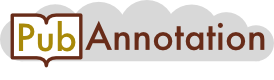
PMC:7796041 / 4030-10186
Annnotations
LitCovid-PubTator
2. Methods
2.1. Patient Selection
All patients with COVID-19 who had been hospitalized at the participating centers were included in this registry from 5 March to 17 June 2020. Only patients with a complete documentation of the clinical stay (i.e., discharged from the respective center or deceased) were considered. Further inclusion criteria were a confirmation of SARS-CoV-2 infection by polymerase chain reaction testing of a nasopharyngeal sample and a duration of hospital stay for ≥ 24 h. In total, 166 patients with were included in this registry. In the majority of patients, COVID-19 was the reason for hospitalization. A subgroup of nine patients had been admitted for different indications and SARS-CoV-2-infection was diagnosed and treated in the course of the hospital stay. The study was conducted in accordance with the Declaration of Helsinki and approved by the ethics committee of the University of Heidelberg (study registration number at the ethics committee: S-281/2020). Due to the retrospective, non-interventional nature of the study based solely on data generated and documented during clinical routine processes, informed and written consent was not required in accordance with the statement of the local ethics committee.
2.2. Data Collection
All available clinical records including physicians’ and nurses’ written reports, diagnostic test results, telemetry logs and electronic patient files were used for systematic data collection. Demographic and clinical baseline parameters, comorbidities, biomarkers, medical therapy as well as information on endpoints regarding the clinical course of disease were extracted. Clinically relevant arrhythmias previously diagnosed or incident during hospitalization were recorded and classified according to arrhythmia diagnosis. Electrocardiograms (ECGs) conducted at admission to hospital were evaluated regarding QRS and QTc duration, if available. If ECG documentation of the arrhythmia was available in the patient file, the diagnosis was confirmed by electrophysiology experts of the study group. With respect to biomarker analyses, peak values of high-sensitive troponin T (hsTnT), N-terminal pro-B-type natriuretic peptide (NTproBNP), lactate dehydrogenase (LDH), C-reactive protein (CRP) and interleukin-6 (IL-6) were recorded, if assessed during hospitalization. Data on body mass index (BMI) or presence of obesity were available in 138 patients. Obesity was defined as BMI > 25 kg/m2 for the purpose of this study.
2.3. Statistical Methods
The patient cohort was described using summary measures of the empirical distribution. Continuous variables are reported as median (with inter-quartile range, 25th percentile = P25; 75th percentile = P75) or mean ± standard deviation (SD). The t-test or Mann-Whitney-Wilcoxon-test were applied for between-group comparisons. Dichotomous variables are presented as absolute and relative frequencies and were compared applying the Fisher Boschloo-test from the R package “Exact” [11].
For the purpose of selecting variables with predictive impact on the incidence of arrhythmia, the variables sex, age, hypertension, cardiovascular disease, hydroxychloroquine, and combined therapy with hydroxychloroquine and azithromycin were initially considered in terms of variable selection. First, regularized logistic regression using the elastic net penalty implemented in the package “glmnet” was computed [12,13]. The hyperparameters α (elastic net mixing parameter) and β (shrinkage parameter) were tuned conducting 5-fold cross-validation (CV) and a grid search. Subsequently, multiple logistic regression modeling was conducted only incorporating the selected variables, to estimate the odds ratios (ORs) and their 95% confidence intervals (CI). The area under the curve (AUC) value was computed applying the receiver operating characteristics (ROC) curve to evaluate the model using the package “pROC” [14]. To prevent overestimation of the model’s performance measure, the AUC-value was calculated applying 5-fold CV. During 5-fold CV, each patient is part of the training set for four times and is assigned exactly once to the testing set. Hence, in each step a model is fitted based on 80% of the data whereas a probability of the remaining 20% of the patients is estimated with respect to the incidence of arrhythmia.
