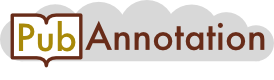
CORD-19:a0ecc9b46addbc1faab491434b5331702f7883b8 8 Projects
Title: A mathematical model for estimating the age-specific transmissibility of a novel 3 coronavirus 4 Running title: Age-specific transmissibility of SARS-CoV-2 5
Abstract
Background: A novel coronavirus named as "SARS-CoV-2" has spread widely in many countries since 38 December 2019, especially in China. This study aimed to quantify the age-specific transmissibility by 39 using a mathematical model.
In December 2019, a series of cases were identified by a novel coronavirus named severe acute 63 respiratory syndrome coronavirus 2 (SARS-CoV-2) was reported by Wuhan City, Hubei Province,
Age-related transmissibility of COVID-19 has become a public health concern. The reported cases 70 at the early stage (before 2 January 2020) of the transmission were higher than 18 years old and 71 mainly were on 25-64 years old [1] . A study with 425 patients indicated that the median age was 59 72 years (range, 15 to 89) and reported different case distributions in four age groups: 0 -14, 15 -44, 45 73 -64, and 65 years [2] . However, there is not enough epidemiological evidence to classify the age 74 groups in transmission. In this study, we added another age classification scenario according to our 75 previous research on influenza that people were divided into five age groups: 0 -5, 6 -14, 15 -24, 25 76 -59, and 60 years [4] , to better understand the transmissibility of the disease for different ages.
There were several studies focusing on mathematical modelling on 3] , calculating the 78 R 0 by using the serial intervals and intrinsic growth rate [2, 5, 6] , or using ordinary differential equations 79 and Markov Chain Monte Carlo methods [3] . We also developed a Bats-Hosts-Reservoir-People (BHRP)
. CC-BY-NC-ND 4.0 International license It is made available under a is the author/funder, who has granted medRxiv a license to display the preprint in perpetuity.
The copyright holder for this preprint . https: //doi.org/10.1101 //doi.org/10. /2020
In this study, based on the RP model which we developed, we built an age-specific susceptible -84 exposedsymptomaticasymptomaticrecoveredreservoir (SEIARW) model.
In this study, people were divided into 4 age groups based on the published literature[1], and 5 age 97 groups based on our previous study [4] . We used the subscript i and j to represent age group 1 to 4 (i ≠ j; 98 1: 0 -14 years; 2: 15 -44 years; 3: 45 -64 years; 4: ≥ 65 years) and 1 to 5 (i ≠ j; 1: 0 -5 years; 2: 6-14 99 years; 3: 15-24 years; 4: 25-59 years; 4: ≥ 60 years), respectively.
Age-specific transmission model
Our previous study showed that the SEIARW model could be adopted to simulate the infectious 102 diseases transmitted from reservoir (such as water or food) to people and from person to person [8, 9] .
In this study, the age-specific SEIARW model was developed based on two transmission routes (form 104 market to person and person to person). In the model, people were divided into five compartments:
. CC-BY-NC-ND 4.0 International license It is made available under a is the author/funder, who has granted medRxiv a license to display the preprint in perpetuity.
(which was not peer-reviewed) The copyright holder for this preprint . https: //doi.org/10.1101 //doi.org/10. /2020 6 susceptible (S), exposed (E), symptomatic (I), asymptomatic (A), recovered (R), and the seafood 106 market were defined as reservoir (W). The definitions of the six compartments were shown in Table 1 .
The model was based on following assumptions: 108 a) Susceptible individuals become infected by contact with infected/asymptomatic people; 109 b) SARS-CoV-2 can transmit within each age group. The transmission rate of age group i and j are 110 β ii and β jj respectively. 111 c) SARS-CoV-2 can be transmitted between different age groups. The transmission rate from age 112 group i to j is β ij and from j to i is β ji . 113 d) Susceptible people will be infected after contact with seafood market, and the infection rate 114 coefficient is β w ; 115 e) All the newborn individuals which were assumed as susceptible were added into age group 1.
