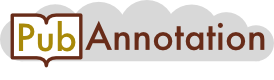
PMC:7786642 / 21829-32462
Annnotations
LitCovid-PD-HP
{"project":"LitCovid-PD-HP","denotations":[{"id":"T10","span":{"begin":8719,"end":8731},"obj":"Phenotype"},{"id":"T11","span":{"begin":8746,"end":8750},"obj":"Phenotype"},{"id":"T12","span":{"begin":9911,"end":9923},"obj":"Phenotype"},{"id":"T13","span":{"begin":9938,"end":9942},"obj":"Phenotype"}],"attributes":[{"id":"A10","pred":"hp_id","subj":"T10","obj":"http://purl.obolibrary.org/obo/HP_0000822"},{"id":"A11","pred":"hp_id","subj":"T11","obj":"http://purl.obolibrary.org/obo/HP_0006510"},{"id":"A12","pred":"hp_id","subj":"T12","obj":"http://purl.obolibrary.org/obo/HP_0000822"},{"id":"A13","pred":"hp_id","subj":"T13","obj":"http://purl.obolibrary.org/obo/HP_0006510"}],"text":"4 Discussion\n\n4.1 Main findings\nThis is the first nationwide study in England investigating the effect of long-term exposure to NO2 and PM2.5 during 2014–2018 on COVID-19 mortality at LSOA level. The unadjusted models indicate that for every 1 μg/m3 increase in the long-term exposure to NO2 and PM2.5 the COVID-19 mortality risk increases. After considering the effect of confounding and spatial autocorrelation there is still some evidence of an effect, albeit is less strong, for NO2, while for PM2.5 there is larger uncertainty. The spatial relative risk has strong spatial patterns, identical for the different pollutants, potentially highlighting the effect of disease spread.\n\n4.2 Comparison with previous studies focusing on NO2\nOur study is comparable with previous studies in the US, England and the Netherlands assessing the long-term effect of NO2 in COVID-19 mortality. The study in the US focused on deaths reported by April 29, 2020, using 3122 counties. For the exposure, they calculated the mean of daily concentrations during 2010–2016 as modelled by a previously described ensemble machine learning model (R2 = 0.79) (Di et al., 2019a). They reported a 7.1% (95% Confidence Interval: 1.2%, 13.4%) increase in mortality per 4.5 ppb (1 ppb = 1.25 μg/m3) increase in NO2 after adjusting for confounders and spatial autocorrelation(Liang et al. 2020)(that is approximately 1.3% increase per 1 μg/m3). A study in England, with partly overlapping data as in our analysis, also reported a significant association between NO2 and COVID-19 mortality (p \u003c 0.05). For the analysis they focused on COVID-19 deaths reported in England up to April 10, 2020, used 317 LTLAs, and did not account for spatial autocorrelation (Travaglio et al. 2020). The study in the Netherlands using 335 municipalities, mean exposure during 2015–2019 and COVID-19 deaths up to June 5, 2020 reported 0.35 (95% CI: 0.04, 0.66) additional COVID-19 deaths for every 1 μg/m3 increase in NO2 after adjusting for confounders and certain spatial controls, such as transmission beyond the Dutch national borders (Cole et al. 2020). Since the mean number of deaths in their sample is 16.86, the above estimate translates to a 2.0% increase in the COVID-19 mortality for every 1 μg/m3 increase in NO2. An ONS report in England using 175 sampling units, 10-year averaged NO2 exposure (PCM) and COVID-19 deaths up to June 12, 2020 found a 0.6% (95% CI: −0.1%, 2.2%) increase in the COVID-19 mortality for every 1 μg/m3 increase in averaged NO2 exposure (Statistics 2020).\n\n4.3 Comparison with previous studies focusing on PM2.5\nOur study is comparable with previous studies assessing the long-term effect of PM2.5 on COVID-19 mortality. The aforementioned study in the US also assessed the effect of PM2.5 on COVID-19 mortality(Liang et al. 2020). Their exposure model was previously validated having an R2 = 0.89 for the annual estimates (Di et al. 2019b). The evidence for PM2.5 was weak, namely 10.8% (95% CI:-1.1%, 24.1%) per 3.4 μg/m3 increase in PM2.5 concentration (that is approximately 3.2% increase per 1 μg/m3) after adjusting for confounding and spatial autocorrelation. The ONS report in England found a 1% (95% CI: −3%, 6%) increase in the COVID-19 mortality for every 1 μg/m3 increase in the 10-year averaged PM2.5 exposure (Statistics 2020). Our study comes in contrast with another study in the US that used deaths reported until April 22nd, 2020 and counties as the geographical unit (Wu et al. 2020). For the exposure, they used previously validated monthly PM2.5 concentrations (R2 = 0.70) (Van Donkelaar et al. 2019) and averaged them during 2000 and 2016. After adjusting for confounding but not for spatial autocorrelation, they found an 11% (95% CI: 6%, 17%) increase in the COVID-19 death rate for an increase of 1 μg/m3 in PM2.5 concentration (Wu et al. 2020). Our study comes also in contrast with the study in the Netherlands that reported 2.3 (95% CI: 1.3, 3.0) additional COVID-19 deaths for an increase of 1 μg/m3 in the averaged long-term PM2.5 concentration (Cole et al. 2020). Having a mean number of deaths equal to 16.86, the above estimate translates to a 13.6% increase in the COVID-19 mortality rate for an increase of 1 μg/m3 in PM2.5 concentration.\n\n4.4 Strengths and limitations\nOur study is the first study to examine the association between long-term exposure to NO2 and PM2.5 and COVID-19 mortality at very high geographical precision. The spatial unit of our analysis is LSOAs, for which there are 32,844 in England (~130 000 km2), whereas previous studies have used 317 LTLAs or 175 sampling units in England, counties in the US (3 122 in an area ~9.8 million km2) and municipalities in the Netherlands (334 in an area ~41 500 km2). Such high-resolution allows capturing the localised geographical patterns of the pollutants but also ensures adequate confounding and spatial autocorrelation adjustment. Our study also covers, so far, the largest temporal window of the epidemic (capturing COVID-19 deaths attributable to the first wave, Supplemental Material Fig. S34), while most previous studies focused on the early to mid-stages of the first wave. This ensures better generalisability of the results. In addition, physical distancing and other public health interventions were introduced nationwide in England during the first epidemic, mitigating any distortion between air-pollution and COVID-19 mortality due to potential regional level differences. Our results are also consistent in a sensitivity analysis focusing on the pre-lockdown period, in the absence of public health interventions. Based on the scientific literature, we adjusted for several variables which would act as the confounders of the relationship between air pollution and COVID-19 mortality. Nevertheless, since the aetiology and the factors contributing to COVID-19 mortality are not fully understood yet, we included a spatial random effect to capture unknown spatial confounding. The spatial random effect was found to be a crucial component in the model. Not accounting for spatial autocorrelation, when spatial autocorrelation is present, is expected to give rise to narrower credible intervals and false positive effects (Lee and Sarran 2015).