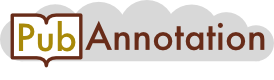
PMC:7738161 / 12871-16248
Annnotations
LitCovid-PubTator
{"project":"LitCovid-PubTator","denotations":[{"id":"79","span":{"begin":197,"end":206},"obj":"Disease"},{"id":"81","span":{"begin":1090,"end":1100},"obj":"Disease"},{"id":"83","span":{"begin":1514,"end":1523},"obj":"Disease"},{"id":"85","span":{"begin":2184,"end":2190},"obj":"Species"}],"attributes":[{"id":"A79","pred":"tao:has_database_id","subj":"79","obj":"MESH:D007239"},{"id":"A81","pred":"tao:has_database_id","subj":"81","obj":"MESH:D007239"},{"id":"A83","pred":"tao:has_database_id","subj":"83","obj":"MESH:D007239"},{"id":"A85","pred":"tao:has_database_id","subj":"85","obj":"Tax:9606"}],"namespaces":[{"prefix":"Tax","uri":"https://www.ncbi.nlm.nih.gov/taxonomy/"},{"prefix":"MESH","uri":"https://id.nlm.nih.gov/mesh/"},{"prefix":"Gene","uri":"https://www.ncbi.nlm.nih.gov/gene/"},{"prefix":"CVCL","uri":"https://web.expasy.org/cellosaurus/CVCL_"}],"text":"Epidemiological model\nWe used a susceptible-exposed-infectious-recovered (SEIR) model. Our model allows for self-isolation and quarantine through a quarantine compartment and a reduced duration of infection (compared to the clinical course of disease). We modelled a fixed portion of the population that was able to participate in physical distancing; each of the SEIR compartments has an analogous compartment in the distancing group (Fig 2; Table 1; Supplemental Methods in S1 Text).\nFig 2 Schematic of the epidemiological model.\nCompartments are: susceptible to the virus (S); exposed (E1); exposed, pre-symptomatic, and infectious (E2); symptomatic and infectious (I); quarantined (Q); and recovered or deceased (R). Recovered individuals are assumed to be immune. The model includes analogous variables for individuals practising physical distancing: Sd, E1d, E2d, Id, Qd, and Rd. Solid arrows represent flow of individuals between compartments at rates indicated by the mathematical terms (see Supplement for full definitions). Dashed lines show which compartments contribute to new infections. An individual in some compartment X can begin distancing and move to the corresponding compartment Xd at rate ud. The reverse transition occurs at rate ur. The model quickly settles on a fraction e = ud/(ud + ur) participating in distancing, and dynamics depend on this fraction, rather than on the rates ud and ur. The physical distancing compartments contribute a reduced amount (a fraction f) to the force of infection, with f = 1 representing no physical distancing. We made f time-dependent to represent changes in physical distancing. We modelled the change in physical distancing by a simple linear function whereby physical distancing increases (f(t) decreases from 1 to a final value f2, which we estimated) over one week between March 15 (t = t1) and March 22 (t = t2) in BC, and as informed by policy and mobility data in the other jurisdictions (Table B in S1 Text; Eq. 3 in S1 Text). We fixed f(t) = f2 until the final day of observed data and changed f(t) for any future days to represent different scenarios regarding relaxation of physical distancing.\nThe number of people per day who become symptomatic is the number per day moving from the pre-symptomatic E2 and E2d compartments to the symptomatic I and Id compartments (Fig 2). Due to the delay between symptom onset and reporting, the model’s predicted number of reported cases, μr, on day r is comprised of contributions from previous days weighted by a delay, which we estimate. We used a negative binomial model combined with information about testing changes over time to form a likelihood function, linking observed cases to the model (S1 Text).\nThe proportion of anticipated cases on day r that are tested and reported varies over time due to changes in the testing protocols, lab capacity, and other factors. In our BC data, the number of tests performed each day jumped dramatically on March 14; we modelled this with a sharp increase in ψr on that date. We fit a random walk to this function as a supplementary analysis. In other jurisdictions we fixed ψr. We used a Weibull distribution for the delay function w(s), based on [22], and fit the shape and scale parameters using the case-specific data of reported cases and time of symptom onset (Fig 1), accounting for right truncation (S1 Text)."}
LitCovid-sentences
