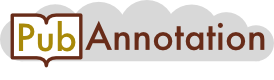
PMC:7029158 / 16231-25683
Annnotations
LitCovid-PD-MONDO
{"project":"LitCovid-PD-MONDO","denotations":[{"id":"T24","span":{"begin":2157,"end":2167},"obj":"Disease"},{"id":"T25","span":{"begin":3004,"end":3018},"obj":"Disease"},{"id":"T26","span":{"begin":3164,"end":3168},"obj":"Disease"},{"id":"T27","span":{"begin":3628,"end":3638},"obj":"Disease"},{"id":"T28","span":{"begin":4510,"end":4520},"obj":"Disease"},{"id":"T29","span":{"begin":5171,"end":5181},"obj":"Disease"},{"id":"T30","span":{"begin":7707,"end":7711},"obj":"Disease"},{"id":"T31","span":{"begin":7790,"end":7794},"obj":"Disease"},{"id":"T32","span":{"begin":7877,"end":7897},"obj":"Disease"},{"id":"T33","span":{"begin":7888,"end":7897},"obj":"Disease"},{"id":"T34","span":{"begin":8118,"end":8122},"obj":"Disease"}],"attributes":[{"id":"A24","pred":"mondo_id","subj":"T24","obj":"http://purl.obolibrary.org/obo/MONDO_0005550"},{"id":"A25","pred":"mondo_id","subj":"T25","obj":"http://purl.obolibrary.org/obo/MONDO_0005550"},{"id":"A26","pred":"mondo_id","subj":"T26","obj":"http://purl.obolibrary.org/obo/MONDO_0005091"},{"id":"A27","pred":"mondo_id","subj":"T27","obj":"http://purl.obolibrary.org/obo/MONDO_0005550"},{"id":"A28","pred":"mondo_id","subj":"T28","obj":"http://purl.obolibrary.org/obo/MONDO_0005550"},{"id":"A29","pred":"mondo_id","subj":"T29","obj":"http://purl.obolibrary.org/obo/MONDO_0005550"},{"id":"A30","pred":"mondo_id","subj":"T30","obj":"http://purl.obolibrary.org/obo/MONDO_0005091"},{"id":"A31","pred":"mondo_id","subj":"T31","obj":"http://purl.obolibrary.org/obo/MONDO_0005091"},{"id":"A32","pred":"mondo_id","subj":"T32","obj":"http://purl.obolibrary.org/obo/MONDO_0043544"},{"id":"A33","pred":"mondo_id","subj":"T33","obj":"http://purl.obolibrary.org/obo/MONDO_0005550"},{"id":"A34","pred":"mondo_id","subj":"T34","obj":"http://purl.obolibrary.org/obo/MONDO_0005091"}],"text":"Discussion\nIn our previous article (Tang et al., 2020), we had estimated a reproduction number of 6.47 (95% CI 5.71–7.23), which represents a higher value than those so far computed. For instance, the WHO has estimated a reproduction number of 1.4–2.5, Li and colleagues (Li et al., 2020) have computed a slightly higher value of 2.2 (95% CI 1.4 to 3.9), while in several other mathematical models which have been so far devised and released as pre-prints or undergone peer-review and published, the reproduction number varies from 1.3 to 4.7.\nWithin the existing literature, two studies estimated under-estimation of coronavirus cases: the investigations by Zhao and collaborators (Zhao et al., 2020) and by Read et al. (Read, Bridgen, Cummings, Ho, \u0026 Jewell, 2020). Specifically, Zhao and coworkers (Zhao et al., 2020) have assessed from a quantitative standpoint the under-reporting rate of coronavirus cases, modeling the epidemic growth curve using the exponential growing Poisson process. Authors computed the number of under-reported coronavirus cases to be 469 (95% CI 403–540). Based on this estimate, the basic reproduction number was found to be 2.56 (95% CI 2.49–2.63). In a previous version of the investigation, released in the bioRxiv pre-print server, the reproduction number was computed to oscillate between 3.30 (95% CI 2.73–3.96) and 5.47 (95% CI 4.16–7.10). The basic reproduction number was also found to be associated with 0-fold–2-fold increase in the reporting rate. More in detail, with report rates increasing over the time, the mean value was statistically likely to be higher than 3 but less than 5. Read and coauthors (Read et al., 2020) used a deterministic SEIR model, assuming coronavirus cases being Poisson distributed and with parameter inference being achieved by maximum likelihood estimation utilizing the Nelder-Mead optimization approach. According to the dynamics transmission model, an ascertainment rate of 5.0% (95% CI 3.6–7.4) was computed and, based on this, authors estimated that as of January 22nd, 2020 in Wuhan there were 14,464 (95% CI 6510–25095) infected individuals, and 21,022 (95% CI 11,090–33,490) infections. The basic reproduction number was computed to be 3.11 (95% CI 2.39–4.13). In terms of public health implications, in order to stop the outbreak, at least 58–76% of transmissions should be blocked.\nThere are three further models incorporating data from international travels: the models of Imai and coauthors (Imai et al., 2020), of Kucharski et al. (Kucharski et al., 2020) and of Wu and collaborators (Wu, Leung, \u0026 Leung, 2020). In particular, Imai and coworkers (Imai et al., 2020) estimated a reproduction number of 2.6 (uncertainty range: 1.5–3.5). Depending on the different scenarios and levels of zoonotic exposure, the reproduction number was found to vary from 1.7 to 2.6 to 1.9–4.2. Depending on the different estimates of generation time, the reproduction number oscillated from 1.3 to 2.7 to 1.7–4.3. Based on the level of infectiousness, the reproduction number varied in the range of 1.6–2.9. Finally, assuming that the novel virus would cause more mild-to-moderate cases than the SARS virus, the reproduction number would be 2.0 (uncertainty 1.4–2.3). Moreover, authors found that only public health interventions blocking over 60% of transmission would be really effective in controlling and containing the coronavirus outbreak. Partially based on the findings of Imai and coworkers (Imai et al., 2020) and building on a SIR model, Yu (Yu, 2020) has computed a basic reproduction number of 3.5 and has estimated that only a quarantine rate of infectious population higher than 90% would enable to effectively control the coronavirus outbreak. Kucharski and colleagues (Kucharski et al., 2020) designed a stochastic SEIR model, based on the Euler-Maruyama algorithm with a 6-h time-step and with the transmission rate following a geometric Brownian motion. Time-varying reproduction number was estimated using the sequential Monte-Carlo approach. Authors utilized several datasets to overcome the issue of unreliability of some data sources and to provide real-time estimates, relying on the Poisson probability calculation. Transmission was modeled as a random process, fluctuating and varying over the time. Similar to the model of Imai and coworkers (Imai et al., 2020), the risk of transmission and the risk of causing a large outbreak were modeled based on a negative binomial offspring distribution, with incubation and infectious period being Erlang distributed. Median reproduction number was found to oscillate between 1.6 and 2.9 before the introduction and implementation of travel restriction. The study by Wu and collaborators (Wu et al., 2020), based on nowcasting and forecasting approach, estimated a basic reproductive number of 2.68 (95% credible interval or CrI 2.47–2.86) with 75,815 individuals (95% CrI 37,304–130,330) being infected in Wuhan as of January 25th, 2020. The epidemics doubling time was found to be 6.4 days (95% CrI 5.8–7.1). The dynamics transmission model by Shen and coworkers (Shen, Peng, Xiao, \u0026 Zhang, 2020) predicted 8042 (95% CI 4199–11,884) infections and 898 (95% CI 368–1429) deaths, with a fatality rate of 11.02% (95% CI 9.26–12.78%). Authors computed a basic reproduction number of 4.71 (95% CI 4.50–4.92), which decreased to 2.08 (95% CI 1.99–2.18) on January 22nd, 2020. Based on these estimates, the pandemics outbreak is expected to significantly decrease within 77 [95% CI 75–80] days from its beginning. Furthermore, authors found that every one-day reduction in the duration of the period from illness/symptom onset to isolation would reduce the peak population size by 72–84% and the cumulative infected cases and deaths both by 68–80%. The study by Majumder and Mandl (Majumder \u0026 Mandl, 2020) utilized the “Incidence Decay and Exponential Adjustment” (IDEA) model and led to an estimate of the reproduction number in the range of 2.0–3.1. Finally, Riou and Althaus (Riou \u0026 Althaus, 2020), using a stochastic model simulating epidemics trajectories, computed a reproduction number of 2.2 (90% high density interval 1.4–3.8). Using statistical approaches, namely exponential growth and maximum likelihood techniques, Liu and colleagues (Liu et al., 2020) estimated the value of the reproduction number ranging from 2.90 (95% CI 2.32–3.63) to 2.92 (95% CI 2.28–3.67). Zhang and Wang (Zhang \u0026 Wang, 2020), employing a Bayesian framework to infer time-calibrated phylogeny from 33 available genomic sequences, found that the time of the most recent common ancestor (MRCA) was December 17th, 2019 (95% highest posterior density interval from December 7th, 2019 to December 23rd, 2019) and that the value of the reproduction number oscillated between 1.1 and 1.6.\nThese different findings may be due to several methodological issues, including different assumptions and choice of parameters, utilized models (stochastic versus deterministic, compartmental versus IDEA, etc.), used datasets and estimation period. Furthermore, by comparing the various updated versions of the above-mentioned investigations, the reproduction number was found to vary, reflecting the dynamics of transmission of the coronavirus outbreak as well as the dynamics of case reporting. More in detail, the reproduction number tended to increase over the time in parallel with the increase in cases being reported and the findings were highly sensitive and dependent on the period in which the estimate was made and on the data available at that given time. It should be mentioned that much of the model frameworks and data fitting and analysis have been developed from earlier studies about the SARS outbreak (Chowell et al., 2004; Gumel et al., 2004). It was believed that the SARS outbreak was characterized by a large basic reproduction number within hospitals (nosocomial infection) and a relatively small basic reproduction number in the general community, leading to a moderate basic production number overall. We argue that the current 2019 n-Cov situation is similar to what was observed about the SARS, except that the entire city of Wuhan is the epicenter with a population of over 11 million inhabitants, and the community is the entire country due to the travels done before the shutdown of the epidemic center.\nThe outbreak situation is fast evolving both in the epicenter of Wuhan, the Hubei province and throughout China. Our simulations show that the control outcome depends highly on the interventions implemented in the field, which depend on the resources provided to the frontline workers and patients infected. The size of peak value and peak time depends on a number of factors including in particular the speed of diagnoses and hospitalization of confirmed cases.\nThere are essential differences between Wuhan, Hubei, and the rest of the country as we discussed above, therefore, a more realistic model should involve the Wuhan-Hubei-China coupled system with different initial data, varying resources, and changing mobility patterns from the epicenter to the province, and the rest of the country. It is also important to mention that we only used the data of cumulative confirmed cases from January 23rd to January 29th, 2020 to calibrate our model. Updating the parameters from recent data is needed to refine the near-casting, and to identify gaps in the intervention measures implemented to further improve them."}
LitCovid-PD-CLO
