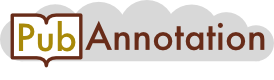
PMC:4636534 / 9027-13041
Annnotations
TEST0
{"project":"TEST0","denotations":[{"id":"26003789-77-83-67117","span":{"begin":259,"end":261},"obj":"[\"22990083\"]"},{"id":"26003789-88-93-67118","span":{"begin":2116,"end":2117},"obj":"[\"19944595\"]"},{"id":"26003789-235-241-67119","span":{"begin":2691,"end":2693},"obj":"[\"22990084\"]"}],"text":"Developing the decision model\nFollowing the definition of the decision problem, the second step consists of developing the decision model by choosing an appropriate model structure. In medical imaging, decision trees and Markov models are most commonly used [24].\n\nThe decision tree\nTo visualize and calculate the effects of imaging on costs and health outcome in a static situation and short time frame, a flow model in the form of a decision tree can be used and easily made in Excel (Microsoft, Redmond, WA) or other spreadsheet application software. A hypothetical cohort of patients passes through the model and is divided over the different pathways in the decision tree, according to the assigned probabilities. A decision tree starts with a decision node, after which the alternative strategies are depicted. In each strategy, patients in the model do or do not have the disease or condition of interest and undergo an imaging test for diagnostic workup. Medical care decisions are based on the imaging results and determine whether patients undergo further imaging or other diagnostic tests and possible therapy. At the end of the decision tree, the cohort is divided into different possible health outcomes due to characteristics of diagnostic imaging and choice of therapy. These health outcomes can be assigned QALYs and are based on whether patients are alive and, when they are alive, whether they experience problems that may impact quality of life. Costs are the sum of the costs that occur in the pathways, such as costs for the imaging tests and eventual therapy, and health outcome related costs. Two or more diagnostic tests can be compared with each other: using the sensitivity and specificity of the different imaging modalities, the probabilities to be accurately diagnosed with corresponding optimal treatment can be assessed in the decision tree model. A schematic decision tree for the example of 18FDG-PET-CT versus CT versus chest X-ray in the follow-up of curatively treated NSCLC is shown in Fig. 2, which is a simplified version of a decision tree used in a recently published study [6].\nFig. 2 Schematic example of a decision tree model\n\nThe Markov model\nIn non-static situations, such as in chronic diseases or malignancies, health states of the patients may alter depending on imaging and treatment options. In such situations, a Markov model can be used to reflect alterations in health states during the period of CEA. In a Markov model, the clinical situation is described in terms of the conditions that patients can be in (‘health states’), how they can move in such states (‘transitions’), and how likely such moves are (‘transition probabilities’) [25]. The time frame of the analysis can be chosen as suitable for the underlying medical question with respect to the available literature. For CEA of screening imaging tests and follow-up imaging in cancer, a long frame over several years or lifetime may be adequate. For example, in a Markov model used for the decision problem on optimal follow-up imaging after treated NSCLC, patients can be in the health states “no evidence of disease”, “progressive disease” or “dead” (Fig. 3). Progressive disease can be detected (i.e., the true positive test results) or not (the false negative test results). No evidence of disease represents the paths in the decision tree with true negatives and the false positives test results. At each time point of follow-up imaging, patients stay in the state they are, or move to different states, e.g., from no evidence of disease to progressive disease (and vice versa) or from no evidence of disease or progressive disease to dead. Please note that in this example, the Markov model directly follows on the health outcomes in the decision tree. It is common practice in imaging studies to combine a decision tree for the short-term diagnostic accuracy and treatment decision with a Markov model for the longer-term consequences of the disease.\nFig. 3 Schematic example of a Markov model"}
0_colil
{"project":"0_colil","denotations":[{"id":"26003789-22990083-67117","span":{"begin":259,"end":261},"obj":"22990083"},{"id":"26003789-19944595-67118","span":{"begin":2116,"end":2117},"obj":"19944595"},{"id":"26003789-22990084-67119","span":{"begin":2691,"end":2693},"obj":"22990084"}],"text":"Developing the decision model\nFollowing the definition of the decision problem, the second step consists of developing the decision model by choosing an appropriate model structure. In medical imaging, decision trees and Markov models are most commonly used [24].\n\nThe decision tree\nTo visualize and calculate the effects of imaging on costs and health outcome in a static situation and short time frame, a flow model in the form of a decision tree can be used and easily made in Excel (Microsoft, Redmond, WA) or other spreadsheet application software. A hypothetical cohort of patients passes through the model and is divided over the different pathways in the decision tree, according to the assigned probabilities. A decision tree starts with a decision node, after which the alternative strategies are depicted. In each strategy, patients in the model do or do not have the disease or condition of interest and undergo an imaging test for diagnostic workup. Medical care decisions are based on the imaging results and determine whether patients undergo further imaging or other diagnostic tests and possible therapy. At the end of the decision tree, the