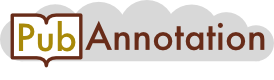
PMC:7738161 / 7146-17893
Annnotations
LitCovid-PubTator
{"project":"LitCovid-PubTator","denotations":[{"id":"57","span":{"begin":579,"end":587},"obj":"Species"},{"id":"58","span":{"begin":660,"end":666},"obj":"Species"},{"id":"59","span":{"begin":75,"end":91},"obj":"Disease"},{"id":"60","span":{"begin":712,"end":732},"obj":"Disease"},{"id":"61","span":{"begin":1011,"end":1019},"obj":"Disease"},{"id":"63","span":{"begin":1272,"end":1288},"obj":"Disease"},{"id":"67","span":{"begin":1724,"end":1726},"obj":"Chemical"},{"id":"68","span":{"begin":1728,"end":1730},"obj":"Chemical"},{"id":"69","span":{"begin":1733,"end":1735},"obj":"Chemical"},{"id":"72","span":{"begin":2328,"end":2334},"obj":"Species"},{"id":"73","span":{"begin":2390,"end":2396},"obj":"Species"},{"id":"75","span":{"begin":3701,"end":3706},"obj":"Disease"},{"id":"77","span":{"begin":5254,"end":5262},"obj":"Disease"},{"id":"79","span":{"begin":5922,"end":5931},"obj":"Disease"},{"id":"81","span":{"begin":6815,"end":6825},"obj":"Disease"},{"id":"83","span":{"begin":7239,"end":7248},"obj":"Disease"},{"id":"85","span":{"begin":7909,"end":7915},"obj":"Species"}],"attributes":[{"id":"A57","pred":"tao:has_database_id","subj":"57","obj":"Tax:9606"},{"id":"A58","pred":"tao:has_database_id","subj":"58","obj":"Tax:9606"},{"id":"A59","pred":"tao:has_database_id","subj":"59","obj":"OMIM:176500"},{"id":"A60","pred":"tao:has_database_id","subj":"60","obj":"MESH:D012818"},{"id":"A61","pred":"tao:has_database_id","subj":"61","obj":"MESH:D007239"},{"id":"A63","pred":"tao:has_database_id","subj":"63","obj":"OMIM:176500"},{"id":"A67","pred":"tao:has_database_id","subj":"67","obj":"MESH:D014529"},{"id":"A68","pred":"tao:has_database_id","subj":"68","obj":"MESH:D014529"},{"id":"A72","pred":"tao:has_database_id","subj":"72","obj":"Tax:9606"},{"id":"A73","pred":"tao:has_database_id","subj":"73","obj":"Tax:9606"},{"id":"A75","pred":"tao:has_database_id","subj":"75","obj":"MESH:C000657245"},{"id":"A77","pred":"tao:has_database_id","subj":"77","obj":"MESH:C000657245"},{"id":"A79","pred":"tao:has_database_id","subj":"79","obj":"MESH:D007239"},{"id":"A81","pred":"tao:has_database_id","subj":"81","obj":"MESH:D007239"},{"id":"A83","pred":"tao:has_database_id","subj":"83","obj":"MESH:D007239"},{"id":"A85","pred":"tao:has_database_id","subj":"85","obj":"Tax:9606"}],"namespaces":[{"prefix":"Tax","uri":"https://www.ncbi.nlm.nih.gov/taxonomy/"},{"prefix":"MESH","uri":"https://id.nlm.nih.gov/mesh/"},{"prefix":"Gene","uri":"https://www.ncbi.nlm.nih.gov/gene/"},{"prefix":"CVCL","uri":"https://web.expasy.org/cellosaurus/CVCL_"}],"text":"Methods\n\nData\nWe fit the physical distancing model to case-count data from British Columbia from March 1, 2020 (when a total of eight cases had been detected in the province) to April 11, 2020 at which time 1445 cases had been confirmed. These data are available in press releases from the BC Centre for Disease Control (BCCDC) [10], from the public data dashboard [17], and from the code repository associated with this paper. Testing procedures were adapted over the course of the outbreak. In particular, lab testing criteria were changed on March 16 to focus on hospitalized patients, healthcare workers, long-term care facility residents/staff, and those people part of an existing cluster and experiencing respiratory symptoms. This led to high variability in case counts in the surrounding days with some large jumps in the number of identified cases. We accounted for this in the model by adjusting the testing fraction ψr to accommodate widening the testing pool and thereby increasing the fraction of infected individuals being tested (Table 1). There was also variability in the daily testing rate. During March, the daily number of completed tests ranged from approximately 100 to 3500, and did not strictly increase over time.\nTable 1 Values and sources for British Columbia parameterization of the model (see Supplemental Methods and Table B in S1 Text for other jurisdictions).