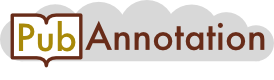
PMC:7786642 / 16343-21827
Annnotations
LitCovid-PD-HP
{"project":"LitCovid-PD-HP","denotations":[{"id":"T7","span":{"begin":4703,"end":4715},"obj":"Phenotype"},{"id":"T8","span":{"begin":4717,"end":4754},"obj":"Phenotype"},{"id":"T9","span":{"begin":4756,"end":4760},"obj":"Phenotype"}],"attributes":[{"id":"A7","pred":"hp_id","subj":"T7","obj":"http://purl.obolibrary.org/obo/HP_0000822"},{"id":"A8","pred":"hp_id","subj":"T8","obj":"http://purl.obolibrary.org/obo/HP_0006510"},{"id":"A9","pred":"hp_id","subj":"T9","obj":"http://purl.obolibrary.org/obo/HP_0006510"}],"text":"3 Results\n\n3.1 Study population\nWe identified 38,573 COVID-19 deaths with a laboratory confirmed test in England between 2nd March and 30th June (Fig. 1). The age, sex and ethnicity distribution of the deaths follows patterns reported previously (Supplemental Material Tables S2-3).\nFig. 1 Flowchart of the COVID-19 deaths.\n\n3.2 Exposure\nFig. 2 shows the population weighted air-pollutants at LSOA level in England. We observe that the localised variation of NO2, for instance due to the highways, is adequately captured at the spatial resolution of the LSOAs. The mean of NO2 is 16.17 μg/m3 and it varies from 2.99 μg/m3 in highly rural areas to 50.69 μg/m3 in the big urban centres (Fig. 2). The mean of PM2.5 is 9.84 μg/m3 with a smaller variation, 5.14–14.22 μg/m3 (Fig. 2).\nFig. 2 Population weighted exposure per LSOA.\n\n3.3 Confounders\nPlots and maps of the confounders can be found in Supplemental Material, Fig. S7-17.\n\n3.4 No2\nWe observe a 2.6% (95%CrI: 2.4%, 2.7%) increase in the COVID-19 mortality risk for every 1 μg/m3 increase in the long-term exposure to NO2, based on model 1 (Fig. 3 \u0026 Supplemental Material Table S4). There is still evidence of an effect, albeit smaller, once we adjust for spatial autocorrelation or confounders, with increases in the long-term exposure to NO2 of, respectively, 1.3% (95% CrI: 0.8%, 1.8%), 1.8% (95% CrI: 1.5%, 2.1%) for every 1 μg/m3. When we adjust for both autocorrelation and confounders the evidence is less strong, with estimates of 0.5% (95% CrI: −0.2%, 1.2%) for every 1 μg/m3 (Fig. 3 \u0026 Supplemental Material Table S4) and posterior probability of a positive effect reaching 0.93. The spatial relative risk in England varies from 0.24 (95% CrI: 0.08, 0.69) to 2.09 (95% CrI: 1.30, 3.11) in model 2 and from 0.30 (95% CrI: 0.10, 0.84) to 1.87 (95% CrI: 1.18, 2.93) in model 4, implying that the confounders explain very little of the observed variation (Fig. 3). The variation is more pronounced in the cities and suburban areas (with posterior probability higher than 1; Fig. 3).\nFig. 3 Density strips for the posterior of COVID-19 mortality relative risk with 1 μg/m3 increase in NO2 (top panel) and PM2.5 (bottom panel) averaged long-term exposure.\n\n3.5 Pm2.5\nWe observe a 4.4% (95% CrI: 3.7%, 5.1%) increase in the mortality risk for every 1 μg/m3 increase in the long-term exposure to PM2.5, based on model 1 (Fig. 3 \u0026 Supplemental Material Table S5). When we adjust for spatial autocorrelation the effect increases slightly but the credible intervals are wider, 5.4% (95% CrI: 2.5%, 8.4%), whereas it is similar when we adjust for confounding 4.9% (95% CrI: 3.7%, 6.2%) (Fig. 3 \u0026 Supplemental Material Table S5). The effect is weak when we account for confounders and spatial autocorrelation 1.4% (95% CrI: −2.1%, 5.1%) (Fig. 3 \u0026 Supplemental Material Table S5). The posterior probability of a positive effect is lower than observed for NO2, and equal to 0.78. The spatial relative risk follows similar patterns as the one reported in the models for NO2, with the posterior median relative risk varying from 0.24 (95% CrI: 0.12, 0.46) to 2.26 (95% CrI: 1.32, 3.85) in model 2 and from 0.30 (95% CrI: 0.15, 0.57) to 1.90 (95% CrI: 1.14, 3.17) in model 4 (Supplemental Material, Fig. S18).