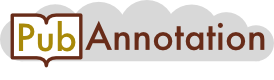
PMC:7738161 / 17895-26389
Annnotations
LitCovid-PubTator
{"project":"LitCovid-PubTator","denotations":[{"id":"87","span":{"begin":2113,"end":2122},"obj":"Disease"}],"attributes":[{"id":"A87","pred":"tao:has_database_id","subj":"87","obj":"MESH:D007239"}],"namespaces":[{"prefix":"Tax","uri":"https://www.ncbi.nlm.nih.gov/taxonomy/"},{"prefix":"MESH","uri":"https://id.nlm.nih.gov/mesh/"},{"prefix":"Gene","uri":"https://www.ncbi.nlm.nih.gov/gene/"},{"prefix":"CVCL","uri":"https://web.expasy.org/cellosaurus/CVCL_"}],"text":"Results\nWe found that, as of April 11, 2020, physical distancing had considerably reduced the contact rate in BC. We estimated that individuals practising physical distancing experienced approximately 0.22 (0.11–0.34 90% CI [credible interval]) of their normal contact rate, which was below the critical threshold (0.55; Fig 3; Figures E–H and Table C in S1 Text). The model described the count data well, with reported cases showing a peak in late March, approximately two weeks after the initiation of distancing measures (Fig 3A). The data were informative with respect to both main parameters with the posteriors distinctly different and more peaked than the priors (Fig 3B and 3D). We used a fixed value of e, the fraction engaged in distancing; this choice was motivated by the survey and behavioural data. If e were lower, the estimated strength of distancing would be higher to achieve the same case counts. We found this trade-off analytically using the basic reproduction number for the full model (Supplemental Methods and Figure A in S1 Text).\nFig 3 (A) Observed and estimated case counts, (C) estimated prevalence, and posterior estimates for (B) R0b and (D) fraction of normal contacts (f2) among those distancing. These projections do not account for introduced cases from other jurisdictions and they assume that distancing measures remain in place. The fraction of normal contacts is the model’s portion of contacts that remain among those who are engaged in physical distancing. In panel A, the blue line represents the posterior mean and the shaded ribbons represent 50% and 90% credible intervals on new observations. Dots and black lines represent the reported data. Grey region indicates the projection. In panel C, lines represent example draws from the posterior. In panels B and D, priors are shown in grey and posteriors in blue. In panel D, the dashed vertical line denotes the threshold above which an exponential increase in prevalence is expected (see Figure J in S1 Text). Note: Model prevalence depends on assumptions about underestimation, incubation period, and the duration of infection, none of which we can estimate well from these data (Figure M in S1 Text). Much higher values of the prevalence are consistent with our data. We found that with a shorter incubation period and duration of infectiousness, a lower reproduction number would fit the same overall growth rate, and conversely if the infectious duration and serial interval were longer, a higher reproduction number would be required but the fit to data would be similar (Figure K in S1 Text); this relationship is well known [35]. The conclusion that distancing measures reduced contact is robust to these alternatives (Figure K in S1 Text). The model depends on the fraction engaged in distancing, but not strongly on the rates ud and ur; Figure L in S1 Text illustrates that we obtained the same results with these rates increased by a factor of 10. We also explored the robustness to the unknown underestimation fractions (Figure M in S1 Text), and a random walk pattern in the fraction of cases sampled (Figure N in S1 Text); again, the data are consistent with a range of underestimation fractions, but the conclusion about the contact fraction is robust.