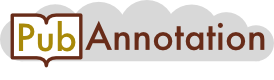
PMC:7210464 / 29770-33549
Annnotations
LitCovid-PD-MONDO
{"project":"LitCovid-PD-MONDO","denotations":[{"id":"T80","span":{"begin":257,"end":266},"obj":"Disease"},{"id":"T81","span":{"begin":903,"end":912},"obj":"Disease"},{"id":"T82","span":{"begin":1271,"end":1279},"obj":"Disease"}],"attributes":[{"id":"A80","pred":"mondo_id","subj":"T80","obj":"http://purl.obolibrary.org/obo/MONDO_0005550"},{"id":"A81","pred":"mondo_id","subj":"T81","obj":"http://purl.obolibrary.org/obo/MONDO_0005550"},{"id":"A82","pred":"mondo_id","subj":"T82","obj":"http://purl.obolibrary.org/obo/MONDO_0100096"}],"text":"Table 3 reports the estimation results of the OLS and IV regressions of Eq. 2, in which only within-city transmission is considered. After controlling for time-invariant city fixed effects and time effects that are common to all cities, on average, one new infection leads to 1.142 more cases in the next week, but 0.824 fewer cases 1 week later. The negative effect can be attributed to the fact that both local authorities and residents would have taken more protective measures in response to a higher perceived risk of contracting the virus given more time. Information disclosure on newly confirmed cases at the daily level by official media and information dissemination on social media throughout China may have promoted more timely actions by the public, resulting in slower virus transmissions. We then compare the transmission rates in different time windows. In the first sub-sample, one new infection leads to 2.135 more cases within a week, implying a fast growth in the number of cases. However, in the second sub-sample, the effect decreases to 1.077, suggesting that public health measures imposed in late January were effective in limiting a further spread of the virus. Similar patterns are also observed in model B.\nTable 3 Within-city transmission of COVID-19\nJan 19–Feb 29 Jan 19–Feb 1 Feb 2–Feb 29\n(1) (2) (3) (4) (5) (6)\nOLS IV OLS IV OLS IV\nAll cities excluding Wuhan\nModel A: lagged variables are averages over the preceding first and second week separately\nAverage # of new cases 0.873*** 1.142*** 1.692*** 2.135*** 0.768*** 1.077***\n1-week lag (0.00949) (0.0345) (0.0312) (0.0549) (0.0120) (0.0203)\nAverage # of new cases − 0.415*** − 0.824*** 0.860 − 6.050*** − 0.408*** − 0.796***\n2-week lag (0.00993) (0.0432) (2.131) (2.314) (0.00695) (0.0546)\nModel B: lagged variables are averages over the preceding 2 weeks\nAverage # of new case 0.474*** 0.720*** 3.310*** 3.860*** 0.494*** 1.284***\nPrevious 14 days (0.0327) (0.143) (0.223) (0.114) (0.00859) (0.107)\nObservations 12,768 12,768 4256 4256 8512 8512\nNumber of cities 304 304 304 304 304 304\nWeather controls Yes Yes Yes Yes Yes Yes\nCity FE Yes Yes Yes Yes Yes Yes\nDate FE Yes Yes Yes Yes Yes Yes\nAll cities excluding cities in Hubei Province\nModel A: lagged variables are averages over the preceding first and second week separately\nAverage # of new cases 0.725*** 1.113*** 1.050*** 1.483*** 0.620*** 0.903***\n1-week lag (0.141) (0.0802) (0.0828) (0.205) (0.166) (0.0349)\nAverage # of new cases − 0.394*** − 0.572*** 0.108 − 3.664 − 0.228*** − 0.341***\n2-week lag (0.0628) (0.107) (0.675) (2.481) (0.0456) (0.121)\nModel B: lagged variables are averages over the preceding 2 weeks\nAverage # of new cases 0.357*** 0.631*** 1.899*** 2.376*** 0.493*** 0.745***\nPrevious 14 days (0.0479) (0.208) (0.250) (0.346) (0.122) (0.147)\nObservations 12,096 12,096 4032 4032 8064 8064\nNumber of cities 288 288 288 288 288 288\nWeather controls Yes Yes Yes Yes Yes Yes\nCity FE Yes Yes Yes Yes Yes Yes\nDate FE Yes Yes Yes Yes Yes Yes\nThe dependent variable is the