cohort is divided into different possible health outcomes due to characteristics of diagnostic imaging and choice of therapy. These health outcomes can be assigned QALYs and are based on whether patients are alive and, when they are alive, whether they experience problems that may impact quality of life. Costs are the sum of the costs that occur in the pathways, such as costs for the imaging tests and eventual therapy, and health outcome related costs. Two or more diagnostic tests can be compared with each other: using the sensitivity and specificity of the different imaging modalities, the probabilities to be accurately diagnosed with corresponding optimal treatment can be assessed in the decision tree model. A schematic decision tree for the example of 18FDG-PET-CT versus CT versus chest X-ray in the follow-up of curatively treated NSCLC is shown in Fig. 2, which is a simplified version of a decision tree used in a recently published study [6].\nFig. 2 Schematic example of a decision tree model\n\nThe Markov model\nIn non-static situations, such as in chronic diseases or malignancies, health states of the patients may alter depending on imaging and treatment options. In such situations, a Markov model can be used to reflect alterations in health states during the period of CEA. In a Markov model, the clinical situation is described in terms of the conditions that patients can be in (‘health states’), how they can move in such states (‘transitions’), and how likely such moves are (‘transition probabilities’) [25]. The time frame of the analysis can be chosen as suitable for the underlying medical question with respect to the available literature. For CEA of screening imaging tests and follow-up imaging in cancer, a long frame over several years or lifetime may be adequate. For example, in a Markov model used for the decision problem on optimal follow-up imaging after treated NSCLC, patients can be in the health states “no evidence of disease”, “progressive disease” or “dead” (Fig. 3). Progressive disease can be detected (i.e., the true positive test results) or not (the false negative test results). No evidence of disease represents the paths in the decision tree with true negatives and the false positives test results. At each time point of follow-up imaging, patients stay in the state they are, or move to different states, e.g., from no evidence of disease to progressive disease (and vice versa) or from no evidence of disease or progressive disease to dead. Please note that in this example, the Markov model directly follows on the health outcomes in the decision tree. It is common practice in imaging studies to combine a decision tree for the short-term diagnostic accuracy and treatment decision with a Markov model for the longer-term consequences of the disease.\nFig. 3 Schematic example of a Markov model"}
MyTest
{"project":"MyTest","denotations":[{"id":"26003789-22990083-29373360","span":{"begin":259,"end":261},"obj":"22990083"},{"id":"26003789-19944595-29373361","span":{"begin":2116,"end":2117},"obj":"19944595"},{"id":"26003789-22990084-29373362","span":{"begin":2691,"end":2693},"obj":"22990084"}],"namespaces":[{"prefix":"_base","uri":"https://www.uniprot.org/uniprot/testbase"},{"prefix":"UniProtKB","uri":"https://www.uniprot.org/uniprot/"},{"prefix":"uniprot","uri":"https://www.uniprot.org/uniprotkb/"}],"text":"Developing the decision model\nFollowing the definition of the decision problem, the second step consists of developing the decision model by choosing an appropriate model structure. In medical imaging, decision trees and Markov models are most commonly used [24].\n\nThe decision tree\nTo visualize and calculate the effects of imaging on costs and health outcome in a static situation and short time frame, a flow model in the form of a decision tree can be used and easily made in Excel (Microsoft, Redmond, WA) or other spreadsheet application software. A hypothetical cohort of patients passes through the model and is divided over the different pathways in the decision tree, according to the assigned probabilities. A decision tree starts with a decision node, after which the alternative strategies are depicted. In each strategy, patients in the model do or do not have the disease or condition of interest and undergo an imaging test for diagnostic workup. Medical care decisions are based on the imaging results and determine whether patients undergo further imaging or other diagnostic tests and possible therapy. At the end of the decision tree, the cohort is divided into different possible health outcomes due to characteristics of diagnostic imaging and choice of therapy. These health outcomes can be assigned QALYs and are based on whether patients are alive and, when they are alive, whether they experience problems that may impact quality of life. Costs are the sum of the costs that occur in the pathways, such as costs for the imaging tests and eventual therapy, and health outcome related costs. Two or more diagnostic tests can be compared with each other: using the sensitivity and specificity of the different imaging modalities, the probabilities to be accurately diagnosed with corresponding optimal treatment can be assessed in the decision tree model. A schematic decision tree for the example of 18FDG-PET-CT versus CT versus chest X-ray in the follow-up of curatively treated NSCLC is shown in Fig. 2, which is a simplified version of a decision tree used in a recently published study [6].