{"project":"LitCovid-PD-CLO","denotations":[{"id":"T83","span":{"begin":73,"end":74},"obj":"http://purl.obolibrary.org/obo/CLO_0001020"},{"id":"T84","span":{"begin":140,"end":141},"obj":"http://purl.obolibrary.org/obo/CLO_0001020"},{"id":"T85","span":{"begin":205,"end":208},"obj":"http://purl.obolibrary.org/obo/CLO_0051582"},{"id":"T86","span":{"begin":219,"end":220},"obj":"http://purl.obolibrary.org/obo/CLO_0001020"},{"id":"T87","span":{"begin":253,"end":255},"obj":"http://purl.obolibrary.org/obo/CLO_0001022"},{"id":"T88","span":{"begin":253,"end":255},"obj":"http://purl.obolibrary.org/obo/CLO_0007314"},{"id":"T89","span":{"begin":272,"end":274},"obj":"http://purl.obolibrary.org/obo/CLO_0001022"},{"id":"T90","span":{"begin":272,"end":274},"obj":"http://purl.obolibrary.org/obo/CLO_0007314"},{"id":"T91","span":{"begin":303,"end":304},"obj":"http://purl.obolibrary.org/obo/CLO_0001020"},{"id":"T92","span":{"begin":469,"end":473},"obj":"http://purl.obolibrary.org/obo/CLO_0008416"},{"id":"T93","span":{"begin":469,"end":473},"obj":"http://purl.obolibrary.org/obo/CLO_0050081"},{"id":"T94","span":{"begin":840,"end":841},"obj":"http://purl.obolibrary.org/obo/CLO_0001020"},{"id":"T95","span":{"begin":1185,"end":1186},"obj":"http://purl.obolibrary.org/obo/CLO_0001020"},{"id":"T96","span":{"begin":1673,"end":1674},"obj":"http://purl.obolibrary.org/obo/CLO_0001020"},{"id":"T97","span":{"begin":2663,"end":2664},"obj":"http://purl.obolibrary.org/obo/CLO_0001020"},{"id":"T98","span":{"begin":3109,"end":3114},"obj":"http://purl.obolibrary.org/obo/NCBITaxon_10239"},{"id":"T99","span":{"begin":3169,"end":3174},"obj":"http://purl.obolibrary.org/obo/NCBITaxon_10239"},{"id":"T100","span":{"begin":3504,"end":3505},"obj":"http://purl.obolibrary.org/obo/CLO_0001020"},{"id":"T101","span":{"begin":3531,"end":3534},"obj":"http://purl.obolibrary.org/obo/CLO_0051582"},{"id":"T102","span":{"begin":3544,"end":3545},"obj":"http://purl.obolibrary.org/obo/CLO_0001020"},{"id":"T103","span":{"begin":3583,"end":3586},"obj":"http://purl.obolibrary.org/obo/CLO_0051582"},{"id":"T104","span":{"begin":3607,"end":3608},"obj":"http://purl.obolibrary.org/obo/CLO_0001020"},{"id":"T105","span":{"begin":3787,"end":3788},"obj":"http://purl.obolibrary.org/obo/CLO_0001020"},{"id":"T106","span":{"begin":3855,"end":3858},"obj":"http://purl.obolibrary.org/obo/CLO_0001602"},{"id":"T107","span":{"begin":3855,"end":3858},"obj":"http://purl.obolibrary.org/obo/CLO_0001603"},{"id":"T108","span":{"begin":3855,"end":3858},"obj":"http://purl.obolibrary.org/obo/CLO_0050248"},{"id":"T109","span":{"begin":3855,"end":3858},"obj":"http://purl.obolibrary.org/obo/CLO_0052463"},{"id":"T110","span":{"begin":3912,"end":3913},"obj":"http://purl.obolibrary.org/obo/CLO_0001020"},{"id":"T111","span":{"begin":4237,"end":4238},"obj":"http://purl.obolibrary.org/obo/CLO_0001020"},{"id":"T112","span":{"begin":4407,"end":4408},"obj":"http://purl.obolibrary.org/obo/CLO_0001020"},{"id":"T113","span":{"begin":4446,"end":4447},"obj":"http://purl.obolibrary.org/obo/CLO_0001020"},{"id":"T114","span":{"begin":4799,"end":4800},"obj":"http://purl.obolibrary.org/obo/CLO_0001020"},{"id":"T115","span":{"begin":5221,"end":5222},"obj":"http://purl.obolibrary.org/obo/CLO_0001020"},{"id":"T116","span":{"begin":5286,"end":5287},"obj":"http://purl.obolibrary.org/obo/CLO_0001020"},{"id":"T117","span":{"begin":6039,"end":6040},"obj":"http://purl.obolibrary.org/obo/CLO_0001020"},{"id":"T118","span":{"begin":6102,"end":6103},"obj":"http://purl.obolibrary.org/obo/CLO_0001020"},{"id":"T119","span":{"begin":6456,"end":6457},"obj":"http://purl.obolibrary.org/obo/CLO_0001020"},{"id":"T120","span":{"begin":6648,"end":6657},"obj":"http://purl.obolibrary.org/obo/UBERON_0001353"},{"id":"T121","span":{"begin":7825,"end":7826},"obj":"http://purl.obolibrary.org/obo/CLO_0001020"},{"id":"T122","span":{"begin":7903,"end":7904},"obj":"http://purl.obolibrary.org/obo/CLO_0001020"},{"id":"T123","span":{"begin":7985,"end":7986},"obj":"http://purl.obolibrary.org/obo/CLO_0001020"},{"id":"T124","span":{"begin":8183,"end":8184},"obj":"http://purl.obolibrary.org/obo/CLO_0001020"},{"id":"T125","span":{"begin":8204,"end":8206},"obj":"http://purl.obolibrary.org/obo/CLO_0053733"},{"id":"T126","span":{"begin":8550,"end":8555},"obj":"http://purl.obolibrary.org/obo/UBERON_0007688"},{"id":"T127","span":{"begin":8692,"end":8693},"obj":"http://purl.obolibrary.org/obo/CLO_0001020"},{"id":"T128","span":{"begin":8915,"end":8916},"obj":"http://purl.obolibrary.org/obo/CLO_0001020"}],"text":"Discussion\nIn our previous article (Tang et al., 2020), we had estimated a reproduction number of 6.47 (95% CI 5.71–7.23), which represents a higher value than those so far computed. For instance, the WHO has estimated a reproduction number of 1.4–2.5, Li and colleagues (Li et al., 2020) have computed a slightly higher value of 2.2 (95% CI 1.4 to 3.9), while in several other mathematical models which have been so far devised and released as pre-prints or undergone peer-review and published, the reproduction number varies from 1.3 to 4.7.\nWithin the existing literature, two studies estimated under-estimation of coronavirus cases: the investigations by Zhao and collaborators (Zhao et al., 2020) and by Read et al. (Read, Bridgen, Cummings, Ho, \u0026 Jewell, 2020). Specifically, Zhao and coworkers (Zhao et al., 2020) have assessed from a quantitative standpoint the under-reporting rate of coronavirus cases, modeling the epidemic growth curve using the exponential growing Poisson process. Authors computed the number of under-reported coronavirus cases to be 469 (95% CI 403–540). Based on this estimate, the basic reproduction number was found to be 2.56 (95% CI 2.49–2.63). In a previous version of the investigation, released in the bioRxiv pre-print server, the reproduction number was computed to oscillate between 3.30 (95% CI 2.73–3.96) and 5.47 (95% CI 4.16–7.10). The basic reproduction number was also found to be associated with 0-fold–2-fold increase in the reporting rate. More in detail, with report rates increasing over the time, the mean value was statistically likely to be higher than 3 but less than 5. Read and coauthors (Read et al., 2020) used a deterministic SEIR model, assuming coronavirus cases being Poisson distributed and with parameter inference being achieved by maximum likelihood estimation utilizing the Nelder-Mead optimization approach. According to the dynamics transmission model, an ascertainment rate of 5.0% (95% CI 3.6–7.4) was computed and, based on this, authors estimated that as of January 22nd, 2020 in Wuhan there were 14,464 (95% CI 6510–25095) infected individuals, and 21,022 (95% CI 11,090–33,490) infections. The basic reproduction number was computed to be 3.11 (95% CI 2.39–4.13). In terms of public health implications, in order to stop the outbreak, at least 58–76% of transmissions should be blocked.\nThere are three further models incorporating data from international travels: the models of Imai and coauthors (Imai et al., 2020), of Kucharski et al. (Kucharski et al., 2020) and of Wu and collaborators (Wu, Leung, \u0026 Leung, 2020). In particular, Imai and coworkers (Imai et al., 2020) estimated a reproduction number of 2.6 (uncertainty range: 1.5–3.5). Depending on the different scenarios and levels of zoonotic exposure, the reproduction number was found to vary from 1.7 to 2.6 to 1.9–4.2. Depending on the different estimates of generation time, the reproduction number oscillated from 1.3 to 2.7 to 1.7–4.3. Based on the level of infectiousness, the reproduction number varied in the range of 1.6–2.9. Finally, assuming that the novel virus would cause more mild-to-moderate cases than the SARS virus, the reproduction number would be 2.0 (uncertainty 1.4–2.3). Moreover, authors found that only public health interventions blocking over 60% of transmission would be really effective in controlling and containing the coronavirus outbreak. Partially based on the findings of Imai and coworkers (Imai et al., 2020) and building on a SIR model, Yu (Yu, 2020) has computed a basic reproduction number of 3.5 and has estimated that only a quarantine rate of infectious population higher than 90% would enable to effectively control the coronavirus outbreak. Kucharski and colleagues (Kucharski et al., 2020) designed a stochastic SEIR model, based on the Euler-Maruyama algorithm with a 6-h time-step and with the transmission rate following a geometric Brownian motion. Time-varying reproduction number was estimated using the sequential Monte-Carlo approach. Authors utilized several datasets to overcome the issue of unreliability of some data sources and to provide real-time estimates, relying on the Poisson probability calculation. Transmission was modeled as a random process, fluctuating and varying over the time. Similar to the model of Imai and coworkers (Imai et al., 2020), the risk of transmission and the risk of causing a large outbreak were modeled based on a negative binomial offspring distribution, with incubation and infectious period being Erlang distributed. Median reproduction number was found to oscillate between 1.6 and 2.9 before the introduction and implementation of travel restriction. The study by Wu and collaborators (Wu et al., 2020), based on nowcasting and forecasting approach, estimated a basic reproductive number of 2.68 (95% credible interval or CrI 2.47–2.86) with 75,815 individuals (95% CrI 37,304–130,330) being infected in Wuhan as of January 25th, 2020. The epidemics doubling time was found to be 6.4 days (95% CrI 5.8–7.1). The dynamics transmission model by Shen and coworkers (Shen, Peng, Xiao, \u0026 Zhang, 2020) predicted 8042 (95% CI 4199–11,884) infections and 898 (95% CI 368–1429) deaths, with a fatality rate of 11.02% (95% CI 9.26–12.78%). Authors computed a basic reproduction number of 4.71 (95% CI 4.50–4.92), which decreased to 2.08 (95% CI 1.99–2.18) on January 22nd, 2020. Based on these estimates, the pandemics outbreak is expected to significantly decrease within 77 [95% CI 75–80] days from its beginning. Furthermore, authors found that every one-day reduction in the duration of the period from illness/symptom onset to isolation would reduce the peak population size by 72–84% and the cumulative infected cases and deaths both by 68–80%. The study by Majumder and Mandl (Majumder \u0026 Mandl, 2020) utilized the “Incidence Decay and Exponential Adjustment” (IDEA) model and led to an estimate of the reproduction number in the range of 2.0–3.1. Finally, Riou and Althaus (Riou \u0026 Althaus, 2020), using a stochastic model simulating epidemics trajectories, computed a reproduction number of 2.2 (90% high density interval 1.4–3.8). Using statistical approaches, namely exponential growth and maximum likelihood techniques, Liu and colleagues (Liu et al., 2020) estimated the value of the reproduction number ranging from 2.90 (95% CI 2.32–3.63) to 2.92 (95% CI 2.28–3.67). Zhang and Wang (Zhang \u0026 Wang, 2020), employing a Bayesian framework to infer time-calibrated phylogeny from 33 available genomic sequences, found that the time of the most recent common ancestor (MRCA) was December 17th, 2019 (95% highest posterior density interval from December 7th, 2019 to December 23rd, 2019) and that the value of the reproduction number oscillated between 1.1 and 1.6.