Each age group has a natural mortality rate. We set the natural birth rate is br, and the natural mortality 117 rate is dr;
f) The incubation period of exposed person is 1/. The exposed person will become asymptomatic 119 people after a latent period of 1/'. We describe p (0 ≤ p ≤ 1) as the proportion of asymptomatic 120 infection. Exposed individuals will become asymptomatic person A with a daily rate of pE, and become 121 symptomatic person with a daily rate of (1-p)E; 122 g) The transmissibility of A is times (0 ≤ ≤ 1) of that of I. 123
h) The individuals I and A will become recovered person (R) after an infectious period of 1/γ and 124 1/γ'. A part of I would die due to the infection. We assumed that the case fatality rate was f.
. CC-BY-NC-ND 4.0 International license It is made available under a is the author/funder, who has granted medRxiv a license to display the preprint in perpetuity.
The copyright holder for this preprint . https://doi.org/10.1101/2020.03.05.20031849 doi: medRxiv preprint 7 j) SARS-CoV-2 will remove in the market after a given period (the life time of the virus is 1/ε), and 128 the daily decreasing rate of the pathogen is εW.
The flowchart of the model was shown in Figure 1
Since the different dimension of people and the virus, we adopt the following sets to perform the 133 normalization:
136 137 . CC-BY-NC-ND 4.0 International license It is made available under a is the author/funder, who has granted medRxiv a license to display the preprint in perpetuity.
The copyright holder for this preprint . https://doi.org/10.1101/2020.03.05.20031849 doi: medRxiv preprint 8 In the normalization, parameter c refers to relative shedding coefficients of A compared to I. The 138 normalized model is expressed as follows: . CC-BY-NC-ND 4.0 International license It is made available under a is the author/funder, who has granted medRxiv a license to display the preprint in perpetuity.
The copyright holder for this preprint . https://doi.org/10. 1101 /2020 Since there was no data on the proportion of asymptomatic infection of the virus, we simulated the 148 baseline value of proportion of 0.5 (p = 0.5). Since there was no evidence about the transmissibility of 149 asymptomatic infection, we assumed that the transmissibility of asymptomatic infection was 0.5 times 150 that of symptomatic infection ( = 0.5), which was the similar value as influenza [12] . We assumed that 151 the relative shedding rate of A compared to I was 0.5. Thus, c = 0.5. Since the SARS-CoV-2 is an RNA 152 virus, we assumed that it could be died in the environment in a short time, but it could stay for a longer 153 time (10 days) in the unknown hosts in the market. We set ε = 0.1.
There is a mean 5-day delay from symptom onset to detection/hospitalization of a case (the cases 155 detected in Thailand and Japan were hospitalized from 3-7 days after onset, respectively) [13] [14] [15] .
Another study showed that the mean time from illness onset to hospital admission (for treatment and/or 157 isolation) was estimated at 3-4 days without truncation and at 5-9 days when right truncated [11] . As 158 reported by Xu et al [10] , the median time from onset of illness to first hospital admission was 2 (range 159 from 1-4) days. A study including 45 patients diagnosed before January 1 was estimated to have a 160 mean of 5.8 days (95% CI: 4.3-7.5) from illness onset to first medical visit[2]. In our model, we set the 161 infectious period of the cases as 6 days. Therefore, γ = γ' = 0.1667.
According the official report by National Health Commission of the People's Republic of China, we 163 collected the data of daily fatality from January 24 th to 30 th , 2020 [16] . Therefore, we set f as 0.02348
(range: 0.02198-0.03186). In this study, we set the total population as 11,081,000 so that br = 4.266 165 10 -5 and dr = 3.184 10 -5 based on the 2018 Wuhan Statistical Yearbook (Table 2) .