{"project":"LitCovid-sentences","denotations":[{"id":"T91","span":{"begin":0,"end":21},"obj":"Sentence"},{"id":"T92","span":{"begin":22,"end":86},"obj":"Sentence"},{"id":"T93","span":{"begin":87,"end":252},"obj":"Sentence"},{"id":"T94","span":{"begin":253,"end":485},"obj":"Sentence"},{"id":"T95","span":{"begin":486,"end":532},"obj":"Sentence"},{"id":"T96","span":{"begin":533,"end":721},"obj":"Sentence"},{"id":"T97","span":{"begin":722,"end":769},"obj":"Sentence"},{"id":"T98","span":{"begin":770,"end":856},"obj":"Sentence"},{"id":"T99","span":{"begin":857,"end":886},"obj":"Sentence"},{"id":"T100","span":{"begin":887,"end":1034},"obj":"Sentence"},{"id":"T101","span":{"begin":1035,"end":1101},"obj":"Sentence"},{"id":"T102","span":{"begin":1102,"end":1215},"obj":"Sentence"},{"id":"T103","span":{"begin":1216,"end":1257},"obj":"Sentence"},{"id":"T104","span":{"begin":1258,"end":1417},"obj":"Sentence"},{"id":"T105","span":{"begin":1418,"end":1572},"obj":"Sentence"},{"id":"T106","span":{"begin":1573,"end":1642},"obj":"Sentence"},{"id":"T107","span":{"begin":1643,"end":1998},"obj":"Sentence"},{"id":"T108","span":{"begin":1999,"end":2169},"obj":"Sentence"},{"id":"T109","span":{"begin":2170,"end":2349},"obj":"Sentence"},{"id":"T110","span":{"begin":2350,"end":2553},"obj":"Sentence"},{"id":"T111","span":{"begin":2554,"end":2723},"obj":"Sentence"},{"id":"T112","span":{"begin":2724,"end":2888},"obj":"Sentence"},{"id":"T113","span":{"begin":2889,"end":3035},"obj":"Sentence"},{"id":"T114","span":{"begin":3036,"end":3102},"obj":"Sentence"},{"id":"T115","span":{"begin":3103,"end":3138},"obj":"Sentence"},{"id":"T116","span":{"begin":3139,"end":3377},"obj":"Sentence"}],"namespaces":[{"prefix":"_base","uri":"http://pubannotation.org/ontology/tao.owl#"}],"text":"Epidemiological model\nWe used a susceptible-exposed-infectious-recovered (SEIR) model. Our model allows for self-isolation and quarantine through a quarantine compartment and a reduced duration of infection (compared to the clinical course of disease). We modelled a fixed portion of the population that was able to participate in physical distancing; each of the SEIR compartments has an analogous compartment in the distancing group (Fig 2; Table 1; Supplemental Methods in S1 Text).\nFig 2 Schematic of the epidemiological model.\nCompartments are: susceptible to the virus (S); exposed (E1); exposed, pre-symptomatic, and infectious (E2); symptomatic and infectious (I); quarantined (Q); and recovered or deceased (R). Recovered individuals are assumed to be immune. The model includes analogous variables for individuals practising physical distancing: Sd, E1d, E2d, Id, Qd, and Rd. Solid arrows represent flow of individuals between compartments at rates indicated by the mathematical terms (see Supplement for full definitions). Dashed lines show which compartments contribute to new infections. An individual in some compartment X can begin distancing and move to the corresponding compartment Xd at rate ud. The reverse transition occurs at rate ur. The model quickly settles on a fraction e = ud/(ud + ur) participating in distancing, and dynamics depend on this fraction, rather than on the rates ud and ur. The physical distancing compartments contribute a reduced amount (a fraction f) to the force of infection, with f = 1 representing no physical distancing. We made f time-dependent to represent changes in physical distancing. We modelled the change in physical distancing by a simple linear function whereby physical distancing increases (f(t) decreases from 1 to a final value f2, which we estimated) over one week between March 15 (t = t1) and March 22 (t = t2) in BC, and as informed by policy and mobility data in the other jurisdictions (Table B in S1 Text; Eq. 3 in S1 Text). We fixed f(t) = f2 until the final day of observed data and changed f(t) for any future days to represent different scenarios regarding relaxation of physical distancing.\nThe number of people per day who become symptomatic is the number per day moving from the pre-symptomatic E2 and E2d compartments to the symptomatic I and Id compartments (Fig 2). Due to the delay between symptom onset and reporting, the model’s predicted number of reported cases, μr, on day r is comprised of contributions from previous days weighted by a delay, which we estimate. We used a negative binomial model combined with information about testing changes over time to form a likelihood function, linking observed cases to the model (S1 Text).\nThe proportion of anticipated cases on day r that are tested and reported varies over time due to changes in the testing protocols, lab capacity, and other factors. In our BC data, the number of tests performed each day jumped dramatically on March 14; we modelled this with a sharp increase in ψr on that date. We fit a random walk to this function as a supplementary analysis. In other jurisdictions we fixed ψr. We used a Weibull distribution for the delay function w(s), based on [22], and fit the shape and scale parameters using the case-specific data of reported cases and time of symptom onset (Fig 1), accounting for right truncation (S1 Text)."}