number of daily new cases. The endogenous explanatory variables include the average numbers of new confirmed cases in the own city in the preceding first and second weeks (model A) and the average number in the preceding 14 days (model B). Weekly averages of daily maximum temperature, precipitation, wind speed, the interaction between precipitation and wind speed, and the inverse log distance weighted sum of each of these variables in other cities, during the preceding third and fourth weeks, are used as instrumental variables in the IV regressions. Weather controls include contemporaneous weather variables in the preceding first and second weeks. Standard errors in parentheses are clustered by provinces. *** p \u003c 0.01, ** p \u003c 0.05, * p \u003c 0.1"}
LitCovid-PD-CLO
{"project":"LitCovid-PD-CLO","denotations":[{"id":"T142","span":{"begin":496,"end":497},"obj":"http://purl.obolibrary.org/obo/CLO_0001020"},{"id":"T143","span":{"begin":539,"end":544},"obj":"http://purl.obolibrary.org/obo/NCBITaxon_10239"},{"id":"T144","span":{"begin":783,"end":788},"obj":"http://purl.obolibrary.org/obo/NCBITaxon_10239"},{"id":"T145","span":{"begin":946,"end":947},"obj":"http://purl.obolibrary.org/obo/CLO_0001020"},{"id":"T146","span":{"begin":963,"end":964},"obj":"http://purl.obolibrary.org/obo/CLO_0001020"},{"id":"T147","span":{"begin":1157,"end":1158},"obj":"http://purl.obolibrary.org/obo/CLO_0001020"},{"id":"T148","span":{"begin":1181,"end":1186},"obj":"http://purl.obolibrary.org/obo/NCBITaxon_10239"},{"id":"T149","span":{"begin":1232,"end":1233},"obj":"http://purl.obolibrary.org/obo/CLO_0001021"},{"id":"T150","span":{"begin":1329,"end":1334},"obj":"http://purl.obolibrary.org/obo/CLO_0001302"},{"id":"T151","span":{"begin":1398,"end":1399},"obj":"http://purl.obolibrary.org/obo/CLO_0001020"},{"id":"T152","span":{"begin":1567,"end":1570},"obj":"http://purl.obolibrary.org/obo/CLO_0050236"},{"id":"T153","span":{"begin":1717,"end":1720},"obj":"http://purl.obolibrary.org/obo/CLO_0050236"},{"id":"T154","span":{"begin":1781,"end":1782},"obj":"http://purl.obolibrary.org/obo/CLO_0001021"},{"id":"T155","span":{"begin":2230,"end":2231},"obj":"http://purl.obolibrary.org/obo/CLO_0001020"},{"id":"T156","span":{"begin":2399,"end":2402},"obj":"http://purl.obolibrary.org/obo/CLO_0050236"},{"id":"T157","span":{"begin":2542,"end":2545},"obj":"http://purl.obolibrary.org/obo/CLO_0050236"},{"id":"T158","span":{"begin":2602,"end":2603},"obj":"http://purl.obolibrary.org/obo/CLO_0001021"},{"id":"T159","span":{"begin":3206,"end":3207},"obj":"http://purl.obolibrary.org/obo/CLO_0001020"},{"id":"T160","span":{"begin":3264,"end":3265},"obj":"http://purl.obolibrary.org/obo/CLO_0001021"},{"id":"T161","span":{"begin":3538,"end":3550},"obj":"http://purl.obolibrary.org/obo/OBI_0000968"}],"text":"Table 3 reports the estimation results of the OLS and IV regressions of Eq. 2, in which only within-city transmission is considered. After controlling for time-invariant city fixed effects and time effects that are common to all cities, on average, one new infection leads to 1.142 more cases in the next week, but 0.824 fewer cases 1 week later. The negative effect can be attributed to the fact that both local authorities and residents would have taken more protective measures in response to a higher perceived risk of contracting the virus given more time. Information disclosure on newly confirmed cases at the daily level by official media and information dissemination on social media throughout China may have promoted more timely actions by the public, resulting in slower virus transmissions. We then compare the transmission rates in different time windows. In the first sub-sample, one new infection leads to 2.135 more cases within a week, implying a fast growth in the number of cases. However, in the second sub-sample, the effect decreases to 1.077, suggesting that public health measures imposed in late January were effective in limiting a further spread of the virus. Similar patterns are also observed in model B.