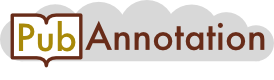
PMC:1687185 / 4750-5763
Annnotations
{"target":"https://pubannotation.org/docs/sourcedb/PMC/sourceid/1687185","sourcedb":"PMC","sourceid":"1687185","source_url":"https://www.ncbi.nlm.nih.gov/pmc/1687185","text":"Existing approaches used to solve the motif finding problem can be classified into two main categories [7]. The first group of algorithms utilizes a generative probabilistic representation of the nucleotide positions to discover a consensus DNA pattern that maximizes the information content score. In this approach, the original problem of finding the best consensus pattern is formulated as finding the global maximum of a continuous non-convex function. The main advantage of this approach is that the generated profiles are highly representative of the signals being determined [8]. The disadvantage, however, is that the determination of the \"best\" motif cannot be guaranteed and is often a very difficult problem since finding global maximum of any continuous non-convex function is a challenging problem. Current algorithms converge to the nearest local optimum instead of the global solution. Gibbs sampling [5], MEME [6], greedy CONSENSUS algorithm [9] and HMM based methods [10] belong to this category.","tracks":[{"project":"2_test","denotations":[{"id":"17129371-8211139-1691518","span":{"begin":917,"end":918},"obj":"8211139"},{"id":"17129371-10487864-1691519","span":{"begin":959,"end":960},"obj":"10487864"},{"id":"17129371-9918945-1691520","span":{"begin":985,"end":987},"obj":"9918945"}],"attributes":[{"subj":"17129371-8211139-1691518","pred":"source","obj":"2_test"},{"subj":"17129371-10487864-1691519","pred":"source","obj":"2_test"},{"subj":"17129371-9918945-1691520","pred":"source","obj":"2_test"}]}],"config":{"attribute types":[{"pred":"source","value type":"selection","values":[{"id":"2_test","color":"#ccec93","default":true}]}]}}