\nOur study has also some limitations. The downscaling procedure will likely inflate the reported credible intervals. However, this naturally reflects the uncertainty of the place of residence resulted from the downscaling approach. Although we consider small areas, the study is still an ecological one and thus the reported effects do not reflect individual associations (Wakefield 2008). Case fatality might have been a more appropriate metric for the analysis, since disease spread is accounted for in the denominator. Nevertheless, given the asymptomatic infections and the fact that number of reported infections is not a random sample of the general population, the number of COVID-19 cases per LTLA is not reliable at this stage. For the same reason, using the number of reported cases to adjust for disease progression and clustering of cases and deaths might not adequately capture disease progression and clustering of cases and deaths. However, part of this clustering was captured in the spatial autocorrelation term. We did not account for population mobility during 2014–2018 and assumed constant residence and thus levels of exposure to air-pollution. While this is a limitation, we believe that it would have a minimal impact on the results given that 1) the exposure period is relatively short and 2) almost 93% of the deaths in our dataset occurred in people 60 years or older (Supplemental Material Table S2). This comprises a population less likely to have moved during the past 5 years (Burgess and Quinio 2020). We also could not account for non-residential air-pollution exposure. Spatiotemporal variation in the strains of COVID-19 can introduce bias (Villeneuve and Goldberg 2020), however at the time of publication there was no evidence supporting that strain types can confound the relationship between COVID-19 mortality and air-pollution.\n\n4.5 Interpretation\nCompared to the previous studies, our results are the smallest in magnitude, likely because of the high geographical precision that allows more accurate confounding and spatial autocorrelation adjustment. In addition, we report weak evidence of an effect, which could also be due to lack of power and individual exposure data. Nevertheless, as for NO2 we find a high posterior probability of an effect on mortality, we argue that a potential explanation might be the mediation effect of pre-existing conditions. While in our analysis the inclusion of area-level prevalence of hypertension, diabetes and COPD did not change the results, the ecological nature of the pre-existing conditions data does not allow us to account for the mediation effect at the individual level. Our study focuses on the mortality after contracting SARS-CoV-2, however we cannot rule out individual susceptibility to becoming infected as an explanation to the uncertainty in the effect estimates (Villeneuve and Goldberg 2020). Such susceptibility can reflect immunosuppression, leading to later increases in inflammation (Edoardo Conticini et al. 2020) and thus worse prognosis, or even disease spread, as recent studies have suggested that PM2.5 can proliferate COVID-19 transmission (Bianconi et al. 2020).\nOur analysis captured strong spatial autocorrelation. The observed pattern could reflect residual variation from a potential inadequate covariate adjustment (including disease spread), spatial variation of pre-existing conditions, other unknown spatial confounders or a combination from all above. In a sensitivity analysis, we observed that the factors associated with disease transmission left the latent field unchanged (Supplemental Material Fig. S21), as did the inclusion of hypertension, diabetes and COPD (Supplemental Material Fig. S33). When we restricted the analysis to the pre-lockdown period, the latent field for both pollutants captured London and Birmingham, i.e. the cities with the first outbreaks. Considering the above, and the fact that COVID-19 is an infectious disease, we believe that large variation of Fig. 4 is likely due to disease spread, which is not adequately captured in the disease progression covariates.\nFig. 4 Median posterior spatial relative risk (exponential of the spatial autocorrelation term) and posterior probability that the spatial relative risk is larger than 1 for the models with NO2 and a spatial autocorrelation term and the fully adjusted NO2 model."}
LitCovid-PubTator