\n\n3.6 Sensitivity analyses\nWhen LTLAs are the main geographical unit for analysis, the results are consistent, but higher in magnitude, potentially due to inadequate covariate and spatial autocorrelation adjustment due to the coarse geographical resolution (Supplemental Material Tables S6-7, Fig. S19-20). Restricting the study period to March 23, 2020 (N = 698) also results in similar estimates for both pollutants, however the uncertainty is higher (Supplemental Material Tables S8-9, Fig. S21-22). The latent field of model 4, with NO2 as the pollutant, is similar to the latent fields of the models with and without the disease progression variables, with a correlation coefficient of 0.94 and 0.93 respectively (Supplemental Material Fig. S23). The use of quintiles of the pollutants justifies the linearity assumption (Supplemental Material Fig. S24). The results are consistent, but the evidence weaker, when suspected COVID-19 deaths are included (Supplemental Material Tables S10-11, Fig. S25-26). The results are also similar when we used a 3 or a 10-year mean of the air-pollutants concentration (Supplemental Material Fig. S27). The results are consistent when we fitted a zero-inflated Poisson (Supplemental Material Tables S12-13 and Fig. S28-29).\n\n3.7 Post-hoc analysis\nIn a post-hoc analysis we investigated if the evidence of an effect of NO2 on COVID-19 mortality can be attributed to pre-existing conditions. We selected hypertension, chronic obstructive pulmonary disease (COPD) and diabetes, because of 1) indications of previous literature that they increase the COVID-19 mortality risk (Williamson et al., 2020, Yang et al., 2020), 2) previous literature that suggest an effect with long-term exposure NO2 (Balti et al., 2014, Cai et al., 2016, Zhang et al., 2018) and 3) data availability. We retrieved prevalence data for these pre-existing conditions from PHE available at the GP practice level during 2018–2019 (https://fingertips.phe.org.uk/), Supplemental Material Fig. S30-32. The effect of NO2 remains similar, 0.6% (95% CrI: −0.1%, 1.3%) with the posterior probability being 0.94 whereas the spatial relative risk highlights the same geographical locations, Supplemental Material Fig. S33."}
LitCovid-PubTator
{"project":"LitCovid-PubTator","denotations":[{"id":"144","span":{"begin":309,"end":317},"obj":"Disease"},{"id":"145","span":{"begin":318,"end":324},"obj":"Disease"},{"id":"149","span":{"begin":55,"end":63},"obj":"Disease"},{"id":"150","span":{"begin":64,"end":70},"obj":"Disease"},{"id":"151","span":{"begin":204,"end":210},"obj":"Disease"},{"id":"153","span":{"begin":937,"end":940},"obj":"Chemical"},{"id":"157","span":{"begin":2147,"end":2150},"obj":"Chemical"},{"id":"158","span":{"begin":2089,"end":2097},"obj":"Disease"},{"id":"159","span":{"begin":2098,"end":2107},"obj":"Disease"},{"id":"164","span":{"begin":1076,"end":1079},"obj":"Chemical"},{"id":"165","span":{"begin":1298,"end":1301},"obj":"Chemical"},{"id":"166","span":{"begin":996,"end":1004},"obj":"Disease"},{"id":"167","span":{"begin":1005,"end":1014},"obj":"Disease"},{"id":"171","