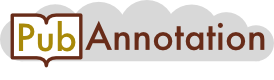
PMC:7551987 / 7701-18716
Annnotations
2_test
{"project":"2_test","denotations":[{"id":"32971954-31480919-143921949","span":{"begin":1900,"end":1902},"obj":"31480919"},{"id":"32971954-32163436-143921950","span":{"begin":1903,"end":1905},"obj":"32163436"},{"id":"32971954-32083643-143921951","span":{"begin":2662,"end":2664},"obj":"32083643"},{"id":"32971954-32446187-143921952","span":{"begin":2665,"end":2667},"obj":"32446187"},{"id":"32971954-32728118-143921953","span":{"begin":2925,"end":2927},"obj":"32728118"},{"id":"32971954-23333764-143921954","span":{"begin":3233,"end":3235},"obj":"23333764"},{"id":"32971954-23348785-143921955","span":{"begin":3236,"end":3238},"obj":"23348785"},{"id":"32971954-30642334-143921956","span":{"begin":3239,"end":3241},"obj":"30642334"},{"id":"32971954-32015543-143921957","span":{"begin":4048,"end":4050},"obj":"32015543"},{"id":"32971954-15682873-143921958","span":{"begin":5614,"end":5616},"obj":"15682873"},{"id":"32971954-25087040-143921959","span":{"begin":6236,"end":6237},"obj":"25087040"},{"id":"32971954-16299384-143921960","span":{"begin":6238,"end":6240},"obj":"16299384"},{"id":"32971954-25087040-143921961","span":{"begin":8870,"end":8871},"obj":"25087040"}],"text":"2. Materials and Methods\n\n2.1. Model Motivation and Application\nThe mathematical model explored in this study is adapted from a recent one developed to investigate environmental transmission of SARS-CoV-2 during the early-stage outbreak dynamics of coronavirus disease 2019 (COVID-19), with parameter values based on fits to actual country outbreak data [22]. In this study, we utilize this model to examine questions about the evolution of free-living survival. While the phenomenon we examine is a very relevant one that manifests in the real world, we want to emphasize that none of the methods or results in this study are intended to be applied to the current COVID-19 pandemic (as of September, 2020). This study is an attempt at responsible theoretical biology, with data-informed models and inferences that are germane to the natural world. However, neither do we support the extrapolation of these findings to any particular aspect of COVID-19 nor should they inform a policy or intervention. The model applies to a number of scenarios that include outbreaks in a naïve host population. This describes situations such as the evolution of novel viral lineages, viral spillover events, or host shifts, where a virus with a preexisting relationship between virulence and survival emerges in a population of new hosts. Another such scenario where this model applies is one where a virus has already emerged but evolves in a subpopulation in the novel hosts before a migration event of some kind introduces the evolved virus population to a fully susceptible population of hosts.\n\n2.2. Model Description\nThe model is implemented via a set of ordinary differential equations, defined by Equations (1)–(6). It implements viral free-living survival via the “Waterborne Abiotic or other Indirect Transmission (WAIT)” modelling framework, coupling individuals and the pathogen within the environment [23,24].\nWithin the model, the βw term allows for individuals to become infected via viral pathogen deposited in the environment and terms 𝜎A and 𝜎I allow asymptomatic and symptomatic individuals to deposit pathogens into the environment, respectively. Adapted from the more traditional SEIR (susceptible-exposed-infected-recovered) model, the SEAIR-W (susceptible-exposed-asymptomatic-infected-recovered-WAIT) model interrogates the consequences of the two hypotheses outlined above while representing the dynamics of a very relevant disease system (SARS-CoV-2) that includes an asymptomatic infectious population. While the importance of asymptomatic transmission was debated early in the pandemic, many studies have affirmed its role in the spread of disease [25,26,27]. Though environmental transmission of SARS-CoV-2 remains a controversial topic, it is plausible that asymptomatic individuals may spread disease through frequent contact with the environment, thus increasing the proportion of virus that is free-living [28]. We acknowledge that mathematical models of epidemics can be limited by “identifiability,” which can obfuscate the relative importance of some routes of transmission. In models that have both indirect and direct routes of transmission, it can be very difficult to conclude that one route is predominant [29,30,31]. (1) dSdt=μN−S−βAA+βIIN+βWWS (2) dEdt=βAA+βIIN+βWWS−ε+μE (3) dAdt=εE−ω+μA (4) dIdt=1−pωA−v+μII (5) dRdt=pωA+vI−μR (6) dWdt=σAA+σIIN1−W−kW\nFigure 1 depicts the compartmental diagram for the model. The direction of the arrows corresponds to the flow of the individuals and the pathogen through the system. Note that individuals can move directly from the asymptomatically infected compartment to the recovered compartment (bypassing the symptomatic compartment) via what we call a “mild track”. The dashed arrows represent WAIT coupling to the environment. The model is inspired by one developed to interrogate environmental transmission of SARS-CoV-2 [22].