A two-step curve fitting method was adopted to estimate the parameter b W , b ii , b jj , b ij , and b ji . At the 167 first step, we used the mix-age SEIARW model to fit the data and to estimate the parameter b W and 168 mix-age b (named as b p ). At the second step, we used the age-specific SEIARW model to fit the data 169 . CC-BY-NC-ND 4.0 International license It is made available under a is the author/funder, who has granted medRxiv a license to display the preprint in perpetuity.
The copyright holder for this preprint . https://doi.org/10. 1101 /2020 and to estimate the parameter b ii , b jj , b ij , and b ji .
In the model the age-specific secondary attack rate (SAR) was employed to quantify the 172 transmissibility of SARS-CoV-2. They were calculated as follows:
In the equations, SAR ii , SAR ij , SAR ji , and SAR jj refer to the age-specific SAR among age group i, 174 from age group i to j, from age group j to i, and among age group j, respectively.
To quantify the age-specific transmissibility of SARS-CoV-2, we also performed a simulation which 176 was named as "knock-out" simulation in our previous study [17] . In this study, the "knock-out" simulation 177 was defined as cutting off the route of transmission between or within different age groups, and was is the author/funder, who has granted medRxiv a license to display the preprint in perpetuity.
The copyright holder for this preprint , P > 0.999). Thus, the prevalence of COVID-19 in each age group was simulated in Figure 4 .
Based on the four-age-group SEIARW model, the highest transmissibility occurred from age group 208 2 to 3 (SAR 23 = 17.56 per 10 million persons), followed by from age group 3 to 2 (SAR 32 = 10.17 per 10 209 . CC-BY-NC-ND 4.0 International license It is made available under a is the author/funder, who has granted medRxiv a license to display the preprint in perpetuity.
The copyright holder for this preprint . https://doi.org/10.1101/2020.03.05.20031849 doi: medRxiv preprint million persons), from age group 4 to 4 (SAR 44 = 6.99 per 10 million persons), and from age group 3 to 210 4 (SAR 34 = 5.69 per 10 million persons). The lowest transmissibility occurred from age group 1 to 2 211 (SAR 12 = 0.002 per 10 million persons), followed by from age group 3 to 1 (SAR 31 = 0.003 per 10 million 212 persons), from age group 4 to 2 (SAR 42 = 0.52 per 10 million persons), and from age group 1 to 3 213 (SAR 13 = 1.08 per 10 million persons) ( Figure 5-A) .
Based on the five-age-group SEIARW model, the highest transmissibility occurred from age group 215 4 to 5 (SAR 45 = 12.40 per 10 million persons), followed by from age group 5 to 4 (SAR 54 = 6.61 per 10 216 million persons), from age group 4 to 4 (SAR 44 = 5.08 per 10 million persons), and from age group 2 to 217 1 (SAR 21 = 4.90 per 10 million persons). The lowest transmissibility occurred from age group 3 to 4 218 (SAR 34 = 0.0002 per 10 million persons), followed by from age group 1 to 1 (SAR 11 = 0.22 per 10 million 219 persons), from age group 1 to 3 (SAR 13 = 1.54 per 10 million persons), and from age group 1 to 2 220 (SAR 12 = 1.59 per 10 million persons) ( Figure 5-B) .
The results of the "knock-out" simulation showed that, based on the four-age-group SEIARW
The results of sensitivity analysis showed that the models were slightly sensitive to parameters p, 229 , and γ but not sensitive to parameters , c, ε, f, ', and γ' (Figures 7 -8) . 230 231 . CC-BY-NC-ND 4.0 International license It is made available under a is the author/funder, who has granted medRxiv a license to display the preprint in perpetuity.
The copyright holder for this preprint . https: //doi.org/10.1101 //doi.org/10. /2020 13
This is the first study to develop an age-specific SEIARW model to quantify the transmissibility of 233 COVID-19 among different age groups. The results showed that our model fitted the reported data well, 234 thus has the capability of estimating or predicting? the age-specific transmissibility of the virus.