span":{"begin":2909,"end":2912},"obj":"Chemical"},{"id":"172","span":{"begin":3022,"end":3025},"obj":"Chemical"},{"id":"173","span":{"begin":2285,"end":2294},"obj":"Disease"},{"id":"177","span":{"begin":3797,"end":3800},"obj":"Chemical"},{"id":"178","span":{"begin":4188,"end":4196},"obj":"Disease"},{"id":"179","span":{"begin":4197,"end":4203},"obj":"Disease"},{"id":"191","span":{"begin":4619,"end":4622},"obj":"Chemical"},{"id":"192","span":{"begin":5221,"end":5224},"obj":"Chemical"},{"id":"193","span":{"begin":5284,"end":5287},"obj":"Chemical"},{"id":"194","span":{"begin":4626,"end":4634},"obj":"Disease"},{"id":"195","span":{"begin":4635,"end":4644},"obj":"Disease"},{"id":"196","span":{"begin":4703,"end":4715},"obj":"Disease"},{"id":"197","span":{"begin":4717,"end":4754},"obj":"Disease"},{"id":"198","span":{"begin":4756,"end":4760},"obj":"Disease"},{"id":"199","span":{"begin":4766,"end":4774},"obj":"Disease"},{"id":"200","span":{"begin":4848,"end":4856},"obj":"Disease"},{"id":"201","span":{"begin":4857,"end":4866},"obj":"Disease"}],"attributes":[{"id":"A144","pred":"tao:has_database_id","subj":"144","obj":"MESH:C000657245"},{"id":"A145","pred":"tao:has_database_id","subj":"145","obj":"MESH:D003643"},{"id":"A149","pred":"tao:has_database_id","subj":"149","obj":"MESH:C000657245"},{"id":"A150","pred":"tao:has_database_id","subj":"150","obj":"MESH:D003643"},{"id":"A151","pred":"tao:has_database_id","subj":"151","obj":"MESH:D003643"},{"id":"A158","pred":"tao:has_database_id","subj":"158","obj":"MESH:C000657245"},{"id":"A159","pred":"tao:has_database_id","subj":"159","obj":"MESH:D003643"},{"id":"A166","pred":"tao:has_database_id","subj":"166","obj":"MESH:C000657245"},{"id":"A167","pred":"tao:has_database_id","subj":"167","obj":"MESH:D003643"},{"id":"A173","pred":"tao:has_database_id","subj":"173","obj":"MESH:D003643"},{"id":"A178","pred":"tao:has_database_id","subj":"178","obj":"MESH:C000657245"},{"id":"A179","pred":"tao:has_database_id","subj":"179","obj":"MESH:D003643"},{"id":"A192","pred":"tao:has_database_id","subj":"192","obj":"MESH:D010649"},{"id":"A194","pred":"tao:has_database_id","subj":"194","obj":"MESH:C000657245"},{"id":"A195","pred":"tao:has_database_id","subj":"195","obj":"MESH:D003643"},{"id":"A196","pred":"tao:has_database_id","subj":"196","obj":"MESH:D006973"},{"id":"A197","pred":"tao:has_database_id","subj":"197","obj":"MESH:D029424"},{"id":"A198","pred":"tao:has_database_id","subj":"198","obj":"MESH:D029424"},{"id":"A199","pred":"tao:has_database_id","subj":"199","obj":"MESH:D003920"},{"id":"A200","pred":"tao:has_database_id","subj":"200","obj":"MESH:C000657245"},{"id":"A201","pred":"tao:has_database_id","subj":"201","obj":"MESH:D003643"}],"namespaces":[{"prefix":"Tax","uri":"https://www.ncbi.nlm.nih.gov/taxonomy/"},{"prefix":"MESH","uri":"https://id.nlm.nih.gov/mesh/"},{"prefix":"Gene","uri":"https://www.ncbi.nlm.nih.gov/gene/"},{"prefix":"CVCL","uri":"https://web.expasy.org/cellosaurus/CVCL_"}],"text":"3 Results\n\n3.1 Study population\nWe identified 38,573 COVID-19 deaths with a laboratory confirmed test in England between 2nd March and 30th June (Fig. 1). The age, sex and ethnicity distribution of the deaths follows patterns reported previously (Supplemental Material Tables S2-3).\nFig. 1 Flowchart of the COVID-19 deaths.