\nThese different findings may be due to several methodological issues, including different assumptions and choice of parameters, utilized models (stochastic versus deterministic, compartmental versus IDEA, etc.), used datasets and estimation period. Furthermore, by comparing the various updated versions of the above-mentioned investigations, the reproduction number was found to vary, reflecting the dynamics of transmission of the coronavirus outbreak as well as the dynamics of case reporting. More in detail, the reproduction number tended to increase over the time in parallel with the increase in cases being reported and the findings were highly sensitive and dependent on the period in which the estimate was made and on the data available at that given time. It should be mentioned that much of the model frameworks and data fitting and analysis have been developed from earlier studies about the SARS outbreak (Chowell et al., 2004; Gumel et al., 2004). It was believed that the SARS outbreak was characterized by a large basic reproduction number within hospitals (nosocomial infection) and a relatively small basic reproduction number in the general community, leading to a moderate basic production number overall. We argue that the current 2019 n-Cov situation is similar to what was observed about the SARS, except that the entire city of Wuhan is the epicenter with a population of over 11 million inhabitants, and the community is the entire country due to the travels done before the shutdown of the epidemic center.\nThe outbreak situation is fast evolving both in the epicenter of Wuhan, the Hubei province and throughout China. Our simulations show that the control outcome depends highly on the interventions implemented in the field, which depend on the resources provided to the frontline workers and patients infected. The size of peak value and peak time depends on a number of factors including in particular the speed of diagnoses and hospitalization of confirmed cases.\nThere are essential differences between Wuhan, Hubei, and the rest of the country as we discussed above, therefore, a more realistic model should involve the Wuhan-Hubei-China coupled system with different initial data, varying resources, and changing mobility patterns from the epicenter to the province, and the rest of the country. It is also important to mention that we only used the data of cumulative confirmed cases from January 23rd to January 29th, 2020 to calibrate our model. Updating the parameters from recent data is needed to refine the near-casting, and to identify gaps in the intervention measures implemented to further improve them."}
LitCovid-PD-CHEBI
{"project":"LitCovid-PD-CHEBI","denotations":[{"id":"T31","span":{"begin":253,"end":255},"obj":"Chemical"},{"id":"T32","span":{"begin":272,"end":274},"obj":"Chemical"},{"id":"T33","span":{"begin":747,"end":749},"obj":"Chemical"}],"attributes":[{"id":"A31","pred":"chebi_id","subj":"T31","obj":"http://purl.obolibrary.org/obo/CHEBI_30145"},{"id":"A32","pred":"chebi_id","subj":"T32","obj":"http://purl.obolibrary.org/obo/CHEBI_30145"},{"id":"A33","pred":"chebi_id","subj":"T33","obj":"http://purl.obolibrary.org/obo/CHEBI_49648"}],"text":"Discussion\nIn our previous article (Tang et al., 2020), we had estimated a reproduction number of 6.47 (95% CI 5.71–7.23), which represents a higher value than those so far computed. For instance, the WHO has estimated a reproduction number of 1.4–2.5, Li and colleagues (Li et al., 2020) have computed a slightly higher value of 2.2 (95% CI 1.4 to 3.9), while in several other mathematical models which have been so far devised and released as pre-prints or undergone peer-review and published, the reproduction number varies from 1.3 to 4.7.\nWithin the existing literature, two studies estimated under-estimation of coronavirus cases: the investigations by Zhao and collaborators (Zhao et al., 2020) and by Read et al. (Read, Bridgen, Cummings, Ho, \u0026 Jewell, 2020). Specifically, Zhao and coworkers (Zhao et al., 2020) have assessed from a quantitative standpoint the under-reporting rate of coronavirus cases, modeling the epidemic growth curve using the exponential growing Poisson process. Authors computed the number of under-reported coronavirus cases to be 469 (95% CI 403–540). Based on this estimate, the basic reproduction number was found to be 2.56 (95% CI 2.49–2.63). In a previous version of the investigation, released in the bioRxiv pre-print server, the reproduction number was computed to oscillate between 3.30 (95% CI 2.73–3.96) and 5.47 (95% CI 4.16–7.10). The basic reproduction number was also found to be associated with 0-fold–2-fold increase in the reporting rate. More in detail, with report rates increasing over the time, the mean value was statistically likely to be higher than 3 but less than 5. Read and coauthors (Read et al., 2020) used a deterministic SEIR model, assuming coronavirus cases being Poisson distributed and with parameter inference being achieved by maximum likelihood estimation utilizing the Nelder-Mead optimization approach. According to the dynamics transmission model, an ascertainment rate of 5.0% (95% CI 3.6–7.4) was computed and, based on this, authors estimated that as of January 22nd, 2020 in Wuhan there were 14,464 (95% CI 6510–25095) infected individuals, and 21,022 (95% CI 11,090–33,490) infections. The basic reproduction number was computed to be 3.11 (95% CI 2.39–4.13). In terms of public health implications, in order to stop the outbreak, at least 58–76% of transmissions should be blocked.\nThere are three further models incorporating data from international travels: the models of Imai and coauthors (Imai et al., 2020), of Kucharski et al. (Kucharski et al., 2020) and of Wu and collaborators (Wu, Leung, \u0026 Leung, 2020). In particular, Imai and coworkers (Imai et al., 2020) estimated a reproduction number of 2.6 (uncertainty range: 1.5–3.5). Depending on the different scenarios and levels of zoonotic exposure, the reproduction number was found to vary from 1.7 to 2.6 to 1.9–4.2. Depending on the different estimates of generation time, the reproduction number oscillated from 1.3 to 2.7 to 1.7–4.3. Based on the level of infectiousness, the reproduction number varied in the range of 1.6–2.9. Finally, assuming that the novel virus would cause more mild-to-moderate cases than the SARS virus, the reproduction number would be 2.0 (uncertainty 1.4–2.3). Moreover, authors found that only public health interventions blocking over 60% of transmission would be really effective in controlling and containing the coronavirus outbreak. Partially based on the findings of Imai and coworkers (Imai et al., 2020) and building on a SIR model, Yu (Yu, 2020) has computed a basic reproduction number of 3.5 and has estimated that only a quarantine rate of infectious population higher than 90% would enable to effectively control the coronavirus outbreak. Kucharski and colleagues (Kucharski et al., 2020) designed a stochastic SEIR model, based on the Euler-Maruyama algorithm with a 6-h time-step and with the transmission rate following a geometric Brownian motion. Time-varying reproduction number was estimated using the sequential Monte-Carlo approach. Authors utilized several datasets to overcome the issue of unreliability of some data sources and to provide real-time estimates, relying on the Poisson probability calculation. Transmission was modeled as a random process, fluctuating and varying over the time. Similar to the model of Imai and coworkers (Imai et al., 2020), the risk of transmission and the risk of causing a large outbreak were modeled based on a negative binomial offspring distribution, with incubation and infectious period being Erlang distributed. Median reproduction number was found to oscillate between 1.6 and 2.9 before the introduction and implementation of travel restriction. The study by Wu and collaborators (Wu et al., 2020), based on nowcasting and forecasting approach, estimated a basic reproductive number of 2.68 (95% credible interval or CrI 2.47–2.86) with 75,815 individuals (95% CrI 37,304–130,330) being infected in Wuhan as of January 25th, 2020. The epidemics doubling time was found to be 6.4 days (95% CrI 5.8–7.1). The dynamics transmission model by Shen and coworkers (Shen, Peng, Xiao, \u0026 Zhang, 2020) predicted 8042 (95% CI 4199–11,884) infections and 898 (95% CI 368–1429) deaths, with a fatality rate of 11.02% (95% CI 9.26–12.78%). Authors computed a basic reproduction number of 4.71 (95% CI 4.50–4.92), which decreased to 2.08 (95% CI 1.99–2.18) on January 22nd, 2020. Based on these estimates, the pandemics outbreak is expected to significantly decrease within 77 [95% CI 75–80] days from its beginning. Furthermore, authors found that every one-day reduction in the duration of the period from illness/symptom onset to isolation would reduce the peak population size by 72–84% and the cumulative infected cases and deaths both by 68–80%. The study by Majumder and Mandl (Majumder \u0026 Mandl, 2020) utilized the “Incidence Decay and Exponential Adjustment” (IDEA) model and led to an estimate of the reproduction number in the range of 2.0–3.1. Finally, Riou and Althaus (Riou \u0026 Althaus, 2020), using a stochastic model simulating epidemics trajectories, computed a reproduction number of 2.2 (90% high density interval 1.4–3.8). Using statistical approaches, namely exponential growth and maximum likelihood techniques, Liu and colleagues (Liu et al., 2020) estimated the value of the reproduction number ranging from 2.90 (95% CI 2.32–3.63) to 2.92 (95% CI 2.28–3.67). Zhang and Wang (Zhang \u0026 Wang, 2020), employing a Bayesian framework to infer time-calibrated phylogeny from 33 available genomic sequences, found that the time of the most recent common ancestor (MRCA) was December 17th, 2019 (95% highest posterior density interval from December 7th, 2019 to December 23rd, 2019) and that the value of the reproduction number oscillated between 1.1 and 1.6.\nThese different findings may be due to several methodological issues, including different assumptions and choice of parameters, utilized models (stochastic versus deterministic, compartmental versus IDEA, etc.), used datasets and estimation period. Furthermore, by comparing the various updated versions of the above-mentioned investigations, the reproduction number was found to vary, reflecting the dynamics of transmission of the coronavirus outbreak as well as the dynamics of case reporting. More in detail, the reproduction number tended to increase over the time in parallel with the increase in cases being reported and the findings were highly sensitive and dependent on the period in which the estimate was made and on the data available at that given time. It should be mentioned that much of the model frameworks and data fitting and analysis have been developed from earlier studies about the SARS outbreak (Chowell et al., 2004; Gumel et al., 2004). It was believed that the SARS outbreak was characterized by a large basic reproduction number within hospitals (nosocomial infection) and a relatively small basic reproduction number in the general community, leading to a moderate basic production number overall. We argue that the current 2019 n-Cov situation is similar to what was observed about the SARS, except that the entire city of Wuhan is the epicenter with a population of over 11 million inhabitants, and the community is the entire country due to the travels done before the shutdown of the epidemic center.\nThe outbreak situation is fast evolving both in the epicenter of Wuhan, the Hubei province and throughout China. Our simulations show that the control outcome depends highly on the interventions implemented in the field, which depend on the resources provided to the frontline workers and patients infected. The size of peak value and peak time depends on a number of factors including in particular the speed of diagnoses and hospitalization of confirmed cases.\nThere are essential differences between Wuhan, Hubei, and the rest of the country as we discussed above, therefore, a more realistic model should involve the Wuhan-Hubei-China coupled system with different initial data, varying resources, and changing mobility patterns from the epicenter to the province, and the rest of the country. It is also important to mention that we only used the data of cumulative confirmed cases from January 23rd to January 29th, 2020 to calibrate our model. Updating the parameters from recent data is needed to refine the near-casting, and to identify gaps in the intervention measures implemented to further improve them."}
