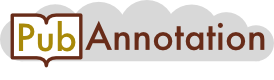
PMC:7553147 / 5535-6158
Annnotations
LitCovid-PD-FMA-UBERON
{"project":"LitCovid-PD-FMA-UBERON","denotations":[{"id":"T9","span":{"begin":379,"end":382},"obj":"Body_part"},{"id":"T10","span":{"begin":448,"end":451},"obj":"Body_part"}],"attributes":[{"id":"A9","pred":"fma_id","subj":"T9","obj":"http://purl.org/sig/ont/fma/fma67847"},{"id":"A10","pred":"fma_id","subj":"T10","obj":"http://purl.org/sig/ont/fma/fma67847"}],"text":"The basic elements of the workflow combine classic cryo-EM algorithms with recent improvements in particle picking (Sanchez-Garcia et al., 2018 ▸; Sanchez-Garcia, Segura et al., 2020 ▸; Wagner et al., 2019 ▸) and the key ideas of meta classifiers, which integrate multiple classifiers by a ‘consensus’ approach (Sorzano et al., 2020 ▸), and finish with a totally new approach to map post-processing based on deep learning that we term Deep cryo-EM Map Enhancer (DeepEMhancer; Sanchez-Garcia, Gomez-Blanco et al., 2020 ▸), which complements our previous proposal on local deblurring (Ramírez-Aportela, Vilas et al., 2020 ▸)."}
LitCovid-PD-CLO
{"project":"LitCovid-PD-CLO","denotations":[{"id":"T32","span":{"begin":139,"end":143},"obj":"http://purl.obolibrary.org/obo/CLO_0001185"},{"id":"T33","span":{"begin":288,"end":289},"obj":"http://purl.obolibrary.org/obo/CLO_0001020"},{"id":"T34","span":{"begin":353,"end":354},"obj":"http://purl.obolibrary.org/obo/CLO_0001020"}],"text":"The basic elements of the workflow combine classic cryo-EM algorithms with recent improvements in particle picking (Sanchez-Garcia et al., 2018 ▸; Sanchez-Garcia, Segura et al., 2020 ▸; Wagner et al., 2019 ▸) and the key ideas of meta classifiers, which integrate multiple classifiers by a ‘consensus’ approach (Sorzano et al., 2020 ▸), and finish with a totally new approach to map post-processing based on deep learning that we term Deep cryo-EM Map Enhancer (DeepEMhancer; Sanchez-Garcia, Gomez-Blanco et al., 2020 ▸), which complements our previous proposal on local deblurring (Ramírez-Aportela, Vilas et al., 2020 ▸)."}
LitCovid-PD-GO-BP
{"project":"LitCovid-PD-GO-BP","denotations":[{"id":"T1","span":{"begin":413,"end":421},"obj":"http://purl.obolibrary.org/obo/GO_0007612"}],"text":"The basic elements of the workflow combine classic cryo-EM algorithms with recent improvements in particle picking (Sanchez-Garcia et al., 2018 ▸; Sanchez-Garcia, Segura et al., 2020 ▸; Wagner et al., 2019 ▸) and the key ideas of meta classifiers, which integrate multiple classifiers by a ‘consensus’ approach (Sorzano et al., 2020 ▸), and finish with a totally new approach to map post-processing based on deep learning that we term Deep cryo-EM Map Enhancer (DeepEMhancer; Sanchez-Garcia, Gomez-Blanco et al., 2020 ▸), which complements our previous proposal on local deblurring (Ramírez-Aportela, Vilas et al., 2020 ▸)."}
LitCovid-sentences
{"project":"LitCovid-sentences","denotations":[{"id":"T35","span":{"begin":0,"end":623},"obj":"Sentence"}],"namespaces":[{"prefix":"_base","uri":"http://pubannotation.org/ontology/tao.owl#"}],"text":"The basic elements of the workflow combine classic cryo-EM algorithms with recent improvements in particle picking (Sanchez-Garcia et al., 2018 ▸; Sanchez-Garcia, Segura et al., 2020 ▸; Wagner et al., 2019 ▸) and the key ideas of meta classifiers, which integrate multiple classifiers by a ‘consensus’ approach (Sorzano et al., 2020 ▸), and finish with a totally new approach to map post-processing based on deep learning that we term Deep cryo-EM Map Enhancer (DeepEMhancer; Sanchez-Garcia, Gomez-Blanco et al., 2020 ▸), which complements our previous proposal on local deblurring (Ramírez-Aportela, Vilas et al., 2020 ▸)."}
LitCovid-PubTator
{"project":"LitCovid-PubTator","denotations":[{"id":"56","span":{"begin":116,"end":130},"obj":"Disease"},{"id":"57","span":{"begin":147,"end":161},"obj":"Disease"},{"id":"58","span":{"begin":462,"end":474},"obj":"Disease"},{"id":"59","span":{"begin":476,"end":490},"obj":"Disease"}],"attributes":[{"id":"A56","pred":"tao:has_database_id","subj":"56","obj":"MESH:C536767"},{"id":"A57","pred":"tao:has_database_id","subj":"57","obj":"MESH:C536767"},{"id":"A59","pred":"tao:has_database_id","subj":"59","obj":"MESH:C536767"}],"namespaces":[{"prefix":"Tax","uri":"https://www.ncbi.nlm.nih.gov/taxonomy/"},{"prefix":"MESH","uri":"https://id.nlm.nih.gov/mesh/"},{"prefix":"Gene","uri":"https://www.ncbi.nlm.nih.gov/gene/"},{"prefix":"CVCL","uri":"https://web.expasy.org/cellosaurus/CVCL_"}],"text":"The basic elements of the workflow combine classic cryo-EM algorithms with recent improvements in particle picking (Sanchez-Garcia et al., 2018 ▸; Sanchez-Garcia, Segura et al., 2020 ▸; Wagner et al., 2019 ▸) and the key ideas of meta classifiers, which integrate multiple classifiers by a ‘consensus’ approach (Sorzano et al., 2020 ▸), and finish with a totally new approach to map post-processing based on deep learning that we term Deep cryo-EM Map Enhancer (DeepEMhancer; Sanchez-Garcia, Gomez-Blanco et al., 2020 ▸), which complements our previous proposal on local deblurring (Ramírez-Aportela, Vilas et al., 2020 ▸)."}