\nFig. 2 Schematic example of a decision tree model\n\nThe Markov model\nIn non-static situations, such as in chronic diseases or malignancies, health states of the patients may alter depending on imaging and treatment options. In such situations, a Markov model can be used to reflect alterations in health states during the period of CEA. In a Markov model, the clinical situation is described in terms of the conditions that patients can be in (‘health states’), how they can move in such states (‘transitions’), and how likely such moves are (‘transition probabilities’) [25]. The time frame of the analysis can be chosen as suitable for the underlying medical question with respect to the available literature. For CEA of screening imaging tests and follow-up imaging in cancer, a long frame over several years or lifetime may be adequate. For example, in a Markov model used for the decision problem on optimal follow-up imaging after treated NSCLC, patients can be in the health states “no evidence of disease”, “progressive disease” or “dead” (Fig. 3). Progressive disease can be detected (i.e., the true positive test results) or not (the false negative test results). No evidence of disease represents the paths in the decision tree with true negatives and the false positives test results. At each time point of follow-up imaging, patients stay in the state they are, or move to different states, e.g., from no evidence of disease to progressive disease (and vice versa) or from no evidence of disease or progressive disease to dead. Please note that in this example, the Markov model directly follows on the health outcomes in the decision tree. It is common practice in imaging studies to combine a decision tree for the short-term diagnostic accuracy and treatment decision with a Markov model for the longer-term consequences of the disease.\nFig. 3 Schematic example of a Markov model"}
2_test
{"project":"2_test","denotations":[{"id":"26003789-22990083-29373360","span":{"begin":259,"end":261},"obj":"22990083"},{"id":"26003789-19944595-29373361","span":{"begin":2116,"end":2117},"obj":"19944595"},{"id":"26003789-22990084-29373362","span":{"begin":2691,"end":2693},"obj":"22990084"}],"text":"Developing the decision model\nFollowing the definition of the decision problem, the second step consists of developing the decision model by choosing an appropriate model structure. In medical imaging, decision trees and Markov models are most commonly used [24].\n\nThe decision tree\nTo visualize and calculate the effects of imaging on costs and health outcome in a static situation and short time frame, a flow model in the form of a decision tree can be used and easily made in Excel (Microsoft, Redmond, WA) or other spreadsheet application software. A hypothetical cohort of patients passes through the model and is divided over the different pathways in the decision tree, according to the assigned probabilities. A decision tree starts with a decision node, after which the alternative strategies are depicted. In each strategy, patients in the model do or do not have the disease or condition of interest and undergo an imaging test for diagnostic workup. Medical care decisions are based on the imaging results and determine whether patients undergo further imaging or other diagnostic tests and possible therapy. At the end of the decision tree, the cohort is divided into different possible health outcomes due to characteristics of diagnostic imaging and choice of therapy. These health outcomes can be assigned QALYs and are based on whether patients are alive and, when they are alive, whether they experience problems that may impact quality of life. Costs are the sum of the costs that occur in the pathways, such as costs for the imaging tests and eventual therapy, and health outcome related costs. Two or more diagnostic tests can be compared with each other: using the sensitivity and specificity of the different imaging modalities, the probabilities to be accurately diagnosed with corresponding optimal treatment can be assessed in the decision tree model. A schematic decision tree for the example of 18FDG-PET-CT versus CT versus chest X-ray in the follow-up of curatively treated NSCLC is shown in Fig. 2, which is a simplified version of a decision tree used in a recently published study [6].\nFig. 2 Schematic example of a decision tree model\n\nThe Markov model\nIn non-static situations, such as in chronic diseases or malignancies, health states of the patients may alter depending on imaging and treatment options. In such situations, a Markov model can be used to reflect alterations in health states during the period of CEA. In a Markov model, the clinical situation is described in terms of the conditions that patients can be in (‘health states’), how they can move in such states (‘transitions’), and how likely such moves are (‘transition probabilities’) [25]. The time frame of the analysis can be chosen as suitable for the underlying medical question with respect to the available literature. For CEA of screening imaging tests and follow-up imaging in cancer, a long frame over several years or lifetime may be adequate. For example, in a Markov model used for the decision problem on optimal follow-up imaging after treated NSCLC, patients can be in the health states “no evidence of disease”, “progressive disease” or “dead” (Fig. 3). Progressive disease can be detected (i.e., the true positive test results) or not (the false negative test results). No evidence of disease represents the paths in the decision tree with true negatives and the false positives test results. At each time point of follow-up imaging, patients stay in the state they are, or move to different states, e.g., from no evidence of disease to progressive disease (and vice versa) or from no evidence of disease or progressive disease to dead. Please note that in this example, the Markov model directly follows on the health outcomes in the decision tree. It is common practice in imaging studies to combine a decision tree for the short-term diagnostic accuracy and treatment decision with a Markov model for the longer-term consequences of the disease.\nFig. 3 Schematic example of a Markov model"}