LitCovid-PD-GO-BP
{"project":"LitCovid-PD-GO-BP","denotations":[{"id":"T19","span":{"begin":75,"end":87},"obj":"http://purl.obolibrary.org/obo/GO_0000003"},{"id":"T20","span":{"begin":221,"end":233},"obj":"http://purl.obolibrary.org/obo/GO_0000003"},{"id":"T21","span":{"begin":500,"end":512},"obj":"http://purl.obolibrary.org/obo/GO_0000003"},{"id":"T22","span":{"begin":935,"end":941},"obj":"http://purl.obolibrary.org/obo/GO_0040007"},{"id":"T23","span":{"begin":1121,"end":1133},"obj":"http://purl.obolibrary.org/obo/GO_0000003"},{"id":"T24","span":{"begin":1272,"end":1284},"obj":"http://purl.obolibrary.org/obo/GO_0000003"},{"id":"T25","span":{"begin":1389,"end":1401},"obj":"http://purl.obolibrary.org/obo/GO_0000003"},{"id":"T26","span":{"begin":2179,"end":2191},"obj":"http://purl.obolibrary.org/obo/GO_0000003"},{"id":"T27","span":{"begin":2665,"end":2677},"obj":"http://purl.obolibrary.org/obo/GO_0000003"},{"id":"T28","span":{"begin":2796,"end":2808},"obj":"http://purl.obolibrary.org/obo/GO_0000003"},{"id":"T29","span":{"begin":2923,"end":2935},"obj":"http://purl.obolibrary.org/obo/GO_0000003"},{"id":"T30","span":{"begin":3024,"end":3036},"obj":"http://purl.obolibrary.org/obo/GO_0000003"},{"id":"T31","span":{"begin":3180,"end":3192},"obj":"http://purl.obolibrary.org/obo/GO_0000003"},{"id":"T32","span":{"begin":3552,"end":3564},"obj":"http://purl.obolibrary.org/obo/GO_0000003"},{"id":"T33","span":{"begin":3954,"end":3966},"obj":"http://purl.obolibrary.org/obo/GO_0000003"},{"id":"T34","span":{"begin":4561,"end":4573},"obj":"http://purl.obolibrary.org/obo/GO_0000003"},{"id":"T35","span":{"begin":5294,"end":5306},"obj":"http://purl.obolibrary.org/obo/GO_0000003"},{"id":"T36","span":{"begin":5938,"end":5950},"obj":"http://purl.obolibrary.org/obo/GO_0000003"},{"id":"T37","span":{"begin":6104,"end":6116},"obj":"http://purl.obolibrary.org/obo/GO_0000003"},{"id":"T38","span":{"begin":6217,"end":6223},"obj":"http://purl.obolibrary.org/obo/GO_0040007"},{"id":"T39","span":{"begin":6324,"end":6336},"obj":"http://purl.obolibrary.org/obo/GO_0000003"},{"id":"T40","span":{"begin":6749,"end":6761},"obj":"http://purl.obolibrary.org/obo/GO_0000003"},{"id":"T41","span":{"begin":7148,"end":7160},"obj":"http://purl.obolibrary.org/obo/GO_0000003"},{"id":"T42","span":{"begin":7318,"end":7330},"obj":"http://purl.obolibrary.org/obo/GO_0000003"},{"id":"T43","span":{"begin":7839,"end":7851},"obj":"http://purl.obolibrary.org/obo/GO_0000003"},{"id":"T44","span":{"begin":7928,"end":7940},"obj":"http://purl.obolibrary.org/obo/GO_0000003"}],"text":"Discussion\nIn our previous article (Tang et al., 2020), we had estimated a reproduction number of 6.47 (95% CI 5.71–7.23), which represents a higher value than those so far computed. For instance, the WHO has estimated a reproduction number of 1.4–2.5, Li and colleagues (Li et al., 2020) have computed a slightly higher value of 2.2 (95% CI 1.4 to 3.9), while in several other mathematical models which have been so far devised and released as pre-prints or undergone peer-review and published, the reproduction number varies from 1.3 to 4.7.\nWithin the existing literature, two studies estimated under-estimation of coronavirus cases: the investigations by Zhao and collaborators (Zhao et al., 2020) and by Read et al. (Read, Bridgen, Cummings, Ho, \u0026 Jewell, 2020). Specifically, Zhao and coworkers (Zhao et al., 2020) have assessed from a quantitative standpoint the under-reporting rate of coronavirus cases, modeling the epidemic growth curve using the exponential growing Poisson process. Authors computed the number of under-reported coronavirus cases to be 469 (95% CI 403–540). Based on this estimate, the basic reproduction number was found to be 2.56 (95% CI 2.49–2.63). In a previous version of the investigation, released in the bioRxiv pre-print server, the reproduction number was computed to oscillate between 3.30 (95% CI 2.73–3.96) and 5.47 (95% CI 4.16–7.10). The basic reproduction number was also found to be associated with 0-fold–2-fold increase in the reporting rate. More in detail, with report rates increasing over the time, the mean value was statistically likely to be higher than 3 but less than 5. Read and coauthors (Read et al., 2020) used a deterministic SEIR model, assuming coronavirus cases being Poisson distributed and with parameter inference being achieved by maximum likelihood estimation utilizing the Nelder-Mead optimization approach. According to the dynamics transmission model, an ascertainment rate of 5.0% (95% CI 3.6–7.4) was computed and, based on this, authors estimated that as of January 22nd, 2020 in Wuhan there were 14,464 (95% CI 6510–25095) infected individuals, and 21,022 (95% CI 11,090–33,490) infections. The basic reproduction number was computed to be 3.11 (95% CI 2.39–4.13). In terms of public health implications, in order to stop the outbreak, at least 58–76% of transmissions should be blocked.\nThere are three further models incorporating data from international travels: the models of Imai and coauthors (Imai et al., 2020), of Kucharski et al. (Kucharski et al., 2020) and of Wu and collaborators (Wu, Leung, \u0026 Leung, 2020). In particular, Imai and coworkers (Imai et al., 2020) estimated a reproduction number of 2.6 (uncertainty range: 1.5–3.5). Depending on the different scenarios and levels of zoonotic exposure, the reproduction number was found to vary from 1.7 to 2.6 to 1.9–4.2. Depending on the different estimates of generation time, the reproduction number oscillated from 1.3 to 2.7 to 1.7–4.3. Based on the level of infectiousness, the reproduction number varied in the range of 1.6–2.9. Finally, assuming that the novel virus would cause more mild-to-moderate cases than the SARS virus, the reproduction number would be 2.0 (uncertainty 1.4–2.3). Moreover, authors found that only public health interventions blocking over 60% of transmission would be really effective in controlling and containing the coronavirus outbreak. Partially based on the findings of Imai and coworkers (Imai et al., 2020) and building on a SIR model, Yu (Yu, 2020) has computed a basic reproduction number of 3.5 and has estimated that only a quarantine rate of infectious population higher than 90% would enable to effectively control the coronavirus outbreak. Kucharski and colleagues (Kucharski et al., 2020) designed a stochastic SEIR model, based on the Euler-Maruyama algorithm with a 6-h time-step and with the transmission rate following a geometric Brownian motion. Time-varying reproduction number was estimated using the sequential Monte-Carlo approach. Authors utilized several datasets to overcome the issue of unreliability of some data sources and to provide real-time estimates, relying on the Poisson probability calculation. Transmission was modeled as a random process, fluctuating and varying over the time. Similar to the model of Imai and coworkers (Imai et al., 2020), the risk of transmission and the risk of causing a large outbreak were modeled based on a negative binomial offspring distribution, with incubation and infectious period being Erlang distributed. Median reproduction number was found to oscillate between 1.6 and 2.9 before the introduction and implementation of travel restriction. The study by Wu and collaborators (Wu et al., 2020), based on nowcasting and forecasting approach, estimated a basic reproductive number of 2.68 (95% credible interval or CrI 2.47–2.86) with 75,815 individuals (95% CrI 37,304–130,330) being infected in Wuhan as of January 25th, 2020. The epidemics doubling time was found to be 6.4 days (95% CrI 5.8–7.1). The dynamics transmission model by Shen and coworkers (Shen, Peng, Xiao, \u0026 Zhang, 2020) predicted 8042 (95% CI 4199–11,884) infections and 898 (95% CI 368–1429) deaths, with a fatality rate of 11.02% (95% CI 9.26–12.78%). Authors computed a basic reproduction number of 4.71 (95% CI 4.50–4.92), which decreased to 2.08 (95% CI 1.99–2.18) on January 22nd, 2020. Based on these estimates, the pandemics outbreak is expected to significantly decrease within 77 [95% CI 75–80] days from its beginning. Furthermore, authors found that every one-day reduction in the duration of the period from illness/symptom onset to isolation would reduce the peak population size by 72–84% and the cumulative infected cases and deaths both by 68–80%. The study by Majumder and Mandl (Majumder \u0026 Mandl, 2020) utilized the “Incidence Decay and Exponential Adjustment” (IDEA) model and led to an estimate of the reproduction number in the range of 2.0–3.1. Finally, Riou and Althaus (Riou \u0026 Althaus, 2020), using a stochastic model simulating epidemics trajectories, computed a reproduction number of 2.2 (90% high density interval 1.4–3.8). Using statistical approaches, namely exponential growth and maximum likelihood techniques, Liu and colleagues (Liu et al., 2020) estimated the value of the reproduction number ranging from 2.90 (95% CI 2.32–3.63) to 2.92 (95% CI 2.28–3.67). Zhang and Wang (Zhang \u0026 Wang, 2020), employing a Bayesian framework to infer time-calibrated phylogeny from 33 available genomic sequences, found that the time of the most recent common ancestor (MRCA) was December 17th, 2019 (95% highest posterior density interval from December 7th, 2019 to December 23rd, 2019) and that the value of the reproduction number oscillated between 1.1 and 1.6.\nThese different findings may be due to several methodological issues, including different assumptions and choice of parameters, utilized models (stochastic versus deterministic, compartmental versus IDEA, etc.), used datasets and estimation period. Furthermore, by comparing the various updated versions of the above-mentioned investigations, the reproduction number was found to vary, reflecting the dynamics of transmission of the coronavirus outbreak as well as the dynamics of case reporting. More in detail, the reproduction number tended to increase over the time in parallel with the increase in cases being reported and the findings were highly sensitive and dependent on the period in which the estimate was made and on the data available at that given time. It should be mentioned that much of the model frameworks and data fitting and analysis have been developed from earlier studies about the SARS outbreak (Chowell et al., 2004; Gumel et al., 2004). It was believed that the SARS outbreak was characterized by a large basic reproduction number within hospitals (nosocomial infection) and a relatively small basic reproduction number in the general community, leading to a moderate basic production number overall. We argue that the current 2019 n-Cov situation is similar to what was observed about the SARS, except that the entire city of Wuhan is the epicenter with a population of over 11 million inhabitants, and the community is the entire country due to the travels done before the shutdown of the epidemic center.\nThe outbreak situation is fast evolving both in the epicenter of Wuhan, the Hubei province and throughout China. Our simulations show that the control outcome depends highly on the interventions implemented in the field, which depend on the resources provided to the frontline workers and patients infected. The size of peak value and peak time depends on a number of factors including in particular the speed of diagnoses and hospitalization of confirmed cases.\nThere are essential differences between Wuhan, Hubei, and the rest of the country as we discussed above, therefore, a more realistic model should involve the Wuhan-Hubei-China coupled system with different initial data, varying resources, and changing mobility patterns from the epicenter to the province, and the rest of the country. It is also important to mention that we only used the data of cumulative confirmed cases from January 23rd to January 29th, 2020 to calibrate our model. Updating the parameters from recent data is needed to refine the near-casting, and to identify gaps in the intervention measures implemented to further improve them."}
