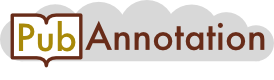
PMC:4996411 / 12540-35515
Annnotations
{"target":"https://pubannotation.org/docs/sourcedb/PMC/sourceid/4996411","sourcedb":"PMC","sourceid":"4996411","source_url":"https://www.ncbi.nlm.nih.gov/pmc/4996411","text":"3. Points to Consider before Sample Printing\n\n3.1. Experimental Design\nSuccessful biomarker discovery requires a careful reflection of all aspects that might play a role during data analysis. Key points comprise the experimental design that was chosen to approach the clinical question at hand. Thus, a definition of quality control (QC) measures and control samples meaningful for data analysis is required to analyze RPPA data. Using the same types of controls is also necessary to compare RPPA data across different RPPA platforms.\nAs RPPA enables a relative quantification of proteins in large sample sets, experimental effects that might take influence on raw data must be taken into account such as the dynamic range of measurements or spatial effects that might result from staining artifacts of the signal detection approach. In addition, sample loading has to match the signal detection range. To compensate experimental noise, for example, normalization approaches were developed which require additionally printed spots, such as sample dilution series or so-called loading control spots to account for uneven staining. All normalization methods require an array design that comprises printing of control samples and technical replicates to gain statistically relevant results. Apart from statistical aspects, the information inherent to replicate spots serves several other functions that become important during data analysis: on the one hand, it presents the basis to apply certain normalization methods, on the other hand, it also facilitates data comparability with already existing RPPA data sets, provided that the same controls were used.\nFor RPPA, two fundamentally different approaches exist. The first one is based on printing each sample as serial dilution, thus simultaneously providing a sufficient number of data points for downstream data analysis. Samples can also be printed in a single concentration which may be the approach of choice when the sample volume is too limited to allow for the preparation of serial dilutions, for example when working with scarce patient material. In this instance, it is highly important to choose a method for signal detection with a low experimental noise since this negatively impacts on data quality. However, co-printing serial dilutions of meaningful controls is also required in case samples are supposed to be printed in a single concentration to calibrate the signals obtained by the actual samples as realized in the second approach for RPPA. In this instance, samples are printed as three technical replicate spots to balance statistical power and to guarantee optimal spatial usage [22,28]. Relative protein quantification approaches, as commonly employed in RPPA, can also benefit from not simply aggregating sample replicates but using the information of individual replicate spots as measure of within-sample variability, as realized by the non-parametric estimation of protein expression levels by Li and coworkers as the Reno-approach [31].\n\n3.2. Protein Quantification\nTwo principally different mathematical approaches are employed to infer numeric values that reflect the expression level of proteins assessed by RPPA: parametric and non-parametric models (Figure 2).\nFigure 2 Parametric and non-parametric approaches for protein quantification in RPPA data sets. While parametric approaches employ pre-defined functions to describe the relationship between measured expression levels and protein concentration, non-parametric approaches are comparably more data-adaptive and use e.g., protein-specific response curves. Such statistical models contain so-called parameters that can either describe certain experimental characteristics, e.g., background noise or saturation level, and are defined before fitting the model to the data or are not defined initially. Thus, parametric models employ certain assumptions regarding the function used to describe the relation between the observed expression levels and the unobserved and yet unknown protein concentration. On the contrary, non-parametric models do not imply such a predefined form of the model, so that they are highly data-adaptive.\nParametric approaches have employed linear models [11,32] or sigmoidal curves [12,28] to determine a response curve, the relationship between the observed signal and the protein concentration. Zhang et al. [23] used the Sips model, which is similar to a logistic model, to determine the relationship between signals in successive dilution steps. Zhang and colleagues argued that the response curve depends on factors such as the target protein concentration and exposure time during signal detection, specific or non-specific interactions of reporter molecules with proteins of a particular sample or of the slide matrix. While the impact of experimental factors might be difficult to quantify and to incorporate into a statistical model, the serial dilution curve method allows the determination of experimentally meaningful model parameters that can be optimized further.