\nOur estimates suggest that, as of April 11, 2020, some relaxation of current distancing measures in BC would have been possible without bringing the growth rate above zero, but if measures were relaxed too much (in the absence of monitoring and re-starting measures), the prevalence and case counts would begin to increase exponentially (Fig 4A and 4B), reaching high levels by June 2020 if distancing were to cease entirely (Figure I in S1 Text). These are illustrative scenarios only; public health responses with renewed or revised measures would likely be put in place rapidly were such rises to be observed. The speed of growth depends on how close the system is to the epidemic threshold (Figure J in S1 Text). If strong enough measures are not maintained, the model predicts a range of possible epidemic curves (Figure I in S1 Text) consistent with simple and complex published models [11, 12, 14].\nFig 4 Scenarios of relaxing distancing measures.\nDistancing measures are relaxed to (A) 60% (A) and (B) 80% levels of normal contacts and exponential growth is observed at moderate and rapid rates. (C, D) Two scenarios of cycling between physical distancing levels. Here, the percentage of normal contacts alternates between 80% (dark-grey shading) and 22% (light-grey shading) at (C) 3-week and (D) 4-week intervals. Reducing contacts to 22% of normal is approximately the level estimated by our model (Fig 3). Note the lag between changes in physical distancing and reported case counts. Figure description is otherwise the same as for Fig 3A and 3C. There has been interest in relaxing distancing measures and re-introducing them when a threshold has been reached, such as when intensive care capacity is nearly reached [36]. We did not explore a dynamic trigger, but did explore the behaviour when distancing measures are introduced and relaxed repeatedly (Fig 4C and 4D). If the relaxation period is such that the outbreak remains contained throughout (with an effective reproduction number less than one), then the prevalence would decline at alternating faster and slower rates. In a scenario of switching between the current mean estimate (22% of normal contacts) and 80% of normal contacts, reported cases rise, lagging the relaxation of distancing (Fig 4C and 4D). Illustrative simulations in which distancing alternates every three or four weeks allows an overall continued decline; however, the longer the period of relaxation, the more the prevalence is able to rise in between periods of distancing (Fig 4C and 4D). Control of delayed feedback systems is challenging [37], and ideally if a dynamic trigger such as reported cases or ICU admissions were to be used, monitoring would need to be as rapid as possible. Monitoring of distancing behaviour and population contact patterns would be important, in addition to monitoring cases.\nWe estimated the fraction of normal contact rate in five additional jurisdictions as of May 7, 2020 (Fig 5), and give numerical results as the median (and 90% CI) of the “contact ratio”: the ratio of the fraction of normal contacts to the threshold (above which prevalence increases) for each jurisdiction. We found that while New York had a high peak in reported cases, overall control there was strong as of May 7 and there may have been room to relax distancing measures while remaining below the threshold above which cases would be expected to increase, since the contact ratio is 0.60 (0.43–0.74). We estimated contact ratios of 0.86 (0.76–0.96) for Florida and 0.84 (0.79–0.90) for Washington as of May 7. These are not far below 1.0, and so any re-opening or relaxation of distancing measures would be expected to result in rising case numbers. In contrast, while some areas in California experienced strong distancing and mobility data suggest movement on par with Florida, New York, and Washington (Fig 5F), overall case counts in California had not declined as of May 7, and our model estimated that contacts were exceeding the critical threshold on average (contact ratio of 1.15 [1.07–1.23]). In contrast, New Zealand had extremely effective control measures and we estimated that nearly all contacts were removed among those distancing as of May 6, 2020, with a contact ratio of 0.22 (0.11–0.34). This left considerable room for re-opening (in concert with careful border measures and continued contact tracing and case finding).\nFig 5 Observed and estimated case counts for (A) New York, (B) Florida, (C) Washington, (D) California, and (E) New Zealand. Solid curves represent the posterior means and shaded ribbons represent 50% and 90% credible intervals of estimated counts. Dots and black lines represent the reported data. Inset histograms show the posterior distributions of the fraction of normal contacts (f2), with the vertical lines denoting the threshold above which an exponential increase in prevalence is expected (as in Fig 3D). (F) Reduction in movement from Google mobility transit-station data [21] colour-coded for each jurisdiction. Thin lines are raw data; thick lines are smoothed values from a generalized additive model. See Table B and Supplemental Methods in S1 Text for details on the regional modelling parameters and initialization."}
