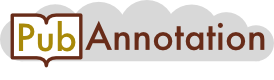
PMC:7572126 / 35474-50931
Annnotations
MyTest
{"project":"MyTest","denotations":[{"id":"33074097-30073906-26739287","span":{"begin":273,"end":277},"obj":"30073906"},{"id":"33074097-100702-26739288","span":{"begin":683,"end":687},"obj":"100702"},{"id":"33074097-100702-26739289","span":{"begin":2821,"end":2825},"obj":"100702"},{"id":"33074097-100702-26739290","span":{"begin":3276,"end":3280},"obj":"100702"},{"id":"33074097-18782390-26739291","span":{"begin":4511,"end":4515},"obj":"18782390"},{"id":"33074097-24695404-26739292","span":{"begin":4750,"end":4754},"obj":"24695404"},{"id":"33074097-26418763-26739293","span":{"begin":6527,"end":6531},"obj":"26418763"},{"id":"33074097-30377376-26739294","span":{"begin":7038,"end":7042},"obj":"30377376"},{"id":"33074097-26157614-26739295","span":{"begin":7272,"end":7276},"obj":"26157614"},{"id":"33074097-18027744-26739296","span":{"begin":8094,"end":8098},"obj":"18027744"},{"id":"33074097-18027744-26739297","span":{"begin":9661,"end":9665},"obj":"18027744"},{"id":"33074097-21702898-26739298","span":{"begin":10539,"end":10543},"obj":"21702898"}],"namespaces":[{"prefix":"_base","uri":"https://www.uniprot.org/uniprot/testbase"},{"prefix":"UniProtKB","uri":"https://www.uniprot.org/uniprot/"},{"prefix":"uniprot","uri":"https://www.uniprot.org/uniprotkb/"}],"text":"Materials and methods\n\nParticipants and setting\nThis observational study recruited participants from the Well Persons Health Check (WPHC). In 1997, the Australian government started a broad community health promotion and screening programme called the WPHC (Berger et al., 2020) conducted by the Torres and Cape Hospital and Health Service (TCHHS). Through WPHC several Indigenous (Aboriginal and/or Torres Strait Islander) communities in northern Queensland were screened annually for sexually transmitted infections and chronic cardiovascular as well as chronic metabolic conditions. In 2016, TCHHS collaborated with James Cook University (Zenadth Kes Health Partnership; Weiland, 1978) to conduct these surveys in two Australian island communities, Waiben and Mer. These islands are located in the Torres Strait Islands (TSI) archipelago, which lies in the waters separating the far northern continental Australia’s Cape York Peninsula (northern-most tip of Queensland) and the Western Province of Papua New Guinea, off the continent of Australia. Mer (Murray Island) is a 4.3 km2 remote island located on the eastern periphery of the Torres Strait region, 210 km northeast of Waiben and 340 km west of Port Moresby, with a population of 450 in 2016. The island is less connected with no known fast-food outlet. Waiben (Thursday Island) is a 3.5 km2 landmass located about 39 km north of Cape York Peninsula with a population of 2938 in 2016. It is the administrative and commercial center of the Torres Strait Island Region.\nIn addition to the health check that was a standard component of the WPHC, participants consented to have additional health data collected for research purposes. Verbal consent was used whenever a written one could not be obtained. In addition to individual consent, parent/legal guardian consent was obtained for all participants younger than 18 years. The study incorporated all WPHC participants who identified as Aboriginal and/or Torres Strait Islander and attended the screening in October, November, and December 2016. There were 214 individuals aged 15 years and older who participated in the October, November, and December 2016 WPHC and identified as Aboriginal and/or Torres Strait Islander. Participants were excluded due to insufficient survey information (n = 2) and or not providing consent to participate in the research component of the health check (n = 1). Only participants who provided stool samples for metagenomic sequencing (total n = 100; 50 participants from each site) were then included in the current analysis. The study was granted ethical approval by the Far North Queensland Human Research Ethics Committee (HREC/16/QCH/70–1059). In addition, written support from the local Community Council, Primary Health Care Service, and TCHHS was also provided (Weiland, 1978).