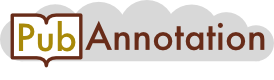
PMC:1570465 / 10632-41365
Annnotations
{"target":"https://pubannotation.org/docs/sourcedb/PMC/sourceid/1570465","sourcedb":"PMC","sourceid":"1570465","source_url":"https://www.ncbi.nlm.nih.gov/pmc/1570465","text":"Methods\n\nBroad problem formulation\nMotif discovery is modeled here as the problem of finding an ungapped local multiple sequence alignment (MSA) of fixed length with the best sum-of-pairs (SP) score. That is, given N sequences {S1, ..., SN} and a block length parameter l, the goal is to find an l-long subsequence from each input sequence so that the total similarity among selected blocks is maximized. More formally, let sik MathType@MTEF@5@5@+=feaafiart1ev1aaatCvAUfKttLearuWrP9MDH5MBPbIqV92AaeXatLxBI9gBaebbnrfifHhDYfgasaacH8akY=wiFfYdH8Gipec8Eeeu0xXdbba9frFj0=OqFfea0dXdd9vqai=hGuQ8kuc9pgc9s8qqaq=dirpe0xb9q8qiLsFr0=vr0=vr0dc8meaabaqaciaacaGaaeqabaqabeGadaaakeaacqWGZbWCdaqhaaWcbaGaemyAaKgabaGaem4AaSgaaaaa@3102@ refer to the l-long subsequence in sequence Si beginning in position k and let sim(x, y) denote a similarity score between the l-long subsequences x, y. The objective is then to find the set of positions {k1, ..., kN} in each sequence, such that the SP-score ∑i\u003cj sim (siki MathType@MTEF@5@5@+=feaafiart1ev1aaatCvAUfKttLearuWrP9MDH5MBPbIqV92AaeXatLxBI9gBaebbnrfifHhDYfgasaacH8akY=wiFfYdH8Gipec8Eeeu0xXdbba9frFj0=OqFfea0dXdd9vqai=hGuQ8kuc9pgc9s8qqaq=dirpe0xb9q8qiLsFr0=vr0=vr0dc8meaabaqaciaacaGaaeqabaqabeGadaaakeaacqWGZbWCdaqhaaWcbaGaemyAaKgabaGaem4AaS2aaSbaaWqaaiabdMgaPbqabaaaaaaa@328A@, sjkj MathType@MTEF@5@5@+=feaafiart1ev1aaatCvAUfKttLearuWrP9MDH5MBPbIqV92AaeXatLxBI9gBaebbnrfifHhDYfgasaacH8akY=wiFfYdH8Gipec8Eeeu0xXdbba9frFj0=OqFfea0dXdd9vqai=hGuQ8kuc9pgc9s8qqaq=dirpe0xb9q8qiLsFr0=vr0=vr0dc8meaabaqaciaacaGaaeqabaqabeGadaaakeaacqWGZbWCdaqhaaWcbaGaemOAaOgabaGaem4AaS2aaSbaaWqaaiabdQgaQbqabaaaaaaa@328E@) is maximized.\nThis problem can be formulated in graph-theoretic terms [40]. Let G be an undirected N-partite graph with node set V1 ∪ ... ∪ VN, where Vi includes a node u for each l-long subsequence sik MathType@MTEF@5@5@+=feaafiart1ev1aaatCvAUfKttLearuWrP9MDH5MBPbIqV92AaeXatLxBI9gBaebbnrfifHhDYfgasaacH8akY=wiFfYdH8Gipec8Eeeu0xXdbba9frFj0=OqFfea0dXdd9vqai=hGuQ8kuc9pgc9s8qqaq=dirpe0xb9q8qiLsFr0=vr0=vr0dc8meaabaqaciaacaGaaeqabaqabeGadaaakeaacqWGZbWCdaqhaaWcbaGaemyAaKgabaGaem4AaSgaaaaa@3102@ in the i-th sequence. Note that the subsequences corresponding to two consecutive vertices overlap in l - 1 positions, and that the Vi's may have varying sizes. Each pair of nodes u ∈ Vi and v ∈ Vj (i ≠ j), corresponding to subsequences sik MathType@MTEF@5@5@+=feaafiart1ev1aaatCvAUfKttLearuWrP9MDH5MBPbIqV92AaeXatLxBI9gBaebbnrfifHhDYfgasaacH8akY=wiFfYdH8Gipec8Eeeu0xXdbba9frFj0=OqFfea0dXdd9vqai=hGuQ8kuc9pgc9s8qqaq=dirpe0xb9q8qiLsFr0=vr0=vr0dc8meaabaqaciaacaGaaeqabaqabeGadaaakeaacqWGZbWCdaqhaaWcbaGaemyAaKgabaGaem4AaSgaaaaa@3102@ and sjk′ MathType@MTEF@5@5@+=feaafiart1ev1aaatCvAUfKttLearuWrP9MDH5MBPbIqV92AaeXatLxBI9gBaebbnrfifHhDYfgasaacH8akY=wiFfYdH8Gipec8Eeeu0xXdbba9frFj0=OqFfea0dXdd9vqai=hGuQ8kuc9pgc9s8qqaq=dirpe0xb9q8qiLsFr0=vr0=vr0dc8meaabaqaciaacaGaaeqabaqabeGadaaakeaacqWGZbWCdaqhaaWcbaGaemOAaOgabaGafm4AaSMbauaaaaaaaa@3110@ in Si and Sj respectively, is joined by an edge with weight of wuv = sim (sik MathType@MTEF@5@5@+=feaafiart1ev1aaatCvAUfKttLearuWrP9MDH5MBPbIqV92AaeXatLxBI9gBaebbnrfifHhDYfgasaacH8akY=wiFfYdH8Gipec8Eeeu0xXdbba9frFj0=OqFfea0dXdd9vqai=hGuQ8kuc9pgc9s8qqaq=dirpe0xb9q8qiLsFr0=vr0=vr0dc8meaabaqaciaacaGaaeqabaqabeGadaaakeaacqWGZbWCdaqhaaWcbaGaemyAaKgabaGaem4AaSgaaaaa@3102@, sjk′ MathType@MTEF@5@5@+=feaafiart1ev1aaatCvAUfKttLearuWrP9MDH5MBPbIqV92AaeXatLxBI9gBaebbnrfifHhDYfgasaacH8akY=wiFfYdH8Gipec8Eeeu0xXdbba9frFj0=OqFfea0dXdd9vqai=hGuQ8kuc9pgc9s8qqaq=dirpe0xb9q8qiLsFr0=vr0=vr0dc8meaabaqaciaacaGaaeqabaqabeGadaaakeaacqWGZbWCdaqhaaWcbaGaemOAaOgabaGafm4AaSMbauaaaaaaaa@3110@). By this construction G is a complete N-partite graph. The MSA is achieved by picking the highest weight N-partite clique in graph G.