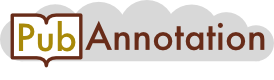
PMC:4979051 / 1544-9441
Annnotations
{"target":"https://pubannotation.org/docs/sourcedb/PMC/sourceid/4979051","sourcedb":"PMC","sourceid":"4979051","source_url":"https://www.ncbi.nlm.nih.gov/pmc/4979051","text":"1. Introduction\nMicroarrays are a widely used methodology for measuring the expression of thousands of genes simultaneously. A common application of microarrays is to compare the expression levels of genes in samples drawn from two different experimental conditions in order to determine which genes are differentially expressed. Typically, a microarray dataset has in the order of ten thousand genes, whereas only a small subset of these genes is relevant, and the number of samples range from a few tens up to a few hundreds. Multiple processing steps are required in order to identify the genes of interest and errors in these steps compromise the reliability of the analysis. As a result, the comparison of the lists of differentially expressed genes (DEG) reported by different groups have revealed very small overlap [1,2]. Therefore, understanding the sources of this lack of robustness and the development of more reliable methods for the identification of the DEG remains a crucial issue.\nThe preprocessing of the raw probe intensity data constitutes the initial step in microarray data analysis and its goal is to infer a variable that represents the gene concentration. Usually, the preprocessing analysis is performed in three steps: normalization, background correction and summarization. First, the normalization is performed to reduce sources of variation of non-biological origin among the arrays in order to make them comparable. Next, in background correction, the background intensity due to non-specific hybridization and optical noise is inferred and subtracted from the normalized intensity. Finally, in the summarization step, the multiple probe intensities for each probe set is combined into a single gene expression value.\nThe probe intensity measure Y can be modeled as a combination of background B (due to optical noise and non-specific hybridization) and specific hybridization S [3,4]:(1) Yijg=Bjg+Sijg=Bjg+ϕjgθig where θig denotes the expression measure for the gene g in the ith sample, and the indexes i, j and g represent the sample, the probe and the gene, respectively. This model assumes that the intensity value Yijg increases linearly as θig increases, but the rate of increase of the expression θig is different for each probe j and is represented by ϕjg. It is also assumed that after normalization, the background Bjg is independent of the sample [5].\nBackground correction is the most critical step because errors associated with the inference of this variable strongly affect the genes with low expression values—therefore decreasing the statistical power of further analysis. In the Affymetrix platform it is common to include an extra probe—referred to as the mismatch—created by changing the middle (13th) base with the intention of directly measuring the effect of background noise. The methods dChip [3] as well as the Affymetrix methods MAS5.0 and PLIER [6] use the mismatch intensity as an estimate of the background. Therefore, Irizarry et al. [4] showed that subtracting the mismatch intensity from the perfect match intensity (Y) results in expression estimates with an exaggerated error, mainly for low expression values. Thus, they proposed a background adjustment step that ignores the mismatch intensities, named RMA [4], which uses the posterior mean E[S|Y] as a background adjustment.\nOnce a gene expression estimation is obtained, it is common to take the logarithmic transformation of this variable because the difference in transformed data, the fold change, is considered easier to manipulate and interpret. Some preprocessing algorithms return the expression concentration in log scale (like RMA and Plier) while others do not (like MAS5.0). It seems that there is no consensus about what scale should be chosen when using t-test or others ranking methods. Nevertheless, this choice is important because the t-test, for example, is not invariant under monotone transformations, i.e., the results of the test is different if x is replaced by log2x.