\n\nParticipant demographic, dietary, and behavioral factors\nWe collected the island of residence, age, gender, physical activity, and daily alcohol consumption as well as smoking status. Dietary intake of seafood, takeaway foods, vegetables, fruits, and sugar-sweetened beverages was determined using a culturally-appropriate food frequency questionnaire that assessed food and beverage consumption through the use of non-leading questions (Weiland, 1978).\nAge, gender, height, weight, ethnicity, systolic blood pressure (SBP), diastolic blood pressure (DBP), known diabetes status, and known diabetes therapy were documented from WPHC clinical records. The mean arterial pressure (MAP) was calculated from brachial pressure values as follows:MAP=SBP+(2×DBP)3\nBody mass index (BMI; kg/m2) was computed from weight and height records. Hypertension was defined as SBP \u003e140 and/or DBP \u003e 90. Tobacco smoking and alcohol consumption were assessed using a standarized questionnaire.\n\nClinical sample collection\nA summary of key resources utilized, including sample collection kits, DNA isolation and library prep kits, DNA sequencing platform, and software is provided in Supplementary file 1e Each participant provided a sample of stool, blood, and urine. The stool was collected by participants using the OMNIgene GUT collection kit (DNA Genotek, Ontario, Canada), according to manufacturer’s instructions. Briefly, the stool was deposited in a sterile container, transferred into the OMNIgene tube using the provided spatula, mixed with the stabilizing liquid, and stored at room temperature until collection. Blood and urine were collected by TCHHS as described previously (Zhao et al., 2008).\n\nMeasurements of inflammatory and cardiometabolic markers\nConcentrations of various biomarkers in blood and/or urine were measured using chemistry multiplex immunoassays and enzyme-linked immunosorbent assays (ELISA; Bolger et al., 2014) as follows: Interleukins (IL) IL-1β, IL-2, IL-4, IL-5, IL-6, IL-10, IL-12p40, IL-12p70, IL-13, IL-15, IL-17A, IL-18, and IL-33; type II interferon (IFNγ), monocyte chemoattractant protein 1 (MCP1/CCL2), macrophage inflammatory protein (MIP-1α/CCL3), and tumor necrosis factor alpha (TNFα) were measured using Luminex High Sensitivity (8-plex and 9-plex) Human ProcartaPlex Panels. Serum C-reactive protein (CRP) was measured using the CRP Human ProcartaPlex Simplex Kit. Serum lipopolysaccharide-binding protein (LBP) was also measured using Human LBP ELISA Kit. Random blood glucose (RBG) and glycated hemoglobin or hemoglobin A1c (HbA1c) were measured using blood glucose meter and Haemoglobin A1c (HbA1c) (Human) ELISA Kit, respectively. All commercial assays were performed according to manufacturers’ instructions.\n\nDNA isolation and metagenomic sequencing\nMicrobial DNA was isolated from stool samples using DNeasy Powerlyzer PowerSoil Kit (Qiagen, Hilden, Germany). DNA yield was determined in Qubit Fluorometric Quantification using Quant-iT High-Sensitivity dsDNA Assay Kit. Libraries for shotgun metagenomic sequencing were fragmented using Nextera XT Library Preparation Kit v2 (Illumina Inc, San Diego, CA) and indexed using the Illumina Nextera XT Index Kit v2 according to manufacturer’s instructions. Amplicon libraries were then sequenced on the Illumina HiSeq 2500 platform at the SAHMRI David R Gunn Genomics Suite using Illumina HiSeq SBS 2 × 125bp v4 kit (Illumina Inc). Reads were converted into FASTQ format using bcl2fastq v.1.8.4. On average, 16,666,136 sequencing reads were obtained from each sample before quality filtering.\n\nBioinformatic processing\nPaired-end reads were adapter- and quality-filtered using Trimmomatic v.0.36 (Truong et al., 2015) with the following parameters: PE ILLUMINACLIP:TruSeq3-PE:3:30:10 LEADING:10 MINLEN:30 SLIDINGWINDOW: 4:30; HEADCROP:10. Samples included in the downstream analysis had at least 7.4 million paired-end reads of at least 91 bp in length after quality control. The quality of reads was checked before and after this process using FastQC v.0.11.5.