\nHowever, non-parametric methods seem to prevail due to their flexibility and their robust results. Non-parametric examples include the model of Hu et al. [16] which was based on the assumption that protein expression equals a non-parametric monotonically increasing function. The regularized approach by Li et al. [31] proposed an estimation of protein levels based on individual non-aggregated dilution series replicates to account for within-sample or within-group variability. Non-parametric parameters are more difficult to interpret but do not impose prior assumptions on experimental factors such as the reaction kinetics of the RPPA signal detection procedure.\n\n3.3. Loading Control Normalization\nLoading controls are required to account for measurable effects caused by properties of the starting material e.g., unequal total protein concentration or different cell numbers. Thus, a normalization step is required to permit the actual data analysis. Different approaches have been described which are based on adjusting the total protein concentration of individual samples to a pre-defined value prior to spotting. Protein quantification assays such as BCA or Bradford are frequently employed to determine the total protein concentration prior to spotting. In general, the accuracy of total protein assays is restricted by chemical inference with certain compounds and limited by a short linear range, not to mention the additional time needed for the experimental protocol. Alternatively, signals can be adjusted post detection. Post-printing normalization with a total protein dye requires additional slides of a print run to be stained with a total protein dye, for example Fast Green FCF, Sypro Ruby or colloidal gold. Antibody-detected slides are normalized based on data of a corresponding normalizer slide via a spot-specific correction factor that reflects the deviation of the protein concentration determined from the median of all spots. Target protein signals are then corrected via division by the correction factors, rescaling can be carried out by multiplication of spot intensities with the median of the corresponding normalizer array. Housekeeping proteins such as β-Actin have been used to normalize RPPA data [33,34]. However, even housekeeping proteins are subjected to biological regulation and have therefore limited these approaches.\nDifferent normalization approaches were specifically tailored towards the needs of RPPA data analysis, e.g., median loading, loading control, variable slope, and invariable protein set normalization, as reviewed in [35]. Median Loading (ML) normalization considers row and sample effects as additive at the log scale. The sample effect is estimated from the median protein expression estimates of the samples across all arrays. The main assumptions of the median loading approach is that all arrays are printed in a consistent manner and that changes observed for up- or downregulated proteins can still be seen after median normalization [36]. A key idea behind this approach is that the majority of target proteins assessed by RPPA will be comparable for the majority of samples. However, if a low number of target proteins are probed by RPPA or only proteins subjected to regulation will be measured, the ML approach will be biased. Loading control incorporates similar ideas, yet the value reflecting median expression is calculated individually for each target protein and then subtracted from a particular sample [35].\nVariable Slope (VS) normalization [17] takes into account the independent nature of individually stained RPPA slides. A slide-specific value is determined and included in the additive sample and row effect model in a multiplicative manner, thus yielding slightly different response curves for different slides. This approach was coupled with the “joint sample” model implemented in the suite of R packages “SuperCurve” (Table 1). These “joint sample” models use all the information of the array together with the individual protein concentrations for each sample to estimate parameters. The array information is based on assumptions such as that the surface chemistry and therefore the interactions of antibodies probed on a slide probed with a specific antibody are similar. For example, information available for each dilution point about rate of signal increase is used to yield improved estimates of protein concentration with a lower variance. “SuperCurve” relies on a three-parameter logistic equation to model the dependency of signal intensities from unknown protein expression values.