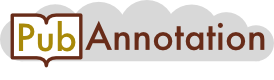
PMC:7033348 / 7549-8650
Annnotations
LitCovid-PD-MONDO
{"project":"LitCovid-PD-MONDO","denotations":[{"id":"T10","span":{"begin":182,"end":192},"obj":"Disease"},{"id":"T11","span":{"begin":213,"end":217},"obj":"Disease"},{"id":"T12","span":{"begin":219,"end":224},"obj":"Disease"},{"id":"T13","span":{"begin":235,"end":244},"obj":"Disease"},{"id":"T14","span":{"begin":250,"end":256},"obj":"Disease"},{"id":"T15","span":{"begin":844,"end":848},"obj":"Disease"}],"attributes":[{"id":"A10","pred":"mondo_id","subj":"T10","obj":"http://purl.obolibrary.org/obo/MONDO_0005550"},{"id":"A11","pred":"mondo_id","subj":"T11","obj":"http://purl.obolibrary.org/obo/MONDO_0005091"},{"id":"A12","pred":"mondo_id","subj":"T12","obj":"http://purl.obolibrary.org/obo/MONDO_0005737"},{"id":"A13","pred":"mondo_id","subj":"T13","obj":"http://purl.obolibrary.org/obo/MONDO_0005812"},{"id":"A14","pred":"mondo_id","subj":"T14","obj":"http://purl.obolibrary.org/obo/MONDO_0005502"},{"id":"A15","pred":"mondo_id","subj":"T15","obj":"http://purl.obolibrary.org/obo/MONDO_0005091"}],"text":"We generate short-term forecasts in real-time using three phenomenological models that have been previously used to derive short-term forecasts for a number of epidemics for several infectious diseases, including SARS, Ebola, pandemic influenza, and dengue (Chowell, Tariq, \u0026 Hyman, 2019; Pell et al., 2018; Wang, Wu, \u0026 Yang, 2012). The generalized logistic growth model (GLM) extends the simple logistic growth model to accommodate sub-exponential growth dynamics with a scaling of growth parameter, p (Viboud, Simonsen, \u0026 Chowell, 2016). The Richards model also includes a scaling parameter, a, to allow for deviation from the symmetric logistic curve (Chowell, 2017; Richards, 1959; Wang et al., 2012). We also include a recently developed sub-epidemic wave model that supports complex epidemic trajectories, including multiple peaks (i.e., SARS in Singapore (Chowell et al., 2019)). In this approach, the observed reported curve is assumed to be the aggregate of multiple underlying sub-epidemics (Chowell et al., 2019). A detailed description for each of the models is included in the Supplement."}
LitCovid-PD-CLO
{"project":"LitCovid-PD-CLO","denotations":[{"id":"T38","span":{"begin":148,"end":149},"obj":"http://purl.obolibrary.org/obo/CLO_0001020"},{"id":"T39","span":{"begin":302,"end":306},"obj":"http://purl.obolibrary.org/obo/CLO_0001185"},{"id":"T40","span":{"begin":470,"end":471},"obj":"http://purl.obolibrary.org/obo/CLO_0001020"},{"id":"T41","span":{"begin":573,"end":574},"obj":"http://purl.obolibrary.org/obo/CLO_0001020"},{"id":"T42","span":{"begin":594,"end":595},"obj":"http://purl.obolibrary.org/obo/CLO_0001020"},{"id":"T43","span":{"begin":722,"end":723},"obj":"http://purl.obolibrary.org/obo/CLO_0001020"},{"id":"T44","span":{"begin":1025,"end":1026},"obj":"http://purl.obolibrary.org/obo/CLO_0001020"}],"text":"We generate short-term forecasts in real-time using three phenomenological models that have been previously used to derive short-term forecasts for a number of epidemics for several infectious diseases, including SARS, Ebola, pandemic influenza, and dengue (Chowell, Tariq, \u0026 Hyman, 2019; Pell et al., 2018; Wang, Wu, \u0026 Yang, 2012). The generalized logistic growth model (GLM) extends the simple logistic growth model to accommodate sub-exponential growth dynamics with a scaling of growth parameter, p (Viboud, Simonsen, \u0026 Chowell, 2016). The Richards model also includes a scaling parameter, a, to allow for deviation from the symmetric logistic curve (Chowell, 2017; Richards, 1959; Wang et al., 2012). We also include a recently developed sub-epidemic wave model that supports complex epidemic trajectories, including multiple peaks (i.e., SARS in Singapore (Chowell et al., 2019)). In this approach, the observed reported curve is assumed to be the aggregate of multiple underlying sub-epidemics (Chowell et al., 2019). A detailed description for each of the models is included in the Supplement."}
