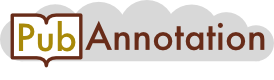
PMC:7799291 / 1733-6821
Annnotations
{"target":"http://pubannotation.org/docs/sourcedb/PMC/sourceid/7799291","sourcedb":"PMC","sourceid":"7799291","source_url":"https://www.ncbi.nlm.nih.gov/pmc/7799291","text":"Introduction\nSince the discovery of the novel coronavirus SARS-CoV-2 [4, 107] toward the tail end of 2019, the disease caused by the virus, COVID-19, has swept through the globe and drastically altered all aspects of our lives. Governments and researchers, academic and industry alike, have coalesced around the common goals of healthcare resource management, social policy determination, prevention and treatment and vaccine development. The scientific community, correspondingly, has responded rapidly to the pandemic. Scientific output on the subject of COVID-19 and coronaviruses has emerged at an unprecedented rate, placing significant strain upon clinicians, researchers and others who must keep up-to-date on this new literature. By different metrics, somewhere upwards of 55–100 000 papers and preprints on COVID-19 have been released in 2020 thus far (please refer to https://www.ncbi.nlm.nih.gov/pmc/about/covid-19/, https://www.semanticscholar.org/cord19 and https://covid19primer.com/dashboard for possible paper counts; estimate made on 12 September 2020), accelerating to the current rates of many hundreds of new articles a day. Even on the low-end of this estimate, conventional reading methods are challenged and we must rely on automated text mining approaches to address this tidal wave of research output.\nOne of the major application areas of biomedical text mining is managing information overload [3, 19, 40, 116]. As per [19], text mining focuses on solving specific problems such as retrieving relevant documents or extracting nuggets of information from those documents. In the process of addressing these problems, text mining systems may use techniques for information retrieval, information extraction, text classification, etc. and leverage methods from related fields such as natural language processing and knowledge base (KB) construction. While there lacks consensus on the precise relationships between these various tasks and/or fields of study [3, 19, 40, 116], in this review, we focus on approaches for addressing information overload and adopt ‘text mining’ as a general term to refer to methods from the aforementioned areas.\nIn response to the large volume of literature published on COVID-19, the computing community has introduced text mining corpora, modeling resources, systems and community-wide shared tasks specific to COVID-19 to address the mounting challenge. Corpora are collections of documents, preprocessed to extract machine-readable text, that are used for text mining; in this case, we focus on corpora-containing scientific articles. Modeling resources can be incorporated by text mining practitioners into production systems and consist of things such as text embeddings, data annotations, pretrained language models, knowledge graphs and more. Systems are applications that incorporate text mining models and user interfaces to provide functionalities such as the ability to search, discover or visualize article content. Shared tasks are community competitions that promote concentrated work on specific scientific problems.\nFigure 1 illustrates how a text mining practitioner might approach developing a system to address information overload for researchers. Unfortunately, the process of corpus construction, data enrichment, model development, evaluation and eventual deployment can take months or years, which is unacceptable during a public health crisis. In the current situation, public corpora help to remove the burden of corpus creation, while shared community annotations contribute to addressing the challenges of data enrichment and annotation. Finally, shared tasks help to promote faster iteration of this process by centralizing evaluation and also serving as a source of annotated data.\nFig. 1 A typical workflow for creating a literature text mining system may consist of corpus construction, data enrichment, model development and evaluation. A text mining practitioner (e.g. engineer, researcher, enthusiast, etc.) may be responsible for each of these steps in the gray box, whether by identifying and adapting existing datasets and models or by creating their own. For COVID-19, centralization of parts of this workflow have helped to reduce the burden around some of these steps. In this review, we summarize the corpora (Section on “Text mining corpora”), modeling resources (Section on “Text mining modeling resources”), systems (Section on “Text mining systems”) and shared tasks (Section on “Shared tasks”) that have been created/implemented to support text mining over the COVID-19 literature. We note standout systems that either provide strong performance on fundamental tasks such as search or question answering (QA) or provide novel functionality such as multi-document summarization or linking between articles and clinical trials. We also discuss strategies for building performant and useful systems, specifically advocating for systems that facilitate the production of systematic reviews, or those that directly address the needs of clinicians, researchers and public health officials.","divisions":[{"label":"title","span":{"begin":0,"end":12}},{"label":"p","span":{"begin":13,"end":1326}},{"label":"p","span":{"begin":1327,"end":2167}},{"label":"p","span":{"begin":2168,"end":3088}},{"label":"p","span":{"begin":3089,"end":3768}},{"label":"figure","span":{"begin":3769,"end":4267}},{"label":"label","span":{"begin":3769,"end":3775}},{"label":"caption","span":{"begin":3777,"end":4267}},{"label":"p","span":{"begin":3777,"end":4267}}],"tracks":[]}