\nSimilarity between l-long subsequences can be defined using a simple scoring scheme, such as counting up the number of matching bases or amino acids when the subsequences are aligned. However, for DNA sequences, the background distribution of the input sequence can vary substantially, and in order to reward matches of more infrequent bases, instead of using 1 for a match, we assign a score of log(1/f(b)) for a base b pairing, where f(b) is the zero-corrected frequency of base b in the background, and 0 for any mismatch. (We also experimented with a scheme that assigns a score of 1/f(b) for a base b match; both methods perform similarly). In practice, we work with integral scores by scaling the floating point numbers to the desired degree of accuracy and rounding (here, we use the scale factor of 100). For protein sequences, on the other hand, compositional bias is not as major an issue, and instead, to better capture the relationships between the amino acids, we score the similarity between two amino acids using substitution matrices. This assigns higher scores to more favorable substitutions and better reflects shared biochemical properties of such pairings. We experiment with both PAM [11] and BLOSUM [12] matrix families.\n\nInteger linear programming formulation\nThe motif finding problem can be formulated as an ILP as follows. For a graph G = (V, E) corresponding to the motif finding problem, where V = V1 ∪ ... ∪ VN and E = {(u, v): u ∈ Vi, v ∈ Vj, i ≠ j}, we introduce a binary decision variable xu for every vertex u, and a binary decision variable yuv for every edge (u, v). Setting xu to 1 corresponds to selecting vertex u for the clique and thus choosing the sequence position corresponding to u in the alignment. Edge variable yuv is set to 1 if both endpoints u and v are selected for the clique. Then the following ILP solves the motif finding problem formulated above:\nMaximize ∑ ( u , v ) ∈ E w u v ⋅ y u v subject to ∑ u ∈ V j x u = 1 for 1 ≤ j ≤ N ( n o d e constraints ) ∑ u ∈ V j y u v = x v for 1 ≤ j ≤ N , v ∈ V \\ V j ( e d g e constraints ) x u , y u v ∈ { 0 , 1 } for u ∈ V , ( u , v ) ∈ E MathType@MTEF@5@5@+=feaafiart1ev1aaatCvAUfKttLearuWrP9MDH5MBPbIqV92AaeXatLxBI9gBaebbnrfifHhDYfgasaacH8akY=wiFfYdH8Gipec8Eeeu0xXdbba9frFj0=OqFfea0dXdd9vqai=hGuQ8kuc9pgc9s8qqaq=dirpe0xb9q8qiLsFr0=vr0=vr0dc8meaabaqaciaacaGaaeqabaqabeGadaaakeaafaqaaeqbdaaaaeaacqqGnbqtcqqGHbqycqqG4baEcqqGPbqAcqqGTbqBcqqGPbqAcqqG6bGEcqqGLbqzaeaadaaeqaqaaiabdEha3naaBaaaleaacqWG1bqDcqWG2bGDaeqaaOGaeyyXICTaemyEaK3aaSbaaSqaaiabdwha1jabdAha2bqabaaabaGaeiikaGIaemyDauNaeiilaWIaemODayNaeiykaKIaeyicI4SaemyraueabeqdcqGHris5aaGcbaaabaGaee4CamNaeeyDauNaeeOyaiMaeeOAaOMaeeyzauMaee4yamMaeeiDaqNaeeiiaaIaeeiDaqNaee4Ba8gabaaabaaabaWaaabeaeaacqWG4baEdaWgaaWcbaGaemyDauhabeaakiabg2da9iabigdaXaWcbaGaemyDauNaeyicI4SaemOvay1aaSbaaWqaaiabdQgaQbqabaaaleqaniabggHiLdaakeaacqqGMbGzcqqGVbWBcqqGYbGCcqqGGaaicqaIXaqmcqGHKjYOcqWGQbGAcqGHKjYOcqWGobGtaeaacqGGOaakcqWGUbGBcqWGVbWBcqWGKbazcqWGLbqzcqqGGaaicqqGJbWycqqGVbWBcqqGUbGBcqqGZbWCcqqG0baDcqqGYbGCcqqGHbqycqqGPbqAcqqGUbGBcqqG0baDcqqGZbWCcqGGPaqkaeaadaaeqaqaaiabdMha5naaBaaaleaacqWG1bqDcqWG2bGDaeqaaOGaeyypa0JaemiEaG3aaSbaaSqaaiabdAha2bqabaaabaGaemyDauNaeyicI4SaemOvay1aaSbaaWqaaiabdQgaQbqabaaaleqaniabggHiLdaakeaacqqGMbGzcqqGVbWBcqqGYbGCcqqGGaaicqaIXaqmcqGHKjYOcqWGQbGAcqGHKjYOcqWGobGtcqGGSaalcqWG2bGDcqGHiiIZcqWGwbGvcqGGCbaxcqWGwbGvdaWgaaWcbaGaemOAaOgabeaaaOqaaiabcIcaOiabdwgaLjabdsgaKjabdEgaNjabdwgaLjabbccaGiabbogaJjabb+gaVjabb6gaUjabbohaZjabbsha0jabbkhaYjabbggaHjabbMgaPjabb6gaUjabbsha0jabbohaZjabcMcaPaqaaiabdIha4naaBaaaleaacqWG1bqDaeqaaOGaeiilaWIaemyEaK3aaSbaaSqaaiabdwha1jabdAha2bqabaGccqGHiiIZcqGG7bWEcqaIWaamcqGGSaalcqaIXaqmcqGG9bqFaeaacqqGMbGzcqqGVbWBcqqGYbGCcqqGGaaicqWG1bqDcqGHiiIZcqWGwbGvcqGGSaalcqGGOaakcqWG1bqDcqGGSaalcqWG2bGDcqGGPaqkcqGHiiIZcqWGfbqraeaaaaaaaa@E7E9@\nThe first set of constraints ensures that exactly one vertex is picked from every graph part, corresponding to one position being chosen from every input sequence. The second set of constraints relates vertex variables to edge variables, allowing the objective function to be expressed in terms of finding a maximum edge-weight clique. An edge is chosen only if it connects two chosen vertices. This formulation is similar to that used by us [41] in fixed-backbone protein design and homology modeling.\nILP itself is NP-hard, but replacing the integrality constraints on the x and y variables with 0 ≤ xu, yuv ≤ 1 gives an LP that can be solved in polynomial time. If the LP solution happens to be integral, it is guaranteed to be optimal for the original ILP and motif finding problem. Non-integral solutions, on the other hand, are not feasible for the ILP and do not translate to a selection of positions for the MSA problem; in these cases, more computationally intensive ILP solvers must be invoked.