\nRecently, Liu et al. [35] employed an approach initially introduced for the analysis of high-throughput expression profiling data for loading control and variance stabilization, which was based on the invariant marker set concept [36]. This concept was adjusted to RPPA specific settings by introduction of a set of invariant proteins, so-called markers that form a virtual reference sample to normalize all samples. First, target protein signals are ranked and the variance is calculated across all samples, and data showing the highest rank variance are removed from the RPPA data set. This selection process is repeated until the number of target-protein derived data has reached a pre-determined number. Then, in this way, the reduced data set is trimmed further by removing the 25% highest and 25% lowest values. Next, averaging the remaining values of every protein across all samples generates the virtual reference sample (VS). The actual sample data is then normalized with respect to the virtual reference sample by lowess smoothing using an MA-plot approach as described in Pelz et al. [36]. So-called MA-plots or Bland-Altman-plots are often used to visualize the distribution of pairwise comparisons in transcriptomic experiments. The x-axis presents the log2 gene expression level and the y-axis reflects log2 fold-change with respect to a reference sample. This concept was employed by the VS approach and showed promising results with respect to loading effect correction and variance stabilization, and resulted in RPPA data that showed a good correlation with IHC/fluorescence in situ hybridization (FISH) data available for the same set of samples.\n\n3.4. Spatial Normalization Methods\nRPPA data quality depends strongly on the image quality obtained by the signal detection approach of choice. Certain detection methods, especially those comprising several working steps, can result in unevenly stained images caused by rim effects, for example. This spatial bias needs to be addressed by proper data analysis measures. The most obvious and most simple approach to tackle artifacts resulting from uneven staining or surface inhomogeneity is choosing a random sample distribution. However, in recent years, sophisticated methods were developed to improve RPPA data quality by co-printing of control spots.\nIn 2009, Anderson et al. [37] suggested to increase the statistical power by reducing the coefficient of variation so that variability resulting from spatial heterogeneity can be kept under control. This approach, termed “Array Microenvironment Normalization”, foresees printing a layout composed of an alternating checkerboard pattern of positive control spots and experimental sample spots. Controls were designed to match samples with respect to their total protein concentration as well as having a target protein concentration within the linear range of detection. Assuming that the relation of these concentrations is equal for all controls and independent from the position on the array, variations between individual control spots were attributed to spatial heterogeneity. Although the method improved the reproducibility of protein quantification, this approach is associated with a considerable increase of costs and efforts, as the number of samples that can be analyzed by RPPA is reduced.\nThe surface adjustment method developed by Neeley et al. [18] in 2012 requires a significantly lower number of control spots and relies on duplicate sample delivery in two differently defined printing patterns. This approach uses a generalized additive model to estimate a smoothed surface from which the positive control values are estimated for each spot of the array in relation to all other positive control spots. In case positive control spots were printed as dilution series, step-to-step differences can be used to perform an intensity-based adjustment by scaling each spot to the signal intensity of its immediate surface environment. With this method, a higher inter-slide reproducibility was obtained. However, the power of this approach was not directly compared with the one developed by Anderson and colleagues.\nA recent approach from Kaushik et al. [38] accounts for spatial variability by using a simple bi-linear interpolation technique that yields a theoretical surface representing the spatial variation as basis for a calculation of correction factors. Inter-slide and intra-slide technical replicate agreement and intra-slide biological replicate agreement were determined in a 238-slide melanoma cell line study to evaluate this method. Intra-slide reproducibility of technical replicates was good and correlation between inter-slide replicates was high, however, the evaluation via correlation was not a good measure of data quality after normalization because variability between biological replicates can occur for other reasons besides surface inhomogeneity or signal detection artifacts.