\nTable 3 Within-city transmission of COVID-19\nJan 19–Feb 29 Jan 19–Feb 1 Feb 2–Feb 29\n(1) (2) (3) (4) (5) (6)\nOLS IV OLS IV OLS IV\nAll cities excluding Wuhan\nModel A: lagged variables are averages over the preceding first and second week separately\nAverage # of new cases 0.873*** 1.142*** 1.692*** 2.135*** 0.768*** 1.077***\n1-week lag (0.00949) (0.0345) (0.0312) (0.0549) (0.0120) (0.0203)\nAverage # of new cases − 0.415*** − 0.824*** 0.860 − 6.050*** − 0.408*** − 0.796***\n2-week lag (0.00993) (0.0432) (2.131) (2.314) (0.00695) (0.0546)\nModel B: lagged variables are averages over the preceding 2 weeks\nAverage # of new case 0.474*** 0.720*** 3.310*** 3.860*** 0.494*** 1.284***\nPrevious 14 days (0.0327) (0.143) (0.223) (0.114) (0.00859) (0.107)\nObservations 12,768 12,768 4256 4256 8512 8512\nNumber of cities 304 304 304 304 304 304\nWeather controls Yes Yes Yes Yes Yes Yes\nCity FE Yes Yes Yes Yes Yes Yes\nDate FE Yes Yes Yes Yes Yes Yes\nAll cities excluding cities in Hubei Province\nModel A: lagged variables are averages over the preceding first and second week separately\nAverage # of new cases 0.725*** 1.113*** 1.050*** 1.483*** 0.620*** 0.903***\n1-week lag (0.141) (0.0802) (0.0828) (0.205) (0.166) (0.0349)\nAverage # of new cases − 0.394*** − 0.572*** 0.108 − 3.664 − 0.228*** − 0.341***\n2-week lag (0.0628) (0.107) (0.675) (2.481) (0.0456) (0.121)\nModel B: lagged variables are averages over the preceding 2 weeks\nAverage # of new cases 0.357*** 0.631*** 1.899*** 2.376*** 0.493*** 0.745***\nPrevious 14 days (0.0479) (0.208) (0.250) (0.346) (0.122) (0.147)\nObservations 12,096 12,096 4032 4032 8064 8064\nNumber of cities 288 288 288 288 288 288\nWeather controls Yes Yes Yes Yes Yes Yes\nCity FE Yes Yes Yes Yes Yes Yes\nDate FE Yes Yes Yes Yes Yes Yes\nThe dependent variable is the number of daily new cases. The endogenous explanatory variables include the average numbers of new confirmed cases in the own city in the preceding first and second weeks (model A) and the average number in the preceding 14 days (model B). Weekly averages of daily maximum temperature, precipitation, wind speed, the interaction between precipitation and wind speed, and the inverse log distance weighted sum of each of these variables in other cities, during the preceding third and fourth weeks, are used as instrumental variables in the IV regressions. Weather controls include contemporaneous weather variables in the preceding first and second weeks. Standard errors in parentheses are clustered by provinces. *** p \u003c 0.01, ** p \u003c 0.05, * p \u003c 0.1"}
LitCovid-PD-GO-BP
{"project":"LitCovid-PD-GO-BP","denotations":[{"id":"T4","span":{"begin":970,"end":976},"obj":"http://purl.obolibrary.org/obo/GO_0040007"}],"text":"Table 3 reports the estimation results of the OLS and IV regressions of Eq. 2, in which only within-city transmission is considered. After controlling for time-invariant city fixed effects and time effects that are common to all cities, on average, one new infection leads to 1.142 more cases in the next week, but 0.824 fewer cases 1 week later. The negative effect can be attributed to the fact that both local authorities and residents would have taken more protective measures in response to a higher perceived risk of contracting the virus given more time. Information disclosure on newly confirmed cases