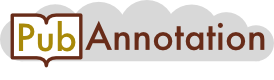
PMC:4979051 / 18835-21132
Annnotations
{"target":"https://pubannotation.org/docs/sourcedb/PMC/sourceid/4979051","sourcedb":"PMC","sourceid":"4979051","source_url":"https://www.ncbi.nlm.nih.gov/pmc/4979051","text":"4. Discussion\nOver the years, a large number of preprocessing algorithms have been suggested. Many of them are based on underlying manipulations and assumptions that are difficult to understand. For example, the Plier method, suggested by Affymetrix, has been regarded as a good choice [28] despite being considered having biologically implausible assumptions [29]. Among the steps required for preprocessing, background correction is probably the most important since errors due to this step can more severely affect the genes with low expression values. Because of that, the standard deviation becomes dependent on the gene expression level. To overcome this issue, t-test modifications like SAM, LIMMA and other shrinkage methods have been developed and considered better choices. In fact, these methodologies present a good improvement in the task of identifying the differentially expressed genes when compared with standard t-test, as we showed using spike-in experiments. However, we highlight that by modifying the pooled variance, these methods tend to ignore the genes with low differences in expression and also, although improving the sensitivity when compared with standard t-test, these strategies do not show a significant improvement in the task of selecting a robust predictive list.\nHere, we introduce an alternative approach for statistical analysis of microarray data that skips the background correction step, leading to a more powerful and robust test. Our procedure makes use of the standard t-test location- and scale-invariance property and relies on a well-established model that relates the probe intensity level with the gene expression level. Our method is easy to understand and to implement, however it does not offer an estimate of the expression level for each gene. We highlight that if the question under consideration is the identification of differentially expressed genes (DEG) or the predictive gene lists (PGLs), then intermediate estimation of expression levels is an unnecessary detour, and our method is useful. We also point out that our methodology is useful when the important genes are expected to have a small difference in expression. In this case, shrinkage methodologies are not recommended since they tend to ignore genes with small fold change.","divisions":[{"label":"Title","span":{"begin":0,"end":13}}],"tracks":[{"project":"2_test","denotations":[{"id":"27600352-19461970-69481375","span":{"begin":287,"end":289},"obj":"19461970"},{"id":"27600352-19259420-69481376","span":{"begin":361,"end":363},"obj":"19259420"}],"attributes":[{"subj":"27600352-19461970-69481375","pred":"source","obj":"2_test"},{"subj":"27600352-19259420-69481376","pred":"source","obj":"2_test"}]}],"config":{"attribute types":[{"pred":"source","value type":"selection","values":[{"id":"2_test","color":"#e1ec93","default":true}]}]}}