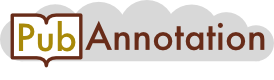
PMC:7786642 / 26129-28082
Annnotations
LitCovid-PubTator
{"project":"LitCovid-PubTator","denotations":[{"id":"305","span":{"begin":86,"end":89},"obj":"Chemical"},{"id":"306","span":{"begin":104,"end":112},"obj":"Disease"},{"id":"307","span":{"begin":113,"end":122},"obj":"Disease"},{"id":"308","span":{"begin":715,"end":723},"obj":"Disease"},{"id":"309","span":{"begin":724,"end":730},"obj":"Disease"},{"id":"310","span":{"begin":1119,"end":1127},"obj":"Disease"},{"id":"311","span":{"begin":1128,"end":1137},"obj":"Disease"},{"id":"312","span":{"begin":1476,"end":1484},"obj":"Disease"},{"id":"313","span":{"begin":1485,"end":1494},"obj":"Disease"},{"id":"314","span":{"begin":1562,"end":1570},"obj":"Disease"},{"id":"315","span":{"begin":1571,"end":1580},"obj":"Disease"}],"attributes":[{"id":"A306","pred":"tao:has_database_id","subj":"306","obj":"MESH:C000657245"},{"id":"A307","pred":"tao:has_database_id","subj":"307","obj":"MESH:D003643"},{"id":"A308","pred":"tao:has_database_id","subj":"308","obj":"MESH:C000657245"},{"id":"A309","pred":"tao:has_database_id","subj":"309","obj":"MESH:D003643"},{"id":"A310","pred":"tao:has_database_id","subj":"310","obj":"MESH:C000657245"},{"id":"A311","pred":"tao:has_database_id","subj":"311","obj":"MESH:D003643"},{"id":"A312","pred":"tao:has_database_id","subj":"312","obj":"MESH:C000657245"},{"id":"A313","pred":"tao:has_database_id","subj":"313","obj":"MESH:D003643"},{"id":"A314","pred":"tao:has_database_id","subj":"314","obj":"MESH:C000657245"},{"id":"A315","pred":"tao:has_database_id","subj":"315","obj":"MESH:D003643"}],"namespaces":[{"prefix":"Tax","uri":"https://www.ncbi.nlm.nih.gov/taxonomy/"},{"prefix":"MESH","uri":"https://id.nlm.nih.gov/mesh/"},{"prefix":"Gene","uri":"https://www.ncbi.nlm.nih.gov/gene/"},{"prefix":"CVCL","uri":"https://web.expasy.org/cellosaurus/CVCL_"}],"text":"Our study is the first study to examine the association between long-term exposure to NO2 and PM2.5 and COVID-19 mortality at very high geographical precision. The spatial unit of our analysis is LSOAs, for which there are 32,844 in England (~130 000 km2), whereas previous studies have used 317 LTLAs or 175 sampling units in England, counties in the US (3 122 in an area ~9.8 million km2) and municipalities in the Netherlands (334 in an area ~41 500 km2). Such high-resolution allows capturing the localised geographical patterns of the pollutants but also ensures adequate confounding and spatial autocorrelation adjustment. Our study also covers, so far, the largest temporal window of the epidemic (capturing COVID-19 deaths attributable to the first wave, Supplemental Material Fig. S34), while most previous studies focused on the early to mid-stages of the first wave. This ensures better generalisability of the results. In addition, physical distancing and other public health interventions were introduced nationwide in England during the first epidemic, mitigating any distortion between air-pollution and COVID-19 mortality due to potential regional level differences. Our results are also consistent in a sensitivity analysis focusing on the pre-lockdown period, in the absence of public health interventions. Based on the scientific literature, we adjusted for several variables which would act as the confounders of the relationship between air pollution and COVID-19 mortality. Nevertheless, since the aetiology and the factors contributing to COVID-19 mortality are not fully understood yet, we included a spatial random effect to capture unknown spatial confounding. The spatial random effect was found to be a crucial component in the model. Not accounting for spatial autocorrelation, when spatial autocorrelation is present, is expected to give rise to narrower credible intervals and false positive effects (Lee and Sarran 2015)."}
LitCovid-sentences
{"project":"LitCovid-sentences","denotations":[{"id":"T224","span":{"begin":0,"end":159},"obj":"Sentence"},{"id":"T225","span":{"begin":160,"end":458},"obj":"Sentence"},{"id":"T226","span":{"begin":459,"end":628},"obj":"Sentence"},{"id":"T227","span":{"begin":629,"end":877},"obj":"Sentence"},{"id":"T228","span":{"begin":878,"end":930},"obj":"Sentence"},{"id":"T229","span":{"begin":931,"end":1182},"obj":"Sentence"},{"id":"T230","span":{"begin":1183,"end":1324},"obj":"Sentence"},{"id":"T231","span":{"begin":1325,"end":1495},"obj":"Sentence"},{"id":"T232","span":{"begin":1496,"end":1686},"obj":"Sentence"},{"id":"T233","span":{"begin":1687,"end":1762},"obj":"Sentence"},{"id":"T234","span":{"begin":1763,"end":1953},"obj":"Sentence"}],"namespaces":[{"prefix":"_base","uri":"http://pubannotation.org/ontology/tao.owl#"}],"text":"Our study is the first study to examine the association between long-term exposure to NO2 and PM2.5 and COVID-19 mortality at very high geographical precision. The spatial unit of our analysis is LSOAs, for which there are 32,844 in England (~130 000 km2), whereas previous studies have used 317 LTLAs or 175 sampling units in England, counties in the US (3 122 in an area ~9.8 million km2) and municipalities in the Netherlands (334 in an area ~41 500 km2). Such high-resolution allows capturing the localised geographical patterns of the pollutants but also ensures adequate confounding and spatial autocorrelation adjustment. Our study also covers, so far, the largest temporal window of the epidemic (capturing COVID-19 deaths attributable to the first wave, Supplemental Material Fig. S34), while most previous studies focused on the early to mid-stages of the first wave. This ensures better generalisability of the results. In addition, physical distancing and other public health interventions were introduced nationwide in England during the first epidemic, mitigating any distortion between air-pollution and COVID-19 mortality due to potential regional level differences. Our results are also consistent in a sensitivity analysis focusing on the pre-lockdown period, in the absence of public health interventions. Based on the scientific literature, we adjusted for several variables which would act as the confounders of the relationship between air pollution and COVID-19 mortality. Nevertheless, since the aetiology and the factors contributing to COVID-19 mortality are not fully understood yet, we included a spatial random effect to capture unknown spatial confounding. The spatial random effect was found to be a crucial component in the model. Not accounting for spatial autocorrelation, when spatial autocorrelation is present, is expected to give rise to narrower credible intervals and false positive effects (Lee and Sarran 2015)."}