\n\n3.2 Exposure\nFig. 2 shows the population weighted air-pollutants at LSOA level in England. We observe that the localised variation of NO2, for instance due to the highways, is adequately captured at the spatial resolution of the LSOAs. The mean of NO2 is 16.17 μg/m3 and it varies from 2.99 μg/m3 in highly rural areas to 50.69 μg/m3 in the big urban centres (Fig. 2). The mean of PM2.5 is 9.84 μg/m3 with a smaller variation, 5.14–14.22 μg/m3 (Fig. 2).\nFig. 2 Population weighted exposure per LSOA.\n\n3.3 Confounders\nPlots and maps of the confounders can be found in Supplemental Material, Fig. S7-17.\n\n3.4 No2\nWe observe a 2.6% (95%CrI: 2.4%, 2.7%) increase in the COVID-19 mortality risk for every 1 μg/m3 increase in the long-term exposure to NO2, based on model 1 (Fig. 3 \u0026 Supplemental Material Table S4). There is still evidence of an effect, albeit smaller, once we adjust for spatial autocorrelation or confounders, with increases in the long-term exposure to NO2 of, respectively, 1.3% (95% CrI: 0.8%, 1.8%), 1.8% (95% CrI: 1.5%, 2.1%) for every 1 μg/m3. When we adjust for both autocorrelation and confounders the evidence is less strong, with estimates of 0.5% (95% CrI: −0.2%, 1.2%) for every 1 μg/m3 (Fig. 3 \u0026 Supplemental Material Table S4) and posterior probability of a positive effect reaching 0.93. The spatial relative risk in England varies from 0.24 (95% CrI: 0.08, 0.69) to 2.09 (95% CrI: 1.30, 3.11) in model 2 and from 0.30 (95% CrI: 0.10, 0.84) to 1.87 (95% CrI: 1.18, 2.93) in model 4, implying that the confounders explain very little of the observed variation (Fig. 3). The variation is more pronounced in the cities and suburban areas (with posterior probability higher than 1; Fig. 3).\nFig. 3 Density strips for the posterior of COVID-19 mortality relative risk with 1 μg/m3 increase in NO2 (top panel) and PM2.5 (bottom panel) averaged long-term exposure.\n\n3.5 Pm2.5\nWe observe a 4.4% (95% CrI: 3.7%, 5.1%) increase in the mortality risk for every 1 μg/m3 increase in the long-term exposure to PM2.5, based on model 1 (Fig. 3 \u0026 Supplemental Material Table S5). When we adjust for spatial autocorrelation the effect increases slightly but the credible intervals are wider, 5.4% (95% CrI: 2.5%, 8.4%), whereas it is similar when we adjust for confounding 4.9% (95% CrI: 3.7%, 6.2%) (Fig. 3 \u0026 Supplemental Material Table S5). The effect is weak when we account for confounders and spatial autocorrelation 1.4% (95% CrI: −2.1%, 5.1%) (Fig. 3 \u0026 Supplemental Material Table S5). The posterior probability of a positive effect is lower than observed for NO2, and equal to 0.78. The spatial relative risk follows similar patterns as the one reported in the models for NO2, with the posterior median relative risk varying from 0.24 (95% CrI: 0.12, 0.46) to 2.26 (95% CrI: 1.32, 3.85) in model 2 and from 0.30 (95% CrI: 0.15, 0.57) to 1.90 (95% CrI: 1.14, 3.17) in model 4 (Supplemental Material, Fig. S18).\n\n3.6 Sensitivity analyses\nWhen LTLAs are the main geographical unit for analysis, the results are consistent, but higher in magnitude, potentially due to inadequate covariate and spatial autocorrelation adjustment due to the coarse geographical resolution (Supplemental Material Tables S6-7, Fig. S19-20). Restricting the study period to March 23, 2020 (N = 698) also results in similar estimates for both pollutants, however the uncertainty is higher (Supplemental Material Tables S8-9, Fig. S21-22). The latent field of model 4, with NO2 as the pollutant, is similar to the latent fields of the models with and without the disease progression variables, with a correlation coefficient of 0.94 and 0.93 respectively (Supplemental Material Fig. S23). The use of quintiles of the pollutants justifies the linearity assumption (Supplemental Material Fig. S24). The results are consistent, but the evidence weaker, when suspected COVID-19 deaths are included (Supplemental Material Tables S10-11, Fig. S25-26). The results are also similar when we used a 3 or a 10-year mean of the air-pollutants concentration (Supplemental Material Fig. S27). The results are consistent when we fitted a zero-inflated Poisson (Supplemental Material Tables S12-13 and Fig. S28-29).