\nUsing the quality-controlled paired-end reads, taxonomic profiling was performed using the metagenomic phylogenetic analysis (MetaPhlAn) v.2.6 (Franzosa et al., 2018), which uses a database of clade-specific markers to quantify microbiota constituents at the species and higher taxonomic levels. The generation of the abundance of metabolic pathways was performed using HUMAnN2 (Asnicar et al., 2015). Taxonomic and metabolic profiles for both islands were visualized using GraPhlAn v.0.9.7 (Whittaker, 1972). Sequenced metagenomic data were submitted to the National Center for Biotechnology Information (NCBI) Sequence Read Archive (SRA) under BioProject ID PRJNA503909. Summary of the quality-controlled fecal microbiome sequencing reads is shown in Supplementary file 1f.\n\nStatistical analysis\nStatistical analyses and graphical illustrations were accomplished in R software for statistical computing, Primer 7, GraphPad Prism 7, and Statistical Analysis Software (SAS/STAT) suite. Rare taxa, present in less than 40% of the participants at \u003c1% relative abundance, were excluded from the analysis.\nAlpha-diversity is defined as a local measure of average species diversity in a community. (Whittaker, 1972; Jost, 2007). To estimate the species alpha diversity in Mer and Waiben, we examined the microbial composition in each community using the vegan (Oksanen et al., 2018) package in R. (R Development Core Team, 2010) Accordingly, the 'estimate' function was used to calculate Chao1’s measure of richness; a measure of the number of species (or other taxonomic levels) present at a site. The 'diversity' function was used to estimate the Shannon-Wiener Index (H'); a measure of how many different types of species are present in the community. Species diversity takes into account both species richness and the dominance or evenness of the species. Evenness measures homogeneity in a community in terms of species relative abundances. A community in which all species are proportionately common is regarded as stable and has a high degree of evenness. Since vegan does not provide a function for Shannon’s evenness/equitability (E), we used a modified R function to estimated E by dividing the actual diversity (H') with the maximum possible diversity value (Hmax). The reasoning is, H' decreases when the species composition is more uneven and reaches a maximum with the highest evenness. Therefore, when all species are equally common, Hmax=lnS (where S is the total number of species), and E=H'÷Hmax. Differences in species composition within Mer and Waiben were analyzed in GraphPad using the Mann-Whitney U-test the with FDR multiple-testing adjustment.\nBeta diversity is defined as the change in species composition from one community to the next (Whittaker, 1972; Jost, 2007). Species relative abundances were square root-transformed and used to calculate Bray-Curtis distances of samples dissimilarity in Primer6. To test the null hypothesis of no difference in species relative abundance between islands, we used permutational multivariate analysis of variance (PERMANOVA) model on the Bray-Curtis dissimilarity data under a partial (type III) sum of squares, a fixed-effects sum to zero for mixed terms, 10,000 unrestricted parameters permutation of residuals and Monte Carlo tests in Primer v.6. The matrix of Bray-Curtis distances was also used as input to calculate non-metric multidimensional scaling (NMDS) coordinates using vegan package in R (R Development Core Team, 2010). The first and second components were used to create an ordination plot using ggvegan.\nLinear discriminant analysis (LDA) effect size (LEfSe) v.1.0 (Segata et al., 2011) was used to determine the species typifying the disparities between Mer and Waiben populations. To achieve this, a table of pre-filtered species relative abundances was normalised to ensure values for each species across all samples summed up to 1 million. Island of sample origin was used as a class variable. Non-parametric factorial Kruskal-Wallis (KW) sum-rank tests were then performed to detect species with significant (α \u003c0.05) differential abundance with respect to the class. The species that were significantly different between islands were used to build an LDA model from which the relative difference among classes was used to rank the features. The threshold for the logarithmic LDA score was set at ≥3. Finally, the ranked effect-size values were visualized in a LEfSe plot. Significant functional pathways were stratified into bacterial species, and the resulting stratified pathways were filtered to include only those that were present in at least 80% in either the Mer or Waiben populations. Stratified pathway abundances that remain significantly different between Mer and Waiben were determined using the Mann-Whitney U-test with the FDR multiple-testing adjustment, at a significance threshold of 0.05.