\n\n3.5. Combined Methods\nAnother approach which combines quantification, loading control normalization and spatial normalization is the “SuperCurve”-based method NormaCurve, published in 2012 by Troncale et al. [22]. NormaCurve proposes basically an extended “SuperCurve” model composed of a non-parametric model to quantify relative protein expression from Hu et al. [16]. An additive extension takes into account sample effects and spatial effects. Compared to other “Super-Curve”-based models, this one allows a full and reproducible removal of spatial bias as it considers positive control and total protein stained arrays for normalization and spatial covariates for correction of the spatial bias, respectively. The resulting model was further explored to assess the reproducibility of control arrays between slides, the optimal number of replicate spots and the minimally required number of serial dilution steps, as addressed in the following section.\n\n3.6. Number of Serial Dilutions Steps\nIn general, RPPA data analysis relies on serial dilution data of samples to assess the dynamic range of the measurements and to derive valid quantitative data, as outlined by Zhang et al. [23]. To assess the optimal number of dilution steps, Troncale and colleagues [22] printed samples as 15-step serial dilutions and used this information as surrogate gold standard. Hence, data of the 2, 3, 5, 6 or 14 upper dilution steps was compared against the surrogate gold standard. Relative expression levels were estimated for each of the resulting dilution curves and compared to the “true” protein concentrations estimated from the two highest number dilution series, comprising 14 and 15 dilutions steps. Dilution series data were compared by cross-validation. A significant improvement of accuracy was observed for dilution series comprising more than three dilution steps. Based on this, authors recommend printing samples as five-step serial dilution series.\n\n3.7. Analyte Normalization for Complex Biological Samples\nParticularly complex tissue samples such as samples obtained from whole tissue specimens that might include blood vessels or show enrichment with stroma components so that additional normalization measures are required. In that case, analyte normalization, as described by Chiechi et al. [27], can correct for sample-to-sample variability but certainly requires suitable controls to permit a valid data analysis.\nMost RPPA normalization approaches described here are available as non-commercial software tools (Table 1). In any case, experimentalists and data analysts should make themselves familiar with the requirements of their data analysis pipeline, especially as this also concerns sample preparation, identification of suitable positive and negative controls and the choice of a particular detection method for signal visualization. However, it is also important to notice that data normalization comprising a large number of different steps increases the risk of over-normalization and raises the question of adequate data quality.\n\n3.8. Quality Control\nMonitoring the quality of raw data constitutes a key element of RPPA data analysis. Variability of data will be observed even under optimized experimental conditions and needs to be addressed with standardized quality control measures. Image quality control checks have mostly relied on the visual examination of slide image files, correlation analysis of technical replicates, inspection of negative control slides detected by omitting the primary antibody and included also quantile-quantile plots comparing negative control slides and actual RPPA slides.\nA considerable disadvantage of the visual inspection is a high degree of examiner variability that might produce inconsistent results. Ju and coauthors tackled this problem by setting up an automated data analysis pipeline [20]. Inspection of RPPA images relies on a generalized linear model as a logit to a logistic function returning a likelihood factor that represents slide quality. Evaluation of this automated approach showed prediction accuracies of 84%–87% when compared to a combined evaluation of three RPPA experts, considering the fact of missing a gold standard of RPPA quality control. This approach has been implemented in the “SuperCurve” R package and is available for public use. Since the classifier is array design-specific, it is of limited use in other experimental settings. However, RPPA Core Facilities where experimental settings remain unchanged over many years benefit from such a classifier. The lack of flexibility of this type of approaches portrays the need for standards for RPPA-based targeted proteomics. Individual projects with a platform-tailored experimental design still require running quality control steps manually. In such cases meaningful and intuitive display of raw data results are of great help to complement the visual inspection which might take into account spot shape and size, spot intensity, background intensity, uneven patches as well as variation in the positive controls. Zhang et al. [23] suggested to use the “serial dilution curve” as intuitive means for QC (Quality check) as depicted in Figure 3.