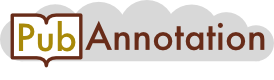
PMC:4979055 / 1230-5755
Annnotations
{"target":"https://pubannotation.org/docs/sourcedb/PMC/sourceid/4979055","sourcedb":"PMC","sourceid":"4979055","source_url":"https://www.ncbi.nlm.nih.gov/pmc/4979055","text":"1. Introduction\nIn comparison to other cancers, over the last 30 years there has been little progress in the median survival time of lung cancer patients [1]. The median survival rate remains at around 3 months. Lung cancer cases account for about 13% of the total number of cancer cases diagnosed each year. It is also an increasingly significant cause of mortality in developing countries where there are a higher proportion of smokers [2]. Non-small cell lung carcinoma (NSCLC) is the most common form of bronchial tumor. Histological analysis of the tumors can divide them into two major sub-groups, adenocarcinoma and squamous cell carcinoma [3]. Some cases do not fall clearly into either of the sub-groups and so these are assigned to having a mixed morphology. Outside of these two main groupings there are also a small number of other rare histological groups.\nMicroarrays can contribute to the treatment and therapy of NSCLC in two important ways. The first is by allowing earlier detection of the tumors through the use of biomarkers [4]. The essential feature of a biomarker is that it must provide an accurate method for detecting the disease, by having a high sensitivity and high specificity. Another important factor is that biomarker detection should be non‑invasive. This is particularly important in lung cancer as you do not want to have to take samples of lung tissue itself and so biomarkers need to be available for either breath samples or blood samples. The second way that microarrays can contribute to the treatment of NSCLC is by identifying genes that are differentially expressed in the tumors and that could therefore be a target for new anti-cancer drugs. In this case the focus for the gene expression is the tumor tissue itself compared to normal lung tissue. Recently a number of large-scale studies have been under-taken to understand the factors involved in tumor progression [5,6,7]. These studies have used a variety of genomic, transcriptomic and proteomic methods and have identified, copy number variation, mutation and DNA methylation as well as changes in gene expression as differentiating between healthy lung tissue and tumors.\nOne of the past difficulties in identifying genes that are important in determining cancer progression (“oncogenes”) has been the large number of differentially expressed genes. Microarray data is often noisy, and there is almost always a lack of technical replicates [8,9,10] One of the best known examples of microarray analysis is the diffuse large B-cell lymphoma study by Alizadeh et al. [11]. The data from this study has been used in a number of subsequent re-analyses, which have produced differing results. One study even suggested that computationally the data was inadequate for resolving the problem of identifying the significant genes [12]. This is a fundamental problem when you have high dimensional data, where a large number of variables produce a small number of outcomes. In this case a large number of gene expression values contribute to a small number of phenotypes (either being a tumor cell or not being a tumor cell). As an absolute minimum the number of biological samples should to exceed the number of independent variables. In the case of microarrays the genes are not expressed independently and so removing genes that show high degrees of correlation, and genes that do not vary at all between all of the different phenotypes can reduce the number of variables. This is why it is usual to perform a gene filtering step in microarray analysis in order to reduce the number of genes that are considered for testing for differential expression [13,14]. The problem is that this filtering can be rather arbitrary and might have an effect on the results of the analysis [15,16]. It would be better to use the complete dataset with appropriate corrections for multiple statistical tests. Another factor in the processing of the microarray data that has been shown to affect the results is normalization of the samples. Once a set of differentially expressed genes has been identified these are often then reduced further by the use of gene set enrichment analysis (GSEA), to show which functional annotations are significantly up or down regulated [17]. An alternative to using GSEA, is to use a network based approach using data from biological pathways.\nIn this paper seven publicly available datasets for NSCLC are reanalyzed and a network based analysis is carried out using the pathways from the Reactome database [18].","divisions":[{"label":"Title","span":{"begin":0,"end":15}}],"tracks":[{"project":"2_test","denotations":[{"id":"27600345-21351269-69480466","span":{"begin":439,"end":440},"obj":"21351269"},{"id":"27600345-11829087-69480467","span":{"begin":648,"end":649},"obj":"11829087"},{"id":"27600345-22960745-69480468","span":{"begin":1914,"end":1915},"obj":"22960745"},{"id":"27600345-22407829-69480469","span":{"begin":1916,"end":1917},"obj":"22407829"},{"id":"27600345-15755808-69480470","span":{"begin":2444,"end":2445},"obj":"15755808"},{"id":"27600345-12454643-69480471","span":{"begin":2446,"end":2447},"obj":"12454643"},{"id":"27600345-16369572-69480472","span":{"begin":2448,"end":2450},"obj":"16369572"},{"id":"27600345-10676951-69480473","span":{"begin":2569,"end":2571},"obj":"10676951"},{"id":"27600345-10521349-69480474","span":{"begin":3773,"end":3775},"obj":"10521349"},{"id":"27600345-23209689-69480475","span":{"begin":3776,"end":3778},"obj":"23209689"},{"id":"27600345-16199517-69480476","span":{"begin":4250,"end":4252},"obj":"16199517"},{"id":"27600345-21067998-69480477","span":{"begin":4521,"end":4523},"obj":"21067998"}],"attributes":[{"subj":"27600345-21351269-69480466","pred":"source","obj":"2_test"},{"subj":"27600345-11829087-69480467","pred":"source","obj":"2_test"},{"subj":"27600345-22960745-69480468","pred":"source","obj":"2_test"},{"subj":"27600345-22407829-69480469","pred":"source","obj":"2_test"},{"subj":"27600345-15755808-69480470","pred":"source","obj":"2_test"},{"subj":"27600345-12454643-69480471","pred":"source","obj":"2_test"},{"subj":"27600345-16369572-69480472","pred":"source","obj":"2_test"},{"subj":"27600345-10676951-69480473","pred":"source","obj":"2_test"},{"subj":"27600345-10521349-69480474","pred":"source","obj":"2_test"},{"subj":"27600345-23209689-69480475","pred":"source","obj":"2_test"},{"subj":"27600345-16199517-69480476","pred":"source","obj":"2_test"},{"subj":"27600345-21067998-69480477","pred":"source","obj":"2_test"}]}],"config":{"attribute types":[{"pred":"source","value type":"selection","values":[{"id":"2_test","color":"#93ece9","default":true}]}]}}