Information on left ventricular ejection fraction (LVEF) was only available in 44 patients. To account for a potential influence of LVEF on the development of cardiac arrhythmia, we performed attempts to impute the missing data (Supplementary Materials). Due to a high number of missing values, LVEF was omitted from further analyses to ensure reliability of the data.
To evaluate the impact of biomarkers on the incidence of arrhythmia, univariate logistic regression modeling was performed. The AUC-values und the Youden index for identifying the optimal cut-off value were computed for each biomarker, respectively [15]. Confidence intervals of the AUC-values were calculated according to DeLong [16].
To assess the prognostic impact of arrhythmia on clinical outcomes univariate and multiple regression modeling was performed. To preserve the validity of multiple regression modeling in the light of the limited number of patients, the models were adjusted for a maximum of two additional covariates. Age and cardiovascular disease were chosen due to their clinical significance regarding outcome in COVID-19 shown by previous studies [2,17].
Due to the high proportion of newly diagnosed atrial fibrillation (AF), we conducted a subgroup analysis comparing patients with incident AF to patients who neither had a history of AF nor displayed AF in the course of the hospitalization. Due to the small sample size in the subgroup of patients with incident AF, only descriptive analyses were performed.
Due to the exploratory character of this analysis, the p-values are interpreted only in a descriptive sense and no adjustment for multiple testing was applied [18]. p-values < 0.05 were denoted as statistically significant. The statistical analysis was performed using R version 4.0.2. [19] and SPSS version 25.
LitCovid-PD-HP
2. Methods
2.1. Patient Selection
All patients with COVID-19 who had been hospitalized at the participating centers were included in this registry from 5 March to 17 June 2020. Only patients with a complete documentation of the clinical stay (i.e., discharged from the respective center or deceased) were considered. Further inclusion criteria were a confirmation of SARS-CoV-2 infection by polymerase chain reaction testing of a nasopharyngeal sample and a duration of hospital stay for ≥ 24 h. In total, 166 patients with were included in this registry. In the majority of patients, COVID-19 was the reason for hospitalization. A subgroup of nine patients had been admitted for different indications and SARS-CoV-2-infection was diagnosed and treated in the course of the hospital stay. The study was conducted in accordance with the Declaration of Helsinki and approved by the ethics committee of the University of Heidelberg (study registration number at the ethics committee: S-281/2020). Due to the retrospective, non-interventional nature of the study based solely on data generated and documented during clinical routine processes, informed and written consent was not required in accordance with the statement of the local ethics committee.
2.2. Data Collection
All available clinical records including physicians’ and nurses’ written reports, diagnostic test results, telemetry logs and electronic patient files were used for systematic data collection. Demographic and clinical baseline parameters, comorbidities, biomarkers, medical therapy as well as information on endpoints regarding the clinical course of disease were extracted. Clinically relevant arrhythmias previously diagnosed or incident during hospitalization were recorded and classified according to arrhythmia diagnosis. Electrocardiograms (ECGs) conducted at admission to hospital were evaluated regarding QRS and QTc duration, if available. If ECG documentation of the arrhythmia was available in the patient file, the diagnosis was confirmed by electrophysiology experts of the study group. With respect to biomarker analyses, peak values of high-sensitive troponin T (hsTnT), N-terminal pro-B-type natriuretic peptide (NTproBNP), lactate dehydrogenase (LDH), C-reactive protein (CRP) and interleukin-6 (IL-6) were recorded, if assessed during hospitalization. Data on body mass index (BMI) or presence of obesity were available in 138 patients. Obesity was defined as BMI > 25 kg/m2 for the purpose of this study.
2.3. Statistical Methods
The patient cohort was described using summary measures of the empirical distribution. Continuous variables are reported as median (with inter-quartile range, 25th percentile = P25; 75th percentile = P75) or mean ± standard deviation (SD). The t-test or Mann-Whitney-Wilcoxon-test were applied for between-group comparisons. Dichotomous variables are presented as absolute and relative frequencies and were compared applying the Fisher Boschloo-test from the R package “Exact” [11].