LitCovid-PD-GO-BP
{"project":"LitCovid-PD-GO-BP","denotations":[{"id":"T5","span":{"begin":358,"end":364},"obj":"http://purl.obolibrary.org/obo/GO_0040007"},{"id":"T6","span":{"begin":405,"end":411},"obj":"http://purl.obolibrary.org/obo/GO_0040007"},{"id":"T7","span":{"begin":449,"end":455},"obj":"http://purl.obolibrary.org/obo/GO_0040007"},{"id":"T8","span":{"begin":483,"end":489},"obj":"http://purl.obolibrary.org/obo/GO_0040007"}],"text":"We generate short-term forecasts in real-time using three phenomenological models that have been previously used to derive short-term forecasts for a number of epidemics for several infectious diseases, including SARS, Ebola, pandemic influenza, and dengue (Chowell, Tariq, \u0026 Hyman, 2019; Pell et al., 2018; Wang, Wu, \u0026 Yang, 2012). The generalized logistic growth model (GLM) extends the simple logistic growth model to accommodate sub-exponential growth dynamics with a scaling of growth parameter, p (Viboud, Simonsen, \u0026 Chowell, 2016). The Richards model also includes a scaling parameter, a, to allow for deviation from the symmetric logistic curve (Chowell, 2017; Richards, 1959; Wang et al., 2012). We also include a recently developed sub-epidemic wave model that supports complex epidemic trajectories, including multiple peaks (i.e., SARS in Singapore (Chowell et al., 2019)). In this approach, the observed reported curve is assumed to be the aggregate of multiple underlying sub-epidemics (Chowell et al., 2019). A detailed description for each of the models is included in the Supplement."}
LitCovid-sentences
{"project":"LitCovid-sentences","denotations":[{"id":"T45","span":{"begin":0,"end":332},"obj":"Sentence"},{"id":"T46","span":{"begin":333,"end":539},"obj":"Sentence"},{"id":"T47","span":{"begin":540,"end":705},"obj":"Sentence"},{"id":"T48","span":{"begin":706,"end":886},"obj":"Sentence"},{"id":"T49","span":{"begin":887,"end":1024},"obj":"Sentence"},{"id":"T50","span":{"begin":1025,"end":1101},"obj":"Sentence"}],"namespaces":[{"prefix":"_base","uri":"http://pubannotation.org/ontology/tao.owl#"}],"text":"We generate short-term forecasts in real-time using three phenomenological models that have been previously used to derive short-term forecasts for a number of epidemics for several infectious diseases, including SARS, Ebola, pandemic influenza, and dengue (Chowell, Tariq, \u0026 Hyman, 2019; Pell et al., 2018; Wang, Wu, \u0026 Yang, 2012). The generalized logistic growth model (GLM) extends the simple logistic growth model to accommodate sub-exponential growth dynamics with a scaling of growth parameter, p (Viboud, Simonsen, \u0026 Chowell, 2016). The Richards model also includes a scaling parameter, a, to allow for deviation from the symmetric logistic curve (Chowell, 2017; Richards, 1959; Wang et al., 2012). We also include a recently developed sub-epidemic wave model that supports complex epidemic trajectories, including multiple peaks (i.e., SARS in Singapore (Chowell et al., 2019)). In this approach, the observed reported curve is assumed to be the aggregate of multiple underlying sub-epidemics (Chowell et al., 2019). A detailed description for each of the models is included in the Supplement."}