\nAfter preprocessing, several types of statistical tests can be applied in order to find the differentially expressed genes under two conditions. The independent t-test is the most popular statistical approach to select differentially expressed genes presumably due to its simplicity to implement and interpret. In this test, it is assumed that the data follows a normal distribution and, under the null hypothesis H0, the average of the gene expression in both experimental conditions are the same. A discordance of the data from what is specified in H0 can be quantified as the probability of observing a value for the test statistic that is at least as extreme as the value that was actually observed. This probability p is referred to as the p-value and a threshold value α can be chosen so that the hypothesis H0 is rejected if p≤α. Then, a statistical significance can be assigned, which is a statistical assessment of whether observations reflect a pattern rather than just chance. The t variable, which follows a Student’s t-distribution when the normality of the data holds, is defined as: (2) tg=〈θ^gi〉i∈A−〈θ^gi〉i∈Bsg where θ^ig is the inferred expression level by a preprocessing method and 〈θ^gi〉i∈A and 〈θ^gi〉i∈B are the expression averages over the samples i under the conditions A and B, respectively.\nThe empirical standard deviation is defined as (3) sg=a∑i∈Aθ^gi−〈θ^gi〉i∈A2+∑i∈Bθ^gi−〈θ^gi〉i∈B2 where a=(1/nA+1/nB)/(nA+nB−2) and the constants nA and nB represent the number of samples under the experimental conditions A and B, respectively.\nDespite being widely used, the t-test has been subject to criticism in the literature since the error in preprocessed data tend to be asymmetric [7,8,9,10]. As a consequence, the variance estimation is dependent on the expression level because the genes with low expression levels are more affected by the errors in background correction. Because of that, modified versions of the standard t-test have been developed as alternative approaches [11,12,13,14,15]. Those approaches modify the t-test by using a procedure called variance shrinkage, which consists in modifying the denominator of the t variable by combining the gene-specific variance and a predictive variance.\nThe method Significance Analysis of Microarrays (SAM) [11] is the most popular alternative to t-test and it consists in a modification of the standard t-test by the inclusion of an extra variable s0, added to the pooled variance sg. The extra variable s0 is chosen in order to minimize the dependency of t on the expression level θ^g and the significant genes are identified comparing the modified t variable with a similar variable obtained under random permutations among the samples. Modifications of the t-test based on Empirical Bayes [13,14,16] have also been widely used and have been considered a good choice [9], and the method Linear Models for Microarray Data (LIMMA) [14] is the most widely used.\nThere is no shortage of more sophisticated alternatives to the t-test. However, given the widespread tendency to use the standard t-test, understanding the reason of its poor performance remains a crucial issue. Here, we show that the standard t-test can be used at the probe level, skipping background correction by using the normalized intensity data directly in the t-test. Several methods have approached this problem at the probe level [17,18,19,20], but all these approaches use background-corrected data. Our methodology outperforms the standard t-test using preprocessed data and the most used shrinkage methods SAM and LIMMA, therefore suggesting that background correction is a major source of error in microarray analysis. The methods were compared in terms of sensitivity by using the Affymetrix spike-in dataset, a commonly used benchmark, and in terms of robustness by using leukemia, breast cancer and multiple myeloma