\nFigure 3 Serial dilution curve. (A) In the serial dilution plot the observed signal is plotted against the observed signal at the next dilution step. Dilution series which are very close or identical with the identity line indicate quality problems, as the dilution series fails to generate lowered signals. a and M are the intersection points at background level and saturation level, respectively; (B) Example of dilution curves from four samples with different initial concentrations. The dilution steps remain constant; (C) Simulated RPPA data generated with the Sips model as presented in Zhang et al. [23]; (D) Serial dilution curve for simulated data shown in (C). The continuous (blue) line corresponds to the serial dilution curve. The dashed (black) line represents the identity line. Scripts for plot generation were taken from [23]. The visual inspection of RPPA images and of quantile-quantile plots is the current practice for quality control although it presents a time-consuming procedure and might entail inconsistent results. Consequently, there is definitely a need for automated and flexible quality check approaches for RPPA to exclude low quality images from RPPA data sets.\n\n3.9. Further Considerations\nAlthough more than 1000 samples may be printed on a single slide and up to a few hundred different proteins can be probed by RPPA [6,15], certain experimental settings might still involve larger numbers of samples to be analyzed in a high-throughput-fashion. Thus, several slides might be required to accommodate all samples of a study. In addition, in clinical settings it might be of interest to compare results from different studies or across different labs.\nIn this instance, issues such as inter-slide variability and normalization are pivotal but finding an appropriate normalization approach presents still a challenge [17,36]. Data of positive control spots, serial dilutions and of slides stained for total protein are required for several reasons. This data increases the accuracy of the estimated protein expression levels, adjusts for slide-to-slide variability and compensates for differences in sample loading. Additionally, effects caused by spatial artifacts can be compensated as these might be additive in comparisons of slides from different print runs or from independent signal detection runs. Thus, the experimental design should include a well-defined set of controls shared by RPPA printing runs over many years and by different groups.\nAgain, the advantage of standardized array design and analysis approaches comes forward and points towards the fact that uniquely designed experimental set-ups might be confounded by a lack of standardization. For this reason, existing methods need to be compared to define an appropriate work flow as standard for RPPA experimentation. Nevertheless, a certain flexibility regarding the experimental design of new projects and new biological questions will require different set-ups. Consequently, flexible methods are needed which in addition can be used in a standardized pipeline for data analysis. Non-flexible tools only developed for in-house solutions do not help to reach this goal in the near future.\n","divisions":[{"label":"Title","span":{"begin":0,"end":44}},{"label":"Section","span":{"begin":46,"end":3018}},{"label":"Title","span":{"begin":46,"end":70}},{"label":"Section","span":{"begin":3020,"end":5716}},{"label":"Title","span":{"begin":3020,"end":3047}},{"label":"Figure caption","span":{"begin":3248,"end":3602}},{"label":"Section","span":{"begin":5718,"end":11302}},{"label":"Title","span":{"begin":5718,"end":5752}},{"label":"Section","span":{"begin":11304,"end":14575}},{"label":"Title","span":{"begin":11304,"end":11338}},{"label":"Section","span":{"begin":14577,"end":15533}},{"label":"Title","span":{"begin":14577,"end":14598}},{"label":"Section","span":{"begin":15535,"end":16532}},{"label":"Title","span":{"begin":15535,"end":15572}},{"label":"Section","span":{"begin":16534,"end":17632}},{"label":"Title","span":{"begin":16534,"end":16591}},{"label":"Section","span":{"begin":17634,"end":20973}},{"label":"Title","span":{"begin":17634,"end":17654}},{"label":"Figure