LitCovid-PubTator
{"project":"LitCovid-PubTator","denotations":[{"id":"27","span":{"begin":182,"end":201},"obj":"Disease"},{"id":"28","span":{"begin":213,"end":217},"obj":"Disease"},{"id":"29","span":{"begin":844,"end":848},"obj":"Disease"}],"attributes":[{"id":"A27","pred":"tao:has_database_id","subj":"27","obj":"MESH:D003141"},{"id":"A28","pred":"tao:has_database_id","subj":"28","obj":"MESH:D045169"},{"id":"A29","pred":"tao:has_database_id","subj":"29","obj":"MESH:D045169"}],"namespaces":[{"prefix":"Tax","uri":"https://www.ncbi.nlm.nih.gov/taxonomy/"},{"prefix":"MESH","uri":"https://id.nlm.nih.gov/mesh/"},{"prefix":"Gene","uri":"https://www.ncbi.nlm.nih.gov/gene/"},{"prefix":"CVCL","uri":"https://web.expasy.org/cellosaurus/CVCL_"}],"text":"We generate short-term forecasts in real-time using three phenomenological models that have been previously used to derive short-term forecasts for a number of epidemics for several infectious diseases, including SARS, Ebola, pandemic influenza, and dengue (Chowell, Tariq, \u0026 Hyman, 2019; Pell et al., 2018; Wang, Wu, \u0026 Yang, 2012). The generalized logistic growth model (GLM) extends the simple logistic growth model to accommodate sub-exponential growth dynamics with a scaling of growth parameter, p (Viboud, Simonsen, \u0026 Chowell, 2016). The Richards model also includes a scaling parameter, a, to allow for deviation from the symmetric logistic curve (Chowell, 2017; Richards, 1959; Wang et al., 2012). We also include a recently developed sub-epidemic wave model that supports complex epidemic trajectories, including multiple peaks (i.e., SARS in Singapore (Chowell et al., 2019)). In this approach, the observed reported curve is assumed to be the aggregate of multiple underlying sub-epidemics (Chowell et al., 2019). A detailed description for each of the models is included in the Supplement."}
2_test
{"project":"2_test","denotations":[{"id":"32110742-27913131-47437629","span":{"begin":302,"end":306},"obj":"27913131"},{"id":"32110742-22889641-47437630","span":{"begin":326,"end":330},"obj":"22889641"},{"id":"32110742-27266847-47437631","span":{"begin":533,"end":537},"obj":"27266847"},{"id":"32110742-22889641-47437632","span":{"begin":699,"end":703},"obj":"22889641"},{"id":"T48089","span":{"begin":302,"end":306},"obj":"27913131"},{"id":"T63529","span":{"begin":326,"end":330},"obj":"22889641"},{"id":"T61091","span":{"begin":533,"end":537},"obj":"27266847"},{"id":"T25904","span":{"begin":699,"end":703},"obj":"22889641"}],"text":"We generate short-term forecasts in real-time using three phenomenological models that have been previously used to derive short-term forecasts for a number of epidemics for several infectious diseases, including SARS, Ebola, pandemic influenza, and dengue (Chowell, Tariq, \u0026 Hyman, 2019; Pell et al., 2018; Wang, Wu, \u0026 Yang, 2012). The generalized logistic growth model (GLM) extends the simple logistic growth model to accommodate sub-exponential growth dynamics with a scaling of growth parameter, p (Viboud, Simonsen, \u0026 Chowell, 2016). The Richards model also includes a scaling parameter, a, to allow for deviation from the symmetric logistic curve (Chowell, 2017; Richards, 1959; Wang et al., 2012). We also include a recently developed sub-epidemic wave model that supports complex epidemic trajectories, including multiple peaks (i.e., SARS in Singapore (Chowell et al., 2019)). In this approach, the observed reported curve is assumed to be the aggregate of multiple underlying sub-epidemics (Chowell et al., 2019). A detailed description for each of the models is included in the Supplement."}