datasets.","divisions":[{"label":"Title","span":{"begin":0,"end":15}}],"tracks":[{"project":"2_test","denotations":[{"id":"27600352-15308542-69481340","span":{"begin":824,"end":825},"obj":"15308542"},{"id":"27600352-16585533-69481341","span":{"begin":826,"end":827},"obj":"16585533"},{"id":"27600352-11134512-69481342","span":{"begin":1911,"end":1912},"obj":"11134512"},{"id":"27600352-12925520-69481343","span":{"begin":1913,"end":1914},"obj":"12925520"},{"id":"27600352-20048383-69481344","span":{"begin":2391,"end":2392},"obj":"20048383"},{"id":"27600352-11134512-69481345","span":{"begin":2851,"end":2852},"obj":"11134512"},{"id":"27600352-12925520-69481346","span":{"begin":2998,"end":2999},"obj":"12925520"},{"id":"27600352-12925520-69481347","span":{"begin":3277,"end":3278},"obj":"12925520"},{"id":"27600352-16026597-69481348","span":{"begin":5718,"end":5719},"obj":"16026597"},{"id":"27600352-16964229-69481349","span":{"begin":5720,"end":5721},"obj":"16964229"},{"id":"27600352-16369572-69481350","span":{"begin":5722,"end":5723},"obj":"16369572"},{"id":"27600352-20838429-69481351","span":{"begin":5724,"end":5726},"obj":"20838429"},{"id":"27600352-11309499-69481352","span":{"begin":6016,"end":6018},"obj":"11309499"},{"id":"27600352-15618528-69481353","span":{"begin":6019,"end":6021},"obj":"15618528"},{"id":"27600352-14668230-69481354","span":{"begin":6022,"end":6024},"obj":"14668230"},{"id":"27600352-16646809-69481355","span":{"begin":6025,"end":6027},"obj":"16646809"},{"id":"27600352-20663218-69481356","span":{"begin":6028,"end":6030},"obj":"20663218"},{"id":"27600352-11309499-69481357","span":{"begin":6300,"end":6302},"obj":"11309499"},{"id":"27600352-14668230-69481358","span":{"begin":6786,"end":6788},"obj":"14668230"},{"id":"27600352-16646809-69481359","span":{"begin":6789,"end":6791},"obj":"16646809"},{"id":"27600352-11395427-69481360","span":{"begin":6792,"end":6794},"obj":"11395427"},{"id":"27600352-16369572-69481361","span":{"begin":6863,"end":6864},"obj":"16369572"},{"id":"27600352-16646809-69481362","span":{"begin":6925,"end":6927},"obj":"16646809"},{"id":"27600352-20504334-69481363","span":{"begin":7396,"end":7398},"obj":"20504334"},{"id":"27600352-16934150-69481364","span":{"begin":7399,"end":7401},"obj":"16934150"},{"id":"27600352-18366694-69481365","span":{"begin":7405,"end":7407},"obj":"18366694"}],"attributes":[{"subj":"27600352-15308542-69481340","pred":"source","obj":"2_test"},{"subj":"27600352-16585533-69481341","pred":"source","obj":"2_test"},{"subj":"27600352-11134512-69481342","pred":"source","obj":"2_test"},{"subj":"27600352-12925520-69481343","pred":"source","obj":"2_test"},{"subj":"27600352-20048383-69481344","pred":"source","obj":"2_test"},{"subj":"27600352-11134512-69481345","pred":"source","obj":"2_test"},{"subj":"27600352-12925520-69481346","pred":"source","obj":"2_test"},{"subj":"27600352-12925520-69481347","pred":"source","obj":"2_test"},{"subj":"27600352-16026597-69481348","pred":"source","obj":"2_test"},{"subj":"27600352-16964229-69481349","pred":"source","obj":"2_test"},{"subj":"27600352-16369572-69481350","pred":"source","obj":"2_test"},{"subj":"27600352-20838429-69481351","pred":"source","obj":"2_test"},{"subj":"27600352-11309499-69481352","pred":"source","obj":"2_test"},{"subj":"27600352-15618528-69481353","pred":"source","obj":"2_test"},{"subj":"27600352-14668230-69481354","pred":"source","obj":"2_test"},{"subj":"27600352-16646809-69481355","pred":"source","obj":"2_test"},{"subj":"27600352-20663218-69481356","pred":"source","obj":"2_test"},{"subj":"27600352-11309499-69481357","pred":"source","obj":"2_test"},{"subj":"27600352-14668230-69481358","pred":"source","obj":"2_test"},{"subj":"27600352-16646809-69481359","pred":"source","obj":"2_test"},{"subj":"27600352-11395427-69481360","pred":"source","obj":"2_test"},{"subj":"27600352-16369572-69481361","pred":"source","obj":"2_test"},{"subj":"27600352-16646809-69481362","pred":"source","obj":"2_test"},{"subj":"27600352-20504334-69481363","pred":"source","obj":"2_test"},{"subj":"27600352-16934150-69481364","pred":"source","obj":"2_test"},{"subj":"27600352-18366694-69481365","pred":"source","obj":"2_test"}]}],"config":{"attribute types":[{"pred":"source","value type":"selection","values":[{"id":"2_test","color":"#ece193","default":true}]}]}}