\n\nGraph pruning techniques\nIn this section, we introduce a number of successively more powerful optimality-preserving dead-end elimination (DEE) techniques for pruning graphs corresponding to motif finding problems. The basic idea is to discard vertices and/or edges that cannot possibly be part of the optimal solution.\n\nBasic clique-bounds DEE\nThe idea of our first pruning technique is as follows. Suppose there exists a clique of weight C* in G. Then a vertex u, whose participation in any possible clique in G reduces the weight of that clique below C*, is incompatible with the optimal alignment and can be safely eliminated (similar to [36]).\nFor u ∈ Vi define star(u) to be a selection of vertices from every graph part other than Vi. Let Fu be the value induced by the edge weights for a star(u) that form best pairwise alignments with u:\nF u = ∑ j ≠ i max v ∈ V j w u v ( 1 ) MathType@MTEF@5@5@+=feaafiart1ev1aaatCvAUfKttLearuWrP9MDH5MBPbIqV92AaeXatLxBI9gBaebbnrfifHhDYfgasaacH8akY=wiFfYdH8Gipec8Eeeu0xXdbba9frFj0=OqFfea0dXdd9vqai=hGuQ8kuc9pgc9s8qqaq=dirpe0xb9q8qiLsFr0=vr0=vr0dc8meaabaqaciaacaGaaeqabaqabeGadaaakeaacqWGgbGrdaWgaaWcbaGaemyDauhabeaakiabg2da9maaqafabaWaaCbeaeaacyGGTbqBcqGGHbqycqGG4baEaSqaaiabdAha2jabgIGiolabdAfawnaaBaaameaacqWGQbGAaeqaaaWcbeaakiabbccaGaWcbaGaemOAaOMaeyiyIKRaemyAaKgabeqdcqGHris5aOGaem4DaC3aaSbaaSqaaiabdwha1jabdAha2bqabaGccaWLjaGaaCzcamaabmGabaGaeGymaedacaGLOaGaayzkaaaaaa@4A63@\nIf u were to participate in any clique in G, it cannot possibly contribute more than Fu to the weight of the clique. Similarly, let be the value of the best possible star(u) among all u ∈ Vi:\nF i ∗ = max u ∈ V i F u ( 2 ) MathType@MTEF@5@5@+=feaafiart1ev1aaatCvAUfKttLearuWrP9MDH5MBPbIqV92AaeXatLxBI9gBaebbnrfifHhDYfgasaacH8akY=wiFfYdH8Gipec8Eeeu0xXdbba9frFj0=OqFfea0dXdd9vqai=hGuQ8kuc9pgc9s8qqaq=dirpe0xb9q8qiLsFr0=vr0=vr0dc8meaabaqaciaacaGaaeqabaqabeGadaaakeaacqWGgbGrdaqhaaWcbaGaemyAaKgabaGaey4fIOcaaOGaeyypa0ZaaCbeaeaacyGGTbqBcqGGHbqycqGG4baEaSqaaiabdwha1jabgIGiolabdAfawnaaBaaameaacqWGPbqAaeqaaaWcbeaakiabdAeagnaaBaaaleaacqWG1bqDaeqaaOGaaCzcaiaaxMaadaqadiqaaiabikdaYaGaayjkaiaawMcaaaaa@41EF@\nFi∗ MathType@MTEF@5@5@+=feaafiart1ev1aaatCvAUfKttLearuWrP9MDH5MBPbIqV92AaeXatLxBI9gBaebbnrfifHhDYfgasaacH8akY=wiFfYdH8Gipec8Eeeu0xXdbba9frFj0=OqFfea0dXdd9vqai=hGuQ8kuc9pgc9s8qqaq=dirpe0xb9q8qiLsFr0=vr0=vr0dc8meaabaqaciaacaGaaeqabaqabeGadaaakeaacqWGgbGrdaqhaaWcbaGaemyAaKgabaGaey4fIOcaaaaa@3038@ is an upper bound on what any vertex in Vi can contribute to any alignment.\nNow, if Fz, the most a vertex z ∈ Vk can contribute to a clique, assuming the best possible contributions from all other graph parts, is insufficient compared to the value C* of an existing clique, i.e. if\nF z \u003c 2 × C ∗ − ∑ i ≠ k F i ∗ , ( 3 ) MathType@MTEF@5@5@+=feaafiart1ev1aaatCvAUfKttLearuWrP9MDH5MBPbIqV92AaeXatLxBI9gBaebbnrfifHhDYfgasaacH8akY=wiFfYdH8Gipec8Eeeu0xXdbba9frFj0=OqFfea0dXdd9vqai=hGuQ8kuc9pgc9s8qqaq=dirpe0xb9q8qiLsFr0=vr0=vr0dc8meaabaqaciaacaGaaeqabaqabeGadaaakeaacqWGgbGrdaWgaaWcbaGaemOEaOhabeaakiabgYda8iabikdaYiabgEna0kabdoeadnaaCaaaleqabaGaey4fIOcaaOGaeyOeI0YaaabuaeaacqWGgbGrdaqhaaWcbaGaemyAaKgabaGaey4fIOcaaaqaaiabdMgaPjabgcMi5kabdUgaRbqab0GaeyyeIuoakiabcYcaSiaaxMaacaWLjaWaaeWaceaacqaIZaWmaiaawIcacaGLPaaaaaa@4575@\nz can be discarded. The clique value C* is used with a factor of 2 since two edges are accounted for between every pair of graph parts in the above inequality.\nIn fact, the values of Fi∗ MathType@MTEF@5@5@+=feaafiart1ev1aaatCvAUfKttLearuWrP9MDH5MBPbIqV92AaeXatLxBI9gBaebbnrfifHhDYfgasaacH8akY=wiFfYdH8Gipec8Eeeu0xXdbba9frFj0=OqFfea0dXdd9vqai=hGuQ8kuc9pgc9s8qqaq=dirpe0xb9q8qiLsFr0=vr0=vr0dc8meaabaqaciaacaGaaeqabaqabeGadaaakeaacqWGgbGrdaqhaaWcbaGaemyAaKgabaGaey4fIOcaaaaa@3038@ are further constrained by requiring a connection to z when z is under consideration. That is, when considering a node z ∈ Vk to eliminate, and calculating Fi∗ MathType@MTEF@5@5@+=feaafiart1ev1aaatCvAUfKttLearuWrP9MDH5MBPbIqV92AaeXatLxBI9gBaebbnrfifHhDYfgasaacH8akY=wiFfYdH8Gipec8Eeeu0xXdbba9frFj0=OqFfea0dXdd9vqai=hGuQ8kuc9pgc9s8qqaq=dirpe0xb9q8qiLsFr0=vr0=vr0dc8meaabaqaciaacaGaaeqabaqabeGadaaakeaacqWGgbGrdaqhaaWcbaGaemyAaKgabaGaey4fIOcaaaaa@3038@ according to Equation 2 among all possible u ∈ Vi, the Fu of Equation 1 is instead computed as:\nF u = w z u + ∑ j ≠ i , k max v ∈ V j w u v ( 4 ) MathType@MTEF@5@5@+=feaafiart1ev1aaatCvAUfKttLearuWrP9MDH5MBPbIqV92AaeXatLxBI9gBaebbnrfifHhDYfgasaacH8akY=wiFfYdH8Gipec8Eeeu0xXdbba9frFj0=OqFfea0dXdd9vqai=hGuQ8kuc9pgc9s8qqaq=dirpe0xb9q8qiLsFr0=vr0=vr0dc8meaabaqaciaacaGaaeqabaqabeGadaaakeaacqWGgbGrdaWgaaWcbaGaemyDauhabeaakiabg2da9iabdEha3naaBaaaleaacqWG6bGEcqWG1bqDaeqaaOGaey4kaSYaaabuaeaadaWfqaqaaiGbc2gaTjabcggaHjabcIha4bWcbaGaemODayNaeyicI4SaemOvay1aaSbaaWqaaiabdQgaQbqabaaaleqaaaqaaiabdQgaQjabgcMi5kabdMgaPjabcYcaSiabdUgaRbqab0GaeyyeIuoakiabbccaGiabdEha3naaBaaaleaacqWG1bqDcqWG2bGDaeqaaOGaaCzcaiaaxMaadaqadiqaaiabisda0aGaayjkaiaawMcaaaaa@5212@\nThe value of C* can be computed from any \"good\" alignment. We use the weight of the clique imposed by the best overall star.