\n\n2.3. Simulations of Outbreaks\nThe system was numerically integrated using the “odeint” solver in the Scipy 1.4—Python scientific computation suite [32]. The simulations track the populations for each of the compartments listed in Figure 1. Each model run occurred over 250 days, which amounts to over 8 months of the epidemic or 5× the peak of the outbreak. This length of time is consistent with the antecedent SARS-CoV-2 model [22], long enough for the dynamics of the system to manifest. Note however that, for this study, we are especially interested in the early window of an outbreak: the first 30 days. We focus on this window because this is the time frame that best captures the underlying physics of an epidemic, as 30 days is often before populations are able to adjust their individual behaviors. The code constructed for the analysis in this study is publicly available on github: https://github.com/OgPlexus/Pharaohlocks.\n\n2.4. Population Definitions and Parameter Values\nTable 1 outlines the definitions of each population and provides the initial population values used for all simulations conducted in this study. The nominal parameter values used are defined in Table 2. The initial values are drawn from the aforementioned COVID-19 outbreak study, derived from empirical findings and country-level outbreak data [22].\n\n2.5. Virulence Definition\nIn this study, we define virulence as the capacity to cause a disease. In order to measure it, we utilize a set of parameters that uniformly increase the rate or probability of causing symptomatic disease or the severity of those symptoms (including death). Our definition is more comprehensive than many other models of parasite virulence (e.g., [4,13]), which tend to focus on a single aspect of the natural history of disease associated with harm to a host (e.g., the fitness consequences of an infection on the host population or the case fatality rate). Instead of having to justify a definition built around a single term (e.g., the term associated with fatality), we took a collective approach to defining virulence through all terms that foment the viral-induced onset of symptomatic disease and death. This definition allows for the reality of pleiotropic effects in viral pathogens, where adaptations can have multiple effects on the natural history of disease [2,33]. Our definition of virulence emphasizes terms that influence host wellness and/or are symptoms of disease. The iteration of virulence used in this study also undermines the potential for overly weighting only one or a small number of parameters under a large umbrella of virulence. Because so many varying definitions exist for virulence, we have also performed calculations according to a different definition of virulence, one that exclusively considers terms that have a detrimental direct effect on the host and neither of the terms that reflect symptoms of severe disease (𝜎a and 𝜎I). These calculations can be found in the Supplementary Materials.\nThe collection of parameters that we use to define virulence are as follows: the infected population death rate (𝜇I), the incubation period of SARS-CoV-2 (𝜂), the rate of transfer from asymptomatic to symptomatic (1/⍵), the infected population recovery rate (ν), the percent of individuals that move from the asymptomatic to the recovered compartment without showing symptoms (the “mild” recovery track, p), the contact rate of people with people × the transmission probability of people to people by an asymptomatic individual (βA), the contact rate of people with people × the transmission probability of people to people by an asymptomatically infected person (βI), the contact rate of people with the environment × the probability of shedding by an asymptomatic individual to the environmental (𝜎A), the contact rate of people with the environment × the probability of symptomatically infected individuals shedding in the environment (𝜎I), and the average number of days before infection (1/ε).\nTable 3 outlines the direction in which each of the virulence-associated parameters are modulated as virulence decreases or increases. An up arrow (↑) indicates the parameter increases (by an equivalent percent) when the percent virulence is changed. A down arrow (↓) indicates the parameter decreases (by an equivalent percent) when the percent change in virulence is applied. Changes in virulence are then defined, in this study, as an equivalent uniform (percent) change in each of the parameters listed above. For the purposes of our study, we modify virulence by changing all parameters associated with virulence by 5%. One could also disambiguate virulence into changes in individual subcomponents; however, that is not the focus of this current study.