LitCovid-sentences
{"project":"LitCovid-sentences","denotations":[{"id":"T102","span":{"begin":0,"end":10},"obj":"Sentence"},{"id":"T103","span":{"begin":11,"end":182},"obj":"Sentence"},{"id":"T104","span":{"begin":183,"end":543},"obj":"Sentence"},{"id":"T105","span":{"begin":544,"end":767},"obj":"Sentence"},{"id":"T106","span":{"begin":768,"end":994},"obj":"Sentence"},{"id":"T107","span":{"begin":995,"end":1086},"obj":"Sentence"},{"id":"T108","span":{"begin":1087,"end":1181},"obj":"Sentence"},{"id":"T109","span":{"begin":1182,"end":1378},"obj":"Sentence"},{"id":"T110","span":{"begin":1379,"end":1491},"obj":"Sentence"},{"id":"T111","span":{"begin":1492,"end":1628},"obj":"Sentence"},{"id":"T112","span":{"begin":1629,"end":1879},"obj":"Sentence"},{"id":"T113","span":{"begin":1880,"end":2168},"obj":"Sentence"},{"id":"T114","span":{"begin":2169,"end":2242},"obj":"Sentence"},{"id":"T115","span":{"begin":2243,"end":2365},"obj":"Sentence"},{"id":"T116","span":{"begin":2366,"end":2598},"obj":"Sentence"},{"id":"T117","span":{"begin":2599,"end":2711},"obj":"Sentence"},{"id":"T118","span":{"begin":2712,"end":2721},"obj":"Sentence"},{"id":"T119","span":{"begin":2722,"end":2861},"obj":"Sentence"},{"id":"T120","span":{"begin":2862,"end":2981},"obj":"Sentence"},{"id":"T121","span":{"begin":2982,"end":3075},"obj":"Sentence"},{"id":"T122","span":{"begin":3076,"end":3235},"obj":"Sentence"},{"id":"T123","span":{"begin":3236,"end":3413},"obj":"Sentence"},{"id":"T124","span":{"begin":3414,"end":3727},"obj":"Sentence"},{"id":"T125","span":{"begin":3728,"end":3940},"obj":"Sentence"},{"id":"T126","span":{"begin":3941,"end":4030},"obj":"Sentence"},{"id":"T127","span":{"begin":4031,"end":4208},"obj":"Sentence"},{"id":"T128","span":{"begin":4209,"end":4293},"obj":"Sentence"},{"id":"T129","span":{"begin":4294,"end":4553},"obj":"Sentence"},{"id":"T130","span":{"begin":4554,"end":4689},"obj":"Sentence"},{"id":"T131","span":{"begin":4690,"end":4974},"obj":"Sentence"},{"id":"T132","span":{"begin":4975,"end":5046},"obj":"Sentence"},{"id":"T133","span":{"begin":5047,"end":5268},"obj":"Sentence"},{"id":"T134","span":{"begin":5269,"end":5407},"obj":"Sentence"},{"id":"T135","span":{"begin":5408,"end":5544},"obj":"Sentence"},{"id":"T136","span":{"begin":5545,"end":5779},"obj":"Sentence"},{"id":"T137","span":{"begin":5780,"end":5982},"obj":"Sentence"},{"id":"T138","span":{"begin":5983,"end":6167},"obj":"Sentence"},{"id":"T139","span":{"begin":6168,"end":6408},"obj":"Sentence"},{"id":"T140","span":{"begin":6409,"end":6800},"obj":"Sentence"},{"id":"T141","span":{"begin":6801,"end":7049},"obj":"Sentence"},{"id":"T142","span":{"begin":7050,"end":7297},"obj":"Sentence"},{"id":"T143","span":{"begin":7298,"end":7568},"obj":"Sentence"},{"id":"T144","span":{"begin":7569,"end":7764},"obj":"Sentence"},{"id":"T145","span":{"begin":7765,"end":8028},"obj":"Sentence"},{"id":"T146","span":{"begin":8029,"end":8335},"obj":"Sentence"},{"id":"T147","span":{"begin":8336,"end":8448},"obj":"Sentence"},{"id":"T148","span":{"begin":8449,"end":8643},"obj":"Sentence"},{"id":"T149","span":{"begin":8644,"end":8798},"obj":"Sentence"},{"id":"T150","span":{"begin":8799,"end":9133},"obj":"Sentence"},{"id":"T151","span":{"begin":9134,"end":9286},"obj":"Sentence"},{"id":"T152","span":{"begin":9287,"end":9452},"obj":"Sentence"}],"namespaces":[{"prefix":"_base","uri":"http://pubannotation.org/ontology/tao.owl#"}],"text":"Discussion\nIn our previous article (Tang et al., 2020), we had estimated a reproduction number of 6.47 (95% CI 5.71–7.23), which represents a higher value than those so far computed. For instance, the WHO has estimated a reproduction number of 1.4–2.5, Li and colleagues (Li et al., 2020) have computed a slightly higher value of 2.2 (95% CI 1.4 to 3.9), while in several other mathematical models which have been so far devised and released as pre-prints or undergone peer-review and published, the reproduction number varies from 1.3 to 4.7.\nWithin the existing literature, two studies estimated under-estimation of coronavirus cases: the investigations by Zhao and collaborators (Zhao et al., 2020) and by Read et al. (Read, Bridgen, Cummings, Ho, \u0026 Jewell, 2020). Specifically, Zhao and coworkers (Zhao et al., 2020) have assessed from a quantitative standpoint the under-reporting rate of coronavirus cases, modeling the epidemic growth curve using the exponential growing Poisson process. Authors computed the number of under-reported coronavirus cases to be 469 (95% CI 403–540). Based on this estimate, the basic reproduction number was found to be 2.56 (95% CI 2.49–2.63). In a previous version of the investigation, released in the bioRxiv pre-print server, the reproduction number was computed to oscillate between 3.30 (95% CI 2.73–3.96) and 5.47 (95% CI 4.16–7.10). The basic reproduction number was also found to be associated with 0-fold–2-fold increase in the reporting rate. More in detail, with report rates increasing over the time, the mean value was statistically likely to be higher than 3 but less than 5. Read and coauthors (Read et al., 2020) used a deterministic SEIR model, assuming coronavirus cases being Poisson distributed and with parameter inference being achieved by maximum likelihood estimation utilizing the Nelder-Mead optimization approach. According to the dynamics transmission model, an ascertainment rate of 5.0% (95% CI 3.6–7.4) was computed and, based on this, authors estimated that as of January 22nd, 2020 in Wuhan there were 14,464 (95% CI 6510–25095) infected individuals, and 21,022 (95% CI 11,090–33,490) infections. The basic reproduction number was computed to be 3.11 (95% CI 2.39–4.13). In terms of public health implications, in order to stop the outbreak, at least 58–76% of transmissions should be blocked.\nThere are three further models incorporating data from international travels: the models of Imai and coauthors (Imai et al., 2020), of Kucharski et al. (Kucharski et al., 2020) and of Wu and collaborators (Wu, Leung, \u0026 Leung, 2020). In particular, Imai and coworkers (Imai et al., 2020) estimated a reproduction number of 2.6 (uncertainty range: 1.5–3.5). Depending on the different scenarios and levels of zoonotic exposure, the reproduction number was found to vary from 1.7 to 2.6 to 1.9–4.2. Depending on the different estimates of generation time, the reproduction number oscillated from 1.3 to 2.7 to 1.7–4.3. Based on the level of infectiousness, the reproduction number varied in the range of 1.6–2.9. Finally, assuming that the novel virus would cause more mild-to-moderate cases than the SARS virus, the reproduction number would be 2.0 (uncertainty 1.4–2.3). Moreover, authors found that only public health interventions blocking over 60% of transmission would be really effective in controlling and containing the coronavirus outbreak. Partially based on the findings of Imai and coworkers (Imai et al., 2020) and building on a SIR model, Yu (Yu, 2020) has computed a basic reproduction number of 3.5 and has estimated that only a quarantine rate of infectious population higher than 90% would enable to effectively control the coronavirus outbreak. Kucharski and colleagues (Kucharski et al., 2020) designed a stochastic SEIR model, based on the Euler-Maruyama algorithm with a 6-h time-step and with the transmission rate following a geometric Brownian motion. Time-varying reproduction number was estimated using the sequential Monte-Carlo approach. Authors utilized several datasets to overcome the issue of unreliability of some data sources and to provide real-time estimates, relying on the Poisson probability calculation. Transmission was modeled as a random process, fluctuating and varying over the time. Similar to the model of Imai and coworkers (Imai et al., 2020), the risk of transmission and the risk of causing a large outbreak were modeled based on a negative binomial offspring distribution, with incubation and infectious period being Erlang distributed. Median reproduction number was found to oscillate between 1.6 and 2.9 before the introduction and implementation of travel restriction. The study by Wu and collaborators (Wu et al., 2020), based on nowcasting and forecasting approach, estimated a basic reproductive number of 2.68 (95% credible interval or CrI 2.47–2.86) with 75,815 individuals (95% CrI 37,304–130,330) being infected in Wuhan as of January 25th, 2020. The epidemics doubling time was found to be 6.4 days (95% CrI 5.8–7.1). The dynamics transmission model by Shen and coworkers (Shen, Peng, Xiao, \u0026 Zhang, 2020) predicted 8042 (95% CI 4199–11,884) infections and 898 (95% CI 368–1429) deaths, with a fatality rate of 11.02% (95% CI 9.26–12.78%). Authors computed a basic reproduction number of 4.71 (95% CI 4.50–4.92), which decreased to 2.08 (95% CI 1.99–2.18) on January 22nd, 2020. Based on these estimates, the pandemics outbreak is expected to significantly decrease within 77 [95% CI 75–80] days from its beginning. Furthermore, authors found that every one-day reduction in the duration of the period from illness/symptom onset to isolation would reduce the peak population size by 72–84% and the cumulative infected cases and deaths both by 68–80%. The study by Majumder and Mandl (Majumder \u0026 Mandl, 2020) utilized the “Incidence Decay and Exponential Adjustment” (IDEA) model and led to an estimate of the reproduction number in the range of 2.0–3.1. Finally, Riou and Althaus (Riou \u0026 Althaus, 2020), using a stochastic model simulating epidemics trajectories, computed a reproduction number of 2.2 (90% high density interval 1.4–3.8). Using statistical approaches, namely exponential growth and maximum likelihood techniques, Liu and colleagues (Liu et al., 2020) estimated the value of the reproduction number ranging from 2.90 (95% CI 2.32–3.63) to 2.92 (95% CI 2.28–3.67). Zhang and Wang (Zhang \u0026 Wang, 2020), employing a Bayesian framework to infer time-calibrated phylogeny from 33 available genomic sequences, found that the time of the most recent common ancestor (MRCA) was December 17th, 2019 (95% highest posterior density interval from December 7th, 2019 to December 23rd, 2019) and that the value of the reproduction number oscillated between 1.1 and 1.6.