at the daily level by official media and information dissemination on social media throughout China may have promoted more timely actions by the public, resulting in slower virus transmissions. We then compare the transmission rates in different time windows. In the first sub-sample, one new infection leads to 2.135 more cases within a week, implying a fast growth in the number of cases. However, in the second sub-sample, the effect decreases to 1.077, suggesting that public health measures imposed in late January were effective in limiting a further spread of the virus. Similar patterns are also observed in model B.\nTable 3 Within-city transmission of COVID-19\nJan 19–Feb 29 Jan 19–Feb 1 Feb 2–Feb 29\n(1) (2) (3) (4) (5) (6)\nOLS IV OLS IV OLS IV\nAll cities excluding Wuhan\nModel A: lagged variables are averages over the preceding first and second week separately\nAverage # of new cases 0.873*** 1.142*** 1.692*** 2.135*** 0.768*** 1.077***\n1-week lag (0.00949) (0.0345) (0.0312) (0.0549) (0.0120) (0.0203)\nAverage # of new cases − 0.415*** − 0.824*** 0.860 − 6.050*** − 0.408*** − 0.796***\n2-week lag (0.00993) (0.0432) (2.131) (2.314) (0.00695) (0.0546)\nModel B: lagged variables are averages over the preceding 2 weeks\nAverage # of new case 0.474*** 0.720*** 3.310*** 3.860*** 0.494*** 1.284***\nPrevious 14 days (0.0327) (0.143) (0.223) (0.114) (0.00859) (0.107)\nObservations 12,768 12,768 4256 4256 8512 8512\nNumber of cities 304 304 304 304 304 304\nWeather controls Yes Yes Yes Yes Yes Yes\nCity FE Yes Yes Yes Yes Yes Yes\nDate FE Yes Yes Yes Yes Yes Yes\nAll cities excluding cities in Hubei Province\nModel A: lagged variables are averages over the preceding first and second week separately\nAverage # of new cases 0.725*** 1.113*** 1.050*** 1.483*** 0.620*** 0.903***\n1-week lag (0.141) (0.0802) (0.0828) (0.205) (0.166) (0.0349)\nAverage # of new cases − 0.394*** − 0.572*** 0.108 − 3.664 − 0.228*** − 0.341***\n2-week lag (0.0628) (0.107) (0.675) (2.481) (0.0456) (0.121)\nModel B: lagged variables are averages over the preceding 2 weeks\nAverage # of new cases 0.357*** 0.631*** 1.899*** 2.376*** 0.493*** 0.745***\nPrevious 14 days (0.0479) (0.208) (0.250) (0.346) (0.122) (0.147)\nObservations 12,096 12,096 4032 4032 8064 8064\nNumber of cities 288 288 288 288 288 288\nWeather controls Yes Yes Yes Yes Yes Yes\nCity FE Yes Yes Yes Yes Yes Yes\nDate FE Yes Yes Yes Yes Yes Yes\nThe dependent variable is the number of daily new cases. The endogenous explanatory variables include the average numbers of new confirmed cases in the own city in the preceding first and second weeks (model A) and the average number in the preceding 14 days (model B). Weekly averages of daily maximum temperature, precipitation, wind speed, the interaction between precipitation and wind speed, and the inverse log distance weighted sum of each of these variables in other cities, during the preceding third and fourth weeks, are used as instrumental variables in the IV regressions. Weather controls include contemporaneous weather variables in the preceding first and second weeks. Standard errors in parentheses are clustered by provinces. *** p \u003c 0.01, ** p \u003c 0.05, * p \u003c 0.1"}
LitCovid-sentences