{"project":"LitCovid-PubTator","denotations":[{"id":"209","span":{"begin":130,"end":133},"obj":"Chemical"},{"id":"210","span":{"begin":290,"end":293},"obj":"Chemical"},{"id":"211","span":{"begin":485,"end":488},"obj":"Chemical"},{"id":"212","span":{"begin":164,"end":172},"obj":"Disease"},{"id":"213","span":{"begin":173,"end":182},"obj":"Disease"},{"id":"214","span":{"begin":308,"end":316},"obj":"Disease"},{"id":"215","span":{"begin":317,"end":326},"obj":"Disease"},{"id":"241","span":{"begin":859,"end":862},"obj":"Chemical"},{"id":"242","span":{"begin":1286,"end":1289},"obj":"Chemical"},{"id":"243","span":{"begin":1972,"end":1975},"obj":"Chemical"},{"id":"244","span":{"begin":2276,"end":2279},"obj":"Chemical"},{"id":"245","span":{"begin":2349,"end":2352},"obj":"Chemical"},{"id":"246","span":{"begin":2517,"end":2520},"obj":"Chemical"},{"id":"247","span":{"begin":866,"end":874},"obj":"Disease"},{"id":"248","span":{"begin":875,"end":884},"obj":"Disease"},{"id":"249","span":{"begin":917,"end":923},"obj":"Disease"},{"id":"250","span":{"begin":1231,"end":1240},"obj":"Disease"},{"id":"251","span":{"begin":1544,"end":1552},"obj":"Disease"},{"id":"252","span":{"begin":1553,"end":1562},"obj":"Disease"},{"id":"253","span":{"begin":1608,"end":1616},"obj":"Disease"},{"id":"254","span":{"begin":1617,"end":1623},"obj":"Disease"},{"id":"255","span":{"begin":1845,"end":1853},"obj":"Disease"},{"id":"256","span":{"begin":1854,"end":1860},"obj":"Disease"},{"id":"257","span":{"begin":1926,"end":1934},"obj":"Disease"},{"id":"258","span":{"begin":1935,"end":1941},"obj":"Disease"},{"id":"259","span":{"begin":2138,"end":2144},"obj":"Disease"},{"id":"260","span":{"begin":2227,"end":2235},"obj":"Disease"},{"id":"261","span":{"begin":2236,"end":2245},"obj":"Disease"},{"id":"262","span":{"begin":2372,"end":2380},"obj":"Disease"},{"id":"263","span":{"begin":2381,"end":2387},"obj":"Disease"},{"id":"264","span":{"begin":2459,"end":2467},"obj":"Disease"},{"id":"265","span":{"begin":2468,"end":2477},"obj":"Disease"},{"id":"280","span":{"begin":2695,"end":2703},"obj":"Disease"},{"id":"281","span":{"begin":2704,"end":2713},"obj":"Disease"},{"id":"282","span":{"begin":2787,"end":2795},"obj":"Disease"},{"id":"283","span":{"begin":2796,"end":2805},"obj":"Disease"},{"id":"284","span":{"begin":3232,"end":3240},"obj":"Disease"},{"id":"285","span":{"begin":3241,"end":3250},"obj":"Disease"},{"id":"286","span":{"begin":3403,"end":3409},"obj":"Disease"},{"id":"287","span":{"begin":3777,"end":3785},"obj":"Disease"},{"id":"288","span":{"begin":3786,"end":3791},"obj":"Disease"},{"id":"289","span":{"begin":3980,"end":3988},"obj":"Disease"},{"id":"290","span":{"begin":3989,"end":3995},"obj":"Disease"},{"id":"291","span":{"begin":4113,"end":4119},"obj":"Disease"},{"id":"292","span":{"begin":4193,"end":4201},"obj":"Disease"},{"id":"293","span":{"begin":4202,"end":4211},"obj":"Disease"},{"id":"305","span":{"begin":4386,"end":4389},"obj":"Chemical"},{"id":"306","span":{"begin":4404,"end":4412},"obj":"Disease"},{"id":"307","span":{"begin":4413,"end":4422},"obj":"Disease"},{"id":"308","span":{"begin":5015,"end":5023},"obj":"Disease"},{"id":"309","span":{"begin":5024,"end":5030},"obj":"Disease"},{"id":"310","span":{"begin":5419,"end":5427},"obj":"Disease"},{"id":"311","span":{"begin":5428,"end":5437},"obj":"Disease"},{"id":"312","span":{"begin":5776,"end":5784},"obj":"Disease"},{"id":"313","span":{"begin":5785,"end":5794},"obj":"Disease"},{"id":"314","span":{"begin":5862,"end":5870},"obj":"Disease"},{"id":"315","span":{"begin":5871,"end":5880},"obj":"Disease"},{"id":"326","span":{"begin":7623,"end":7629},"obj":"Species"},{"id":"327","span":{"begin":6812,"end":6822},"obj":"Disease"},{"id":"328","span":{"begin":6860,"end":6870},"obj":"Disease"},{"id":"329","span":{"begin":6935,"end":6943},"obj":"Disease"},{"id":"330","span":{"begin":7108,"end":7114},"obj":"Disease"},{"id":"331","span":{"begin":7192,"end":7198},"obj":"Disease"},{"id":"332","span":{"begin":7589,"end":7595},"obj":"Disease"},{"id":"333","span":{"begin":7900,"end":7908},"obj":"Disease"},{"id":"334","span":{"begin":8084,"end":8092},"obj":"Disease"},{"id":"335","span":{"begin":8093,"end":8102},"obj":"Disease"},{"id":"346","span":{"begin":8969,"end":8979},"obj":"Species"},{"id":"347","span":{"begin":8491,"end":8494},"obj":"Chemical"},{"id":"348","span":{"begin":8548,"end":8557},"obj":"Disease"},{"id":"349","span":{"begin":8719,"end":8731},"obj":"Disease"},{"id":"350","span":{"begin":8733,"end":8741},"obj":"Disease"},{"id":"351","span":{"begin":8746,"end":8750},"obj":"Disease"},{"id":"352","span":{"begin":8941,"end":8950},"obj":"Disease"},{"id":"353","span":{"begin":9046,"end":9054},"obj":"Disease"},{"id":"354","span":{"begin":9229,"end":9241},"obj":"Disease"},{"id":"355","span":{"begin":9384,"end":9392},"obj":"Disease"},{"id":"358","span":{"begin":10561,"end":10564},"obj":"Chemical"},{"id":"359","span":{"begin":10623,"end":10626},"obj":"Chemical"},{"id":"365","span":{"begin":9911,"end":9923},"obj":"Disease"},{"id":"366","span":{"begin":9925,"end":9933},"obj":"Disease"},{"id":"367","span":{"begin":9938,"end":9942},"obj":"Disease"},{"id":"368","span":{"begin":10189,"end":10197},"obj":"Disease"},{"id":"369","span":{"begin":10204,"end":10222},"obj":"Disease"}],"attributes":[{"id":"A212","pred":"tao:has_database_id","subj":"212","obj":"MESH:C000657245"},{"id":"A213","pred":"tao:has_database_id","subj":"213","obj":"MESH:D003643"},{"id":"A214","pred":"tao:has_database_id","subj":"214","obj":"MESH:C000657245"},{"id":"A215","pred":"tao:has_database_id","subj":"215","obj":"MESH:D003643"},{"id":"A247","pred":"tao:has_database_id","subj":"247","obj":"MESH:C000657245"},{"id":"A248","pred":"tao:has_database_id","subj":"248","obj":"MESH:D003643"},{"id":"A249","pred":"tao:has_database_id","subj":"249","obj":"MESH:D003643"},{"id":"A250","pred":"tao:has_database_id","subj":"250","obj":"MESH:D003643"},{"id":"A251","pred":"tao:has_database_id","subj":"251","obj":"MESH:C000657245"},{"id":"A252","pred":"tao:has_database_id","subj":"252","obj":"MESH:D003643"},{"id":"A253","pred":"tao:has_database_id","subj":"253","obj":"MESH:C000657245"},{"id":"A254","pred":"tao:has_database_id","subj":"254","obj":"MESH:D003643"},{"id":"A255","pred":"tao:has_database_id","subj":"255","obj":"MESH:C000657245"},{"id":"A256","pred":"tao:has_database_id","subj":"256","obj":"MESH:D003643"},{"id":"A257","pred":"tao:has_database_id","subj":"257","obj":"MESH:C000657245"},{"id":"A258","pred":"tao:has_database_id","subj":"258","obj":"MESH:D003643"},{"id":"A259","pred":"tao:has_database_id","subj":"259","obj":"MESH:D003643"},{"id":"A260","pred":"tao:has_database_id","subj":"260","obj":"MESH:C000657245"},{"id":"A261","pred":"tao:has_database_id","subj":"261","obj":"MESH:D003643"},{"id":"A262","pred":"tao:has_database_id","subj":"262","obj":"MESH:C000657245"},{"id":"A263","pred":"tao:has_database_id","subj":"263","obj":"MESH:D003643"},{"id":"A264","pred":"tao:has_database_id","subj":"264","obj":"MESH:C000657245"},{"id":"A265","pred":"tao:has_database_id","subj":"265","obj":"MESH:D003643"},{"id":"A280","pred":"tao:has_database_id","subj":"280","obj":"MESH:C000657245"},{"id":"A281","pred":"tao:has_database_id","subj":"281","obj":"MESH:D003643"},{"id":"A282","pred":"tao:has_database_id","subj":"282","obj":"MESH:C000657245"},{"id":"A283","pred":"tao:has_database_id","subj":"283","obj":"MESH:D003643"},{"id":"A284","pred":"tao:has_database_id","subj":"284","obj":"MESH:C000657245"},{"id":"A285","pred":"tao:has_database_id","subj":"285","obj":"MESH:D003643"},{"id":"A286","pred":"tao:has_database_id","subj":"286","obj":"MESH:D003643"},{"id":"A287","pred":"tao:has_database_id","subj":"287","obj":"MESH:C000657245"},{"id":"A288","pred":"tao:has_database_id","subj":"288","obj":"MESH:D003643"},{"id":"A289","pred":"tao:has_database_id","subj":"289","obj":"MESH:C000657245"},{"id":"A290","pred":"tao:has_database_id","subj":"290","obj":"MESH:D003643"},{"id":"A291","pred":"tao:has_database_id","subj":"291","obj":"MESH:D003643"},{"id":"A292","pred":"tao:has_database_id","subj":"292","obj":"MESH:C000657245"},{"id":"A293","pred":"tao:has_database_id","subj":"293","obj":"MESH:D003643"},{"id":"A306","pred":"tao:has_database_id","subj":"306","obj":"MESH:C000657245"},{"id":"A307","pred":"tao:has_database_id","subj":"307","obj":"MESH:D003643"},{"id":"A308","pred":"tao:has_database_id","subj":"308","obj":"MESH:C000657245"},{"id":"A309","pred":"tao:has_database_id","subj":"309","obj":"MESH:D003643"},{"id":"A310","pred":"tao:has_database_id","subj":"310","obj":"MESH:C000657245"},{"id":"A311","pred":"tao:has_database_id","subj":"311","obj":"MESH:D003643"},{"id":"A312","pred":"tao:has_database_id","subj":"312","obj":"MESH:C000657245"},{"id":"A313","pred":"tao:has_database_id","subj":"313","obj":"MESH:D003643"},{"id":"A314","pred":"tao:has_database_id","subj":"314","obj":"MESH:C000657245"},{"id":"A315","pred":"tao:has_database_id","subj":"315","obj":"MESH:D003643"},{"id":"A326","pred":"tao:has_database_id","subj":"326","obj":"Tax:9606"},{"id":"A327","pred":"tao:has_database_id","subj":"327","obj":"MESH:D007239"},{"id":"A328","pred":"tao:has_database_id","subj":"328","obj":"MESH:D007239"},{"id":"A329","pred":"tao:has_database_id","subj":"329","obj":"MESH:C000657245"},{"id":"A330","pred":"tao:has_database_id","subj":"330","obj":"MESH:D003643"},{"id":"A331","pred":"tao:has_database_id","subj":"331","obj":"MESH:D003643"},{"id":"A332","pred":"tao:has_database_id","subj":"332","obj":"MESH:D003643"},{"id":"A333","pred":"tao:has_database_id","subj":"333","obj":"MESH:C000657245"},{"id":"A334","pred":"tao:has_database_id","subj":"334","obj":"MESH:C000657245"},{"id":"A335","pred":"tao:has_database_id","subj":"335","obj":"MESH:D003643"},{"id":"A346","pred":"tao:has_database_id","subj":"346","obj":"Tax:2697049"},{"id":"A348","pred":"tao:has_database_id","subj":"348","obj":"MESH:D003643"},{"id":"A349","pred":"tao:has_database_id","subj":"349","obj":"MESH:D006973"},{"id":"A350","pred":"tao:has_database_id","subj":"350","obj":"MESH:D003920"},{"id":"A351","pred":"tao:has_database_id","subj":"351","obj":"MESH:D029424"},{"id":"A352","pred":"tao:has_database_id","subj":"352","obj":"MESH:D003643"},{"id":"A353","pred":"tao:has_database_id","subj":"353","obj":"MESH:D007239"},{"id":"A354","pred":"tao:has_database_id","subj":"354","obj":"MESH:D007249"},{"id":"A355","pred":"tao:has_database_id","subj":"355","obj":"MESH:C000657245"},{"id":"A365","pred":"tao:has_database_id","subj":"365","obj":"MESH:D006973"},{"id":"A366","pred":"tao:has_database_id","subj":"366","obj":"MESH:D003920"},{"id":"A367","pred":"tao:has_database_id","subj":"367","obj":"MESH:D029424"},{"id":"A368","pred":"tao:has_database_id","subj":"368","obj":"MESH:C000657245"},{"id":"A369","pred":"tao:has_database_id","subj":"369","obj":"MESH:D003141"}],"namespaces":[{"prefix":"Tax","uri":"https://www.ncbi.nlm.nih.gov/taxonomy/"},{"prefix":"MESH","uri":"https://id.nlm.nih.gov/mesh/"},{"prefix":"Gene","uri":"https://www.ncbi.nlm.nih.gov/gene/"},{"prefix":"CVCL","uri":"https://web.expasy.org/cellosaurus/CVCL_"}],"text":"4 Discussion\n\n4.1 Main findings\nThis is the first nationwide study in England investigating the effect of long-term exposure to NO2 and PM2.5 during 2014–2018 on COVID-19 mortality at LSOA level. The unadjusted models indicate that for every 1 μg/m3 increase in the long-term exposure to NO2 and PM2.5 the COVID-19 mortality risk increases. After considering the effect of confounding and spatial autocorrelation there is still some evidence of an effect, albeit is less strong, for NO2, while for PM2.5 there is larger uncertainty. The spatial relative risk has strong spatial patterns, identical for the different pollutants, potentially highlighting the effect of disease spread.\n\n4.2 Comparison with previous studies focusing on NO2\nOur study is comparable with previous studies in the US, England and the Netherlands assessing the long-term effect of NO2 in COVID-19 mortality. The study in the US focused on deaths reported by April 29, 2020, using 3122 counties. For the exposure, they calculated the mean of daily concentrations during 2010–2016 as modelled by a previously described ensemble machine learning model (R2 = 0.79) (Di et al., 2019a). They reported a 7.1% (95% Confidence Interval: 1.2%, 13.4%) increase in mortality per 4.5 ppb (1 ppb = 1.25 μg/m3) increase in NO2 after adjusting for confounders and spatial autocorrelation(Liang et al. 2020)(that is approximately 1.3% increase per 1 μg/m3). A study in England, with partly overlapping data as in our analysis, also reported a significant association between NO2 and COVID-19 mortality (p \u003c 0.05). For the analysis they focused on COVID-19 deaths reported in England up to April 10, 2020, used 317 LTLAs, and did not account for spatial autocorrelation (Travaglio et al. 2020). The study in the Netherlands using 335 municipalities, mean exposure during 2015–2019 and COVID-19 deaths up to June 5, 2020 reported 0.35 (95% CI: 0.04, 0.66) additional COVID-19 deaths for every 1 μg/m3 increase in NO2 after adjusting for confounders and certain spatial controls, such as transmission beyond the Dutch national borders (Cole et al. 2020). Since the mean number of deaths in their sample is 16.86, the above estimate translates to a 2.0% increase in the COVID-19 mortality for every 1 μg/m3 increase in NO2. An ONS report in England using 175 sampling units, 10-year averaged NO2 exposure (PCM) and COVID-19 deaths up to June 12, 2020 found a 0.6% (95% CI: −0.1%, 2.2%) increase in the COVID-19 mortality for every 1 μg/m3 increase in averaged NO2 exposure (Statistics 2020).