\nThese different findings may be due to several methodological issues, including different assumptions and choice of parameters, utilized models (stochastic versus deterministic, compartmental versus IDEA, etc.), used datasets and estimation period. Furthermore, by comparing the various updated versions of the above-mentioned investigations, the reproduction number was found to vary, reflecting the dynamics of transmission of the coronavirus outbreak as well as the dynamics of case reporting. More in detail, the reproduction number tended to increase over the time in parallel with the increase in cases being reported and the findings were highly sensitive and dependent on the period in which the estimate was made and on the data available at that given time. It should be mentioned that much of the model frameworks and data fitting and analysis have been developed from earlier studies about the SARS outbreak (Chowell et al., 2004; Gumel et al., 2004). It was believed that the SARS outbreak was characterized by a large basic reproduction number within hospitals (nosocomial infection) and a relatively small basic reproduction number in the general community, leading to a moderate basic production number overall. We argue that the current 2019 n-Cov situation is similar to what was observed about the SARS, except that the entire city of Wuhan is the epicenter with a population of over 11 million inhabitants, and the community is the entire country due to the travels done before the shutdown of the epidemic center.\nThe outbreak situation is fast evolving both in the epicenter of Wuhan, the Hubei province and throughout China. Our simulations show that the control outcome depends highly on the interventions implemented in the field, which depend on the resources provided to the frontline workers and patients infected. The size of peak value and peak time depends on a number of factors including in particular the speed of diagnoses and hospitalization of confirmed cases.\nThere are essential differences between Wuhan, Hubei, and the rest of the country as we discussed above, therefore, a more realistic model should involve the Wuhan-Hubei-China coupled system with different initial data, varying resources, and changing mobility patterns from the epicenter to the province, and the rest of the country. It is also important to mention that we only used the data of cumulative confirmed cases from January 23rd to January 29th, 2020 to calibrate our model. Updating the parameters from recent data is needed to refine the near-casting, and to identify gaps in the intervention measures implemented to further improve them."}
LitCovid-PD-HP
{"project":"LitCovid-PD-HP","denotations":[{"id":"T2","span":{"begin":7447,"end":7463},"obj":"Phenotype"}],"attributes":[{"id":"A2","pred":"hp_id","subj":"T2","obj":"http://purl.obolibrary.org/obo/HP_0041092"}],"text":"Discussion\nIn our previous article (Tang et al., 2020), we had estimated a reproduction number of 6.47 (95% CI 5.71–7.23), which represents a higher value than those so far computed. For instance, the WHO has estimated a reproduction number of 1.4–2.5, Li and colleagues (Li et al., 2020) have computed a slightly higher value of 2.2 (95% CI 1.4 to 3.9), while in several other mathematical models which have been so far devised and released as pre-prints or undergone peer-review and published, the reproduction number varies from 1.3 to 4.7.\nWithin the existing literature, two studies estimated under-estimation of coronavirus cases: the investigations by Zhao and collaborators (Zhao et al., 2020) and by Read et al. (Read, Bridgen, Cummings, Ho, \u0026 Jewell, 2020). Specifically, Zhao and coworkers (Zhao et al., 2020) have assessed from a quantitative standpoint the under-reporting rate of coronavirus cases, modeling the epidemic growth curve using the exponential growing Poisson process. Authors computed the number of under-reported coronavirus cases to be 469 (95% CI 403–540). Based on this estimate, the basic reproduction number was found to be 2.56 (95% CI 2.49–2.63). In a previous version of the investigation, released in the bioRxiv pre-print server, the reproduction number was computed to oscillate between 3.30 (95% CI 2.73–3.96) and 5.47 (95% CI 4.16–7.10). The basic reproduction number was also found to be associated with 0-fold–2-fold increase in the reporting rate. More in detail, with report rates increasing over the time, the mean value was statistically likely to be higher than 3 but less than 5. Read and coauthors (Read et al., 2020) used a deterministic SEIR model, assuming coronavirus cases being Poisson distributed and with parameter inference being achieved by maximum likelihood estimation utilizing the Nelder-Mead optimization approach. According to the dynamics transmission model, an ascertainment rate of 5.0% (95% CI 3.6–7.4) was computed and, based on this, authors estimated that as of January 22nd, 2020 in Wuhan there were 14,464 (95% CI 6510–25095) infected individuals, and 21,022 (95% CI 11,090–33,490) infections. The basic reproduction number was computed to be 3.11 (95% CI 2.39–4.13). In terms of public health implications, in order to stop the outbreak, at least 58–76% of transmissions should be blocked.\nThere are three further models incorporating data from international travels: the models of Imai and coauthors (Imai et al., 2020), of Kucharski et al. (Kucharski et al., 2020) and of Wu and collaborators (Wu, Leung, \u0026 Leung, 2020). In particular, Imai and coworkers (Imai et al., 2020) estimated a reproduction number of 2.6 (uncertainty range: 1.5–3.5). Depending on the different scenarios and levels of zoonotic exposure, the reproduction number was found to vary from 1.7 to 2.6 to 1.9–4.2. Depending on the different estimates of generation time, the reproduction number oscillated from 1.3 to 2.7 to 1.7–4.3. Based on the level of infectiousness, the reproduction number varied in the range of 1.6–2.9. Finally, assuming that the novel virus would cause more mild-to-moderate cases than the SARS virus, the reproduction number would be 2.0 (uncertainty 1.4–2.3). Moreover, authors found that only public health interventions blocking over 60% of transmission would be really effective in controlling and containing the coronavirus outbreak. Partially based on the findings of Imai and coworkers (Imai et al., 2020) and building on a SIR model, Yu (Yu, 2020) has computed a basic reproduction number of 3.5 and has estimated that only a quarantine rate of infectious population higher than 90% would enable to effectively control the coronavirus outbreak. Kucharski and colleagues (Kucharski et al., 2020) designed a stochastic SEIR model, based on the Euler-Maruyama algorithm with a 6-h time-step and with the transmission rate following a geometric Brownian motion. Time-varying reproduction number was estimated using the sequential Monte-Carlo approach. Authors utilized several datasets to overcome the issue of unreliability of some data sources and to provide real-time estimates, relying on the Poisson probability calculation. Transmission was modeled as a random process, fluctuating and varying over the time. Similar to the model of Imai and coworkers (Imai et al., 2020), the risk of transmission and the risk of causing a large outbreak were modeled based on a negative binomial offspring distribution, with incubation and infectious period being Erlang distributed. Median reproduction number was found to oscillate between 1.6 and 2.9 before the introduction and implementation of travel restriction. The study by Wu and collaborators (Wu et al., 2020), based on nowcasting and forecasting approach, estimated a basic reproductive number of 2.68 (95% credible interval or CrI 2.47–2.86) with 75,815 individuals (95% CrI 37,304–130,330) being infected in Wuhan as of January 25th, 2020. The epidemics doubling time was found to be 6.4 days (95% CrI 5.8–7.1). The dynamics transmission model by Shen and coworkers (Shen, Peng, Xiao, \u0026 Zhang, 2020) predicted 8042 (95% CI 4199–11,884) infections and 898 (95% CI 368–1429) deaths, with a fatality rate of 11.02% (95% CI 9.26–12.78%). Authors computed a basic reproduction number of 4.71 (95% CI 4.50–4.92), which decreased to 2.08 (95% CI 1.99–2.18) on January 22nd, 2020. Based on these estimates, the pandemics outbreak is expected to significantly decrease within 77 [95% CI 75–80] days from its beginning. Furthermore, authors found that every one-day reduction in the duration of the period from illness/symptom onset to isolation would reduce the peak population size by 72–84% and the cumulative infected cases and deaths both by 68–80%. The study by Majumder and Mandl (Majumder \u0026 Mandl, 2020) utilized the “Incidence Decay and Exponential Adjustment” (IDEA) model and led to an estimate of the reproduction number in the range of 2.0–3.1. Finally, Riou and Althaus (Riou \u0026 Althaus, 2020), using a stochastic model simulating epidemics trajectories, computed a reproduction number of 2.2 (90% high density interval 1.4–3.8). Using statistical approaches, namely exponential growth and maximum likelihood techniques, Liu and colleagues (Liu et al., 2020) estimated the value of the reproduction number ranging from 2.90 (95% CI 2.32–3.63) to 2.92 (95% CI 2.28–3.67). Zhang and Wang (Zhang \u0026 Wang, 2020), employing a Bayesian framework to infer time-calibrated phylogeny from 33 available genomic sequences, found that the time of the most recent common ancestor (MRCA) was December 17th, 2019 (95% highest posterior density interval from December 7th, 2019 to December 23rd, 2019) and that the value of the reproduction number oscillated between 1.1 and 1.6.\nThese different findings may be due to several methodological issues, including different assumptions and choice of parameters, utilized models (stochastic versus deterministic, compartmental versus IDEA, etc.), used datasets and estimation period. Furthermore, by comparing the various updated versions of the above-mentioned investigations, the reproduction number was found to vary, reflecting the dynamics of transmission of the coronavirus outbreak as well as the dynamics of case reporting. More in detail, the reproduction number tended to increase over the time in parallel with the increase in cases being reported and the findings were highly sensitive and dependent on the period in which the estimate was made and on the data available at that given time. It should be mentioned that much of the model frameworks and data fitting and analysis have been developed from earlier studies about the SARS outbreak (Chowell et al., 2004; Gumel et al., 2004). It was believed that the SARS outbreak was characterized by a large basic reproduction number within hospitals (nosocomial infection) and a relatively small basic reproduction number in the general community, leading to a moderate basic production number overall. We argue that the current 2019 n-Cov situation is similar to what was observed about the SARS, except that the entire city of Wuhan is the epicenter with a population of over 11 million inhabitants, and the community is the entire country due to the travels done before the shutdown of the epidemic center.\nThe outbreak situation is fast evolving both in the epicenter of Wuhan, the Hubei province and throughout China. Our simulations show that the control outcome depends highly on the interventions implemented in the field, which depend on the resources provided to the frontline workers and patients infected. The size of peak value and peak time depends on a number of factors including in particular the speed of diagnoses and hospitalization of confirmed cases.\nThere are essential differences between Wuhan, Hubei, and the rest of the country as we discussed above, therefore, a more realistic model should involve the Wuhan-Hubei-China coupled system with different initial data, varying resources, and changing mobility patterns from the epicenter to the province, and the rest of the country. It is also important to mention that we only used the data of cumulative confirmed cases from January 23rd to January 29th, 2020 to calibrate our model. Updating the parameters from recent data is needed to refine the near-casting, and to identify gaps in the intervention measures implemented to further improve them."}