{"project":"LitCovid-sentences","denotations":[{"id":"T234","span":{"begin":0,"end":132},"obj":"Sentence"},{"id":"T235","span":{"begin":133,"end":346},"obj":"Sentence"},{"id":"T236","span":{"begin":347,"end":561},"obj":"Sentence"},{"id":"T237","span":{"begin":562,"end":803},"obj":"Sentence"},{"id":"T238","span":{"begin":804,"end":869},"obj":"Sentence"},{"id":"T239","span":{"begin":870,"end":1000},"obj":"Sentence"},{"id":"T240","span":{"begin":1001,"end":1187},"obj":"Sentence"},{"id":"T241","span":{"begin":1188,"end":1234},"obj":"Sentence"},{"id":"T242","span":{"begin":1235,"end":1279},"obj":"Sentence"},{"id":"T243","span":{"begin":1280,"end":1319},"obj":"Sentence"},{"id":"T244","span":{"begin":1320,"end":1343},"obj":"Sentence"},{"id":"T245","span":{"begin":1344,"end":1364},"obj":"Sentence"},{"id":"T246","span":{"begin":1365,"end":1391},"obj":"Sentence"},{"id":"T247","span":{"begin":1392,"end":1482},"obj":"Sentence"},{"id":"T248","span":{"begin":1483,"end":1559},"obj":"Sentence"},{"id":"T249","span":{"begin":1560,"end":1625},"obj":"Sentence"},{"id":"T250","span":{"begin":1626,"end":1709},"obj":"Sentence"},{"id":"T251","span":{"begin":1710,"end":1774},"obj":"Sentence"},{"id":"T252","span":{"begin":1775,"end":1840},"obj":"Sentence"},{"id":"T253","span":{"begin":1841,"end":1916},"obj":"Sentence"},{"id":"T254","span":{"begin":1917,"end":1984},"obj":"Sentence"},{"id":"T255","span":{"begin":1985,"end":2031},"obj":"Sentence"},{"id":"T256","span":{"begin":2032,"end":2072},"obj":"Sentence"},{"id":"T257","span":{"begin":2073,"end":2113},"obj":"Sentence"},{"id":"T258","span":{"begin":2114,"end":2145},"obj":"Sentence"},{"id":"T259","span":{"begin":2146,"end":2177},"obj":"Sentence"},{"id":"T260","span":{"begin":2178,"end":2223},"obj":"Sentence"},{"id":"T261","span":{"begin":2224,"end":2314},"obj":"Sentence"},{"id":"T262","span":{"begin":2315,"end":2391},"obj":"Sentence"},{"id":"T263","span":{"begin":2392,"end":2453},"obj":"Sentence"},{"id":"T264","span":{"begin":2454,"end":2534},"obj":"Sentence"},{"id":"T265","span":{"begin":2535,"end":2595},"obj":"Sentence"},{"id":"T266","span":{"begin":2596,"end":2661},"obj":"Sentence"},{"id":"T267","span":{"begin":2662,"end":2738},"obj":"Sentence"},{"id":"T268","span":{"begin":2739,"end":2804},"obj":"Sentence"},{"id":"T269","span":{"begin":2805,"end":2851},"obj":"Sentence"},{"id":"T270","span":{"begin":2852,"end":2892},"obj":"Sentence"},{"id":"T271","span":{"begin":2893,"end":2933},"obj":"Sentence"},{"id":"T272","span":{"begin":2934,"end":2965},"obj":"Sentence"},{"id":"T273","span":{"begin":2966,"end":2997},"obj":"Sentence"},{"id":"T274","span":{"begin":2998,"end":3054},"obj":"Sentence"},{"id":"T275","span":{"begin":3055,"end":3267},"obj":"Sentence"},{"id":"T276","span":{"begin":3268,"end":3583},"obj":"Sentence"},{"id":"T277","span":{"begin":3584,"end":3683},"obj":"Sentence"},{"id":"T278","span":{"begin":3684,"end":3779},"obj":"Sentence"}],"namespaces":[{"prefix":"_base","uri":"http://pubannotation.org/ontology/tao.owl#"}],"text":"Table 3 reports the estimation results of the OLS and IV regressions of Eq. 2, in which only within-city transmission is considered. After controlling for time-invariant city fixed effects and time effects that are common to all cities, on average, one new infection leads to 1.142 more cases in the next week, but 0.824 fewer cases 1 week later. The negative effect can be attributed to the fact that both local authorities and residents would have taken more protective measures in response to a higher perceived risk of contracting the virus given more time. Information disclosure on newly confirmed cases at the daily level by official media and information dissemination on social media throughout China may have promoted more timely actions by the public, resulting in slower virus transmissions. We then compare the transmission rates in different time windows. In the first sub-sample, one new infection leads to 2.135 more cases within a week, implying a fast growth in the number of cases. However, in the second sub-sample, the effect decreases to 1.077, suggesting that public health measures imposed in late January were effective in limiting a further spread of the virus. Similar patterns are also observed in model B.