\n\nTighter constraints for clique-bounds DEE\nFor a vertex u ∈ Vi and every other Vj, an edge has to connect u to some v ∈ Vj in any alignment. When calculating Fu, we can constrain its value by considering three-way alignments and requiring that the vertices in the best star(u) chosen as neighbors of u in graph parts other than Vj are also good matches to v. Performing this computation for every pair of u, Vj and considering every edge incident on u would be too costly. Therefore, we only consider such three-way alignments for every vertex u ∈ Vi and the next part Vi+1 of the graph (with the last and first parts paired). Essentially, this procedure shifts the emphasis onto edges, allowing better alignments and bounds, and yet eliminates vertices by considering the best edge incident on it.\nFor a given edge (u, v) with endpoints u ∈ Vi and v ∈ Vi+1 we consider an adjacent double star with two centers at u and v, and sharing all the endpoints xj in the other graph parts, denoted as dstar(u, v); the weight of such a dstar(u, v) is 2wuv+∑j≠ij≠i+1(wuxj+wvxj) MathType@MTEF@5@5@+=feaafiart1ev1aaatCvAUfKttLearuWrP9MDH5MBPbIqV92AaeXatLxBI9gBaebbnrfifHhDYfgasaacH8akY=wiFfYdH8Gipec8Eeeu0xXdbba9frFj0=OqFfea0dXdd9vqai=hGuQ8kuc9pgc9s8qqaq=dirpe0xb9q8qiLsFr0=vr0=vr0dc8meaabaqaciaacaGaaeqabaqabeGadaaakeaacqaIYaGmcqWG3bWDdaWgaaWcbaGaemyDauNaemODayhabeaakiabgUcaRmaaqadabaGaeiikaGIaem4DaC3aaSbaaSqaaiabdwha1jabdIha4naaBaaameaacqWGQbGAaeqaaaWcbeaakiabgUcaRiabdEha3naaBaaaleaacqWG2bGDcqWG4baEdaWgaaadbaGaemOAaOgabeaaaSqabaGccqGGPaqkaSqaauaabeqaceaaaeaacqWGQbGAcqGHGjsUcqWGPbqAaeaacqWGQbGAcqGHGjsUcqWGPbqAcqGHRaWkcqaIXaqmaaaabaaaniabggHiLdaaaa@4EE6@. Now consider a clique {u1 ∈ V1, ..., uN ∈ VN} of some value C*, and the sum of its double stars:\n∑ i = 1 N ( 2 w u i u i + 1 + ∑ j ≠ i j ≠ i + 1 ( w u j u i + w u j u i + 1 ) ) = 2 ∑ i = 1 N ∑ j ≠ i w u j u i ( 5 ) MathType@MTEF@5@5@+=feaafiart1ev1aaatCvAUfKttLearuWrP9MDH5MBPbIqV92AaeXatLxBI9gBaebbnrfifHhDYfgasaacH8akY=wiFfYdH8Gipec8Eeeu0xXdbba9frFj0=OqFfea0dXdd9vqai=hGuQ8kuc9pgc9s8qqaq=dirpe0xb9q8qiLsFr0=vr0=vr0dc8meaabaqaciaacaGaaeqabaqabeGadaaakeaadaaeWbqaaiabcIcaOiabikdaYiabdEha3naaBaaaleaacqWG1bqDdaWgaaadbaGaemyAaKgabeaaliabdwha1naaBaaameaacqWGPbqAcqGHRaWkcqaIXaqmaeqaaaWcbeaakiabgUcaRmaaqafabaGaeiikaGIaem4DaC3aaSbaaSqaaiabdwha1naaBaaameaacqWGQbGAaeqaaSGaemyDau3aaSbaaWqaaiabdMgaPbqabaaaleqaaOGaey4kaSIaem4DaC3aaSbaaSqaaiabdwha1naaBaaameaacqWGQbGAaeqaaSGaemyDau3aaSbaaWqaaiabdMgaPjabgUcaRiabigdaXaqabaaaleqaaOGaeiykaKIaeiykaKcaleaafaqabeGabaaabaGaemOAaOMaeyiyIKRaemyAaKgabaGaemOAaOMaeyiyIKRaemyAaKMaey4kaSIaeGymaedaaaqab0GaeyyeIuoaaSqaaiabdMgaPjabg2da9iabigdaXaqaaiabd6eaobqdcqGHris5aOGaeyypa0JaeGOmaiZaaabCaeaadaaeqbqaaiabdEha3naaBaaaleaacqWG1bqDdaWgaaadbaGaemOAaOgabeaaliabdwha1naaBaaameaacqWGPbqAaeqaaaWcbeaaaeaacqWGQbGAcqGHGjsUcqWGPbqAaeqaniabggHiLdaaleaacqWGPbqAcqGH9aqpcqaIXaqmaeaacqWGobGta0GaeyyeIuoakiaaxMaacaWLjaWaaeWaceaacqaI1aqnaiaawIcacaGLPaaaaaa@7C24@\nThis sum is equal to 4C*, as each edge (ui, uj) is counted four times. We define Fuv with for an edge (u, v) with endpoints u ∈ Vi and v ∈ Vi+1 as\nFuv can be viewed as the weight of the best dstar centered at the pair of vertices u, v (or edge (u, v)) and it is the best possible contribution to any alignment, if the edge (u, v) was required to be a part of the alignment. We define Fu for u ∈ Vi and Fi∗ MathType@MTEF@5@5@+=feaafiart1ev1aaatCvAUfKttLearuWrP9MDH5MBPbIqV92AaeXatLxBI9gBaebbnrfifHhDYfgasaacH8akY=wiFfYdH8Gipec8Eeeu0xXdbba9frFj0=OqFfea0dXdd9vqai=hGuQ8kuc9pgc9s8qqaq=dirpe0xb9q8qiLsFr0=vr0=vr0dc8meaabaqaciaacaGaaeqabaqabeGadaaakeaacqWGgbGrdaqhaaWcbaGaemyAaKgabaGaey4fIOcaaaaa@3038@ for part i similarly to the above definitions as\nF u = max v ∈ V i + 1 F u v ( 7 ) MathType@MTEF@5@5@+=feaafiart1ev1aaatCvAUfKttLearuWrP9MDH5MBPbIqV92AaeXatLxBI9gBaebbnrfifHhDYfgasaacH8akY=wiFfYdH8Gipec8Eeeu0xXdbba9frFj0=OqFfea0dXdd9vqai=hGuQ8kuc9pgc9s8qqaq=dirpe0xb9q8qiLsFr0=vr0=vr0dc8meaabaqaciaacaGaaeqabaqabeGadaaakeaacqWGgbGrdaWgaaWcbaGaemyDauhabeaakiabg2da9maaxababaGagiyBa0MaeiyyaeMaeiiEaGhaleaacqWG2bGDcqGHiiIZcqWGwbGvdaWgaaadbaGaemyAaKMaey4kaSIaeGymaedabeaaaSqabaGccqWGgbGrdaWgaaWcbaGaemyDauNaemODayhabeaakiaaxMaacaWLjaWaaeWaceaacqaI3aWnaiaawIcacaGLPaaaaaa@446A@\nF i ∗ = max u ∈ V i F u ( 8 ) MathType@MTEF@5@5@+=feaafiart1ev1aaatCvAUfKttLearuWrP9MDH5MBPbIqV92AaeXatLxBI9gBaebbnrfifHhDYfgasaacH8akY=wiFfYdH8Gipec8Eeeu0xXdbba9frFj0=OqFfea0dXdd9vqai=hGuQ8kuc9pgc9s8qqaq=dirpe0xb9q8qiLsFr0=vr0=vr0dc8meaabaqaciaacaGaaeqabaqabeGadaaakeaacqWGgbGrdaqhaaWcbaGaemyAaKgabaGaey4fIOcaaOGaeyypa0ZaaCbeaeaacyGGTbqBcqGGHbqycqGG4baEaSqaaiabdwha1jabgIGiolabdAfawnaaBaaameaacqWGPbqAaeqaaaWcbeaakiabdAeagnaaBaaaleaacqWG1bqDaeqaaOGaaCzcaiaaxMaadaqadiqaaiabiIda4aGaayjkaiaawMcaaaaa@41FB@\nFu is the value of the best dstar centered on vertex u ∈ Vi and some vertex v ∈ Vi+1, and Fi∗ MathType@MTEF@5@5@+=feaafiart1ev1aaatCvAUfKttLearuWrP9MDH5MBPbIqV92AaeXatLxBI9gBaebbnrfifHhDYfgasaacH8akY=wiFfYdH8Gipec8Eeeu0xXdbba9frFj0=OqFfea0dXdd9vqai=hGuQ8kuc9pgc9s8qqaq=dirpe0xb9q8qiLsFr0=vr0=vr0dc8meaabaqaciaacaGaaeqabaqabeGadaaakeaacqWGgbGrdaqhaaWcbaGaemyAaKgabaGaey4fIOcaaaaa@3038@ is the value of the best dstar centered on any pair of vertices u ∈ Vi and v ∈ Vi+1.\nFor any clique {u1 ∈ V1, ..., uN ∈ VN} of value C* in the graph, by Equations 5–8 we have\n4 C ∗ ≤ ∑ i = 1... N F u i u i + 1 ≤ ∑ i = 1... N F u i ≤ ∑ i = 1... N F i ∗ ( 9 ) MathType@MTEF@5@5@+=feaafiart1ev1aaatCvAUfKttLearuWrP9MDH5MBPbIqV92AaeXatLxBI9gBaebbnrfifHhDYfgasaacH8akY=wiFfYdH8Gipec8Eeeu0xXdbba9frFj0=OqFfea0dXdd9vqai=hGuQ8kuc9pgc9s8qqaq=dirpe0xb9q8qiLsFr0=vr0=vr0dc8meaabaqaciaacaGaaeqabaqabeGadaaakeaacqaI0aancqWGdbWqdaahaaWcbeqaaiabgEHiQaaakiabgsMiJoaaqafabaGaemOray0aaSbaaSqaaiabdwha1naaBaaameaacqWGPbqAaeqaaSGaemyDau3aaSbaaWqaaiabdMgaPjabgUcaRiabigdaXaqabaaaleqaaaqaaiabdMgaPjabg2da9iabigdaXiabc6caUiabc6caUiabc6caUiabd6eaobqab0GaeyyeIuoakiabgsMiJoaaqafabaGaemOray0aaSbaaSqaaiabdwha1naaBaaameaacqWGPbqAaeqaaaWcbeaaaeaacqWGPbqAcqGH9aqpcqaIXaqmcqGGUaGlcqGGUaGlcqGGUaGlcqWGobGtaeqaniabggHiLdGccqGHKjYOdaaeqbqaaiabdAeagnaaDaaaleaacqWGPbqAaeaacqGHxiIkaaaabaGaemyAaKMaeyypa0JaeGymaeJaeiOla4IaeiOla4IaeiOla4IaemOta4eabeqdcqGHris5aOGaaCzcaiaaxMaadaqadiqaaiabiMda5aGaayjkaiaawMcaaaaa@6583@\nThen Equation 3, with 2C* replaced by 4C*, can be used to eliminate vertices in the same way as before, eliminating a vertex z in a particular graph part if Fz, the value of its best adjacent dstar, is insufficient considering best possible contributions from all other graph parts. For best pruning results the value of C* should be as high as possible; we choose C* as the clique weight induced by the best overall dstar.\n\nGraph decomposition\nWe also use a divide-and-conquer graph decomposition approach for pruning vertices. For every graph part i and vertex u ∈ Vi we consider induced subgraphs Gu = (Vu, Eu) in turn, where Vu = u ∪ V\\Vi. Application of the clique-bounds DEE technique to graphs Gu is very effective since one of the graph parts, Giu MathType@MTEF@5@5@+=feaafiart1ev1aaatCvAUfKttLearuWrP9MDH5MBPbIqV92AaeXatLxBI9gBaebbnrfifHhDYfgasaacH8akY=wiFfYdH8Gipec8Eeeu0xXdbba9frFj0=OqFfea0dXdd9vqai=hGuQ8kuc9pgc9s8qqaq=dirpe0xb9q8qiLsFr0=vr0=vr0dc8meaabaqaciaacaGaaeqabaqabeGadaaakeaacqWGhbWrdaqhaaWcbaGaemyAaKgabaGaemyDauhaaaaa@30BE@ contains only one vertex, u, and all the F and F* values that need to be recomputed for the new graph Gu are greatly constrained. The process of updating the F and F* values is efficient as the changes are localized to one part in the graph. Importantly, the best known clique value C* remains intact, since the clique of that larger value exists in the original graph and can be used for the decomposed one, helping to eliminate vertices. For some of the vertices u, iterative application of the DEE criterion and re-computation of the F and F* values causes Gu to become disconnected, implying that vertex u cannot be part of the optimal alignment. Such a vertex u is marked for deletion, and that information is propagated to all subsequently considered induced subgraphs, further constraining the corresponding F and F* values and helping to eliminate other vertices in turn.