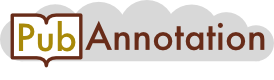
PMC:3480682 / 17165-20012
Annnotations
{"target":"https://pubannotation.org/docs/sourcedb/PMC/sourceid/3480682","sourcedb":"PMC","sourceid":"3480682","source_url":"https://www.ncbi.nlm.nih.gov/pmc/3480682","text":"Identifying CNVs subject to selection\nAs described above, the effect of selection is parameterized by αi in the logistic regression. Thus, two models in the logistic, one that includes both effects of αi (i.e., αi ≠ 0) and βj (selection model, M2) and another one that does not include the effect of selection (i.e., αi = 0) and only includes the effect of βj (neutral model, M1), are considered.\nWe can decide in a Bayesian framework whether or not there is selection at all. This is done by estimating the posterior probabilities for two models for each locus in the logistic regression: neutral (M1) and selection (model 2, M2), on the basis of a dataset N = { aij }. We use a reversible jump MCMC algorithm [28] to estimate the posterior probability of each one of these models, and this is done separately for each locus i. We can then have posterior probability that a locus is subject to selection - that is, P(αi ≠0). This probability is estimated directly from the output of the MCMC by simply counting the number of times αi is included in the model (see Foll and Gaggiotti [27] for a more detailed explanation).\nIn BayeScan, selection decision (model choice decision), with a specific goal of detecting outlier loci in mind, is actually performed by using posterior odds (PO). For this, we first need to describe 'Bayes factors.' For the two models of neutral (M1) and selection (M2), the Bayes factor (BF) for model M2 is given by BF = P(N | M2)/P(N | M1), where N = { aij } is a dataset and P indicates probability. This BF provides a degree of evidence in favor of one model versus another. In the context of multiple testing - that is, testing a large number of loci simultaneously - we also need to incorporate our skepticism about the chance that each locus is under selection. This is done by setting the prior odds for the neutral model P(M1)/P(M2). We make selection decisions by using PO, which is defined as PO = P(M2|N)/P(M1|N) = BF × P(M2)/P(M1). PO are simply the ratio of posterior probabilities and indicate how much more likely the model with selection (M2) is compared to the neutral model (M1) (GENEPOP software). PO of 0.5-1.0, 1.0-1.5, 1.5-2.0, and 2-∞ are, respectively, considered as substantial, strong, very strong, and decisive evidence for selection [14].\nA big advantage of posterior probabilities is that they directly allow the control of the false discovery rate (FDR), where FDR is defined as the expected proportion of false positives among outlier loci. Controlling for FDR has a much greater power than controlling for family-wise error rates using Bonferroni correction, for example [29]. In BayeScan, by first setting a target FDR, the PO threshold achieving this FDR is determined, and outliers equal to or above this PO threshold are listed in the output of R that is provided along with BayeScan.","divisions":[{"label":"Title","span":{"begin":0,"end":37}}],"tracks":[{"project":"2_test","denotations":[{"id":"23105934-18780740-44845829","span":{"begin":1085,"end":1087},"obj":"18780740"}],"attributes":[{"subj":"23105934-18780740-44845829","pred":"source","obj":"2_test"}]}],"config":{"attribute types":[{"pred":"source","value type":"selection","values":[{"id":"2_test","color":"#cbec93","default":true}]}]}}