\nTable 3 Within-city transmission of COVID-19\nJan 19–Feb 29 Jan 19–Feb 1 Feb 2–Feb 29\n(1) (2) (3) (4) (5) (6)\nOLS IV OLS IV OLS IV\nAll cities excluding Wuhan\nModel A: lagged variables are averages over the preceding first and second week separately\nAverage # of new cases 0.873*** 1.142*** 1.692*** 2.135*** 0.768*** 1.077***\n1-week lag (0.00949) (0.0345) (0.0312) (0.0549) (0.0120) (0.0203)\nAverage # of new cases − 0.415*** − 0.824*** 0.860 − 6.050*** − 0.408*** − 0.796***\n2-week lag (0.00993) (0.0432) (2.131) (2.314) (0.00695) (0.0546)\nModel B: lagged variables are averages over the preceding 2 weeks\nAverage # of new case 0.474*** 0.720*** 3.310*** 3.860*** 0.494*** 1.284***\nPrevious 14 days (0.0327) (0.143) (0.223) (0.114) (0.00859) (0.107)\nObservations 12,768 12,768 4256 4256 8512 8512\nNumber of cities 304 304 304 304 304 304\nWeather controls Yes Yes Yes Yes Yes Yes\nCity FE Yes Yes Yes Yes Yes Yes\nDate FE Yes Yes Yes Yes Yes Yes\nAll cities excluding cities in Hubei Province\nModel A: lagged variables are averages over the preceding first and second week separately\nAverage # of new cases 0.725*** 1.113*** 1.050*** 1.483*** 0.620*** 0.903***\n1-week lag (0.141) (0.0802) (0.0828) (0.205) (0.166) (0.0349)\nAverage # of new cases − 0.394*** − 0.572*** 0.108 − 3.664 − 0.228*** − 0.341***\n2-week lag (0.0628) (0.107) (0.675) (2.481) (0.0456) (0.121)\nModel B: lagged variables are averages over the preceding 2 weeks\nAverage # of new cases 0.357*** 0.631*** 1.899*** 2.376*** 0.493*** 0.745***\nPrevious 14 days (0.0479) (0.208) (0.250) (0.346) (0.122) (0.147)\nObservations 12,096 12,096 4032 4032 8064 8064\nNumber of cities 288 288 288 288 288 288\nWeather controls Yes Yes Yes Yes Yes Yes\nCity FE Yes Yes Yes Yes Yes Yes\nDate FE Yes Yes Yes Yes Yes Yes\nThe dependent variable is the number of daily new cases. The endogenous explanatory variables include the average numbers of new confirmed cases in the own city in the preceding first and second weeks (model A) and the average number in the preceding 14 days (model B). Weekly averages of daily maximum temperature, precipitation, wind speed, the interaction between precipitation and wind speed, and the inverse log distance weighted sum of each of these variables in other cities, during the preceding third and fourth weeks, are used as instrumental variables in the IV regressions. Weather controls include contemporaneous weather variables in the preceding first and second weeks. Standard errors in parentheses are clustered by provinces. *** p \u003c 0.01, ** p \u003c 0.05, * p \u003c 0.1"}
LitCovid-PubTator
{"project":"LitCovid-PubTator","denotations":[{"id":"213","span":{"begin":1301,"end":1312},"obj":"Gene"},{"id":"215","span":{"begin":1271,"end":1279},"obj":"Disease"},{"id":"218","span":{"begin":257,"end":266},"obj":"Disease"},{"id":"219","span":{"begin":903,"end":912},"obj":"Disease"}],"attributes":[{"id":"A213","pred":"tao:has_database_id","subj":"213","obj":"Gene:2233"},{"id":"A215","pred":"tao:has_database_id","subj":"215","obj":"MESH:C000657245"},{"id":"A218","pred":"tao:has_database_id","subj":"218","obj":"MESH:D007239"},{"id":"A219","pred":"tao:has_database_id","subj":"219","obj":"MESH:D007239"}],"namespaces":[{"prefix":"Tax","uri":"https://www.ncbi.nlm.nih.gov/taxonomy/"},{"prefix":"MESH","uri":"https://id.nlm.nih.gov/mesh/"},{"prefix":"Gene","uri":"https://www.ncbi.nlm.nih.gov/gene/"},{"prefix":"CVCL","uri":"https://web.expasy.org/cellosaurus/CVCL_"}],"text":"Table 3 reports the estimation results of the OLS and IV regressions of Eq. 2, in which only within-city transmission is