\n\n3.7 Post-hoc analysis\nIn a post-hoc analysis we investigated if the evidence of an effect of NO2 on COVID-19 mortality can be attributed to pre-existing conditions. We selected hypertension, chronic obstructive pulmonary disease (COPD) and diabetes, because of 1) indications of previous literature that they increase the COVID-19 mortality risk (Williamson et al., 2020, Yang et al., 2020), 2) previous literature that suggest an effect with long-term exposure NO2 (Balti et al., 2014, Cai et al., 2016, Zhang et al., 2018) and 3) data availability. We retrieved prevalence data for these pre-existing conditions from PHE available at the GP practice level during 2018–2019 (https://fingertips.phe.org.uk/), Supplemental Material Fig. S30-32. The effect of NO2 remains similar, 0.6% (95% CrI: −0.1%, 1.3%) with the posterior probability being 0.94 whereas the spatial relative risk highlights the same geographical locations, Supplemental Material Fig. S33."}
LitCovid-sentences
{"project":"LitCovid-sentences","denotations":[{"id":"T130","span":{"begin":0,"end":10},"obj":"Sentence"},{"id":"T131","span":{"begin":12,"end":33},"obj":"Sentence"},{"id":"T132","span":{"begin":34,"end":156},"obj":"Sentence"},{"id":"T133","span":{"begin":157,"end":284},"obj":"Sentence"},{"id":"T134","span":{"begin":285,"end":325},"obj":"Sentence"},{"id":"T135","span":{"begin":327,"end":340},"obj":"Sentence"},{"id":"T136","span":{"begin":341,"end":418},"obj":"Sentence"},{"id":"T137","span":{"begin":419,"end":563},"obj":"Sentence"},{"id":"T138","span":{"begin":564,"end":696},"obj":"Sentence"},{"id":"T139","span":{"begin":697,"end":781},"obj":"Sentence"},{"id":"T140","span":{"begin":782,"end":827},"obj":"Sentence"},{"id":"T141","span":{"begin":829,"end":845},"obj":"Sentence"},{"id":"T142","span":{"begin":846,"end":930},"obj":"Sentence"},{"id":"T143","span":{"begin":932,"end":940},"obj":"Sentence"},{"id":"T144","span":{"begin":941,"end":967},"obj":"Sentence"},{"id":"T145","span":{"begin":968,"end":1140},"obj":"Sentence"},{"id":"T146","span":{"begin":1141,"end":1334},"obj":"Sentence"},{"id":"T147","span":{"begin":1335,"end":1362},"obj":"Sentence"},{"id":"T148","span":{"begin":1363,"end":1393},"obj":"Sentence"},{"id":"T149","span":{"begin":1394,"end":1646},"obj":"Sentence"},{"id":"T150","span":{"begin":1647,"end":1710},"obj":"Sentence"},{"id":"T151","span":{"begin":1711,"end":1740},"obj":"Sentence"},{"id":"T152","span":{"begin":1741,"end":1787},"obj":"Sentence"},{"id":"T153","span":{"begin":1788,"end":1817},"obj":"Sentence"},{"id":"T154","span":{"begin":1818,"end":1927},"obj":"Sentence"},{"id":"T155","span":{"begin":1928,"end":2045},"obj":"Sentence"},{"id":"T156","span":{"begin":2046,"end":2216},"obj":"Sentence"},{"id":"T157","span":{"begin":2218,"end":2228},"obj":"Sentence"},{"id":"T158","span":{"begin":2229,"end":2256},"obj":"Sentence"},{"id":"T159","span":{"begin":2257,"end":2422},"obj":"Sentence"},{"id":"T160","span":{"begin":2423,"end":2548},"obj":"Sentence"},{"id":"T161","