\nThe duration of the infectious period is shorter than the duration of severe illness, accounting for self-isolation and less severe illnesses. The quarantine parameter q reflects approximately 1/5 of severe cases either ceasing to transmit due to hospitalization or completely self-isolating. The model depends on the combination ur/(ur + ud), the fraction engaged in physical distancing, estimated from the survey data cited above. The testing patterns have changed over time, with laboratories increasing the numbers of tests on approximately March 14 (motivating our change in ψr).\nSymbol Definition Specified/fitted value Justification\nN Population size 5,100,000 [23]\nD Mean duration of the infectious period 5 days [24, 25]\nk 1 (time to infectiousness)−1 (E1 to E2) 0.2 days−1 [26–28]\nk 2 (time period of pre-symptomatic transmissibility)−1 (E2 to I) 1 days−1 [27, 28]\nq Quarantine rate 0.05 [29]\nu d Rate of people moving to physical distancing 0.1 [20]\nu r Rate of people returning from physical distancing 0.02 [20]\nψ r Proportion of anticipated cases on day r that are tested and reported 0.1 Before March 14\n0.3 On and after March 14\nShape Weibull parameter in delay-to-reporting distribution 1.73 (1.60–1.86 95% CI) Fit to data from Fig 1B\nScale Weibull parameter in delay-to-reporting distribution 9.85 (9.30–10.46 95% CI) Fit to data from Fig 1B\nR 0b Basic reproductive number 2.95 (2.88–3.02 95% CI) Fit to data from Fig 1C\nf 2 Fraction of normal contacts during physical distancing 0.22 (0.08– 0.36 95% CI) Fit to data from Fig 1C\nϕ Inverse dispersion from negative binomial (NB2) observation model 6.86 (3.39–12.37 95% CI) Fit to data from Fig 1C For some confirmed cases in BC, estimates of the date of symptom onset are available (Fig 1). We used the delays between symptom onset and cases being reported to parameterize the physical distancing model. In this case-specific data set there were only seven cases reported before February 29, and a decline in reported cases after April 2 (Fig 1). Therefore we used only the 535 cases in the case-specific data that were reported between these dates to parameterize the delay part of the model. For California (CA), New York (NY), and Florida (FL) we used reported case and testing data from The COVID Tracking Project, and assumed a BC-like delay between symptom onset and case reporting. For New Zealand (NZ) we used reported cases from the NZ Ministry of Health [18], and A. Lustig and M. Plank (pers. comm.) fit the delay distribution to NZ case-reporting data using our package [19], since these data are not publicly available (see Supplemental Methods in S1 Text).\nFor our main analysis with BC, we fit our model to data until April 11, 2020. When demonstrating the application of our model to other jurisdictions, we included data until May 6 or 7, 2020, the date we completed this portion of the analysis and before these jurisdictions had begun relaxing physical distancing measures. An outbreak at a poultry plant in BC, combined with an expansion of testing in mid April, meant that in order to extend the BC model to May 6 or 7, we would have had to introduce changes to the methodology that would not be straightforward and would not be possible in other jurisdictions. These include, for example, modelling the poultry workforce’s interventions and contacts and the links between the outbreak and general community transmission, in concert with differential testing. We therefore limited the data used for the BC model to April 11.\nWe motivated the structure of our model based on a survey conducted by the Angus Reid Institute to examine how physical distancing measures changed behaviour in Canada (March 20–23, 2020; n = 1664; [20]). Responses indicated that there was a subset of the population believing that the response to the COVID-19 epidemic was “overblown”, who were less willing than others to engage in distancing behaviours. This motivated treating the distancing and non-distancing compartments of our model separately and assuming that ∼80% of individuals were able and willing to physically distance. We used the timing of known government interventions to inform the timing of behavioural changes, and verified these dates against publicly available mobility data for each region [21].