\nCorrelations between measurements of each circulating biomarker in the host and the taxonomic or functional profiles of the microbiota were analyzed using Spearman’s rank correlation implemented in the Hmisc package in R and visualized using ggplot2 and gplots packages in R. Significance thresholds for the coefficients of association and P-values were respectively set at rho (ρ) ≥ |0.3| and p-value≤0.05, Benjamini-Hochberg (BH) adjusted for multiple hypothesis testing.\nStructural Equation modeling (SEM) was performed in R using the lavaan package (Rosseel, 2012) to assess the direct and indirect associations of host diet, behavior and demographics with host biomarkers of inflammation. Initial path diagrams were visualized using semPlot (Epskamp and Epskamp, 2017) package in R and partly modified into heatmaps using ggplot2. Briefly, we modeled the inflammatory biomarkers using exploratory factor analysis to identify three latent (unobserved) constructs, with TNF-α, IL-4, IL-12P70, as indicators for the first latent variable (Biomarker Factor 1), CRP, IL-18, and MCP1 as indicators for the second latent variable (Biomarker Factor 2), and IL-15, IL-33, IFNγ, and IL-1β indicators for the third latent variable (Biomarker Factor 3). Observed variables potentially important to inflammation, including alcohol consumption, smoking, diet (fruits, vegetables, fish, and intake of fatty and sugary foods typical of takeaways and soft-drinks), and demographics (age, gender, BMI, and island/study site) were also included. Four possible association frameworks were developed to explain the link between the specific exposures (age, gender, island, smoking status and intakes of fruits, vegetables, takeaways, sugar-sweetened beverages, seafood, and alcohol), the microbiome, and host physiology (Figure 3—figure supplement 1): Framework (1) the gut microbiome mediates the exposure-inflammation relationship; Framework (2) the gut microbiome influences pathophysiology but is not influenced by risk exposures; Framework (3) the gut microbiome is influenced by exposures but does not influence pathophysiology; and Framework (4) exposure risk factors are associated with the inflammatory profile, and the inflammatory profile predicts the gut microbiome composition. The model was assessed using the confirmatory factor analysis (CFA) function and estimated using the robust variant of maximum likelihood parameter estimation (MLR) with robust (Huber-White) standard errors and default bootstrapping (asymptomatically equivalent to Yuan-Bentler correction statistic) to account for data non-normality, presence of dichotomous and categorical variables, and correction for multiple hypothesis testing. Equivalents of the final SEM model were investigated and defined by adjusting the directionality of correlation or inferring co-variation instead of causation. Standard SEM fit guidelines (Hooper and Coughlan, 2008; Hu and Bentler, 1999), that is, chi-squared P-value; (χ2 P-value)\u003e0.05; comparative fit index (CFI) \u003e0.8; standardized root-mean residuals (SRMR) \u003c0.08; root-mean-square error of approximation (RSMEA) \u003c0.05; and the lowest Akaike information criterion (AIC) were used to assess model-data fit for each of the four theoretical frameworks.\nIndividual mediation relationships between host exposures, host gut microbiology (as the mediator), and host pathophysiology (as the outcome) were evaluated using the ‘mediation’ package in R. Fitted objects of the exposure and the mediator were analyzed using the ‘mediate’ function with nonparametric bootstrapping methods. Interaction was assessed through the incorporation of an interaction term into regression models."}
