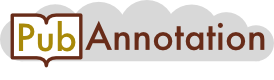
PMC:7143846 / 643-814
Annnotations
LitCovid-PubTator
{"project":"LitCovid-PubTator","denotations":[{"id":"20","span":{"begin":114,"end":121},"obj":"Disease"},{"id":"21","span":{"begin":123,"end":133},"obj":"Disease"}],"attributes":[{"id":"A20","pred":"tao:has_database_id","subj":"20","obj":"MESH:D001007"},{"id":"A21","pred":"tao:has_database_id","subj":"21","obj":"MESH:D000275"}],"namespaces":[{"prefix":"Tax","uri":"https://www.ncbi.nlm.nih.gov/taxonomy/"},{"prefix":"MESH","uri":"https://id.nlm.nih.gov/mesh/"},{"prefix":"Gene","uri":"https://www.ncbi.nlm.nih.gov/gene/"},{"prefix":"CVCL","uri":"https://web.expasy.org/cellosaurus/CVCL_"}],"text":"n several machine-learning predictive models. We calculated word frequency, scores of emotional indicators (e.g., anxiety, depression, indignation, and Oxford happiness) a"}
LitCovid-PD-MONDO
{"project":"LitCovid-PD-MONDO","denotations":[{"id":"T5","span":{"begin":114,"end":133},"obj":"Disease"},{"id":"T6","span":{"begin":114,"end":121},"obj":"Disease"},{"id":"T8","span":{"begin":123,"end":133},"obj":"Disease"}],"attributes":[{"id":"A5","pred":"mondo_id","subj":"T5","obj":"http://purl.obolibrary.org/obo/MONDO_0041086"},{"id":"A6","pred":"mondo_id","subj":"T6","obj":"http://purl.obolibrary.org/obo/MONDO_0005618"},{"id":"A7","pred":"mondo_id","subj":"T6","obj":"http://purl.obolibrary.org/obo/MONDO_0011918"},{"id":"A8","pred":"mondo_id","subj":"T8","obj":"http://purl.obolibrary.org/obo/MONDO_0002050"}],"text":"n several machine-learning predictive models. We calculated word frequency, scores of emotional indicators (e.g., anxiety, depression, indignation, and Oxford happiness) a"}
LitCovid-PD-HP
{"project":"LitCovid-PD-HP","denotations":[{"id":"T1","span":{"begin":114,"end":121},"obj":"Phenotype"},{"id":"T2","span":{"begin":123,"end":133},"obj":"Phenotype"}],"attributes":[{"id":"A1","pred":"hp_id","subj":"T1","obj":"http://purl.obolibrary.org/obo/HP_0000739"},{"id":"A2","pred":"hp_id","subj":"T2","obj":"http://purl.obolibrary.org/obo/HP_0000716"}],"text":"n several machine-learning predictive models. We calculated word frequency, scores of emotional indicators (e.g., anxiety, depression, indignation, and Oxford happiness) a"}
LitCovid-PD-GO-BP
{"project":"LitCovid-PD-GO-BP","denotations":[{"id":"T1","span":{"begin":18,"end":26},"obj":"http://purl.obolibrary.org/obo/GO_0007612"}],"text":"n several machine-learning predictive models. We calculated word frequency, scores of emotional indicators (e.g., anxiety, depression, indignation, and Oxford happiness) a"}