\n\nEpidemiological model\nWe used a susceptible-exposed-infectious-recovered (SEIR) model. Our model allows for self-isolation and quarantine through a quarantine compartment and a reduced duration of infection (compared to the clinical course of disease). We modelled a fixed portion of the population that was able to participate in physical distancing; each of the SEIR compartments has an analogous compartment in the distancing group (Fig 2; Table 1; Supplemental Methods in S1 Text).\nFig 2 Schematic of the epidemiological model.\nCompartments are: susceptible to the virus (S); exposed (E1); exposed, pre-symptomatic, and infectious (E2); symptomatic and infectious (I); quarantined (Q); and recovered or deceased (R). Recovered individuals are assumed to be immune. The model includes analogous variables for individuals practising physical distancing: Sd, E1d, E2d, Id, Qd, and Rd. Solid arrows represent flow of individuals between compartments at rates indicated by the mathematical terms (see Supplement for full definitions). Dashed lines show which compartments contribute to new infections. An individual in some compartment X can begin distancing and move to the corresponding compartment Xd at rate ud. The reverse transition occurs at rate ur. The model quickly settles on a fraction e = ud/(ud + ur) participating in distancing, and dynamics depend on this fraction, rather than on the rates ud and ur. The physical distancing compartments contribute a reduced amount (a fraction f) to the force of infection, with f = 1 representing no physical distancing. We made f time-dependent to represent changes in physical distancing. We modelled the change in physical distancing by a simple linear function whereby physical distancing increases (f(t) decreases from 1 to a final value f2, which we estimated) over one week between March 15 (t = t1) and March 22 (t = t2) in BC, and as informed by policy and mobility data in the other jurisdictions (Table B in S1 Text; Eq. 3 in S1 Text). We fixed f(t) = f2 until the final day of observed data and changed f(t) for any future days to represent different scenarios regarding relaxation of physical distancing.\nThe number of people per day who become symptomatic is the number per day moving from the pre-symptomatic E2 and E2d compartments to the symptomatic I and Id compartments (Fig 2). Due to the delay between symptom onset and reporting, the model’s predicted number of reported cases, μr, on day r is comprised of contributions from previous days weighted by a delay, which we estimate. We used a negative binomial model combined with information about testing changes over time to form a likelihood function, linking observed cases to the model (S1 Text).\nThe proportion of anticipated cases on day r that are tested and reported varies over time due to changes in the testing protocols, lab capacity, and other factors. In our BC data, the number of tests performed each day jumped dramatically on March 14; we modelled this with a sharp increase in ψr on that date. We fit a random walk to this function as a supplementary analysis. In other jurisdictions we fixed ψr. We used a Weibull distribution for the delay function w(s), based on [22], and fit the shape and scale parameters using the case-specific data of reported cases and time of symptom onset (Fig 1), accounting for right truncation (S1 Text).\n\nEstimation\nWe used a Bayesian statistical model to condition our inference about R0b, f2, and expected case counts on the number of reported cases, where R0b is the basic reproductive number. We related the expected number of cases to the observations through a negative binomial observation model with dispersion parameter ϕ. We placed weakly informative priors on R0b, f2, and ϕ (S1 Text). Outside of BC, we estimated the start and end times of the ramp-up of social distancing using priors informed by policy and transit data (S1 Text).\nWe fit our models with Stan 2.19.1 [30, 31] and R 3.6.2 [32]; Stan implements the No-U-Turn Hamiltonian Markov chain Monte Carlo algorithm [33] for Bayesian statistical inference. In our main model run, we sampled from eight chains with 2000 iterations each and discarded the first 1000 iterations of each chain as warm-up. We assessed chain convergence visually via trace plots (Figure G in S1 Text) and via ensuring that R^≤1.01 (the potential scale reduction factor) and that ESS \u003e 200 (the effective sample size), as calculated by the rstan R package [31] (Tables C and D in S1 Text). We represent uncertainty via quantile-based credible intervals. We validated our approach using simulated data (S1 Text).