\n\nStatistical significance\nOnce we have found a motif of a particular SP-score, we evaluate its statistical significance by calculating the number of motifs of equal or better quality expected to occur in random data with the same characteristics. Let the score of the motif of length l in question be denoted by s, and let f(b) be the zero-corrected background frequency of nucleotide (or residue) b in the input sequences, and sim(b1, b2) be the integral score computed for all residue pairs as above. We compute Pl(X), the probability distribution of scores for a motif of length l in N sequences, in the first two steps of the following, and infer the e-value of score s in the last two:\n1. Calculate the exact probability distribution P1(X) for a single column of N random residues. We use the multinomial distribution to compute the probability of observing every combination of bases (or residues) in the column according to the background distribution, and calculate the corresponding SP-score for the column. We then add probabilities for the same scores resulting from different base combinations. To make the computation feasible for the protein alphabet and for large numbers of sequences, we calculate the scores and probabilities in such an order that every new score and probability is computable from the previous one by a local update operation.\n2. Calculate the probability distribution Pl(X) for l random columns by convolution of P1(X)as in [38], where we inductively construct a distribution for i columns based on the distribution for i - 1 columns, Pi-1(X), and the single column distribution P1(X).\n3. For a given score s of interest, we calculate the probability that an l-long pattern has score greater than or equal to s by chance alone. This probability is ∑x\u003e=s Pl(x).\n4. Finally, we compute the total number of possible motifs of length l in the data. If the sequences have lengths L1, ..., LN, then the search space size L = ∏i (Li - l + 1). The expected number of alignments with score at least s by chance alone, or the e-value, is equal to L* ∑x\u003e=s Pl(x).\n\nOverview of approach\nOur basic LP/DEE approach is to: (1) formulate an instance of motif finding as a graph problem (2) apply the DEE techniques described above in the order of increasing complexity so as to prune the graph (3) use mathematical programming to find a solution to the smaller graph problem and (4) evaluate statistical significance.\nWhile applying DEE, if the size of the graph becomes small enough (set at 800 vertices for the described experiments), we submit the appropriate LP to the CPLEX LP solver and, if necessary, to the ILP solver. To reduce the graph to that necessary small size, we apply the DEE variants, running each one of them until either the specified graph size has been reached, or to convergence so that no further pruning is possible. In particular, we first attempt to prune the graph using basic clique-bounds DEE, then we consider tighter bound computations, and lastly we employ graph decomposition in conjunction with the DEE methods.\nIn rare cases the optimality-preserving DEE procedures are unable to prune the graph, and we perform what we call speculative pruning using higher C* values, which do not necessarily correspond to known cliques in graph G. Three outcomes of such pruning are possible: (i) The graph is eliminated completely. This guarantees that a clique of value C* does not exist in G. (ii) The pruning proves once again insufficient to reduce the graph. (iii) The pruning procedure converges to a small graph. We search the space of possible C* values until we find one that produces outcome (iii). To identify such a value we first translate the possible clique scores into their corresponding e-values, and then perform binary search on the e-value exponent. This method converges quickly, typically locating an appropriate C* in fewer than 10 iterations. If the optimal solution for the final reduced graph is better than the C* used in pruning, then it is also optimal for the original graph. Otherwise, the e-value corresponding to C* provides us with a lower bound on the significance of the actual optimal solution.