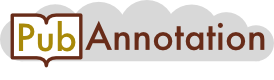
PMC:7799291 / 47263-49376
Annnotations
{"target":"http://pubannotation.org/docs/sourcedb/PMC/sourceid/7799291","sourcedb":"PMC","sourceid":"7799291","source_url":"https://www.ncbi.nlm.nih.gov/pmc/7799291","text":"Kaggle CORD-19 research challenge\nFor the Kaggle challenge (https://www.kaggle.com/allen-institute-for-ai/CORD-19-research-challenge), participants are asked to extract answers to key COVID-19 scientific questions from the documents in the CORD-19 corpus. Round 1 of the challenge began with nine open-ended questions on COVID-19, seeking information on transmission, diagnostics and treatment. Kaggle partnered with medical experts to identify the most useful contributions from the more than 500 submissions it received.\nRound 2 was designed based on this feedback and focuses on the task of table completion. Medical experts define a unique tabular schema for each question from Round 1, and participants are asked to complete the table by extracting information from CORD-19 documents. For example, extractions for risk factors should include disease severity and fatality metrics, while extractions for incubation should include time ranges. Sufficient knowledge of COVID-19 is necessary to define these schema and to understand which fields are important to include (and exclude). An example submission is described in [58]. The table completion task is somewhat analogous to extracting evidence for a systematic review, which we discuss in greater detail in Section on “Systematic review automation”.\nUpon the completion of the Kaggle challenge, the community has moved towards repurposing the submitted contributions. Among the contributions are output review tables from Round 2, which provide a useful overview of research findings(https://www.kaggle.com/covid-19-contributions). Table results have been used to quickly bootstrap QA datasets [48, 87], which will be useful for training COVID-19 QA systems. Early COVID-19 QA systems rely on either existing biomedical QA datasets that do not contain questions specific to COVID-19 (e.g. BioASQ) or had to bootstrap their own COVID-19 training data through expert annotation, which is expensive and results in small-scale data. These new QA datasets and shared tasks like EPIC-QA (Section 5.3) aim to address the lack of domain-specific QA training data.","divisions":[{"label":"title","span":{"begin":0,"end":33}},{"label":"p","span":{"begin":34,"end":522}},{"label":"p","span":{"begin":523,"end":1307}}],"tracks":[]}