2_test
{"project":"2_test","denotations":[{"id":"33074097-30073906-26739287","span":{"begin":273,"end":277},"obj":"30073906"},{"id":"33074097-100702-26739288","span":{"begin":683,"end":687},"obj":"100702"},{"id":"33074097-100702-26739289","span":{"begin":2821,"end":2825},"obj":"100702"},{"id":"33074097-100702-26739290","span":{"begin":3276,"end":3280},"obj":"100702"},{"id":"33074097-18782390-26739291","span":{"begin":4511,"end":4515},"obj":"18782390"},{"id":"33074097-24695404-26739292","span":{"begin":4750,"end":4754},"obj":"24695404"},{"id":"33074097-26418763-26739293","span":{"begin":6527,"end":6531},"obj":"26418763"},{"id":"33074097-30377376-26739294","span":{"begin":7038,"end":7042},"obj":"30377376"},{"id":"33074097-26157614-26739295","span":{"begin":7272,"end":7276},"obj":"26157614"},{"id":"33074097-18027744-26739296","span":{"begin":8094,"end":8098},"obj":"18027744"},{"id":"33074097-18027744-26739297","span":{"begin":9661,"end":9665},"obj":"18027744"},{"id":"33074097-21702898-26739298","span":{"begin":10539,"end":10543},"obj":"21702898"}],"text":"Materials and methods\n\nParticipants and setting\nThis observational study recruited participants from the Well Persons Health Check (WPHC). In 1997, the Australian government started a broad community health promotion and screening programme called the WPHC (Berger et al., 2020) conducted by the Torres and Cape Hospital and Health Service (TCHHS). Through WPHC several Indigenous (Aboriginal and/or Torres Strait Islander) communities in northern Queensland were screened annually for sexually transmitted infections and chronic cardiovascular as well as chronic metabolic conditions. In 2016, TCHHS collaborated with James Cook University (Zenadth Kes Health Partnership; Weiland, 1978) to conduct these surveys in two Australian island communities, Waiben and Mer. These islands are located in the Torres Strait Islands (TSI) archipelago, which lies in the waters separating the far northern continental Australia’s Cape York Peninsula (northern-most tip of Queensland) and the Western Province of Papua New Guinea, off the continent of Australia. Mer (Murray Island) is a 4.3 km2 remote island located on the eastern periphery of the Torres Strait region, 210 km northeast of Waiben and 340 km west of Port Moresby, with a population of 450 in 2016. The island is less connected with no known fast-food outlet. Waiben (Thursday Island) is a 3.5 km2 landmass located about 39 km north of Cape York Peninsula with a population of 2938 in 2016. It is the administrative and commercial center of the Torres Strait Island Region.\nIn addition to the health check that was a standard component of the WPHC, participants consented to have additional health data collected for research purposes. Verbal consent was used whenever a written one could not be obtained. In addition to individual consent, parent/legal guardian consent was obtained for all participants younger than 18 years. The study incorporated all WPHC participants who identified as Aboriginal and/or Torres Strait Islander and attended the screening in October, November, and December 2016. There were 214 individuals aged 15 years and older who participated in the October, November, and December 2016 WPHC and identified as Aboriginal and/or Torres Strait Islander. Participants were excluded due to insufficient survey information (n = 2) and or not providing consent to participate in the research component of the health check (n = 1). Only participants who provided stool samples for metagenomic sequencing (total n = 100; 50 participants from each site) were then included in the current analysis. The study was granted ethical approval by the Far North Queensland Human Research Ethics Committee (HREC/16/QCH/70–1059). In addition, written support from the local Community Council, Primary Health Care Service, and TCHHS was also provided (Weiland, 1978).