\n\n4.3 Comparison with previous studies focusing on PM2.5\nOur study is comparable with previous studies assessing the long-term effect of PM2.5 on COVID-19 mortality. The aforementioned study in the US also assessed the effect of PM2.5 on COVID-19 mortality(Liang et al. 2020). Their exposure model was previously validated having an R2 = 0.89 for the annual estimates (Di et al. 2019b). The evidence for PM2.5 was weak, namely 10.8% (95% CI:-1.1%, 24.1%) per 3.4 μg/m3 increase in PM2.5 concentration (that is approximately 3.2% increase per 1 μg/m3) after adjusting for confounding and spatial autocorrelation. The ONS report in England found a 1% (95% CI: −3%, 6%) increase in the COVID-19 mortality for every 1 μg/m3 increase in the 10-year averaged PM2.5 exposure (Statistics 2020). Our study comes in contrast with another study in the US that used deaths reported until April 22nd, 2020 and counties as the geographical unit (Wu et al. 2020). For the exposure, they used previously validated monthly PM2.5 concentrations (R2 = 0.70) (Van Donkelaar et al. 2019) and averaged them during 2000 and 2016. After adjusting for confounding but not for spatial autocorrelation, they found an 11% (95% CI: 6%, 17%) increase in the COVID-19 death rate for an increase of 1 μg/m3 in PM2.5 concentration (Wu et al. 2020). Our study comes also in contrast with the study in the Netherlands that reported 2.3 (95% CI: 1.3, 3.0) additional COVID-19 deaths for an increase of 1 μg/m3 in the averaged long-term PM2.5 concentration (Cole et al. 2020). Having a mean number of deaths equal to 16.86, the above estimate translates to a 13.6% increase in the COVID-19 mortality rate for an increase of 1 μg/m3 in PM2.5 concentration.\n\n4.4 Strengths and limitations\nOur study is the first study to examine the association between long-term exposure to NO2 and PM2.5 and COVID-19 mortality at very high geographical precision. The spatial unit of our analysis is LSOAs, for which there are 32,844 in England (~130 000 km2), whereas previous studies have used 317 LTLAs or 175 sampling units in England, counties in the US (3 122 in an area ~9.8 million km2) and municipalities in the Netherlands (334 in an area ~41 500 km2). Such high-resolution allows capturing the localised geographical patterns of the pollutants but also ensures adequate confounding and spatial autocorrelation adjustment. Our study also covers, so far, the largest temporal window of the epidemic (capturing COVID-19 deaths attributable to the first wave, Supplemental Material Fig. S34), while most previous studies focused on the early to mid-stages of the first wave. This ensures better generalisability of the results. In addition, physical distancing and other public health interventions were introduced nationwide in England during the first epidemic, mitigating any distortion between air-pollution and COVID-19 mortality due to potential regional level differences. Our results are also consistent in a sensitivity analysis focusing on the pre-lockdown period, in the absence of public health interventions. Based on the scientific literature, we adjusted for several variables which would act as the confounders of the relationship between air pollution and COVID-19 mortality. Nevertheless, since the aetiology and the factors contributing to COVID-19 mortality are not fully understood yet, we included a spatial random effect to capture unknown spatial confounding. The spatial random effect was found to be a crucial component in the model. Not accounting for spatial autocorrelation, when spatial autocorrelation is present, is expected to give rise to narrower credible intervals and false positive effects (Lee and Sarran 2015).\nOur study has also some limitations. The downscaling procedure will likely inflate the reported credible intervals. However, this naturally reflects the uncertainty of the place of residence resulted from the downscaling approach. Although we consider small areas, the study is still an ecological one and thus the reported effects do not reflect individual associations (Wakefield 2008). Case fatality might have been a more appropriate metric for the analysis, since disease spread is accounted for in the denominator. Nevertheless, given the asymptomatic infections and the fact that number of reported infections is not a random sample of the general population, the number of COVID-19 cases per LTLA is not reliable at this stage. For the same reason, using the number of reported cases to adjust for disease progression and clustering of cases and deaths might not adequately capture disease progression and clustering of cases and deaths. However, part of this clustering was captured in the spatial autocorrelation term. We did not account for population mobility during 2014–2018 and assumed constant residence and thus levels of exposure to air-pollution. While this is a limitation, we believe that it would have a minimal impact on the results given that 1) the exposure period is relatively short and 2) almost 93% of the deaths in our dataset occurred in people 60 years or older (Supplemental Material Table S2). This comprises a population less likely to have moved during the past 5 years (Burgess and Quinio 2020). We also could not account for non-residential air-pollution exposure. Spatiotemporal variation in the strains of COVID-19 can introduce bias (Villeneuve and Goldberg 2020), however at the time of publication there was no evidence supporting that strain types can confound the relationship between COVID-19 mortality and air-pollution.