LitCovid-sentences
{"project":"LitCovid-sentences","denotations":[{"id":"T130","span":{"begin":0,"end":7},"obj":"Sentence"},{"id":"T131","span":{"begin":8,"end":113},"obj":"Sentence"},{"id":"T132","span":{"begin":114,"end":364},"obj":"Sentence"},{"id":"T133","span":{"begin":365,"end":533},"obj":"Sentence"},{"id":"T134","span":{"begin":534,"end":686},"obj":"Sentence"},{"id":"T135","span":{"begin":687,"end":812},"obj":"Sentence"},{"id":"T136","span":{"begin":813,"end":915},"obj":"Sentence"},{"id":"T137","span":{"begin":916,"end":1055},"obj":"Sentence"},{"id":"T138","span":{"begin":1056,"end":1229},"obj":"Sentence"},{"id":"T139","span":{"begin":1230,"end":1366},"obj":"Sentence"},{"id":"T140","span":{"begin":1367,"end":1497},"obj":"Sentence"},{"id":"T141","span":{"begin":1498,"end":1638},"obj":"Sentence"},{"id":"T142","span":{"begin":1639,"end":1688},"obj":"Sentence"},{"id":"T143","span":{"begin":1689,"end":1726},"obj":"Sentence"},{"id":"T144","span":{"begin":1727,"end":1788},"obj":"Sentence"},{"id":"T145","span":{"begin":1789,"end":1856},"obj":"Sentence"},{"id":"T146","span":{"begin":1857,"end":2004},"obj":"Sentence"},{"id":"T147","span":{"begin":2005,"end":2010},"obj":"Sentence"},{"id":"T148","span":{"begin":2011,"end":2197},"obj":"Sentence"},{"id":"T149","span":{"begin":2198,"end":2264},"obj":"Sentence"},{"id":"T150","span":{"begin":2265,"end":2631},"obj":"Sentence"},{"id":"T151","span":{"begin":2632,"end":2742},"obj":"Sentence"},{"id":"T152","span":{"begin":2743,"end":2952},"obj":"Sentence"},{"id":"T153","span":{"begin":2953,"end":3261},"obj":"Sentence"},{"id":"T154","span":{"begin":3262,"end":3709},"obj":"Sentence"},{"id":"T155","span":{"begin":3710,"end":3874},"obj":"Sentence"},{"id":"T156","span":{"begin":3875,"end":3978},"obj":"Sentence"},{"id":"T157","span":{"begin":3979,"end":4167},"obj":"Sentence"},{"id":"T158","span":{"begin":4168,"end":4217},"obj":"Sentence"},{"id":"T159","span":{"begin":4218,"end":4434},"obj":"Sentence"},{"id":"T160","span":{"begin":4435,"end":4586},"obj":"Sentence"},{"id":"T161","span":{"begin":4587,"end":4680},"obj":"Sentence"},{"id":"T162","span":{"begin":4681,"end":4758},"obj":"Sentence"},{"id":"T163","span":{"begin":4759,"end":4821},"obj":"Sentence"},{"id":"T164","span":{"begin":4822,"end":4997},"obj":"Sentence"},{"id":"T165","span":{"begin":4998,"end":5145},"obj":"Sentence"},{"id":"T166","span":{"begin":5146,"end":5354},"obj":"Sentence"},{"id":"T167","span":{"begin":5355,"end":5543},"obj":"Sentence"},{"id":"T168","span":{"begin":5544,"end":5798},"obj":"Sentence"},{"id":"T169","span":{"begin":5799,"end":5996},"obj":"Sentence"},{"id":"T170","span":{"begin":5997,"end":6116},"obj":"Sentence"},{"id":"T171","span":{"begin":6117,"end":6423},"obj":"Sentence"},{"id":"T172","span":{"begin":6424,"end":6720},"obj":"Sentence"},{"id":"T173","span":{"begin":6721,"end":6829},"obj":"Sentence"},{"id":"T174","span":{"begin":6830,"end":6969},"obj":"Sentence"},{"id":"T175","span":{"begin":6970,"end":7322},"obj":"Sentence"},{"id":"T176","span":{"begin":7323,"end":7527},"obj":"Sentence"},{"id":"T177","span":{"begin":7528,"end":7660},"obj":"Sentence"},{"id":"T178","span":{"begin":7661,"end":7786},"obj":"Sentence"},{"id":"T179","span":{"begin":7787,"end":7910},"obj":"Sentence"},{"id":"T180","span":{"begin":7911,"end":7960},"obj":"Sentence"},{"id":"T181","span":{"begin":7961,"end":8285},"obj":"Sentence"},{"id":"T182","span":{"begin":8286,"end":8377},"obj":"Sentence"},{"id":"T183","span":{"begin":8378,"end":8494},"obj":"Sentence"}],"namespaces":[{"prefix":"_base","uri":"http://pubannotation.org/ontology/tao.owl#"}],"text":"Results\nWe found that, as of April 