Based on the four-age-group SEIARW model, the highest transmissibility occurred between the 236 age groups 15 -44 years and 45 -64 years, among those 65 years, or from 45 -64 years to 65 237 years. The lowest transmissibility occurred from age group 0-14 years to 15 -44 years, or from 45 -64 238 years to 14 years. Based on the five-age-group SEIARW model, the highest transmissibility occurred 239 between age group 25 -59 years and 60 years, or among 25 -59 years. The lowest transmissibility 240 occurred from age group 15 -24 years to 25 -59 years, or from age group 0-5 years to 6-14 years, or 241 to 15-24 years. The "knock-out" simulation had the similar results. We concluded that the virus was 242 more likely to transmit among older population.
These results revealed that SARS-CoV-2 has high transmissibility among adult older than 25 years 244 old or elder people, but has low transmissibility among children or people younger than 14 years.
These results were similar to the age-specific transmissibility of influenza A (H1N1)[4]. The age-specific 246 control and prevention interventions are needed.
The reasons for the difference of age-specific transmissibility remain unclear. It might be related to 248 the different contact characteristics among different age groups. Adults were more likely to work outside 249 and came into contact with different individuals in work places, buses, subways, or even airplanes.
However, children or younger people could stay at home during the outbreak, and they were less likely 251 to get infected, or if they were infected, it was mostly from adults or elder people in the same family.
Our findings were based on the parameter estimation and the data from literatures. It is known that 253 . CC-BY-NC-ND 4.0 International license It is made available under a is the author/funder, who has granted medRxiv a license to display the preprint in perpetuity.
The copyright holder for this preprint . https://doi.org/10. 1101 /2020 14 the asymptomatic infection of COVID-19 exists. As reported by Bai et al [25] , 1 of 6 cases was 254 asymptomatic infected, with the proportion of asymptomatic of 0.17. As reported by Pan et al [26] , 2 of 3 255 cases was asymptomatic infected, with the proportion of asymptomatic of 0.67.There was a research 256 showed that the asymptomatic infection would shed SARS-CoV-2 for 5 days [27] . However, there was 257 not enough evidence to provide the clear epidemiological estimates of the parameters p, , ', and γ' 258 which were related to asymptomatic characteristics. Although there was clinical evidence to calculate 259 the parameters related to symptomatic cases such as incubation period, fatality rate, and duration from 260 symptoms onset to diagnosis[1, 2], more epidemiological data are needed to explore the parameters.
However, we performed a sensitivity analysis of all the nine parameters from the literatures, and we 262 found that the models was slightly sensitive to parameters p, , and γ but not sensitive to parameters , 263 c, ε, f, ', and γ'. Therefore, our results might be affected slightly by the parameter estimation. 264
Since we could not obtain the first-hand data, the results of our findings might have some 265 uncertainties. However, our study aimed to develop and provide an age-specific transmission model for 266 the public health department who has the big data to investigate the age-specific transmissibility in 267 real world scenarios. CC-BY-NC-ND 4.0 International license It is made available under a is the author/funder, who has granted medRxiv a license to display the preprint in perpetuity.
The copyright holder for this preprint . https: //doi.org/10.1101 //doi.org/10. /2020
The authors declare that they have no competing interests.
Consent for publication 297 . CC-BY-NC-ND 4.0 International license It is made available under a is the author/funder, who has granted medRxiv a license to display the preprint in perpetuity.
The copyright holder for this preprint CC-BY-NC-ND 4.0 International license It is made available under a is the author/funder, who has granted medRxiv a license to display the preprint in perpetuity. CC-BY-NC-ND 4.0 International license It is made available under a is the author/funder, who has granted medRxiv a license to display the preprint in perpetuity.
The copyright holder for this preprint . CC-BY-NC-ND 4.0 International license It is made available under a is the author/funder, who has granted medRxiv a license to display the preprint in perpetuity.
(which was not peer-reviewed) The copyright holder for this preprint . https://doi.org/10. 1101 /2020
|
Annnotations
- Denotations: 0
- Blocks: 0
- Relations: 0