\nWe developed two R packages for this analysis: ‘rightTruncation’ [19] and ‘covidseir’ [34]. ‘rightTruncation’ performs maximum likelihood estimates of delay distributions accounting for right truncation. We used this approach to estimate the time between symptom onset and reporting in BC and New Zealand. The ‘covidseir’ package facilitates the SEIR model fitting of case-count data in Stan."}
LitCovid-sentences
{"project":"LitCovid-sentences","denotations":[{"id":"T45","span":{"begin":0,"end":7},"obj":"Sentence"},{"id":"T46","span":{"begin":9,"end":13},"obj":"Sentence"},{"id":"T47","span":{"begin":14,"end":237},"obj":"Sentence"},{"id":"T48","span":{"begin":238,"end":427},"obj":"Sentence"},{"id":"T49","span":{"begin":428,"end":492},"obj":"Sentence"},{"id":"T50","span":{"begin":493,"end":733},"obj":"Sentence"},{"id":"T51","span":{"begin":734,"end":858},"obj":"Sentence"},{"id":"T52","span":{"begin":859,"end":1055},"obj":"Sentence"},{"id":"T53","span":{"begin":1056,"end":1109},"obj":"Sentence"},{"id":"T54","span":{"begin":1110,"end":1239},"obj":"Sentence"},{"id":"T55","span":{"begin":1240,"end":1393},"obj":"Sentence"},{"id":"T56","span":{"begin":1394,"end":1536},"obj":"Sentence"},{"id":"T57","span":{"begin":1537,"end":1686},"obj":"Sentence"},{"id":"T58","span":{"begin":1687,"end":1826},"obj":"Sentence"},{"id":"T59","span":{"begin":1827,"end":1978},"obj":"Sentence"},{"id":"T60","span":{"begin":1979,"end":2036},"obj":"Sentence"},{"id":"T61","span":{"begin":2037,"end":2072},"obj":"Sentence"},{"id":"T62","span":{"begin":2073,"end":2132},"obj":"Sentence"},{"id":"T63","span":{"begin":2133,"end":2196},"obj":"Sentence"},{"id":"T64","span":{"begin":2197,"end":2283},"obj":"Sentence"},{"id":"T65","span":{"begin":2284,"end":2314},"obj":"Sentence"},{"id":"T66","span":{"begin":2315,"end":2375},"obj":"Sentence"},{"id":"T67","span":{"begin":2376,"end":2443},"obj":"Sentence"},{"id":"T68","span":{"begin":2444,"end":2541},"obj":"Sentence"},{"id":"T69","span":{"begin":2542,"end":2568},"obj":"Sentence"},{"id":"T70","span":{"begin":2569,"end":2678},"obj":"Sentence"},{"id":"T71","span":{"begin":2679,"end":2789},"obj":"Sentence"},{"id":"T72","span":{"begin":2790,"end":2871},"obj":"Sentence"},{"id":"T73","span":{"begin":2872,"end":2982},"obj":"Sentence"},{"id":"T74","span":{"begin":2983,"end":3196},"obj":"Sentence"},{"id":"T75","span":{"begin":3197,"end":3309},"obj":"Sentence"},{"id":"T76","span":{"begin":3310,"end":3452},"obj":"Sentence"},{"id":"T77","span":{"begin":3453,"end":3599},"obj":"Sentence"},{"id":"T78","span":{"begin":3600,"end":3794},"obj":"Sentence"},{"id":"T79","span":{"begin":3795,"end":3882},"obj":"Sentence"},{"id":"T80","span":{"begin":3883,"end":3896},"obj":"Sentence"},{"id":"T81","span":{"begin":3897,"end":4076},"obj":"Sentence"},{"id":"T82","span":{"begin":4077,"end":4154},"obj":"Sentence"},{"id":"T83","span":{"begin":4155,"end":4398},"obj":"Sentence"},{"id":"T84","span":{"begin":4399,"end":4688},"obj":"Sentence"},{"id":"T85","span":{"begin":4689,"end":4886},"obj":"Sentence"},{"id":"T86","span":{"begin":4887,"end":4951},"obj":"Sentence"},{"id":"T87","span":{"begin":4952,"end":5156},"obj":"Sentence"},{"id":"T88","span":{"begin":5157,"end":5358},"obj":"Sentence"},{"id":"T89","span":{"begin":5359,"end":5537},"obj":"Sentence"},{"id":"T90","span":{"begin":5538,"end":5723},"obj":"Sentence"},{"id":"T91","span":{"begin":5725,"end":5746},"obj":"Sentence"},{"id":"T92","span":{"begin":5747,"end":5811},"obj":"Sentence"},{"id":"T93","span":{"begin":5812,"end":5977},"obj":"Sentence"},{"id":"T94","span":{"begin":5978,"end":6210},"obj":"Sentence"},{"id":"T95","span":{"begin":6211,"end":6257},"obj":"Sentence"},{"id":"T96","span":{"begin":6258,"end":6446},"obj":"Sentence"},{"id":"T97","span":{"begin":6447,"end":6494},"obj":"Sentence"},{"id":"T98","span":{"begin":6495,"end":6581},"obj":"Sentence"},{"id":"T99","span":{"begin":6582,"end":6611},"obj":"Sentence"},{"id":"T100","span":{"begin":6612,"end":6759},"obj":"Sentence"},{"id":"T101","span":{"begin":6760,"end":6826},"obj":"Sentence"},{"id":"T102","span":{"begin":6827,"end":6940},"obj":"Sentence"},{"id":"T103","span":{"begin":6941,"end":6982},"obj":"Sentence"},{"id":"T104","span":{"begin":6983,"end":7142},"obj":"Sentence"},{"id":"T105","span":{"begin":7143,"end":7297},"obj":"Sentence"},{"id":"T106","span":{"begin":7298,"end":7367},"obj":"Sentence"},{"id":"T107","span":{"begin":7368,"end":7723},"obj":"Sentence"},{"id":"T108","span":{"begin":7724,"end":7894},"obj":"Sentence"},{"id":"T109","span":{"begin":7895,"end":8074},"obj":"Sentence"},{"id":"T110","span":{"begin":8075,"end":8278},"obj":"Sentence"},{"id":"T111","span":{"begin":8279,"end":8448},"obj":"Sentence"},{"id":"T112","span":{"begin":8449,"end":8613},"obj":"Sentence"},{"id":"T113","span":{"begin":8614,"end":8760},"obj":"Sentence"},{"id":"T114","span":{"begin":8761,"end":8827},"obj":"Sentence"},{"id":"T115","span":{"begin":8828,"end":8863},"obj":"Sentence"},{"id":"T116","span":{"begin":8864,"end":9102},"obj":"Sentence"},{"id":"T117","span":{"begin":9104,"end":9114},"obj":"Sentence"},{"id":"T118","span":{"begin":9115,"end":9295},"obj":"Sentence"},{"id":"T119","span":{"begin":9296,"end":9430},"obj":"Sentence"},{"id":"T120","span":{"begin":9431,"end":9495},"obj":"Sentence"},{"id":"T121","span":{"begin":9496,"end":9643},"obj":"Sentence"},{"id":"T122","span":{"begin":9644,"end":9823},"obj":"Sentence"},{"id":"T123","span":{"begin":9824,"end":9967},"obj":"Sentence"},{"id":"T124","span":{"begin":9968,"end":10232},"obj":"Sentence"},{"id":"T125","span":{"begin":10233,"end":10296},"obj":"Sentence"},{"id":"T126","span":{"begin":10297,"end":10354},"obj":"Sentence"},{"id":"T127","span":{"begin":10355,"end":10558},"obj":"Sentence"},{"id":"T128","span":{"begin":10559,"end":10660},"obj":"Sentence"},{"id":"T129","span":{"begin":10661,"end":10747},"obj":"Sentence"}],"namespaces":[{"prefix":"_base","uri":"http://pubannotation.org/ontology/tao.owl#"}],"text":"Methods\n\nData\nWe fit the physical distancing model to case-count data from British Columbia from March 1, 2020 (when a total of eight cases had been detected in the province) to April 11, 2020 at which time 1445 cases had been confirmed. These data are available in press releases from the BC Centre for Disease Control (BCCDC) [10], from the public data dashboard [17], and from the code repository associated with this paper. Testing procedures were adapted over the course of the outbreak. In particular, lab testing criteria were changed on March 16 to focus on hospitalized patients, healthcare workers, long-term care facility residents/staff, and those people part of an existing cluster and experiencing respiratory symptoms. This led to high variability in case counts in the surrounding days with some large jumps in the number of identified cases. We accounted for this in the model by adjusting the testing fraction ψr to accommodate widening the testing pool and thereby increasing the fraction of infected individuals being tested (Table 1). There was also variability in the daily testing rate. During March, the daily number of completed tests ranged from approximately 100 to 3500, and did not strictly increase over time.\nTable 1 Values and sources for British Columbia parameterization of the model (see Supplemental Methods and Table B in S1 Text for other jurisdictions).\nThe duration of the infectious period is shorter than the duration of severe illness, accounting for self-isolation and less severe illnesses. The quarantine parameter q reflects approximately 1/5 of severe cases either ceasing to transmit due to hospitalization or completely self-isolating. The model depends on the combination ur/(ur + ud), the fraction engaged in physical distancing, estimated from the survey data cited above. The testing patterns have changed over time, with laboratories increasing the numbers of tests on approximately March 14 (motivating our change in ψr).