11, 2020, physical distancing had considerably reduced the contact rate in BC. We estimated that individuals practising physical distancing experienced approximately 0.22 (0.11–0.34 90% CI [credible interval]) of their normal contact rate, which was below the critical threshold (0.55; Fig 3; Figures E–H and Table C in S1 Text). The model described the count data well, with reported cases showing a peak in late March, approximately two weeks after the initiation of distancing measures (Fig 3A). The data were informative with respect to both main parameters with the posteriors distinctly different and more peaked than the priors (Fig 3B and 3D). We used a fixed value of e, the fraction engaged in distancing; this choice was motivated by the survey and behavioural data. If e were lower, the estimated strength of distancing would be higher to achieve the same case counts. We found this trade-off analytically using the basic reproduction number for the full model (Supplemental Methods and Figure A in S1 Text).\nFig 3 (A) Observed and estimated case counts, (C) estimated prevalence, and posterior estimates for (B) R0b and (D) fraction of normal contacts (f2) among those distancing. These projections do not account for introduced cases from other jurisdictions and they assume that distancing measures remain in place. The fraction of normal contacts is the model’s portion of contacts that remain among those who are engaged in physical distancing. In panel A, the blue line represents the posterior mean and the shaded ribbons represent 50% and 90% credible intervals on new observations. Dots and black lines represent the reported data. Grey region indicates the projection. In panel C, lines represent example draws from the posterior. In panels B and D, priors are shown in grey and posteriors in blue. In panel D, the dashed vertical line denotes the threshold above which an exponential increase in prevalence is expected (see Figure J in S1 Text). Note: Model prevalence depends on assumptions about underestimation, incubation period, and the duration of infection, none of which we can estimate well from these data (Figure M in S1 Text). Much higher values of the prevalence are consistent with our data. We found that with a shorter incubation period and duration of infectiousness, a lower reproduction number would fit the same overall growth rate, and conversely if the infectious duration and serial interval were longer, a higher reproduction number would be required but the fit to data would be similar (Figure K in S1 Text); this relationship is well known [35]. The conclusion that distancing measures reduced contact is robust to these alternatives (Figure K in S1 Text). The model depends on the fraction engaged in distancing, but not strongly on the rates ud and ur; Figure L in S1 Text illustrates that we obtained the same results with these rates increased by a factor of 10. We also explored the robustness to the unknown underestimation fractions (Figure M in S1 Text), and a random walk pattern in the fraction of cases sampled (Figure N in S1 Text); again, the data are consistent with a range of underestimation fractions, but the conclusion about the contact fraction is robust.\nOur estimates suggest that, as of April 11, 2020, some relaxation of current distancing measures in BC would have been possible without bringing the growth rate above zero, but if measures were relaxed too much (in the absence of monitoring and re-starting measures), the prevalence and case counts would begin to increase exponentially (Fig 4A and 4B), reaching high levels by June 2020 if distancing were to cease entirely (Figure I in S1 Text). These are illustrative scenarios only; public health responses with renewed or revised measures would likely be put in place rapidly were such rises to be observed. The speed of growth depends on how close the system is to the epidemic threshold (Figure J in S1 Text). If strong enough measures are not maintained, the model predicts a range of possible epidemic curves (Figure I in S1 Text) consistent with simple and complex published models [11, 12, 14].\nFig 4 Scenarios of relaxing distancing measures.