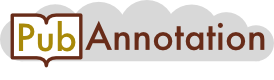
PMC:4979051 / 10997-13112
Annnotations
{"target":"https://pubannotation.org/docs/sourcedb/PMC/sourceid/4979051","sourcedb":"PMC","sourceid":"4979051","source_url":"https://www.ncbi.nlm.nih.gov/pmc/4979051","text":"3.1. Sensitivity\nThe sensitivity in identifying the differentially expressed genes was evaluated by using spike-in experiments. This dataset was obtained from measurements on specifically constructed and controlled DNA microarrays experiments using human genome HG-U133. These experiments were designed by Affymetrix for the purpose of developing and validating the Affymetrix Microarray Suite (MAS) 5.0 expression algorithm [22]. The samples follow a Latin Square design consisting of 42 genes in 14 different concentrations (with 3 repetition each), see Table A1 in the Appendix.\nTo simulate an analysis in which two conditions are compared, we rearranged the data as follows. First, the genes from samples 1 to 3 were attributed to condition A and those from samples 4 to 6 were attributed to condition B. The exceptions were the genes from samples 40 to 42 because we want to probe the effect of small differences in expression, i.e., we only evaluate differences in the fold change equal to 2. We kept the procedure using the replications 4–6 as condition A and 7–9 as condition B, again excluding the 0 and 512 pM differences. We ended up with 14 “experiments” in the two conditions and 23,000 genes of which 39 are differentially expressed. This procedure is similar to the one done in Affycomp [23] to obtain a Receiver Operating Characteristic (ROC) curve with fold change equal to 2.\nThe results are presented as an ROC curve whose definition is given by a true positive (TP) rate against a false positive (FP) rate obtained at different threshold values. To plot a single average ROC curve, we calculated the average TP and FP over the 14 experiments obtained in the Affymetrix spike-in data rearrangement. The proposed approach showed a significant improvement on the sensitivity in recognizing the differentially expressed genes when the t-test was used as the ranking method, Figure 1A. Its performance is similar to the SAM and LIMMA best performance (when using RMA as a preprocessing algorithm), Figure 1B. In all applied tests the data were normalized using the quantile normalization method [24].","divisions":[{"label":"Title","span":{"begin":0,"end":16}}],"tracks":[{"project":"2_test","denotations":[{"id":"27600352-14960458-69481366","span":{"begin":1303,"end":1305},"obj":"14960458"},{"id":"27600352-12538238-69481367","span":{"begin":2111,"end":2113},"obj":"12538238"}],"attributes":[{"subj":"27600352-14960458-69481366","pred":"source","obj":"2_test"},{"subj":"27600352-12538238-69481367","pred":"source","obj":"2_test"}]}],"config":{"attribute types":[{"pred":"source","value type":"selection","values":[{"id":"2_test","color":"#b593ec","default":true}]}]}}