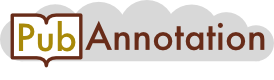
PubMed:32982615
Annnotations
LitCovid-PD-FMA-UBERON
Id | Subject | Object | Predicate | Lexical cue | fma_id |
---|---|---|---|---|---|
T1 | 218-232 | Body_part | denotes | neural network | http://purl.org/sig/ont/fma/fma74616 |
T2 | 647-652 | Body_part | denotes | chest | http://purl.org/sig/ont/fma/fma9576 |
T3 | 1353-1355 | Body_part | denotes | v3 | http://purl.org/sig/ont/fma/fma13442 |
LitCovid-PD-UBERON
Id | Subject | Object | Predicate | Lexical cue | uberon_id |
---|---|---|---|---|---|
T1 | 647-652 | Body_part | denotes | chest | http://purl.obolibrary.org/obo/UBERON_0001443 |
LitCovid-PD-MONDO
Id | Subject | Object | Predicate | Lexical cue | mondo_id |
---|---|---|---|---|---|
T1 | 61-69 | Disease | denotes | COVID-19 | http://purl.obolibrary.org/obo/MONDO_0100096 |
T2 | 177-185 | Disease | denotes | COVID-19 | http://purl.obolibrary.org/obo/MONDO_0100096 |
T3 | 630-638 | Disease | denotes | COVID-19 | http://purl.obolibrary.org/obo/MONDO_0100096 |
T4 | 921-924 | Disease | denotes | SSD | http://purl.obolibrary.org/obo/MONDO_0012038 |
T5 | 1028-1031 | Disease | denotes | SSD | http://purl.obolibrary.org/obo/MONDO_0012038 |
T6 | 1115-1123 | Disease | denotes | COVID-19 | http://purl.obolibrary.org/obo/MONDO_0100096 |
T7 | 1152-1160 | Disease | denotes | COVID-19 | http://purl.obolibrary.org/obo/MONDO_0100096 |
T8 | 1173-1176 | Disease | denotes | SSD | http://purl.obolibrary.org/obo/MONDO_0012038 |
T9 | 1464-1472 | Disease | denotes | COVID-19 | http://purl.obolibrary.org/obo/MONDO_0100096 |
T10 | 1611-1619 | Disease | denotes | COVID-19 | http://purl.obolibrary.org/obo/MONDO_0100096 |
T11 | 1713-1721 | Disease | denotes | COVID-19 | http://purl.obolibrary.org/obo/MONDO_0100096 |
LitCovid-PD-CLO
Id | Subject | Object | Predicate | Lexical cue |
---|---|---|---|---|
T1 | 137-138 | http://purl.obolibrary.org/obo/CLO_0001020 | denotes | a |
T2 | 195-196 | http://purl.obolibrary.org/obo/CLO_0001020 | denotes | a |
T3 | 591-592 | http://purl.obolibrary.org/obo/CLO_0001020 | denotes | a |
T4 | 647-652 | http://www.ebi.ac.uk/efo/EFO_0000965 | denotes | chest |
T5 | 751-755 | http://purl.obolibrary.org/obo/UBERON_0000473 | denotes | test |
T6 | 774-778 | http://purl.obolibrary.org/obo/UBERON_0000473 | denotes | test |
T7 | 1229-1231 | http://purl.obolibrary.org/obo/CLO_0001527 | denotes | 94 |
T8 | 1240-1244 | http://purl.obolibrary.org/obo/UBERON_0000473 | denotes | test |
T9 | 1317-1318 | http://purl.obolibrary.org/obo/CLO_0001020 | denotes | a |
T10 | 1353-1355 | http://purl.obolibrary.org/obo/CLO_0050428 | denotes | v3 |
T11 | 1564-1568 | http://purl.obolibrary.org/obo/UBERON_0000473 | denotes | test |
LitCovid-PD-GO-BP
Id | Subject | Object | Predicate | Lexical cue |
---|---|---|---|---|
T1 | 18-26 | http://purl.obolibrary.org/obo/GO_0007612 | denotes | learning |
LitCovid-sentences
Id | Subject | Object | Predicate | Lexical cue |
---|---|---|---|---|
T1 | 0-121 | Sentence | denotes | An optimized deep learning architecture for the diagnosis of COVID-19 disease based on gravitational search optimization. |
T2 | 122-296 | Sentence | denotes | In this paper, a novel approach called GSA-DenseNet121-COVID-19 based on a hybrid convolutional neural network (CNN) architecture is proposed using an optimization algorithm. |
T3 | 297-451 | Sentence | denotes | The CNN architecture that was used is called DenseNet121, and the optimization algorithm that was used is called the gravitational search algorithm (GSA). |
T4 | 452-553 | Sentence | denotes | The GSA is used to determine the best values for the hyperparameters of the DenseNet121 architecture. |
T5 | 554-666 | Sentence | denotes | To help this architecture to achieve a high level of accuracy in diagnosing COVID-19 through chest x-ray images. |
T6 | 667-770 | Sentence | denotes | The obtained results showed that the proposed approach could classify 98.38% of the test set correctly. |
T7 | 771-872 | Sentence | denotes | To test the efficacy of the GSA in setting the optimum values for the hyperparameters of DenseNet121. |
T8 | 873-1033 | Sentence | denotes | The GSA was compared to another approach called SSD-DenseNet121, which depends on the DenseNet121 and the optimization algorithm called social ski driver (SSD). |
T9 | 1034-1124 | Sentence | denotes | The comparison results demonstrated the efficacy of the proposed GSA-DenseNet121-COVID-19. |
T10 | 1125-1249 | Sentence | denotes | As it was able to diagnose COVID-19 better than SSD-DenseNet121 as the second was able to diagnose only 94% of the test set. |
T11 | 1250-1408 | Sentence | denotes | The proposed approach was also compared to another method based on a CNN architecture called Inception-v3 and manual search to quantify hyperparameter values. |
T12 | 1409-1581 | Sentence | denotes | The comparison results showed that the GSA-DenseNet121-COVID-19 was able to beat the comparison method, as the second was able to classify only 95% of the test set samples. |
T13 | 1582-1661 | Sentence | denotes | The proposed GSA-DenseNet121-COVID-19 was also compared with some related work. |
T14 | 1662-1742 | Sentence | denotes | The comparison results showed that GSA-DenseNet121-COVID-19 is very competitive. |
LitCovid-PubTator
Id | Subject | Object | Predicate | Lexical cue | tao:has_database_id |
---|---|---|---|---|---|
1 | 61-69 | Disease | denotes | COVID-19 | MESH:C000657245 |
13 | 177-185 | Disease | denotes | COVID-19 | MESH:C000657245 |
14 | 630-638 | Disease | denotes | COVID-19 | MESH:C000657245 |
15 | 799-802 | Chemical | denotes | GSA | |
16 | 860-871 | Chemical | denotes | DenseNet121 | |
17 | 1099-1102 | Chemical | denotes | GSA | |
18 | 1115-1123 | Disease | denotes | COVID-19 | MESH:C000657245 |
19 | 1152-1160 | Disease | denotes | COVID-19 | MESH:C000657245 |
20 | 1464-1472 | Disease | denotes | COVID-19 | MESH:C000657245 |
21 | 1611-1619 | Disease | denotes | COVID-19 | MESH:C000657245 |
22 | 1697-1700 | Chemical | denotes | GSA | |
23 | 1713-1721 | Disease | denotes | COVID-19 | MESH:C000657245 |