LitCovid-PubTator
{"project":"LitCovid-PubTator","denotations":[{"id":"130","span":{"begin":618,"end":629},"obj":"Species"},{"id":"131","span":{"begin":894,"end":905},"obj":"Species"},{"id":"132","span":{"begin":1041,"end":1052},"obj":"Species"},{"id":"133","span":{"begin":1710,"end":1721},"obj":"Species"},{"id":"134","span":{"begin":2101,"end":2109},"obj":"Disease"},{"id":"135","span":{"begin":2157,"end":2167},"obj":"Disease"},{"id":"145","span":{"begin":3164,"end":3174},"obj":"Species"},{"id":"146","span":{"begin":3392,"end":3403},"obj":"Species"},{"id":"147","span":{"begin":3706,"end":3717},"obj":"Species"},{"id":"148","span":{"begin":2773,"end":2781},"obj":"Disease"},{"id":"149","span":{"begin":4931,"end":4939},"obj":"Disease"},{"id":"150","span":{"begin":5171,"end":5181},"obj":"Disease"},{"id":"151","span":{"begin":5208,"end":5214},"obj":"Disease"},{"id":"152","span":{"begin":5738,"end":5746},"obj":"Disease"},{"id":"153","span":{"begin":5757,"end":5763},"obj":"Disease"},{"id":"160","span":{"begin":7234,"end":7245},"obj":"Species"},{"id":"161","span":{"begin":8055,"end":8065},"obj":"Species"},{"id":"162","span":{"begin":7707,"end":7711},"obj":"Disease"},{"id":"163","span":{"begin":7790,"end":7794},"obj":"Disease"},{"id":"164","span":{"begin":7877,"end":7897},"obj":"Disease"},{"id":"165","span":{"begin":8118,"end":8122},"obj":"Disease"},{"id":"168","span":{"begin":8625,"end":8633},"obj":"Species"},{"id":"169","span":{"begin":8634,"end":8642},"obj":"Disease"}],"attributes":[{"id":"A130","pred":"tao:has_database_id","subj":"130","obj":"Tax:11118"},{"id":"A131","pred":"tao:has_database_id","subj":"131","obj":"Tax:11118"},{"id":"A132","pred":"tao:has_database_id","subj":"132","obj":"Tax:11118"},{"id":"A133","pred":"tao:has_database_id","subj":"133","obj":"Tax:11118"},{"id":"A134","pred":"tao:has_database_id","subj":"134","obj":"MESH:D007239"},{"id":"A135","pred":"tao:has_database_id","subj":"135","obj":"MESH:D007239"},{"id":"A145","pred":"tao:has_database_id","subj":"145","obj":"Tax:694009"},{"id":"A146","pred":"tao:has_database_id","subj":"146","obj":"Tax:11118"},{"id":"A147","pred":"tao:has_database_id","subj":"147","obj":"Tax:11118"},{"id":"A148","pred":"tao:has_database_id","subj":"148","obj":"MESH:D015047"},{"id":"A149","pred":"tao:has_database_id","subj":"149","obj":"MESH:D007239"},{"id":"A150","pred":"tao:has_database_id","subj":"150","obj":"MESH:D007239"},{"id":"A151","pred":"tao:has_database_id","subj":"151","obj":"MESH:D003643"},{"id":"A152","pred":"tao:has_database_id","subj":"152","obj":"MESH:D007239"},{"id":"A153","pred":"tao:has_database_id","subj":"153","obj":"MESH:D003643"},{"id":"A160","pred":"tao:has_database_id","subj":"160","obj":"Tax:11118"},{"id":"A161","pred":"tao:has_database_id","subj":"161","obj":"Tax:2697049"},{"id":"A162","pred":"tao:has_database_id","subj":"162","obj":"MESH:D045169"},{"id":"A163","pred":"tao:has_database_id","subj":"163","obj":"MESH:D045169"},{"id":"A164","pred":"tao:has_database_id","subj":"164","obj":"MESH:D003428"},{"id":"A165","pred":"tao:has_database_id","subj":"165","obj":"MESH:D045169"},{"id":"A168","pred":"tao:has_database_id","subj":"168","obj":"Tax:9606"},{"id":"A169","pred":"tao:has_database_id","subj":"169","obj":"MESH:D007239"}],"namespaces":[{"prefix":"Tax","uri":"https://www.ncbi.nlm.nih.gov/taxonomy/"},{"prefix":"MESH","uri":"https://id.nlm.nih.gov/mesh/"},{"prefix":"Gene","uri":"https://www.ncbi.nlm.nih.gov/gene/"},{"prefix":"CVCL","uri":"https://web.expasy.org/cellosaurus/CVCL_"}],"text":"Discussion\nIn our previous article (Tang et al., 2020), we had estimated a reproduction number of 6.47 (95% CI 5.71–7.23), which represents a higher value than those so far computed. For instance, the WHO has estimated a reproduction number of 1.4–2.5, Li and colleagues (Li et al., 2020) have computed a slightly higher value of 2.2 (95% CI 1.4 to 3.9), while in several other mathematical models which have been so far devised and released as pre-prints or undergone peer-review and published, the reproduction number varies from 1.3 to 4.7.\nWithin the existing literature, two studies estimated under-estimation of coronavirus cases: the investigations by Zhao and collaborators (Zhao et al., 2020) and by Read et al. (Read, Bridgen, Cummings, Ho, \u0026 Jewell, 2020). Specifically, Zhao and coworkers (Zhao et al., 2020) have assessed from a quantitative standpoint the under-reporting rate of coronavirus cases, modeling the epidemic growth curve using the exponential growing Poisson process. Authors computed the number of under-reported coronavirus cases to be 469 (95% CI 403–540). Based on this estimate, the basic reproduction number was found to be 2.56 (95% CI 2.49–2.63). In a previous version of the investigation, released in the bioRxiv pre-print server, the reproduction number was computed to oscillate between 3.30 (95% CI 2.73–3.96) and 5.47 (95% CI 4.16–7.10). The basic reproduction number was also found to be associated with 0-fold–2-fold increase in the reporting rate. More in detail, with report rates increasing over the time, the mean value was statistically likely to be higher than 3 but less than 5. Read and coauthors (Read et al., 2020) used a deterministic SEIR model, assuming coronavirus cases being Poisson distributed and with parameter inference being achieved by maximum likelihood estimation utilizing the Nelder-Mead optimization approach. According to the dynamics transmission model, an ascertainment rate of 5.0% (95% CI 3.6–7.4) was computed and, based on this, authors estimated that as of January 22nd, 2020 in Wuhan there were 14,464 (95% CI 6510–25095) infected individuals, and 21,022 (95% CI 11,090–33,490) infections. The basic reproduction number was computed to be 3.11 (95% CI 2.39–4.13). In terms of public health implications, in order to stop the outbreak, at least 58–76% of transmissions should be blocked.\nThere are three further models incorporating data from international travels: the models of Imai and coauthors (Imai et al., 2020), of Kucharski et al. (Kucharski et al., 2020) and of Wu and collaborators (Wu, Leung, \u0026 Leung, 2020). In particular, Imai and coworkers (Imai et al., 2020) estimated a reproduction number of 2.6 (uncertainty range: 1.5–3.5). Depending on the different scenarios and levels of zoonotic exposure, the reproduction number was found to vary from 1.7 to 2.6 to 1.9–4.2. Depending on the different estimates of generation time, the reproduction number oscillated from 1.3 to 2.7 to 1.7–4.3. Based on the level of infectiousness, the reproduction number varied in the range of 1.6–2.9. Finally, assuming that the novel virus would cause more mild-to-moderate cases than the SARS virus, the reproduction number would be 2.0 (uncertainty 1.4–2.3). Moreover, authors found that only public health interventions blocking over 60% of transmission would be really effective in controlling and containing the coronavirus outbreak. Partially based on the findings of Imai and coworkers (Imai et al., 2020) and building on a SIR model, Yu (Yu, 2020) has computed a basic reproduction number of 3.5 and has estimated that only a quarantine rate of infectious population higher than 90% would enable to effectively control the coronavirus outbreak. Kucharski and colleagues (Kucharski et al., 2020) designed a stochastic SEIR model, based on the Euler-Maruyama algorithm with a 6-h time-step and with the transmission rate following a geometric Brownian motion. Time-varying reproduction number was estimated using the sequential Monte-Carlo approach. Authors utilized several datasets to overcome the issue of unreliability of some data sources and to provide real-time estimates, relying on the Poisson probability calculation. Transmission was modeled as a random process, fluctuating and varying over the time. Similar to the model of Imai and coworkers (Imai et al., 2020), the risk of transmission and the risk of causing a large outbreak were modeled based on a negative binomial offspring distribution, with incubation and infectious period being Erlang distributed. Median reproduction number was found to oscillate between 1.6 and 2.9 before the introduction and implementation of travel restriction. The study by Wu and collaborators (Wu et al., 2020), based on nowcasting and forecasting approach, estimated a basic reproductive number of 2.68 (95% credible interval or CrI 2.47–2.86) with 75,815 individuals (95% CrI 37,304–130,330) being infected in Wuhan as of January 25th, 2020. The epidemics doubling time was found to be 6.4 days (95% CrI 5.8–7.1). The dynamics transmission model by Shen and coworkers (Shen, Peng, Xiao, \u0026 Zhang, 2020) predicted 8042 (95% CI 4199–11,884) infections and 898 (95% CI 368–1429) deaths, with a fatality rate of 11.02% (95% CI 9.26–12.78%). Authors computed a basic reproduction number of 4.71 (95% CI 4.50–4.92), which decreased to 2.08 (95% CI 1.99–2.18) on January 22nd, 2020. Based on these estimates, the pandemics outbreak is expected to significantly decrease within 77 [95% CI 75–80] days from its beginning. Furthermore, authors found that every one-day reduction in the duration of the period from illness/symptom onset to isolation would reduce the peak population size by 72–84% and the cumulative infected cases and deaths both by 68–80%. The study by Majumder and Mandl (Majumder \u0026 Mandl, 2020) utilized the “Incidence Decay and Exponential Adjustment” (IDEA) model and led to an estimate of the reproduction number in the range of 2.0–3.1. Finally, Riou and Althaus (Riou \u0026 Althaus, 2020), using a stochastic model simulating epidemics trajectories, computed a reproduction number of 2.2 (90% high density interval 1.4–3.8). Using statistical approaches, namely exponential growth and maximum likelihood techniques, Liu and colleagues (Liu et al., 2020) estimated the value of the reproduction number ranging from 2.90 (95% CI 2.32–3.63) to 2.92 (95% CI 2.28–3.67). Zhang and Wang (Zhang \u0026 Wang, 2020), employing a Bayesian framework to infer time-calibrated phylogeny from 33 available genomic sequences, found that the time of the most recent common ancestor (MRCA) was December 17th, 2019 (95% highest posterior density interval from December 7th, 2019 to December 23rd, 2019) and that the value of the reproduction number oscillated between 1.1 and 1.6.