\n\nParticipant demographic, dietary, and behavioral factors\nWe collected the island of residence, age, gender, physical activity, and daily alcohol consumption as well as smoking status. Dietary intake of seafood, takeaway foods, vegetables, fruits, and sugar-sweetened beverages was determined using a culturally-appropriate food frequency questionnaire that assessed food and beverage consumption through the use of non-leading questions (Weiland, 1978).\nAge, gender, height, weight, ethnicity, systolic blood pressure (SBP), diastolic blood pressure (DBP), known diabetes status, and known diabetes therapy were documented from WPHC clinical records. The mean arterial pressure (MAP) was calculated from brachial pressure values as follows:MAP=SBP+(2×DBP)3\nBody mass index (BMI; kg/m2) was computed from weight and height records. Hypertension was defined as SBP \u003e140 and/or DBP \u003e 90. Tobacco smoking and alcohol consumption were assessed using a standarized questionnaire.\n\nClinical sample collection\nA summary of key resources utilized, including sample collection kits, DNA isolation and library prep kits, DNA sequencing platform, and software is provided in Supplementary file 1e Each participant provided a sample of stool, blood, and urine. The stool was collected by participants using the OMNIgene GUT collection kit (DNA Genotek, Ontario, Canada), according to manufacturer’s instructions. Briefly, the stool was deposited in a sterile container, transferred into the OMNIgene tube using the provided spatula, mixed with the stabilizing liquid, and stored at room temperature until collection. Blood and urine were collected by TCHHS as described previously (Zhao et al., 2008).\n\nMeasurements of inflammatory and cardiometabolic markers\nConcentrations of various biomarkers in blood and/or urine were measured using chemistry multiplex immunoassays and enzyme-linked immunosorbent assays (ELISA; Bolger et al., 2014) as follows: Interleukins (IL) IL-1β, IL-2, IL-4, IL-5, IL-6, IL-10, IL-12p40, IL-12p70, IL-13, IL-15, IL-17A, IL-18, and IL-33; type II interferon (IFNγ), monocyte chemoattractant protein 1 (MCP1/CCL2), macrophage inflammatory protein (MIP-1α/CCL3), and tumor necrosis factor alpha (TNFα) were measured using Luminex High Sensitivity (8-plex and 9-plex) Human ProcartaPlex Panels. Serum C-reactive protein (CRP) was measured using the CRP Human ProcartaPlex Simplex Kit. Serum lipopolysaccharide-binding protein (LBP) was also measured using Human LBP ELISA Kit. Random blood glucose (RBG) and glycated hemoglobin or hemoglobin A1c (HbA1c) were measured using blood glucose meter and Haemoglobin A1c (HbA1c) (Human) ELISA Kit, respectively. All commercial assays were performed according to manufacturers’ instructions.\n\nDNA isolation and metagenomic sequencing\nMicrobial DNA was isolated from stool samples using DNeasy Powerlyzer PowerSoil Kit (Qiagen, Hilden, Germany). DNA yield was determined in Qubit Fluorometric Quantification using Quant-iT High-Sensitivity dsDNA Assay Kit. Libraries for shotgun metagenomic sequencing were fragmented using Nextera XT Library Preparation Kit v2 (Illumina Inc, San Diego, CA) and indexed using the Illumina Nextera XT Index Kit v2 according to manufacturer’s instructions. Amplicon libraries were then sequenced on the Illumina HiSeq 2500 platform at the SAHMRI David R Gunn Genomics Suite using Illumina HiSeq SBS 2 × 125bp v4 kit (Illumina Inc). Reads were converted into FASTQ format using bcl2fastq v.1.8.4. On average, 16,666,136 sequencing reads were obtained from each sample before quality filtering.\n\nBioinformatic processing\nPaired-end reads were adapter- and quality-filtered using Trimmomatic v.0.36 (Truong et al., 2015) with the following parameters: PE ILLUMINACLIP:TruSeq3-PE:3:30:10 LEADING:10 MINLEN:30 SLIDINGWINDOW: 4:30; HEADCROP:10. Samples included in the downstream analysis had at least 7.4 million paired-end reads of at least 91 bp in length after quality control. The quality of reads was checked before and after this process using FastQC v.0.11.5.