considered. After controlling for time-invariant city fixed effects and time effects that are common to all cities, on average, one new infection leads to 1.142 more cases in the next week, but 0.824 fewer cases 1 week later. The negative effect can be attributed to the fact that both local authorities and residents would have taken more protective measures in response to a higher perceived risk of contracting the virus given more time. Information disclosure on newly confirmed cases at the daily level by official media and information dissemination on social media throughout China may have promoted more timely actions by the public, resulting in slower virus transmissions. We then compare the transmission rates in different time windows. In the first sub-sample, one new infection leads to 2.135 more cases within a week, implying a fast growth in the number of cases. However, in the second sub-sample, the effect decreases to 1.077, suggesting that public health measures imposed in late January were effective in limiting a further spread of the virus. Similar patterns are also observed in model B.\nTable 3 Within-city transmission of COVID-19\nJan 19–Feb 29 Jan 19–Feb 1 Feb 2–Feb 29\n(1) (2) (3) (4) (5) (6)\nOLS IV OLS IV OLS IV\nAll cities excluding Wuhan\nModel A: lagged variables are averages over the preceding first and second week separately\nAverage # of new cases 0.873*** 1.142*** 1.692*** 2.135*** 0.768*** 1.077***\n1-week lag (0.00949) (0.0345) (0.0312) (0.0549) (0.0120) (0.0203)\nAverage # of new cases − 0.415*** − 0.824*** 0.860 − 6.050*** − 0.408*** − 0.796***\n2-week lag (0.00993) (0.0432) (2.131) (2.314) (0.00695) (0.0546)\nModel B: lagged variables are averages over the preceding 2 weeks\nAverage # of new case 0.474*** 0.720*** 3.310*** 3.860*** 0.494*** 1.284***\nPrevious 14 days (0.0327) (0.143) (0.223) (0.114) (0.00859) (0.107)\nObservations 12,768 12,768 4256 4256 8512 8512\nNumber of cities 304 304 304 304 304 304\nWeather controls Yes Yes Yes Yes Yes Yes\nCity FE Yes Yes Yes Yes Yes Yes\nDate FE Yes Yes Yes Yes Yes Yes\nAll cities excluding cities in Hubei Province\nModel A: lagged variables are averages over the preceding first and second week separately\nAverage # of new cases 0.725*** 1.113*** 1.050*** 1.483*** 0.620*** 0.903***\n1-week lag (0.141) (0.0802) (0.0828) (0.205) (0.166) (0.0349)\nAverage # of new cases − 0.394*** − 0.572*** 0.108 − 3.664 − 0.228*** − 0.341***\n2-week lag (0.0628) (0.107) (0.675) (2.481) (0.0456) (0.121)\nModel B: lagged variables are averages over the preceding 2 weeks\nAverage # of new cases 0.357*** 0.631*** 1.899*** 2.376*** 0.493*** 0.745***\nPrevious 14 days (0.0479) (0.208) (0.250) (0.346) (0.122) (0.147)\nObservations 12,096 12,096 4032 4032 8064 8064\nNumber of cities 288 288 288 288 288 288\nWeather controls Yes Yes Yes Yes Yes Yes\nCity FE Yes Yes Yes Yes Yes Yes\nDate FE Yes Yes Yes Yes Yes Yes\nThe dependent variable is the number of daily new cases. The endogenous explanatory variables include the average numbers of new confirmed cases in the own city in the preceding first and second weeks (model A) and the average number in the preceding 14 days (model B). Weekly averages of daily maximum temperature, precipitation, wind speed, the interaction between precipitation and wind speed, and the inverse log distance weighted sum of each of these variables in other cities, during the preceding third and fourth weeks, are used as instrumental variables in the IV regressions. Weather controls include contemporaneous weather variables in the preceding first and second weeks. Standard errors in parentheses are clustered by provinces. *** p \u003c 0.01, ** p \u003c 0.05, * p \u003c 0.1"}