across all cells, yielding approximately 5,000 variable genes. An approximate principal component analysis was applied to the cells to generate 200 principal components (PCs). Using a combination of the Jackstraw function in Seurat and observing the “elbow” of the standard deviations of PCs, we chose the top 70 PCs for subsequent clustering and visualization. For 2D visualization, we used a Uniform Manifold Approximation and Projection (UMAP) dimensionality reduction technique (https://github.com/lmcinnes/umap) with “min_dist” set to 0.3 and “n_neighbors” set to 50. To identify clusters of transcriptionally similar cells, we employed unsupervised clustering as described above using the FindClusters tool within the Seurat R package with default parameters and k.param set to 10. Resolution was chosen based on maximization of the average silhouette width across all cells. Clusters were merged if a cell type expressed fewer than 25 significantly upregulated genes by differential expression test (FindAllMarkers implemented in Seurat, setting “test.use” to “bimod,” Bonferroni-adjusted p value cutoff \u003c 0.001). Differential expression tests between cells from saline-treated or IFNa-treated mice were assessed using the FindMarkers function with “test.use” set to “bimod. This dataset can be visualized and downloaded here:\nhttps://singlecell.broadinstitute.org/single_cell/study/SCP832?scpbr=the-alexandria-project#study-summary.\n\nLung from MHV68-Infected WT and IFNγR KO Mice\nLibraries corresponding to 14 mice were aligned to a custom reference genome encompassing both murine (mm10) and herpes virus genes: 84 known genes from MHV68 were retrieved from NCBI (NCBI: txid33708) and added to the mm10 mouse genome. Reads were aligned to the custom joint genome and processed according to the Drop-Seq Computational Protocol v2.0 (https://github.com/broadinstitute/Drop-seq). Barcodes with \u003c 200 unique genes, \u003e 20,000 UMI counts, and \u003e 30% of transcript counts derived from mitochondrially encoded genes were discarded. Data analysis was performed using the Scanpy Package following the common procedure, the expression matrices were normalized using scran’s size factor based approach and log transformed via scanpy’s pp.log1p() function (Lun et al., 2016, Wolf et al., 2018). SoupX was utilized to reduce ambient RNA bias, using default parameters with pCut set to 0.3, and was applied to each sample before merging the count matrices (Young and Behjati, 2020). UMI per cell and cell cycle were regressed out. Highly variable genes were selected by running pp.highly_variable_genes() for each sample separately, returning the top 4,000 variable genes per sample, and genes identified in variable in \u003e 5 samples were retained, yielding 14,305 genes. Next, only Epcam+ cells were considered, principal components (PCs) were calculated using only the selected variable genes, and 6 PCs were used to perform unsupervised Louvain clustering. Type I Pneumocytes were excluded from this analysis based on uniformly negative expression of Ace2, resulting in a final dataset subset of 5,558 cells. Cells were identified as infected if at least one viral read was detected.\n\nNasal Washes during Influenza Infection\nSample processing, sequencing, and analysis was performed as in (Cao et al., 2020). Reads were aligned to the GRCh37 reference genome combined with influenza genomes. Mapped reads from each sample were then corrected for Drop-seq barcode synthesis error using the Drop-seq core computational tools developed by the McCarroll Lab (Macosko et al., 2015). Genes were quantified using End Sequence Analysis Toolkit (ESAT, github/garber-lab/ESAT) with parameters -wlen 100 -wOlap 50 -wExt 0 -scPrep (Derr et al., 2016). Finally, UMIs that likely result from sequencing errors were corrected by merging any UMIs that were observed only once and have 1 hamming distance from a UMI detected by two or more aligned reads. Only cell barcodes with more than 1,000 UMIs were analyzed. Cell barcodes with mostly erythrocyte genes (HBA, HBB) were removed. From here on, the remaining cell barcodes in the matrix would be referred to as cells. The final gene by cell matrix was normalized using the scran package v3.10 (Lun et al., 2016). The normalized matrix was used for dimensionality reduction by first selecting variable genes that had a high coefficient of variance (CV) and were expressed (\u003e = 1 UMI) by more than three cells. Influenza viral genes, interferon stimulated genes, and cell cycle related genes were removed from the variable gene list in order to minimize the impact of viral responses and mitosis on clustering and cell type identification. This resulted in the selection of 2484 variable genes. t-distributed stochastic