\nSymbol Definition Specified/fitted value Justification\nN Population size 5,100,000 [23]\nD Mean duration of the infectious period 5 days [24, 25]\nk 1 (time to infectiousness)−1 (E1 to E2) 0.2 days−1 [26–28]\nk 2 (time period of pre-symptomatic transmissibility)−1 (E2 to I) 1 days−1 [27, 28]\nq Quarantine rate 0.05 [29]\nu d Rate of people moving to physical distancing 0.1 [20]\nu r Rate of people returning from physical distancing 0.02 [20]\nψ r Proportion of anticipated cases on day r that are tested and reported 0.1 Before March 14\n0.3 On and after March 14\nShape Weibull parameter in delay-to-reporting distribution 1.73 (1.60–1.86 95% CI) Fit to data from Fig 1B\nScale Weibull parameter in delay-to-reporting distribution 9.85 (9.30–10.46 95% CI) Fit to data from Fig 1B\nR 0b Basic reproductive number 2.95 (2.88–3.02 95% CI) Fit to data from Fig 1C\nf 2 Fraction of normal contacts during physical distancing 0.22 (0.08– 0.36 95% CI) Fit to data from Fig 1C\nϕ Inverse dispersion from negative binomial (NB2) observation model 6.86 (3.39–12.37 95% CI) Fit to data from Fig 1C For some confirmed cases in BC, estimates of the date of symptom onset are available (Fig 1). We used the delays between symptom onset and cases being reported to parameterize the physical distancing model. In this case-specific data set there were only seven cases reported before February 29, and a decline in reported cases after April 2 (Fig 1). Therefore we used only the 535 cases in the case-specific data that were reported between these dates to parameterize the delay part of the model. For California (CA), New York (NY), and Florida (FL) we used reported case and testing data from The COVID Tracking Project, and assumed a BC-like delay between symptom onset and case reporting. For New Zealand (NZ) we used reported cases from the NZ Ministry of Health [18], and A. Lustig and M. Plank (pers. comm.) fit the delay distribution to NZ case-reporting data using our package [19], since these data are not publicly available (see Supplemental Methods in S1 Text).\nFor our main analysis with BC, we fit our model to data until April 11, 2020. When demonstrating the application of our model to other jurisdictions, we included data until May 6 or 7, 2020, the date we completed this portion of the analysis and before these jurisdictions had begun relaxing physical distancing measures. An outbreak at a poultry plant in BC, combined with an expansion of testing in mid April, meant that in order to extend the BC model to May 6 or 7, we would have had to introduce changes to the methodology that would not be straightforward and would not be possible in other jurisdictions. These include, for example, modelling the poultry workforce’s interventions and contacts and the links between the outbreak and general community transmission, in concert with differential testing. We therefore limited the data used for the BC model to April 11.\nWe motivated the structure of our model based on a survey conducted by the Angus Reid Institute to examine how physical distancing measures changed behaviour in Canada (March 20–23, 2020; n = 1664; [20]). Responses indicated that there was a subset of the population believing that the response to the COVID-19 epidemic was “overblown”, who were less willing than others to engage in distancing behaviours. This motivated treating the distancing and non-distancing compartments of our model separately and assuming that ∼80% of individuals were able and willing to physically distance. We used the timing of known government interventions to inform the timing of behavioural changes, and verified these dates against publicly available mobility data for each region [21].\n\nEpidemiological model\nWe used a susceptible-exposed-infectious-recovered (SEIR) model. Our model allows for self-isolation and quarantine through a quarantine compartment and a reduced duration of infection (compared to the clinical course of disease). We modelled a fixed portion of the population that was able to participate in physical distancing; each of the SEIR compartments has an analogous compartment in the distancing group (Fig 2; Table 1; Supplemental Methods in S1 Text).\nFig 2 Schematic of the epidemiological model.