For the purpose of selecting variables with predictive impact on the incidence of arrhythmia, the variables sex, age, hypertension, cardiovascular disease, hydroxychloroquine, and combined therapy with hydroxychloroquine and azithromycin were initially considered in terms of variable selection. First, regularized logistic regression using the elastic net penalty implemented in the package “glmnet” was computed [12,13]. The hyperparameters α (elastic net mixing parameter) and β (shrinkage parameter) were tuned conducting 5-fold cross-validation (CV) and a grid search. Subsequently, multiple logistic regression modeling was conducted only incorporating the selected variables, to estimate the odds ratios (ORs) and their 95% confidence intervals (CI). The area under the curve (AUC) value was computed applying the receiver operating characteristics (ROC) curve to evaluate the model using the package “pROC” [14]. To prevent overestimation of the model’s performance measure, the AUC-value was calculated applying 5-fold CV. During 5-fold CV, each patient is part of the training set for four times and is assigned exactly once to the testing set. Hence, in each step a model is fitted based on 80% of the data whereas a probability of the remaining 20% of the patients is estimated with respect to the incidence of arrhythmia.
Information on left ventricular ejection fraction (LVEF) was only available in 44 patients. To account for a potential influence of LVEF on the development of cardiac arrhythmia, we performed attempts to impute the missing data (Supplementary Materials). Due to a high number of missing values, LVEF was omitted from further analyses to ensure reliability of the data.
To evaluate the impact of biomarkers on the incidence of arrhythmia, univariate logistic regression modeling was performed. The AUC-values und the Youden index for identifying the optimal cut-off value were computed for each biomarker, respectively [15]. Confidence intervals of the AUC-values were calculated according to DeLong [16].
To assess the prognostic impact of arrhythmia on clinical outcomes univariate and multiple regression modeling was performed. To preserve the validity of multiple regression modeling in the light of the limited number of patients, the models were adjusted for a maximum of two additional covariates. Age and cardiovascular disease were chosen due to their clinical significance regarding outcome in COVID-19 shown by previous studies [2,17].
Due to the high proportion of newly diagnosed atrial fibrillation (AF), we conducted a subgroup analysis comparing patients with incident AF to patients who neither had a history of AF nor displayed AF in the course of the hospitalization. Due to the small sample size in the subgroup of patients with incident AF, only descriptive analyses were performed.
Due to the exploratory character of this analysis, the p-values are interpreted only in a descriptive sense and no adjustment for multiple testing was applied [18]. p-values < 0.05 were denoted as statistically significant. The statistical analysis was performed using R version 4.0.2. [19] and SPSS version 25.
LitCovid-sentences
2. Methods
2.1. Patient Selection
All patients with COVID-19 who had been hospitalized at the participating centers were included in this registry from 5 March to 17 June 2020. Only patients with a complete documentation of the clinical stay (i.e., discharged from the respective center or deceased) were considered. Further inclusion criteria were a confirmation of SARS-CoV-2 infection by polymerase chain reaction testing of a nasopharyngeal sample and a duration of hospital stay for ≥ 24 h. In total, 166 patients with were included in this registry. In the majority of patients, COVID-19 was the reason for hospitalization. A subgroup of nine patients had been admitted for different indications and SARS-CoV-2-infection was diagnosed and treated in the course of the hospital stay. The study was conducted in accordance with the Declaration of Helsinki and approved by the ethics committee of the University of Heidelberg (study registration number at the ethics committee: S-281/2020). Due to the retrospective, non-interventional nature of the study based solely on data generated and documented during clinical routine processes, informed and written consent was not required in accordance with the statement of the local ethics committee.