\nThese different findings may be due to several methodological issues, including different assumptions and choice of parameters, utilized models (stochastic versus deterministic, compartmental versus IDEA, etc.), used datasets and estimation period. Furthermore, by comparing the various updated versions of the above-mentioned investigations, the reproduction number was found to vary, reflecting the dynamics of transmission of the coronavirus outbreak as well as the dynamics of case reporting. More in detail, the reproduction number tended to increase over the time in parallel with the increase in cases being reported and the findings were highly sensitive and dependent on the period in which the estimate was made and on the data available at that given time. It should be mentioned that much of the model frameworks and data fitting and analysis have been developed from earlier studies about the SARS outbreak (Chowell et al., 2004; Gumel et al., 2004). It was believed that the SARS outbreak was characterized by a large basic reproduction number within hospitals (nosocomial infection) and a relatively small basic reproduction number in the general community, leading to a moderate basic production number overall. We argue that the current 2019 n-Cov situation is similar to what was observed about the SARS, except that the entire city of Wuhan is the epicenter with a population of over 11 million inhabitants, and the community is the entire country due to the travels done before the shutdown of the epidemic center.\nThe outbreak situation is fast evolving both in the epicenter of Wuhan, the Hubei province and throughout China. Our simulations show that the control outcome depends highly on the interventions implemented in the field, which depend on the resources provided to the frontline workers and patients infected. The size of peak value and peak time depends on a number of factors including in particular the speed of diagnoses and hospitalization of confirmed cases.\nThere are essential differences between Wuhan, Hubei, and the rest of the country as we discussed above, therefore, a more realistic model should involve the Wuhan-Hubei-China coupled system with different initial data, varying resources, and changing mobility patterns from the epicenter to the province, and the rest of the country. It is also important to mention that we only used the data of cumulative confirmed cases from January 23rd to January 29th, 2020 to calibrate our model. Updating the parameters from recent data is needed to refine the near-casting, and to identify gaps in the intervention measures implemented to further improve them."}
2_test
{"project":"2_test","denotations":[{"id":"32099934-15324546-47437414","span":{"begin":7738,"end":7742},"obj":"15324546"},{"id":"T72314","span":{"begin":7738,"end":7742},"obj":"15324546"}],"text":"Discussion\nIn our previous article (Tang et al., 2020), we had estimated a reproduction number of 6.47 (95% CI 5.71–7.23), which represents a higher value than those so far computed. For instance, the WHO has estimated a reproduction number of 1.4–2.5, Li and colleagues (Li et al., 2020) have computed a slightly higher value of 2.2 (95% CI 1.4 to 3.9), while in several other mathematical models which have been so far devised and released as pre-prints or undergone peer-review and published, the reproduction number varies from 1.3 to 4.7.\nWithin the existing literature, two studies estimated under-estimation of coronavirus cases: the investigations by Zhao and collaborators (Zhao et al., 2020) and by Read et al. (Read, Bridgen, Cummings, Ho, \u0026 Jewell, 2020). Specifically, Zhao and coworkers (Zhao et al., 2020) have assessed from a quantitative standpoint the under-reporting rate of coronavirus cases, modeling the epidemic growth curve using the exponential growing Poisson process. Authors computed the number of under-reported coronavirus cases to be 469 (95% CI 403–540). Based on this estimate, the basic reproduction number was found to be 2.56 (95% CI 2.49–2.63). In a previous version of the investigation, released in the bioRxiv pre-print server, the reproduction number was computed to oscillate between 3.30 (95% CI 2.73–3.96) and 5.47 (95% CI 4.16–7.10). The basic reproduction number was also found to be associated with 0-fold–2-fold increase in the reporting rate. More in detail, with report rates increasing over the time, the mean value was statistically likely to be higher than 3 but less than 5. Read and coauthors (Read et al., 2020) used a deterministic SEIR model, assuming coronavirus cases being Poisson distributed and with parameter inference being achieved by maximum likelihood estimation utilizing the Nelder-Mead optimization approach. According to the dynamics transmission model, an ascertainment rate of 5.0% (95% CI 3.6–7.4) was computed and, based on this, authors estimated that as of January 22nd, 2020 in Wuhan there were 14,464 (95% CI 6510–25095) infected individuals, and 21,022 (95% CI 11,090–33,490) infections. The basic reproduction number was computed to be 3.11 (95% CI 2.39–4.13). In terms of public health implications, in order to stop the outbreak, at least 58–76% of transmissions should be blocked.\nThere are three further models incorporating data from international travels: the models of Imai and coauthors (Imai et al., 2020), of Kucharski et al. (Kucharski et al., 2020) and of Wu and collaborators (Wu, Leung, \u0026 Leung, 2020). In particular, Imai and coworkers (Imai et al., 2020) estimated a reproduction number of 2.6 (uncertainty range: 1.5–3.5). Depending on the different scenarios and levels of zoonotic exposure, the reproduction number was found to vary from 1.7 to 2.6 to 1.9–4.2. Depending on the different estimates of generation time, the reproduction number oscillated from 1.3 to 2.7 to 1.7–4.3. Based on the level of infectiousness, the reproduction number varied in the range of 1.6–2.9. Finally, assuming that the novel virus would cause more mild-to-moderate cases than the SARS virus, the reproduction number would be 2.0 (uncertainty 1.4–2.3). Moreover, authors found that only public health interventions blocking over 60% of transmission would be really effective in controlling and containing the coronavirus outbreak. Partially based on the findings of Imai and coworkers (Imai et al., 2020) and building on a SIR model, Yu (Yu, 2020) has computed a basic reproduction number of 3.5 and has estimated that only a quarantine rate of infectious population higher than 90% would enable to effectively control the coronavirus outbreak. Kucharski and colleagues (Kucharski et al., 2020) designed a stochastic SEIR model, based on the Euler-Maruyama algorithm with a 6-h time-step and with the transmission rate following a geometric Brownian motion. Time-varying reproduction number was estimated using the sequential Monte-Carlo approach. Authors utilized several datasets to overcome the issue of unreliability of some data sources and to provide real-time estimates, relying on the Poisson probability calculation. Transmission was modeled as a random process, fluctuating and varying over the time. Similar to the model of Imai and coworkers (Imai et al., 2020), the risk of transmission and the risk of causing a large outbreak were modeled based on a negative binomial offspring distribution, with incubation and infectious period being Erlang distributed. Median reproduction number was found to oscillate between 1.6 and 2.9 before the introduction and implementation of travel restriction. The study by Wu and collaborators (Wu et al., 2020), based on nowcasting and forecasting approach, estimated a basic reproductive number of 2.68 (95% credible interval or CrI 2.47–2.86) with 75,815 individuals (95% CrI 37,304–130,330) being infected in Wuhan as of January 25th, 2020. The epidemics doubling time was found to be 6.4 days (95% CrI 5.8–7.1). The dynamics transmission model by Shen and coworkers (Shen, Peng, Xiao, \u0026 Zhang, 2020) predicted 8042 (95% CI 4199–11,884) infections and 898 (95% CI 368–1429) deaths, with a fatality rate of 11.02% (95% CI 9.26–12.78%). Authors computed a basic reproduction number of 4.71 (95% CI 4.50–4.92), which decreased to 2.08 (95% CI 1.99–2.18) on January 22nd, 2020. Based on these estimates, the pandemics outbreak is expected to significantly decrease within 77 [95% CI 75–80] days from its beginning. Furthermore, authors found that every one-day reduction in the duration of the period from illness/symptom onset to isolation would reduce the peak population size by 72–84% and the cumulative infected cases and deaths both by 68–80%. The study by Majumder and Mandl (Majumder \u0026 Mandl, 2020) utilized the “Incidence Decay and Exponential Adjustment” (IDEA) model and led to an estimate of the reproduction number in the range of 2.0–3.1. Finally, Riou and Althaus (Riou \u0026 Althaus, 2020), using a stochastic model simulating epidemics trajectories, computed a reproduction number of 2.2 (90% high density interval 1.4–3.8). Using statistical approaches, namely exponential growth and maximum likelihood techniques, Liu and colleagues (Liu et al., 2020) estimated the value of the reproduction number ranging from 2.90 (95% CI 2.32–3.63) to 2.92 (95% CI 2.28–3.67). Zhang and Wang (Zhang \u0026 Wang, 2020), employing a Bayesian framework to infer time-calibrated phylogeny from 33 available genomic sequences, found that the time of the most recent common ancestor (MRCA) was December 17th, 2019 (95% highest posterior density interval from December 7th, 2019 to December 23rd, 2019) and that the value of the reproduction number oscillated between 1.1 and 1.6.\nThese different findings may be due to several methodological issues, including different assumptions and choice of parameters, utilized models (stochastic versus deterministic, compartmental versus IDEA, etc.), used datasets and estimation period. Furthermore, by comparing the various updated versions of the above-mentioned investigations, the reproduction number was found to vary, reflecting the dynamics of transmission of the coronavirus outbreak as well as the dynamics of case reporting. More in detail, the reproduction number tended to increase over the time in parallel with the increase in cases being reported and the findings were highly sensitive and dependent on the period in which the estimate was made and on the data available at that given time. It should be mentioned that much of the model frameworks and data fitting and analysis have been developed from earlier studies about the SARS outbreak (Chowell et al., 2004; Gumel et al., 2004). It was believed that the SARS outbreak was characterized by a large basic reproduction number within hospitals (nosocomial infection) and a relatively small basic reproduction number in the general community, leading to a moderate basic production number overall. We argue that the current 2019 n-Cov situation is similar to what was observed about the SARS, except that the entire city of Wuhan is the epicenter with a population of over 11 million inhabitants, and the community is the entire country due to the travels done before the shutdown of the epidemic center.\nThe outbreak situation is fast evolving both in the epicenter of Wuhan, the Hubei province and throughout China. Our simulations show that the control outcome depends highly on the interventions implemented in the field, which depend on the resources provided to the frontline workers and patients infected. The size of peak value and peak time depends on a number of factors including in particular the speed of diagnoses and hospitalization of confirmed cases.\nThere are essential differences between Wuhan, Hubei, and the rest of the country as we discussed above, therefore, a more realistic model should involve the Wuhan-Hubei-China coupled system with different initial data, varying resources, and changing mobility patterns from the epicenter to the province, and the rest of the country. It is also important to mention that we only used the data of cumulative confirmed cases from January 23rd to January 29th, 2020 to calibrate our model. Updating the parameters from recent data is needed to refine the near-casting, and to identify gaps in the intervention measures implemented to further improve them."}