\nCompartments are: susceptible to the virus (S); exposed (E1); exposed, pre-symptomatic, and infectious (E2); symptomatic and infectious (I); quarantined (Q); and recovered or deceased (R). Recovered individuals are assumed to be immune. The model includes analogous variables for individuals practising physical distancing: Sd, E1d, E2d, Id, Qd, and Rd. Solid arrows represent flow of individuals between compartments at rates indicated by the mathematical terms (see Supplement for full definitions). Dashed lines show which compartments contribute to new infections. An individual in some compartment X can begin distancing and move to the corresponding compartment Xd at rate ud. The reverse transition occurs at rate ur. The model quickly settles on a fraction e = ud/(ud + ur) participating in distancing, and dynamics depend on this fraction, rather than on the rates ud and ur. The physical distancing compartments contribute a reduced amount (a fraction f) to the force of infection, with f = 1 representing no physical distancing. We made f time-dependent to represent changes in physical distancing. We modelled the change in physical distancing by a simple linear function whereby physical distancing increases (f(t) decreases from 1 to a final value f2, which we estimated) over one week between March 15 (t = t1) and March 22 (t = t2) in BC, and as informed by policy and mobility data in the other jurisdictions (Table B in S1 Text; Eq. 3 in S1 Text). We fixed f(t) = f2 until the final day of observed data and changed f(t) for any future days to represent different scenarios regarding relaxation of physical distancing.\nThe number of people per day who become symptomatic is the number per day moving from the pre-symptomatic E2 and E2d compartments to the symptomatic I and Id compartments (Fig 2). Due to the delay between symptom onset and reporting, the model’s predicted number of reported cases, μr, on day r is comprised of contributions from previous days weighted by a delay, which we estimate. We used a negative binomial model combined with information about testing changes over time to form a likelihood function, linking observed cases to the model (S1 Text).\nThe proportion of anticipated cases on day r that are tested and reported varies over time due to changes in the testing protocols, lab capacity, and other factors. In our BC data, the number of tests performed each day jumped dramatically on March 14; we modelled this with a sharp increase in ψr on that date. We fit a random walk to this function as a supplementary analysis. In other jurisdictions we fixed ψr. We used a Weibull distribution for the delay function w(s), based on [22], and fit the shape and scale parameters using the case-specific data of reported cases and time of symptom onset (Fig 1), accounting for right truncation (S1 Text).\n\nEstimation\nWe used a Bayesian statistical model to condition our inference about R0b, f2, and expected case counts on the number of reported cases, where R0b is the basic reproductive number. We related the expected number of cases to the observations through a negative binomial observation model with dispersion parameter ϕ. We placed weakly informative priors on R0b, f2, and ϕ (S1 Text). Outside of BC, we estimated the start and end times of the ramp-up of social distancing using priors informed by policy and transit data (S1 Text).\nWe fit our models with Stan 2.19.1 [30, 31] and R 3.6.2 [32]; Stan implements the No-U-Turn Hamiltonian Markov chain Monte Carlo algorithm [33] for Bayesian statistical inference. In our main model run, we sampled from eight chains with 2000 iterations each and discarded the first 1000 iterations of each chain as warm-up. We assessed chain convergence visually via trace plots (Figure G in S1 Text) and via ensuring that R^≤1.01 (the potential scale reduction factor) and that ESS \u003e 200 (the effective sample size), as calculated by the rstan R package [31] (Tables C and D in S1 Text). We represent uncertainty via quantile-based credible intervals. We validated our approach using simulated data (S1 Text).\nWe developed two R packages for this analysis: ‘rightTruncation’ [19] and ‘covidseir’ [34]. ‘rightTruncation’ performs maximum likelihood estimates of delay distributions accounting for right truncation. We used this approach to estimate the time between symptom onset and reporting in BC and New Zealand. The ‘covidseir’ package facilitates the SEIR model fitting of case-count data in Stan."}