\nUsing the quality-controlled paired-end reads, taxonomic profiling was performed using the metagenomic phylogenetic analysis (MetaPhlAn) v.2.6 (Franzosa et al., 2018), which uses a database of clade-specific markers to quantify microbiota constituents at the species and higher taxonomic levels. The generation of the abundance of metabolic pathways was performed using HUMAnN2 (Asnicar et al., 2015). Taxonomic and metabolic profiles for both islands were visualized using GraPhlAn v.0.9.7 (Whittaker, 1972). Sequenced metagenomic data were submitted to the National Center for Biotechnology Information (NCBI) Sequence Read Archive (SRA) under BioProject ID PRJNA503909. Summary of the quality-controlled fecal microbiome sequencing reads is shown in Supplementary file 1f.\n\nStatistical analysis\nStatistical analyses and graphical illustrations were accomplished in R software for statistical computing, Primer 7, GraphPad Prism 7, and Statistical Analysis Software (SAS/STAT) suite. Rare taxa, present in less than 40% of the participants at \u003c1% relative abundance, were excluded from the analysis.\nAlpha-diversity is defined as a local measure of average species diversity in a community. (Whittaker, 1972; Jost, 2007). To estimate the species alpha diversity in Mer and Waiben, we examined the microbial composition in each community using the vegan (Oksanen et al., 2018) package in R. (R Development Core Team, 2010) Accordingly, the 'estimate' function was used to calculate Chao1’s measure of richness; a measure of the number of species (or other taxonomic levels) present at a site. The 'diversity' function was used to estimate the Shannon-Wiener Index (H'); a measure of how many different types of species are present in the community. Species diversity takes into account both species richness and the dominance or evenness of the species. Evenness measures homogeneity in a community in terms of species relative abundances. A community in which all species are proportionately common is regarded as stable and has a high degree of evenness. Since vegan does not provide a function for Shannon’s evenness/equitability (E), we used a modified R function to estimated E by dividing the actual diversity (H') with the maximum possible diversity value (Hmax). The reasoning is, H' decreases when the species composition is more uneven and reaches a maximum with the highest evenness. Therefore, when all species are equally common, Hmax=lnS (where S is the total number of species), and E=H'÷Hmax. Differences in species composition within Mer and Waiben were analyzed in GraphPad using the Mann-Whitney U-test the with FDR multiple-testing adjustment.\nBeta diversity is defined as the change in species composition from one community to the next (Whittaker, 1972; Jost, 2007). Species relative abundances were square root-transformed and used to calculate Bray-Curtis distances of samples dissimilarity in Primer6. To test the null hypothesis of no difference in species relative abundance between islands, we used permutational multivariate analysis of variance (PERMANOVA) model on the Bray-Curtis dissimilarity data under a partial (type III) sum of squares, a fixed-effects sum to zero for mixed terms, 10,000 unrestricted parameters permutation of residuals and Monte Carlo tests in Primer v.6. The matrix of Bray-Curtis distances was also used as input to calculate non-metric multidimensional scaling (NMDS) coordinates using vegan package in R (R Development Core Team, 2010). The first and second components were used to create an ordination plot using ggvegan.\nLinear discriminant analysis (LDA) effect size (LEfSe) v.1.0 (Segata et al., 2011) was used to determine the species typifying the disparities between Mer and Waiben populations. To achieve this, a table of pre-filtered species relative abundances was normalised to ensure values for each species across all samples summed up to 1 million. Island of sample origin was used as a class variable. Non-parametric factorial Kruskal-Wallis (KW) sum-rank tests were then performed to detect species with significant (α \u003c0.05) differential abundance with respect to the class. The species that were significantly different between islands were used to build an LDA model from which the relative difference among classes was used to rank the features. The threshold for the logarithmic LDA score was set at ≥3. Finally, the ranked effect-size values were visualized in a LEfSe plot. Significant functional pathways were stratified into bacterial species, and the resulting stratified pathways were filtered to include only those that were present in at least 80% in either the Mer or Waiben populations. Stratified pathway abundances that remain significantly different between Mer and Waiben were determined using the Mann-Whitney U-test with the FDR multiple-testing adjustment, at a significance threshold of 0.05.