\n\nExtensions for other motif finding frameworks\n\nPhylogenetic footprinting\nAn increasingly common way of finding regulatory sites is to look for them among upstream regions of a set of orthologous genes across species (e.g., [9]). In this case additional data, in the form of the phylogenetic tree relating the species, is available and can be exploited. This is especially important when closely related species are part of the input, and, unweighted, they contribute duplicate information and skew the alignment. We use a phylogenetic tree and branch lengths when calculating the edge weights in the graph, with highly diverged sequence pairs getting larger weights. The precise weighting scheme follows the ideas of weighted progressive alignment [42], in which weights αi are computed for every sequence i. The calculation sums branch lengths along the path from the tree root to the sequence at the leaf, splitting shared branches among the descendant leaves, and thereby reducing the weight for related sequences. In essence, we solve a multiple sequence alignment problem with weighted SP-score using match/mismatch, where the computed weight for a pair of positions in sequences i and j is multiplied by αi × αj. The rest of the algorithm operates as in the basic motif finding case above, employing the same LP formulation and DEE techniques.\n\nSubtle motifs\nAnother widely studied formulation of motif finding is the 'subtle' motifs formulation [17], in which an unknown pattern of a length l is implanted with d modifications into each of the input sequences. The graph version of the problem remains the same except that edges only exist between two vertices that correspond to subsequences whose Hamming distance is at most 2d (since otherwise they cannot both be implanted instances of the same pattern). Edges can either be unweighted, or weighted by the number of mismatches between the corresponding subsequences. Either is easily modeled via slight modification of the ILP given earlier (with variables corresponding to non-existent edges removed, and summations in the edge constraints taken only over existing edges), and the resulting ILP can be used in conjunction with the numerous graph pruning techniques previously developed for this problem (e.g. [17]).\n\nMultiple motifs\nHere we give extensions to address the issue of multiple motifs existing in a set of sequences. Discovery of distinct multiple motifs, such as sets of binding sites for two different transcription factors, can be done iteratively by first locating a single optimal motif, masking it out from the problem instance, and then looking for the next one. We mask the previous motif by deleting its solution vertices from the original graph, and then reapplying the LP/DEE techniques to locate the next optimal solution and its corresponding motif.\nTo identify multiple occurrences of a motif in some of the input sequences, it is possible to iteratively solve several ILPs in order to find multiple near-optimal solutions, corresponding to the best cliques of successively decreasing total weights. At iteration t, we add t - 1 constraints to the ILP formulation so as to exclude all previously discovered solutions:\n∑ u ∈ S k x u ≤ N − 1 for k = 1 , ... , t − 1 , ( 10 ) MathType@MTEF@5@5@+=feaafiart1ev1aaatCvAUfKttLearuWrP9MDH5MBPbIqV92AaeXatLxBI9gBaebbnrfifHhDYfgasaacH8akY=wiFfYdH8Gipec8Eeeu0xXdbba9frFj0=OqFfea0dXdd9vqai=hGuQ8kuc9pgc9s8qqaq=dirpe0xb9q8qiLsFr0=vr0=vr0dc8meaabaqaciaacaGaaeqabaqabeGadaaakeaafaqabeqacaaabaWaaabuaeaacqWG4baEdaWgaaWcbaGaemyDauhabeaakiabgsMiJkabd6eaojabgkHiTiabigdaXaWcbaGaemyDauNaeyicI4Saem4uam1aaSbaaWqaaiabdUgaRbqabaaaleqaniabggHiLdaakeaacqqGMbGzcqqGVbWBcqqGYbGCcqqGGaaicqWGRbWAcqGH9aqpcqaIXaqmcqGGSaalcqGGUaGlcqGGUaGlcqGGUaGlcqGGSaalcqWG0baDcqGHsislcqaIXaqmcqGGSaalaaGaaCzcaiaaxMaadaqadiqaaiabigdaXiabicdaWaGaayjkaiaawMcaaaaa@5202@\nwhere Sk contains the optimal set of vertices found in iteration k. This requires that the new solution differs from all previous ones in at least one graph part. We note that to use this type of constraint for the basic formulation of the motif finding problem, the DEE methods given above have to be modified so as not to eliminate nodes taking part in near-optimal but not necessarily optimal solutions. For the subtle motifs problem, existing DEE methods (e.g., [17]) only eliminate nodes and edges based on whether they can take part in any clique in the graph, and thus constraint 10 can be immediately applied to iteratively find cliques of successively decreasing