neighbor embedding (tSNE) was applied to the first ten principal components (PCs), which explained 95% of the total data variance. Density clustering (Rodriguez and Laio, 2014) was performed on the resulting tSNE coordinates and identified four major clusters: epithelial cells, neutrophils, macrophages and leukocytes. The epithelial cell cluster and the leukocyte cluster were then re-clustered independently, as described above, to identify populations within each metacluster. Specifically, the epithelial cell cluster was re-embedded using 2629 variable genes selected by the same criteria mentioned in the previous section and 13 PCs that explained 95% of the variance. Density clustering over the epithelial cell subset revealed ten clusters. Differential gene expression analysis using edgeR (Robinson et al., 2010) was performed to identify marker genes for each cluster. Influenza-infected and bystander cells were identified after correcting for sample-specific distribution of ambient influenza mRNA contamination and predicted cells most likely to be infected identified using a hurdle zero inflated negative binomial (ZINB) model and a support vector machine (SVM) classifier.\n\nPower Calculations for Detection of Rare Transcripts\nWe conducted the following statistical analysis to estimate the effects of various factors on our ability to make confident claims regarding the presence/absence of transcripts of interest (e.g., ACE2), both within individual cells and clusters (Figure S6). Specifically, we investigated the roles of capture/reverse transcription efficiency, ACE2 expression level, sequencing depth, and cell numbers. Taken together, the results of this power analysis are in agreement with other efforts to model biological and technical sources of zero-inflation within scRNA-seq data (e.g., https://satijalab.org/howmanycells and Kharchenko et al., 2014, Svensson, 2020).\nWe began by quantifying how likely we are to capture and transcribe at least one ACE2 mRNA molecule, as a function of the number ACE2 mRNA molecules per cell and a protocol’s efficiency (Figure S6A). Drop-Seq has a capture/transcription efficiency of ∼10% (as estimated using ERCC spike ins; see (Macosko et al., 2015), and the experimental platforms used in this study are either equivalent (e.g., Seq-Well v1, (Gierahn et al., 2017) or superior (e.g., 10-fold better unique molecule detection, 5-fold better gene detection using Seq-Well S3;(Hughes et al., 2019)). Most relevant to this context, inferior turbinate scrapings were processed using both Seq-Well v1 and Seq-Well S3 (Figure S3B). Importantly, Seq-Well S3 provided \u003e two-fold increase in the detection frequency of rare ACE2 transcripts (i.e., ACE2+: 4.7% for v1 versus 9.8% for S3), making it reasonable to expect that such improvements in single-cell experimental technologies have yielded corresponding improvements in capture and transcription efficiency. Based on Drop-Seq’s 10% efficiency, even if ACE2 is expressed at the low level of 5 mRNA molecules per cell (a reasonable order-of-magnitude estimate, given that non-human primate ileum cells had a maximum of 10 ACE2 unique molecules per cell observed via sequencing and an average of 1.93 molecules per cell in expressing cells, see Figures 3B and 3C), our experimental platforms have a minimum likelihood of 41% to capture and reverse transcribe at least one ACE2 mRNA molecule in any given individual cell. This likelihood rapidly increases if we estimate higher efficiencies for improved scRNA-seq technologies (e.g., 67% likelihood within any individual cell at 20% capture/transcription efficiency, 76% likelihood at 25% efficiency, Figure S6A). Thus, while transcript drop-out may reduce the fraction of positive cells, with the capture and transcription efficiencies of improved single-cell technologies, the impact is likely to be minor (reads are likely underestimated by up to a factor of ∼2.5x), given a sufficient depth of sequencing (see below). We note that this impacts both clusters deemed to contain and not contain ACE2+ cells, and suggests our percentages are likely lower bounds for true expression (within a factor of ∼2.5x).