2.2. Data Collection
All available clinical records including physicians’ and nurses’ written reports, diagnostic test results, telemetry logs and electronic patient files were used for systematic data collection. Demographic and clinical baseline parameters, comorbidities, biomarkers, medical therapy as well as information on endpoints regarding the clinical course of disease were extracted. Clinically relevant arrhythmias previously diagnosed or incident during hospitalization were recorded and classified according to arrhythmia diagnosis. Electrocardiograms (ECGs) conducted at admission to hospital were evaluated regarding QRS and QTc duration, if available. If ECG documentation of the arrhythmia was available in the patient file, the diagnosis was confirmed by electrophysiology experts of the study group. With respect to biomarker analyses, peak values of high-sensitive troponin T (hsTnT), N-terminal pro-B-type natriuretic peptide (NTproBNP), lactate dehydrogenase (LDH), C-reactive protein (CRP) and interleukin-6 (IL-6) were recorded, if assessed during hospitalization. Data on body mass index (BMI) or presence of obesity were available in 138 patients. Obesity was defined as BMI > 25 kg/m2 for the purpose of this study.
2.3. Statistical Methods
The patient cohort was described using summary measures of the empirical distribution. Continuous variables are reported as median (with inter-quartile range, 25th percentile = P25; 75th percentile = P75) or mean ± standard deviation (SD). The t-test or Mann-Whitney-Wilcoxon-test were applied for between-group comparisons. Dichotomous variables are presented as absolute and relative frequencies and were compared applying the Fisher Boschloo-test from the R package “Exact” [11].
For the purpose of selecting variables with predictive impact on the incidence of arrhythmia, the variables sex, age, hypertension, cardiovascular disease, hydroxychloroquine, and combined therapy with hydroxychloroquine and azithromycin were initially considered in terms of variable selection. First, regularized logistic regression using the elastic net penalty implemented in the package “glmnet” was computed [12,13]. The hyperparameters α (elastic net mixing parameter) and β (shrinkage parameter) were tuned conducting 5-fold cross-validation (CV) and a grid search. Subsequently, multiple logistic regression modeling was conducted only incorporating the selected variables, to estimate the odds ratios (ORs) and their 95% confidence intervals (CI). The area under the curve (AUC) value was computed applying the receiver operating characteristics (ROC) curve to evaluate the model using the package “pROC” [14]. To prevent overestimation of the model’s performance measure, the AUC-value was calculated applying 5-fold CV. During 5-fold CV, each patient is part of the training set for four times and is assigned exactly once to the testing set. Hence, in each step a model is fitted based on 80% of the data whereas a probability of the remaining 20% of the patients is estimated with respect to the incidence of arrhythmia.
Information on left ventricular ejection fraction (LVEF) was only available in 44 patients. To account for a potential influence of LVEF on the development of cardiac arrhythmia, we performed attempts to impute the missing data (Supplementary Materials). Due to a high number of missing values, LVEF was omitted from further analyses to ensure reliability of the data.
To evaluate the impact of biomarkers on the incidence of arrhythmia, univariate logistic regression modeling was performed. The AUC-values und the Youden index for identifying the optimal cut-off value were computed for each biomarker, respectively [15]. Confidence intervals of the AUC-values were calculated according to DeLong [16].
To assess the prognostic impact of arrhythmia on clinical outcomes univariate and multiple regression modeling was performed. To preserve the validity of multiple regression modeling in the light of the limited number of patients, the models were adjusted for a maximum of two additional covariates. Age and cardiovascular disease were chosen due to their clinical significance regarding outcome in COVID-19 shown by previous studies [2,17].
Due to the high proportion of newly diagnosed atrial fibrillation (AF), we conducted a subgroup analysis comparing patients with incident AF to patients who neither had a history of AF nor displayed AF in the course of the hospitalization. Due to the small sample size in the subgroup of patients with incident AF, only descriptive analyses were performed.
Due to the exploratory character of this analysis, the p-values are interpreted only in a descriptive sense and no adjustment for multiple testing was applied [18]. p-values < 0.05 were denoted as statistically significant. The statistical analysis was performed using R version 4.0.2. [19] and SPSS version 25.