\n\n4.5 Interpretation\nCompared to the previous studies, our results are the smallest in magnitude, likely because of the high geographical precision that allows more accurate confounding and spatial autocorrelation adjustment. In addition, we report weak evidence of an effect, which could also be due to lack of power and individual exposure data. Nevertheless, as for NO2 we find a high posterior probability of an effect on mortality, we argue that a potential explanation might be the mediation effect of pre-existing conditions. While in our analysis the inclusion of area-level prevalence of hypertension, diabetes and COPD did not change the results, the ecological nature of the pre-existing conditions data does not allow us to account for the mediation effect at the individual level. Our study focuses on the mortality after contracting SARS-CoV-2, however we cannot rule out individual susceptibility to becoming infected as an explanation to the uncertainty in the effect estimates (Villeneuve and Goldberg 2020). Such susceptibility can reflect immunosuppression, leading to later increases in inflammation (Edoardo Conticini et al. 2020) and thus worse prognosis, or even disease spread, as recent studies have suggested that PM2.5 can proliferate COVID-19 transmission (Bianconi et al. 2020).\nOur analysis captured strong spatial autocorrelation. The observed pattern could reflect residual variation from a potential inadequate covariate adjustment (including disease spread), spatial variation of pre-existing conditions, other unknown spatial confounders or a combination from all above. In a sensitivity analysis, we observed that the factors associated with disease transmission left the latent field unchanged (Supplemental Material Fig. S21), as did the inclusion of hypertension, diabetes and COPD (Supplemental Material Fig. S33). When we restricted the analysis to the pre-lockdown period, the latent field for both pollutants captured London and Birmingham, i.e. the cities with the first outbreaks. Considering the above, and the fact that COVID-19 is an infectious disease, we believe that large variation of Fig. 4 is likely due to disease spread, which is not adequately captured in the disease progression covariates.\nFig. 4 Median posterior spatial relative risk (exponential of the spatial autocorrelation term) and posterior probability that the spatial relative risk is larger than 1 for the models with NO2 and a spatial autocorrelation term and the fully adjusted NO2 model."}
LitCovid-sentences
{"project":"LitCovid-sentences","denotations":[{"id":"T183","span":{"begin":0,"end":13},"obj":"Sentence"},{"id":"T184","span":{"begin":15,"end":33},"obj":"Sentence"},{"id":"T185","span":{"begin":34,"end":197},"obj":"Sentence"},{"id":"T186","span":{"begin":198,"end":342},"obj":"Sentence"},{"id":"T187","span":{"begin":343,"end":534},"obj":"Sentence"},{"id":"T188","span":{"begin":535,"end":684},"obj":"Sentence"},{"id":"T189","span":{"begin":686,"end":739},"obj":"Sentence"},{"id":"T190","span":{"begin":740,"end":885},"obj":"Sentence"},{"id":"T191","span":{"begin":886,"end":972},"obj":"Sentence"},{"id":"T192","span":{"begin":973,"end":1158},"obj":"Sentence"},{"id":"T193","span":{"begin":1159,"end":1205},"obj":"Sentence"},{"id":"T194","span":{"begin":1206,"end":1362},"obj":"Sentence"},{"id":"T195","span":{"begin":1363,"end":1418},"obj":"Sentence"},{"id":"T196","span":{"begin":1419,"end":1574},"obj":"Sentence"},{"id":"T197","span":{"begin":1575,"end":1747},"obj":"Sentence"},{"id":"T198","span":{"begin":1748,"end":1754},"obj":"Sentence"},{"id":"T199","span":{"begin":1755,"end":1902},"obj":"Sentence"},{"id":"T200","span":{"begin":1903,"end":2105},"obj":"Sentence"},{"id":"T201","span":{"begin":2106,"end":2112},"obj":"Sentence"},{"id":"T202","span":{"begin":2113,"end":2280},"obj":"Sentence"},{"id":"T203","span":{"begin":2281,"end":2548},"obj":"Sentence"},{"id":"T204","span":{"begin":2550,"end":2605},"obj":"Sentence"},{"id":"T205","span":{"begin":2606,"end":2714},"obj":"Sentence"},{"id":"T206","span":{"begin":2715,"end":2818},"obj":"Sentence"},{"id":"T207","span":{"begin":2819,"end":2825},"obj":"Sentence"},{"id":"T208","span":{"begin":2826,"end":2927},"obj":"Sentence"},{"id":"T209","span":{"begin":2928,"end":2935},"obj":"Sentence"},{"id":"T210","span":{"begin":2936,"end":3160},"obj":"Sentence"},{"id":"T211","span":{"begin":3161,"end":3335},"obj":"Sentence"},{"id":"T212","span":{"begin":3336,"end":3490},"obj":"Sentence"},{"id":"T213","span":{"begin":3491,"end":3497},"obj":"Sentence"},{"id":"T214","span":{"begin":3498,"end":3609},"obj":"Sentence"},{"id":"T215","span":{"begin":3610,"end":3655},"obj":"Sentence"},{"id":"T216","span":{"begin":3656,"end":3751},"obj":"Sentence"},{"id":"T217","span":{"begin":3752,"end":3857},"obj":"Sentence"},{"id":"T218","span":{"begin":3858,"end":3864},"obj":"Sentence"},{"id":"T219","span":{"begin":3865,"end":3958},"obj":"Sentence"},{"id":"T220","span":{"begin":3959,"end":4081},"obj":"Sentence"},{"id":"T221","span":{"begin":4082,"end":4088},"obj":"Sentence"},{"id":"T222","span":{"begin":4089,"end":4267},"obj":"Sentence"},{"id":"T223","span":{"begin":4269,"end":4299},"obj":"Sentence"},{"id":"T224","span":{"begin":4300,"end":4459},"obj":"Sentence"},{"id":"T225","span":{"begin":4460,"end":4758},"obj":"Sentence"},{"id":"T226","span":{"begin":4759,"end":4928},"obj":"Sentence"},{"id":"T227","span":{"begin":4929,"end":5177},"obj":"Sentence"},{"id":"T228","span":{"begin":5178,"end":5230},"obj":"Sentence"},{"id":"T229","span":{"begin":5231,"end":5482},"obj":"Sentence"},{"id":"T230","span":{"begin":5483,"end":5624},"obj":"Sentence"},{"id":"T231","span":{"begin":5625,"end":5795},"obj":"Sentence"},{"id":"T232","span":{"begin":5796,"end":5986},"obj":"Sentence"},{"id":"T233","span":{"begin":5987,"end":6062},"obj":"Sentence"},{"id":"T234","span":{"begin":6063,"end":6253},"obj":"Sentence"},{"id":"T235","span":{"begin":6254,"end":6290},"obj":"Sentence"},{"id":"T236","span":{"begin":6291,"end":6369},"obj":"Sentence"},{"id":"T237","span":{"begin":6370,"end":6484},"obj":"Sentence"},{"id":"T238","span":{"begin":6485,"end":6642},"obj":"Sentence"},{"id":"T239","