caption","span":{"begin":19774,"end":20621}},{"label":"Section","span":{"begin":20975,"end":22974}},{"label":"Title","span":{"begin":20975,"end":21002}}],"tracks":[{"project":"2_test","denotations":[{"id":"27600238-22761696-69479217","span":{"begin":2656,"end":2658},"obj":"22761696"},{"id":"27600238-22467912-69479218","span":{"begin":3014,"end":3016},"obj":"22467912"},{"id":"27600238-19914823-69479219","span":{"begin":4226,"end":4228},"obj":"19914823"},{"id":"27600238-17768165-69479220","span":{"begin":4229,"end":4231},"obj":"17768165"},{"id":"27600238-21901597-69479221","span":{"begin":4254,"end":4256},"obj":"21901597"},{"id":"27600238-19176552-69479222","span":{"begin":4382,"end":4384},"obj":"19176552"},{"id":"27600238-17599930-69479223","span":{"begin":5204,"end":5206},"obj":"17599930"},{"id":"27600238-22467912-69479224","span":{"begin":5364,"end":5366},"obj":"22467912"},{"id":"27600238-16619307-69479225","span":{"begin":7288,"end":7290},"obj":"16619307"},{"id":"27600238-17564428-69479226","span":{"begin":7291,"end":7293},"obj":"17564428"},{"id":"27600238-25374453-69479227","span":{"begin":7632,"end":7634},"obj":"25374453"},{"id":"27600238-19055840-69479228","span":{"begin":8056,"end":8058},"obj":"19055840"},{"id":"27600238-25374453-69479229","span":{"begin":8536,"end":8538},"obj":"25374453"},{"id":"27600238-19336447-69479230","span":{"begin":8576,"end":8578},"obj":"19336447"},{"id":"27600238-25374453-69479231","span":{"begin":9657,"end":9659},"obj":"25374453"},{"id":"27600238-19055840-69479232","span":{"begin":9866,"end":9868},"obj":"19055840"},{"id":"27600238-19055840-69479233","span":{"begin":10733,"end":10735},"obj":"19055840"},{"id":"27600238-19834915-69479234","span":{"begin":11985,"end":11987},"obj":"19834915"},{"id":"27600238-22550399-69479235","span":{"begin":13019,"end":13021},"obj":"22550399"},{"id":"27600238-25501559-69479236","span":{"begin":13826,"end":13828},"obj":"25501559"},{"id":"27600238-22761696-69479237","span":{"begin":14786,"end":14788},"obj":"22761696"},{"id":"27600238-17599930-69479238","span":{"begin":14943,"end":14945},"obj":"17599930"},{"id":"27600238-19176552-69479239","span":{"begin":15762,"end":15764},"obj":"19176552"},{"id":"27600238-22761696-69479240","span":{"begin":15840,"end":15842},"obj":"22761696"},{"id":"27600238-22946677-69479241","span":{"begin":16881,"end":16883},"obj":"22946677"},{"id":"27600238-25380958-69479242","span":{"begin":18437,"end":18439},"obj":"25380958"},{"id":"27600238-19176552-69479243","span":{"begin":19658,"end":19660},"obj":"19176552"},{"id":"27600238-19176552-69479244","span":{"begin":20383,"end":20385},"obj":"19176552"},{"id":"27600238-19176552-69479245","span":{"begin":20615,"end":20617},"obj":"19176552"},{"id":"27600238-23552733-69479246","span":{"begin":21134,"end":21135},"obj":"23552733"},{"id":"27600238-24777629-69479247","span":{"begin":21136,"end":21138},"obj":"24777629"},{"id":"27600238-19336447-69479248","span":{"begin":21631,"end":21633},"obj":"19336447"},{"id":"27600238-19055840-69479249","span":{"begin":21634,"end":21636},"obj":"19055840"}],"attributes":[{"subj":"27600238-22761696-69479217","pred":"source","obj":"2_test"},{"subj":"27600238-22467912-69479218","pred":"source","obj":"2_test"},{"subj":"27600238-19914823-69479219","pred":"source","obj":"2_test"},{"subj":"27600238-17768165-69479220","pred":"source","obj":"2_test"},{"subj":"27600238-21901597-69479221","pred":"source","obj":"2_test"},{"subj":"27600238-19176552-69479222","pred":"source","obj":"2_test"},{"subj":"27600238-17599930-69479223","pred":"source","obj":"2_test"},{"subj":"27600238-22467912-69479224","pred":"source","obj":"2_test"},{"subj":"27600238-16619307-69479225","pred":"source","obj":"2_test"},{"subj":"27600238-17564428-69479226","pred":"source","obj":"2_test"},{"subj":"27600238-25374453-69479227","pred":"source","obj":"2_test"},{"subj":"27600238-19055840-69479228","pred":"source","obj":"2_test"},{"subj":"27600238-25374453-69479229","pred":"source","obj":"2_test"},{"subj":"27600238-19336447-69479230","pred":"source","obj":"2_test"},{"subj":"27600238-25374453-69479231","pred":"source","obj":"2_test"},{"subj":"27600238-19055840-69479232","pred":"source","obj":"2_test"},{"subj":"27600238-19055840-69479233","pred":"source","obj":"2_test"},{"subj":"27600238-19834915-69479234","pred":"source","obj":"2_test"},{"subj":"27600238-22550399-69479235","pred":"source","obj":"2_test"},{"subj":"27600238-25501559-69479236","pred":"source","obj":"2_test"},{"subj":"27600238-22761696-69479237","pred":"source","obj":"2_test"},{"subj":"27600238-17599930-69479238","pred":"source","obj":"2_test"},{"subj":"27600238-19176552-69479239","pred":"source","obj":"2_test"},{"subj":"27600238-22761696-69479240","pred":"source","obj":"2_test"},{"subj":"27600238-22946677-69479241","pred":"source","obj":"2_test"},{"subj":"27600238-25380958-69479242","pred":"source","obj":"2_test"},{"subj":"27600238-19176552-69479243","pred":"source","obj":"2_test"},{"subj":"27600238-19176552-69479244","pred":"source","obj":"2_test"},{"subj":"27600238-19176552-69479245","pred":"source","obj":"2_test"},{"subj":"27600238-23552733-69479246","pred":"source","obj":"2_test"},{"subj":"27600238-24777629-69479247","pred":"source","obj":"2_test"},{"subj":"27600238-19336447-69479248","pred":"source","obj":"2_test"},{"subj":"27600238-19055840-69479249","pred":"source","obj":"2_test"}]}],"config":{"attribute types":[{"pred":"source","value type":"selection","values":[{"id":"2_test","color":"#a493ec","default":true}]}]}}