span":{"begin":2549,"end":2629},"obj":"Sentence"},{"id":"T162","span":{"begin":2630,"end":2684},"obj":"Sentence"},{"id":"T163","span":{"begin":2685,"end":2834},"obj":"Sentence"},{"id":"T164","span":{"begin":2835,"end":2932},"obj":"Sentence"},{"id":"T165","span":{"begin":2933,"end":3094},"obj":"Sentence"},{"id":"T166","span":{"begin":3095,"end":3124},"obj":"Sentence"},{"id":"T167","span":{"begin":3125,"end":3171},"obj":"Sentence"},{"id":"T168","span":{"begin":3172,"end":3201},"obj":"Sentence"},{"id":"T169","span":{"begin":3202,"end":3259},"obj":"Sentence"},{"id":"T170","span":{"begin":3261,"end":3286},"obj":"Sentence"},{"id":"T171","span":{"begin":3287,"end":3566},"obj":"Sentence"},{"id":"T172","span":{"begin":3567,"end":3762},"obj":"Sentence"},{"id":"T173","span":{"begin":3763,"end":4011},"obj":"Sentence"},{"id":"T174","span":{"begin":4012,"end":4119},"obj":"Sentence"},{"id":"T175","span":{"begin":4120,"end":4268},"obj":"Sentence"},{"id":"T176","span":{"begin":4269,"end":4402},"obj":"Sentence"},{"id":"T177","span":{"begin":4403,"end":4523},"obj":"Sentence"},{"id":"T178","span":{"begin":4525,"end":4547},"obj":"Sentence"},{"id":"T179","span":{"begin":4548,"end":4690},"obj":"Sentence"},{"id":"T180","span":{"begin":4691,"end":5076},"obj":"Sentence"},{"id":"T181","span":{"begin":5077,"end":5269},"obj":"Sentence"},{"id":"T182","span":{"begin":5270,"end":5484},"obj":"Sentence"}],"namespaces":[{"prefix":"_base","uri":"http://pubannotation.org/ontology/tao.owl#"}],"text":"3 Results\n\n3.1 Study population\nWe identified 38,573 COVID-19 deaths with a laboratory confirmed test in England between 2nd March and 30th June (Fig. 1). The age, sex and ethnicity distribution of the deaths follows patterns reported previously (Supplemental Material Tables S2-3).\nFig. 1 Flowchart of the COVID-19 deaths.\n\n3.2 Exposure\nFig. 2 shows the population weighted air-pollutants at LSOA level in England. We observe that the localised variation of NO2, for instance due to the highways, is adequately captured at the spatial resolution of the LSOAs. The mean of NO2 is 16.17 μg/m3 and it varies from 2.99 μg/m3 in highly rural areas to 50.69 μg/m3 in the big urban centres (Fig. 2). The mean of PM2.5 is 9.84 μg/m3 with a smaller variation, 5.14–14.22 μg/m3 (Fig. 2).\nFig. 2 Population weighted exposure per LSOA.\n\n3.3 Confounders\nPlots and maps of the confounders can be found in Supplemental Material, Fig. S7-17.\n\n3.4 No2\nWe observe a 2.6% (95%CrI: 2.4%, 2.7%) increase in the COVID-19 mortality risk for every 1 μg/m3 increase in the long-term exposure to NO2, based on model 1 (Fig. 3 \u0026 Supplemental Material Table S4). There is still evidence of an effect, albeit smaller, once we adjust for spatial autocorrelation or confounders, with increases in the long-term exposure to NO2 of, respectively, 1.3% (95% CrI: 0.8%, 1.8%), 1.8% (95% CrI: 1.5%, 2.1%) for every 1 μg/m3. When we adjust for both autocorrelation and confounders the evidence is less strong, with estimates of 0.5% (95% CrI: −0.2%, 1.2%) for every 1 μg/m3 (Fig. 3 \u0026 Supplemental Material Table S4) and posterior probability of a positive effect reaching 0.93. The spatial relative risk in England varies from 0.24 (95% CrI: 0.08, 0.69) to 2.09 (95% CrI: 1.30, 3.11) in model 2 and from 0.30 (95% CrI: 0.10, 0.84) to 1.87 (95% CrI: 1.18, 2.93) in model 4, implying that the confounders explain very little of the observed variation (Fig. 3). The variation is more pronounced in the cities and suburban areas (with posterior probability higher than 1; Fig. 3).