weight.","divisions":[{"label":"title","span":{"begin":0,"end":7}},{"label":"sec","span":{"begin":9,"end":5012}},{"label":"title","span":{"begin":9,"end":34}},{"label":"p","span":{"begin":35,"end":1643}},{"label":"p","span":{"begin":1644,"end":3768}},{"label":"p","span":{"begin":3769,"end":5012}},{"label":"sec","span":{"begin":5014,"end":8665}},{"label":"title","span":{"begin":5014,"end":5052}},{"label":"p","span":{"begin":5053,"end":5672}},{"label":"p","span":{"begin":5673,"end":7660}},{"label":"p","span":{"begin":7661,"end":8163}},{"label":"p","span":{"begin":8164,"end":8665}},{"label":"sec","span":{"begin":8667,"end":21992}},{"label":"title","span":{"begin":8667,"end":8691}},{"label":"p","span":{"begin":8692,"end":8985}},{"label":"sec","span":{"begin":8987,"end":13780}},{"label":"title","span":{"begin":8987,"end":9010}},{"label":"p","span":{"begin":9011,"end":9314}},{"label":"p","span":{"begin":9315,"end":9512}},{"label":"p","span":{"begin":9513,"end":10111}},{"label":"p","span":{"begin":10112,"end":10304}},{"label":"p","span":{"begin":10305,"end":10826}},{"label":"p","span":{"begin":10827,"end":11197}},{"label":"p","span":{"begin":11198,"end":11403}},{"label":"p","span":{"begin":11404,"end":11955}},{"label":"p","span":{"begin":11956,"end":12115}},{"label":"p","span":{"begin":12116,"end":12980}},{"label":"p","span":{"begin":12981,"end":13655}},{"label":"p","span":{"begin":13656,"end":13780}},{"label":"sec","span":{"begin":13782,"end":20489}},{"label":"title","span":{"begin":13782,"end":13823}},{"label":"p","span":{"begin":13824,"end":14579}},{"label":"p","span":{"begin":14580,"end":15527}},{"label":"p","span":{"begin":15528,"end":16757}},{"label":"p","span":{"begin":16758,"end":16904}},{"label":"p","span":{"begin":16905,"end":17503}},{"label":"p","span":{"begin":17504,"end":18046}},{"label":"p","span":{"begin":18047,"end":18568}},{"label":"p","span":{"begin":18569,"end":19038}},{"label":"p","span":{"begin":19039,"end":19128}},{"label":"p","span":{"begin":19129,"end":20065}},{"label":"p","span":{"begin":20066,"end":20489}},{"label":"sec","span":{"begin":20491,"end":21992}},{"label":"title","span":{"begin":20491,"end":20510}},{"label":"p","span":{"begin":20511,"end":21992}},{"label":"sec","span":{"begin":21994,"end":24081}},{"label":"title","span":{"begin":21994,"end":22018}},{"label":"p","span":{"begin":22019,"end":22683}},{"label":"p","span":{"begin":22684,"end":23354}},{"label":"p","span":{"begin":23355,"end":23614}},{"label":"p","span":{"begin":23615,"end":23789}},{"label":"p","span":{"begin":23790,"end":24081}},{"label":"sec","span":{"begin":24083,"end":26169}},{"label":"title","span":{"begin":24083,"end":24103}},{"label":"p","span":{"begin":24104,"end":24430}},{"label":"p","span":{"begin":24431,"end":25060}},{"label":"p","span":{"begin":25061,"end":26169}},{"label":"title","span":{"begin":26171,"end":26216}},{"label":"sec","span":{"begin":26218,"end":27520}},{"label":"title","span":{"begin":26218,"end":26243}},{"label":"p","span":{"begin":26244,"end":27520}},{"label":"sec","span":{"begin":27522,"end":28448}},{"label":"title","span":{"begin":27522,"end":27535}},{"label":"p","span":{"begin":27536,"end":28448}},{"label":"title","span":{"begin":28450,"end":28465}},{"label":"p","span":{"begin":28466,"end":29007}},{"label":"p","span":{"begin":29008,"end":29376}},{"label":"p","span":{"begin":29377,"end":30053}}],"tracks":[{"project":"2_test","denotations":[{"id":"16916460-1438297-1687079","span":{"begin":4992,"end":4994},"obj":"1438297"},{"id":"16916460-15546935-1687080","span":{"begin":8104,"end":8106},"obj":"15546935"},{"id":"16916460-10743561-1687081","span":{"begin":9309,"end":9311},"obj":"10743561"},{"id":"16916460-7991589-1687082","span":{"begin":23454,"end":23456},"obj":"7991589"},{"id":"16916460-11997340-1687083","span":{"begin":26395,"end":26396},"obj":"11997340"},{"id":"16916460-3118049-1687084","span":{"begin":26920,"end":26922},"obj":"3118049"},{"id":"16916460-10977088-1687085","span":{"begin":27624,"end":27626},"obj":"10977088"},{"id":"16916460-10977088-1687086","span":{"begin":28443,"end":28445},"obj":"10977088"},{"id":"16916460-10977088-1687087","span":{"begin":30521,"end":30523},"obj":"10977088"}],"attributes":[{"subj":"16916460-1438297-1687079","pred":"source","obj":"2_test"},{"subj":"16916460-15546935-1687080","pred":"source","obj":"2_test"},{"subj":"16916460-10743561-1687081","pred":"source","obj":"2_test"},{"subj":"16916460-7991589-1687082","pred":"source","obj":"2_test"},{"subj":"16916460-11997340-1687083","pred":"source","obj":"2_test"},{"subj":"16916460-3118049-1687084","pred":"source","obj":"2_test"},{"subj":"16916460-10977088-1687085","pred":"source","obj":"2_test"},{"subj":"16916460-10977088-1687086","pred":"source","obj":"2_test"},{"subj":"16916460-10977088-1687087","pred":"source","obj":"2_test"}]}],"config":{"attribute types":[{"pred":"source","value type":"selection","values":[{"id":"2_test","color":"#c993ec","default":true}]}]}}