span":{"begin":6643,"end":6774},"obj":"Sentence"},{"id":"T240","span":{"begin":6775,"end":6989},"obj":"Sentence"},{"id":"T241","span":{"begin":6990,"end":7199},"obj":"Sentence"},{"id":"T242","span":{"begin":7200,"end":7282},"obj":"Sentence"},{"id":"T243","span":{"begin":7283,"end":7419},"obj":"Sentence"},{"id":"T244","span":{"begin":7420,"end":7681},"obj":"Sentence"},{"id":"T245","span":{"begin":7682,"end":7786},"obj":"Sentence"},{"id":"T246","span":{"begin":7787,"end":7856},"obj":"Sentence"},{"id":"T247","span":{"begin":7857,"end":8121},"obj":"Sentence"},{"id":"T248","span":{"begin":8123,"end":8142},"obj":"Sentence"},{"id":"T249","span":{"begin":8143,"end":8347},"obj":"Sentence"},{"id":"T250","span":{"begin":8348,"end":8469},"obj":"Sentence"},{"id":"T251","span":{"begin":8470,"end":8654},"obj":"Sentence"},{"id":"T252","span":{"begin":8655,"end":8915},"obj":"Sentence"},{"id":"T253","span":{"begin":8916,"end":9147},"obj":"Sentence"},{"id":"T254","span":{"begin":9148,"end":9267},"obj":"Sentence"},{"id":"T255","span":{"begin":9268,"end":9422},"obj":"Sentence"},{"id":"T256","span":{"begin":9423,"end":9429},"obj":"Sentence"},{"id":"T257","span":{"begin":9430,"end":9483},"obj":"Sentence"},{"id":"T258","span":{"begin":9484,"end":9727},"obj":"Sentence"},{"id":"T259","span":{"begin":9728,"end":9976},"obj":"Sentence"},{"id":"T260","span":{"begin":9977,"end":10147},"obj":"Sentence"},{"id":"T261","span":{"begin":10148,"end":10370},"obj":"Sentence"},{"id":"T262","span":{"begin":10371,"end":10633},"obj":"Sentence"}],"namespaces":[{"prefix":"_base","uri":"http://pubannotation.org/ontology/tao.owl#"}],"text":"4 Discussion\n\n4.1 Main findings\nThis is the first nationwide study in England investigating the effect of long-term exposure to NO2 and PM2.5 during 2014–2018 on COVID-19 mortality at LSOA level. The unadjusted models indicate that for every 1 μg/m3 increase in the long-term exposure to NO2 and PM2.5 the COVID-19 mortality risk increases. After considering the effect of confounding and spatial autocorrelation there is still some evidence of an effect, albeit is less strong, for NO2, while for PM2.5 there is larger uncertainty. The spatial relative risk has strong spatial patterns, identical for the different pollutants, potentially highlighting the effect of disease spread.\n\n4.2 Comparison with previous studies focusing on NO2\nOur study is comparable with previous studies in the US, England and the Netherlands assessing the long-term effect of NO2 in COVID-19 mortality. The study in the US focused on deaths reported by April 29, 2020, using 3122 counties. For the exposure, they calculated the mean of daily concentrations during 2010–2016 as modelled by a previously described ensemble machine learning model (R2 = 0.79) (Di et al., 2019a). They reported a 7.1% (95% Confidence Interval: 1.2%, 13.4%) increase in mortality per 4.5 ppb (1 ppb = 1.25 μg/m3) increase in NO2 after adjusting for confounders and spatial autocorrelation(Liang et al. 2020)(that is approximately 1.3% increase per 1 μg/m3). A study in England, with partly overlapping data as in our analysis, also reported a significant association between NO2 and COVID-19 mortality (p \u003c 0.05). For the analysis they focused on COVID-19 deaths reported in England up to April 10, 2020, used 317 LTLAs, and did not account for spatial autocorrelation (Travaglio et al. 2020). The study in the Netherlands using 335 municipalities, mean exposure during 2015–2019 and COVID-19 deaths up to June 5, 2020 reported 0.35 (95% CI: 0.04, 0.66) additional COVID-19 deaths for every 1 μg/m3 increase in NO2 after adjusting for confounders and certain spatial controls, such as transmission beyond the Dutch national borders (Cole et al. 2020). Since the mean number of deaths in their sample is 16.86, the above estimate translates to a 2.0% increase in the COVID-19 mortality for every 1 μg/m3 increase in NO2. An ONS report in England using 175 sampling units, 10-year averaged NO2 exposure (PCM) and COVID-19 deaths up to June 12, 2020 found a 0.6% (95% CI: −0.1%, 2.2%) increase in the COVID-19 mortality for every 1 μg/m3 increase in averaged NO2 exposure (Statistics 2020).\n\n4.3 Comparison with previous studies focusing on PM2.5\nOur study is comparable with previous studies assessing the long-term effect of PM2.5 on COVID-19 mortality. The aforementioned study in the US also assessed the effect of PM2.5 on COVID-19 mortality(Liang et al. 2020). Their exposure model was previously validated having an R2 = 0.89 for the annual estimates (Di et al. 2019b). The evidence for PM2.5 was weak, namely 10.8% (95% CI:-1.1%, 24.1%) per 3.4 μg/m3 increase in PM2.5 concentration (that is approximately 3.2% increase per 1 μg/m3) after adjusting for confounding and spatial autocorrelation. The ONS report in England found a 1% (95% CI: −3%, 6%) increase in the COVID-19 mortality for every 1 μg/m3 increase in the 10-year averaged PM2.5 exposure (Statistics 2020). Our study comes in contrast with another study in the US that used deaths reported until April 22nd, 2020 and counties as the geographical unit (Wu et al. 2020). For the exposure, they used previously validated monthly PM2.5 concentrations (R2 = 0.70) (Van Donkelaar et al. 2019) and averaged them during 2000 and 2016. After adjusting for confounding but not for spatial autocorrelation, they found an 11% (95% CI: 6%, 17%) increase in the COVID-19 death rate for an increase of 1 μg/m3 in PM2.5 concentration (Wu et al. 2020). Our study comes also in contrast with the study in the Netherlands that reported 2.3 (95% CI: 1.3, 3.0) additional COVID-19 deaths for an increase of 1 μg/m3 in the averaged long-term PM2.5 concentration (Cole et al. 2020). Having a mean number of deaths equal to 16.86, the above estimate translates to a 13.6% increase in the COVID-19 mortality rate for an increase of 1 μg/m3 in PM2.5 concentration.