\nCorrelations between measurements of each circulating biomarker in the host and the taxonomic or functional profiles of the microbiota were analyzed using Spearman’s rank correlation implemented in the Hmisc package in R and visualized using ggplot2 and gplots packages in R. Significance thresholds for the coefficients of association and P-values were respectively set at rho (ρ) ≥ |0.3| and p-value≤0.05, Benjamini-Hochberg (BH) adjusted for multiple hypothesis testing.\nStructural Equation modeling (SEM) was performed in R using the lavaan package (Rosseel, 2012) to assess the direct and indirect associations of host diet, behavior and demographics with host biomarkers of inflammation. Initial path diagrams were visualized using semPlot (Epskamp and Epskamp, 2017) package in R and partly modified into heatmaps using ggplot2. Briefly, we modeled the inflammatory biomarkers using exploratory factor analysis to identify three latent (unobserved) constructs, with TNF-α, IL-4, IL-12P70, as indicators for the first latent variable (Biomarker Factor 1), CRP, IL-18, and MCP1 as indicators for the second latent variable (Biomarker Factor 2), and IL-15, IL-33, IFNγ, and IL-1β indicators for the third latent variable (Biomarker Factor 3). Observed variables potentially important to inflammation, including alcohol consumption, smoking, diet (fruits, vegetables, fish, and intake of fatty and sugary foods typical of takeaways and soft-drinks), and demographics (age, gender, BMI, and island/study site) were also included. Four possible association frameworks were developed to explain the link between the specific exposures (age, gender, island, smoking status and intakes of fruits, vegetables, takeaways, sugar-sweetened beverages, seafood, and alcohol), the microbiome, and host physiology (Figure 3—figure supplement 1): Framework (1) the gut microbiome mediates the exposure-inflammation relationship; Framework (2) the gut microbiome influences pathophysiology but is not influenced by risk exposures; Framework (3) the gut microbiome is influenced by exposures but does not influence pathophysiology; and Framework (4) exposure risk factors are associated with the inflammatory profile, and the inflammatory profile predicts the gut microbiome composition. The model was assessed using the confirmatory factor analysis (CFA) function and estimated using the robust variant of maximum likelihood parameter estimation (MLR) with robust (Huber-White) standard errors and default bootstrapping (asymptomatically equivalent to Yuan-Bentler correction statistic) to account for data non-normality, presence of dichotomous and categorical variables, and correction for multiple hypothesis testing. Equivalents of the final SEM model were investigated and defined by adjusting the directionality of correlation or inferring co-variation instead of causation. Standard SEM fit guidelines (Hooper and Coughlan, 2008; Hu and Bentler, 1999), that is, chi-squared P-value; (χ2 P-value)\u003e0.05; comparative fit index (CFI) \u003e0.8; standardized root-mean residuals (SRMR) \u003c0.08; root-mean-square error of approximation (RSMEA) \u003c0.05; and the lowest Akaike information criterion (AIC) were used to assess model-data fit for each of the four theoretical frameworks.\nIndividual mediation relationships between host exposures, host gut microbiology (as the mediator), and host pathophysiology (as the outcome) were evaluated using the ‘mediation’ package in R. Fitted objects of the exposure and the mediator were analyzed using the ‘mediate’ function with nonparametric bootstrapping methods. Interaction was assessed through the incorporation of an interaction term into regression models."}