\nNext, we examined the probability of sequencing an ACE2 transcript as a function of read depth and ACE2’s fractional abundance in each single cell within our sequencing libraries. First, across two different tissues (non-human primate ileum and lung, representing a high expresser of ACE2 and low expresser, respectively), we calculated the proportion of unique ACE2 molecules in our ACE2+ cells (defined as any cell with at least 1 UMI aligning to ACE2) as a fraction of total reads within individual cells to provide an order-of-magnitude estimate for average ACE2 abundance in our single-cell sequencing libraries (i.e., the probability that a read within a cell corresponds to a unique molecule of ACE2, Figure S6B). We highlight that by calculating probabilities based on ACE2 unique molecules divided by an individual cell’s total reads, we are providing a conservative estimate for the probability of observing ACE2 as a function of sequencing depth (e.g., as compared to basing these probabilities on ACE2 non-UMI-collapsed reads divided by total reads). Next, we obtained information on the number of reads in these cell populations to provide estimates of average sequencing depths (Figure S6C). Using the mean fractional abundances of ACE2 from each tissue (Figure S6B) and the mean read depths for all genes (Figure S6C), we calculated the probability of detecting at least 1 ACE2 molecule (i.e., P(detecting \u003e 0 ACE2 molecules) = 1 - (1 - ACE2 fractional abundance)Read depth). This results in a 93.7% probability in ileum-derived cell libraries that contain ACE2, and a 76.0% probability for lung-derived cell libraries, indicating that our sequencing depths are sufficient to detect ACE2+ cells (Figure S6D).\nTo further evaluate whether our ability to detect ACE2+ cells was an artifact of sequencing depth, we compared the number of ACE2+ cells in a cluster to the mean number of reads across all cells in that same cluster (Figure S6E). We did not observe any significant correlation: the ileum cell cluster with the highest number of ACE2+ cells had the lowest sequencing depth of all ileum clusters, and the lung cell cluster with the highest number of ACE2+ cells was approximately average in its read depth (on a log-log scale, Pearson’s r = −0.31, non-significant). Further, when comparing ACE2+ cells to ACE2- cells within a given tissue, we did not observe a positive correlation between read depth and ACE2 status (i.e., mean ± standard error of the mean, SEM, reads among all lung cells = 28,512 ± 344; mean ± SEM reads among ACE2+ lung cells = 28,553 ± 2,988; mean ± SEM reads among all ileum cells = 14,864 ± 288; mean ± SEM reads among ACE2+ ileum cells = 10,591 ± 441, full statistics on cell depth among ACE2+ cells compared to ACE2- cells of the same cell type can be found in Table S9). Thus, we can be confident that the observed differences in ACE2+ proportions across clusters are not driven by differences in sequencing depth.\nFinally, we investigated how observed differences in ACE2+ proportions across clusters might be affected by cell sampling. Using the proportion of ACE2+ cells in a “typical” cluster annotated as being ACE2 positive (i.e., 6.8% in non-human primate type II pneumocytes, Figure 1), we calculated the cluster sizes needed to be confident that the probability of observing zero to a few positive cells is unlikely to have arisen by random chance (probabilities calculated under a negative binomial distribution with parameter p = 0.068, Figure S6E). We found that as cluster sizes approach and exceed 100 cells, the probability of observing zero to a few positive cells rapidly approaches zero, if we assume 6.8% of cells are positive. Further, to examine our confidence in estimating an approximate upper bound (ignoring the impact of protocol inefficiencies discussed above) for the fraction of cells positive in a cluster as a function of the number of cells in that cluster, we also calculated the probability of observing zero (and its complement, probability of observing at least 1) ACE2+ cells as a function of cluster size across true positive proportions ranging from 0.1% to 10% (probabilities calculated under a negative binomial distribution with parameter p = 0.001 to 0.1, representing hypothetical proportions of ACE2+ cells Figure S6F). Given our typical cluster sizes (on the order of hundreds of cells, exact values provided in Table S9), we find that for us to observe 0 ACE2+ cells in a cluster due to sampling artifacts, the fraction of true positives must be ∼1% or less. Thus, these complementary approaches demonstrate that our observed variations in ACE2+ cell proportions across clusters likely reflect underlying biological differences, rather than random chance.\n\nStatistical Testing\nParameters such as sample size, number of replicates, number of independent experiments, measures of center, dispersion, and precision (mean ± SEM) and statistical significances are reported in Figures and Figure Legends. A p value less than 0.05 was considered significant. Where appropriate, a Bonferroni or FDR correction was used to account for multiple tests, alternative correction methods are noted in the figure legends or Methods. All statistical tests corresponding to differential gene expression are described above and completed using R language for Statistical Computing."}