\nFig. 3 Density strips for the posterior of COVID-19 mortality relative risk with 1 μg/m3 increase in NO2 (top panel) and PM2.5 (bottom panel) averaged long-term exposure.\n\n3.5 Pm2.5\nWe observe a 4.4% (95% CrI: 3.7%, 5.1%) increase in the mortality risk for every 1 μg/m3 increase in the long-term exposure to PM2.5, based on model 1 (Fig. 3 \u0026 Supplemental Material Table S5). When we adjust for spatial autocorrelation the effect increases slightly but the credible intervals are wider, 5.4% (95% CrI: 2.5%, 8.4%), whereas it is similar when we adjust for confounding 4.9% (95% CrI: 3.7%, 6.2%) (Fig. 3 \u0026 Supplemental Material Table S5). The effect is weak when we account for confounders and spatial autocorrelation 1.4% (95% CrI: −2.1%, 5.1%) (Fig. 3 \u0026 Supplemental Material Table S5). The posterior probability of a positive effect is lower than observed for NO2, and equal to 0.78. The spatial relative risk follows similar patterns as the one reported in the models for NO2, with the posterior median relative risk varying from 0.24 (95% CrI: 0.12, 0.46) to 2.26 (95% CrI: 1.32, 3.85) in model 2 and from 0.30 (95% CrI: 0.15, 0.57) to 1.90 (95% CrI: 1.14, 3.17) in model 4 (Supplemental Material, Fig. S18).\n\n3.6 Sensitivity analyses\nWhen LTLAs are the main geographical unit for analysis, the results are consistent, but higher in magnitude, potentially due to inadequate covariate and spatial autocorrelation adjustment due to the coarse geographical resolution (Supplemental Material Tables S6-7, Fig. S19-20). Restricting the study period to March 23, 2020 (N = 698) also results in similar estimates for both pollutants, however the uncertainty is higher (Supplemental Material Tables S8-9, Fig. S21-22). The latent field of model 4, with NO2 as the pollutant, is similar to the latent fields of the models with and without the disease progression variables, with a correlation coefficient of 0.94 and 0.93 respectively (Supplemental Material Fig. S23). The use of quintiles of the pollutants justifies the linearity assumption (Supplemental Material Fig. S24). The results are consistent, but the evidence weaker, when suspected COVID-19 deaths are included (Supplemental Material Tables S10-11, Fig. S25-26). The results are also similar when we used a 3 or a 10-year mean of the air-pollutants concentration (Supplemental Material Fig. S27). The results are consistent when we fitted a zero-inflated Poisson (Supplemental Material Tables S12-13 and Fig. S28-29).\n\n3.7 Post-hoc analysis\nIn a post-hoc analysis we investigated if the evidence of an effect of NO2 on COVID-19 mortality can be attributed to pre-existing conditions. We selected hypertension, chronic obstructive pulmonary disease (COPD) and diabetes, because of 1) indications of previous literature that they increase the COVID-19 mortality risk (Williamson et al., 2020, Yang et al., 2020), 2) previous literature that suggest an effect with long-term exposure NO2 (Balti et al., 2014, Cai et al., 2016, Zhang et al., 2018) and 3) data availability. We retrieved prevalence data for these pre-existing conditions from PHE available at the GP practice level during 2018–2019 (https://fingertips.phe.org.uk/), Supplemental Material Fig. S30-32. The effect of NO2 remains similar, 0.6% (95% CrI: −0.1%, 1.3%) with the posterior probability being 0.94 whereas the spatial relative risk highlights the same geographical locations, Supplemental Material Fig. S33."}