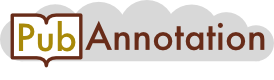
PMC:7796058 / 57422-58756
Annnotations
{"target":"https://pubannotation.org/docs/sourcedb/PMC/sourceid/7796058","sourcedb":"PMC","sourceid":"7796058","source_url":"https://www.ncbi.nlm.nih.gov/pmc/7796058","text":"Convolutional neural network (CNN) architectures use multiple blocks of successive convolution and pooling operations for feature learning and down sampling along the time and feature dimensions, respectively [71]. The VGG16 is a pre-trained CNN [72] used as a base model for transfer learning (Table 6) [73]. VGG16 is a famous CNN architecture that uses multiple stacks of small kernel filters (3 by 3) instead of the shallow architecture of two or three layers with large kernel filters [74]. Using multiple stacks of small kernel filters increases the network’s depth, which results in improving complex feature learning while decreasing computation costs. VGG16 architecture includes 16 convolutional and three fully connected layers. Audio-based risky behavior detection is based on complex features and distinguishable behaviors (e.g., coughing, sneezing, background noise), which requires a deeper CNN model than shallow architecture (i.e., two or three-layer architecture) offers [75]. VGG16 has been adopted for audio event detection and demonstrated significant literature results [71]. The feature maps were flattened to obtain the fully connected layer after the last convolutional layer. For most CNN-based architectures, only the last convolutional layer activations are connected to the final classification layer [76].","tracks":[{"project":"LitCovid-PubTator","denotations":[{"id":"411","span":{"begin":842,"end":850},"obj":"Disease"}],"attributes":[{"id":"A411","pred":"tao:has_database_id","subj":"411","obj":"MESH:D003371"},{"subj":"411","pred":"source","obj":"LitCovid-PubTator"}],"namespaces":[{"prefix":"Tax","uri":"https://www.ncbi.nlm.nih.gov/taxonomy/"},{"prefix":"MESH","uri":"https://id.nlm.nih.gov/mesh/"},{"prefix":"Gene","uri":"https://www.ncbi.nlm.nih.gov/gene/"},{"prefix":"CVCL","uri":"https://web.expasy.org/cellosaurus/CVCL_"}]},{"project":"LitCovid-PD-HP","denotations":[{"id":"T12","span":{"begin":842,"end":850},"obj":"Phenotype"}],"attributes":[{"id":"A12","pred":"hp_id","subj":"T12","obj":"http://purl.obolibrary.org/obo/HP_0012735"},{"subj":"T12","pred":"source","obj":"LitCovid-PD-HP"}]},{"project":"LitCovid-sentences","denotations":[{"id":"T418","span":{"begin":0,"end":214},"obj":"Sentence"},{"id":"T419","span":{"begin":215,"end":309},"obj":"Sentence"},{"id":"T420","span":{"begin":310,"end":494},"obj":"Sentence"},{"id":"T421","span":{"begin":495,"end":659},"obj":"Sentence"},{"id":"T422","span":{"begin":660,"end":738},"obj":"Sentence"},{"id":"T423","span":{"begin":739,"end":993},"obj":"Sentence"},{"id":"T424","span":{"begin":994,"end":1096},"obj":"Sentence"},{"id":"T425","span":{"begin":1097,"end":1200},"obj":"Sentence"},{"id":"T426","span":{"begin":1201,"end":1334},"obj":"Sentence"}],"namespaces":[{"prefix":"_base","uri":"http://pubannotation.org/ontology/tao.owl#"}],"attributes":[{"subj":"T418","pred":"source","obj":"LitCovid-sentences"},{"subj":"T419","pred":"source","obj":"LitCovid-sentences"},{"subj":"T420","pred":"source","obj":"LitCovid-sentences"},{"subj":"T421","pred":"source","obj":"LitCovid-sentences"},{"subj":"T422","pred":"source","obj":"LitCovid-sentences"},{"subj":"T423","pred":"source","obj":"LitCovid-sentences"},{"subj":"T424","pred":"source","obj":"LitCovid-sentences"},{"subj":"T425","pred":"source","obj":"LitCovid-sentences"},{"subj":"T426","pred":"source","obj":"LitCovid-sentences"}]}],"config":{"attribute types":[{"pred":"source","value type":"selection","values":[{"id":"LitCovid-PubTator","color":"#ec939b","default":true},{"id":"LitCovid-PD-HP","color":"#93b5ec"},{"id":"LitCovid-sentences","color":"#cfec93"}]}]}}