\nDistancing measures are relaxed to (A) 60% (A) and (B) 80% levels of normal contacts and exponential growth is observed at moderate and rapid rates. (C, D) Two scenarios of cycling between physical distancing levels. Here, the percentage of normal contacts alternates between 80% (dark-grey shading) and 22% (light-grey shading) at (C) 3-week and (D) 4-week intervals. Reducing contacts to 22% of normal is approximately the level estimated by our model (Fig 3). Note the lag between changes in physical distancing and reported case counts. Figure description is otherwise the same as for Fig 3A and 3C. There has been interest in relaxing distancing measures and re-introducing them when a threshold has been reached, such as when intensive care capacity is nearly reached [36]. We did not explore a dynamic trigger, but did explore the behaviour when distancing measures are introduced and relaxed repeatedly (Fig 4C and 4D). If the relaxation period is such that the outbreak remains contained throughout (with an effective reproduction number less than one), then the prevalence would decline at alternating faster and slower rates. In a scenario of switching between the current mean estimate (22% of normal contacts) and 80% of normal contacts, reported cases rise, lagging the relaxation of distancing (Fig 4C and 4D). Illustrative simulations in which distancing alternates every three or four weeks allows an overall continued decline; however, the longer the period of relaxation, the more the prevalence is able to rise in between periods of distancing (Fig 4C and 4D). Control of delayed feedback systems is challenging [37], and ideally if a dynamic trigger such as reported cases or ICU admissions were to be used, monitoring would need to be as rapid as possible. Monitoring of distancing behaviour and population contact patterns would be important, in addition to monitoring cases.\nWe estimated the fraction of normal contact rate in five additional jurisdictions as of May 7, 2020 (Fig 5), and give numerical results as the median (and 90% CI) of the “contact ratio”: the ratio of the fraction of normal contacts to the threshold (above which prevalence increases) for each jurisdiction. We found that while New York had a high peak in reported cases, overall control there was strong as of May 7 and there may have been room to relax distancing measures while remaining below the threshold above which cases would be expected to increase, since the contact ratio is 0.60 (0.43–0.74). We estimated contact ratios of 0.86 (0.76–0.96) for Florida and 0.84 (0.79–0.90) for Washington as of May 7. These are not far below 1.0, and so any re-opening or relaxation of distancing measures would be expected to result in rising case numbers. In contrast, while some areas in California experienced strong distancing and mobility data suggest movement on par with Florida, New York, and Washington (Fig 5F), overall case counts in California had not declined as of May 7, and our model estimated that contacts were exceeding the critical threshold on average (contact ratio of 1.15 [1.07–1.23]). In contrast, New Zealand had extremely effective control measures and we estimated that nearly all contacts were removed among those distancing as of May 6, 2020, with a contact ratio of 0.22 (0.11–0.34). This left considerable room for re-opening (in concert with careful border measures and continued contact tracing and case finding).\nFig 5 Observed and estimated case counts for (A) New York, (B) Florida, (C) Washington, (D) California, and (E) New Zealand. Solid curves represent the posterior means and shaded ribbons represent 50% and 90% credible intervals of estimated counts. Dots and black lines represent the reported data. Inset histograms show the posterior distributions of the fraction of normal contacts (f2), with the vertical lines denoting the threshold above which an exponential increase in prevalence is expected (as in Fig 3D). (F) Reduction in movement from Google mobility transit-station data [21] colour-coded for each jurisdiction. Thin lines are raw data; thick lines are smoothed values from a generalized additive model. See Table B and Supplemental Methods in S1 Text for details on the regional modelling parameters and initialization."}