\n\n4.4 Strengths and limitations\nOur study is the first study to examine the association between long-term exposure to NO2 and PM2.5 and COVID-19 mortality at very high geographical precision. The spatial unit of our analysis is LSOAs, for which there are 32,844 in England (~130 000 km2), whereas previous studies have used 317 LTLAs or 175 sampling units in England, counties in the US (3 122 in an area ~9.8 million km2) and municipalities in the Netherlands (334 in an area ~41 500 km2). Such high-resolution allows capturing the localised geographical patterns of the pollutants but also ensures adequate confounding and spatial autocorrelation adjustment. Our study also covers, so far, the largest temporal window of the epidemic (capturing COVID-19 deaths attributable to the first wave, Supplemental Material Fig. S34), while most previous studies focused on the early to mid-stages of the first wave. This ensures better generalisability of the results. In addition, physical distancing and other public health interventions were introduced nationwide in England during the first epidemic, mitigating any distortion between air-pollution and COVID-19 mortality due to potential regional level differences. Our results are also consistent in a sensitivity analysis focusing on the pre-lockdown period, in the absence of public health interventions. Based on the scientific literature, we adjusted for several variables which would act as the confounders of the relationship between air pollution and COVID-19 mortality. Nevertheless, since the aetiology and the factors contributing to COVID-19 mortality are not fully understood yet, we included a spatial random effect to capture unknown spatial confounding. The spatial random effect was found to be a crucial component in the model. Not accounting for spatial autocorrelation, when spatial autocorrelation is present, is expected to give rise to narrower credible intervals and false positive effects (Lee and Sarran 2015).\nOur study has also some limitations. The downscaling procedure will likely inflate the reported credible intervals. However, this naturally reflects the uncertainty of the place of residence resulted from the downscaling approach. Although we consider small areas, the study is still an ecological one and thus the reported effects do not reflect individual associations (Wakefield 2008). Case fatality might have been a more appropriate metric for the analysis, since disease spread is accounted for in the denominator. Nevertheless, given the asymptomatic infections and the fact that number of reported infections is not a random sample of the general population, the number of COVID-19 cases per LTLA is not reliable at this stage. For the same reason, using the number of reported cases to adjust for disease progression and clustering of cases and deaths might not adequately capture disease progression and clustering of cases and deaths. However, part of this clustering was captured in the spatial autocorrelation term. We did not account for population mobility during 2014–2018 and assumed constant residence and thus levels of exposure to air-pollution. While this is a limitation, we believe that it would have a minimal impact on the results given that 1) the exposure period is relatively short and 2) almost 93% of the deaths in our dataset occurred in people 60 years or older (Supplemental Material Table S2). This comprises a population less likely to have moved during the past 5 years (Burgess and Quinio 2020). We also could not account for non-residential air-pollution exposure. Spatiotemporal variation in the strains of COVID-19 can introduce bias (Villeneuve and Goldberg 2020), however at the time of publication there was no evidence supporting that strain types can confound the relationship between COVID-19 mortality and air-pollution.\n\n4.5 Interpretation\nCompared to the previous studies, our results are the smallest in magnitude, likely because of the high geographical precision that allows more accurate confounding and spatial autocorrelation adjustment. In addition, we report weak evidence of an effect, which could also be due to lack of power and individual exposure data. Nevertheless, as for NO2 we find a high posterior probability of an effect on mortality, we argue that a potential explanation might be the mediation effect of pre-existing conditions. While in our analysis the inclusion of area-level prevalence of hypertension, diabetes and COPD did not change the results, the ecological nature of the pre-existing conditions data does not allow us to account for the mediation effect at the individual level. Our study focuses on the mortality after contracting SARS-CoV-2, however we cannot rule out individual susceptibility to becoming infected as an explanation to the uncertainty in the effect estimates (Villeneuve and Goldberg 2020). Such susceptibility can reflect immunosuppression, leading to later increases in inflammation (Edoardo Conticini et al. 2020) and thus worse prognosis, or even disease spread, as recent studies have suggested that PM2.5 can proliferate COVID-19 transmission (Bianconi et al. 2020).\nOur analysis captured strong spatial autocorrelation. The observed pattern could reflect residual variation from a potential inadequate covariate adjustment (including disease spread), spatial variation of pre-existing conditions, other unknown spatial confounders or a combination from all above. In a sensitivity analysis, we observed that the factors associated with disease transmission left the latent field unchanged (Supplemental Material Fig. S21), as did the inclusion of hypertension, diabetes and COPD (Supplemental Material Fig. S33). When we restricted the analysis to the pre-lockdown period, the latent field for both pollutants captured London and Birmingham, i.e. the cities with the first outbreaks. Considering the above, and the fact that COVID-19 is an infectious disease, we believe that large variation of Fig. 4 is likely due to disease spread, which is not adequately captured in the disease progression covariates.\nFig. 4 Median posterior spatial relative risk (exponential of the spatial autocorrelation term) and posterior probability that the spatial relative risk is larger than 1 for the models with NO2 and a spatial autocorrelation term and the fully adjusted NO2 model."}