\n\n2.6. Survival Definition\nSurvival is defined as the set of parameters that, when uniformly modulated, increases the pathogen’s probability of surviving the outside environment and successfully infecting a new host [2]. In our model, this includes both the waning virus rate in the environment (k) and the contact rate of an individual with the environment × the transmission probability of the environment to people (βw). Table 4 outlines the direction (increasing or decreasing) in which these parameters are modulated when survival is decreased or increased. Within both models, a (percent) change in survival is defined as an equivalent uniform (percent) change in the survival parameters.\nThroughout this study, the impact of changes in virulence and survival (and the relationship between these traits) are assessed with respect to the following four epidemic metrics: the number of infected individuals (asymptomatic and symptomatic) at the maximum (when the outbreak is at its most severe), the rate at which the peak infected population is reached (tmax−1), the total infected population after 30 days, and the basic reproductive ratio (R0). Importantly, among these signatures, the basic reproductive ratio is the most frequently used in epidemiology and benefits from familiarity and mathematical formalism (see Section 2.7). The other signatures are determined through simulations of an epidemic for a given set of parameter values. Nonetheless, this study’s inclusion of multiple features of the epidemic allows us to examine how variation in virus life-history traits may influence different aspects of an epidemic in peculiar ways.\n\n2.7. Basic Reproductive Ratio\nEquations (7)–(9) give the analytic expression of the basic reproductive ratio (R0) for the model used in this study. This expression for R0 can be deconstructed into two components. Equation (8) only contains parameters associated with person to person transmission (Rp), while Equation (9) solely contains parameters associated with transmission from the environment (Re). In the Supplementary Materials, we provide additional information on these terms and their derivations. Applying the parameters values in Table 2, the numerical value of the basic reproductive ratio is given as R0 ~ 2.82. (7) R0=RpRp2+4Re22 (8) Rp=εβAμI+v+βI1−pωμ+εμ+ωμI+v (9) Re2=εβWσAμI+v+σI1−pωkμ+εμ+ωμI+v"}
LitCovid-PubTator
{"project":"LitCovid-PubTator","denotations":[{"id":"34","span":{"begin":194,"end":204},"obj":"Species"},{"id":"35","span":{"begin":249,"end":273},"obj":"Disease"},{"id":"36","span":{"begin":275,"end":283},"obj":"Disease"},{"id":"37","span":{"begin":317,"end":321},"obj":"Disease"},{"id":"38","span":{"begin":665,"end":673},"obj":"Disease"},{"id":"39","span":{"begin":944,"end":952},"obj":"Disease"},{"id":"45","span":{"begin":2450,"end":2460},"obj":"Species"},{"id":"46","span":{"begin":2710,"end":2720},"obj":"Species"},{"id":"47","span":{"begin":1971,"end":1979},"obj":"Disease"},{"id":"48","span":{"begin":2212,"end":2220},"obj":"Disease"},{"id":"49","span":{"begin":2285,"end":2293},"obj":"Disease"},{"id":"52","span":{"begin":3882,"end":3892},"obj":"Species"},{"id":"53","span":{"begin":3613,"end":3621},"obj":"Disease"},{"id":"55","span":{"begin":4312,"end":4322},"obj":"Species"},{"id":"57","span":{"begin":5142,"end":5150},"obj":"Disease"},{"id":"63","span":{"begin":5514,"end":5519},"obj":"Disease"},{"id":"64","span":{"begin":5585,"end":5603},"obj":"Disease"},{"id":"65","span":{"begin":5735,"end":5742},"obj":"Disease"},{"id":"66","span":{"begin":5762,"end":5771},"obj":"Disease"},{"id":"67","span":{"begin":6068,"end":6073},"obj":"Disease"},{"id":"85","span":{"begin":7039,"end":7049},"obj":"Species"},{"id":"86","span":{"begin":7324,"end":7330},"obj":"Species"},{"id":"87","span":{"begin":7336,"end":7342},"obj":"Species"},{"id":"88","span":{"begin":7377,"end":7383},"obj":"Species"},{"id":"89","span":{"begin":7387,"end":7393},"obj":"Species"},{"id":"90","span":{"begin":7450,"end":7456},"obj":"Species"},{"id":"91","span":{"begin":7462,"end":7468},"obj":"Species"},{"id":"92","span":{"begin":7503,"end":7509},"obj":"Species"},{"id":"93","span":{"begin":7513,"end":7519},"obj":"Species"},{"id":"94","span":{"begin":7585,"end":7591},"obj":"Species"},{"id":"95","span":{"begin":7720,"end":7726},"obj":"Species"},{"id":"96","span":{"begin":6977,"end":6985},"obj":"Disease"},{"id":"97","span":{"begin":6997,"end":7002},"obj":"Disease"},{"id":"98","span":{"begin":7120,"end":7128},"obj":"Disease"},{"id":"99","span":{"begin":7543,"end":7551},"obj":"Disease"},{"id":"100","span":{"begin":7785,"end":7793},"obj":"Disease"},{"id":"101","span":{"begin":7878,"end":7887},"obj":"Disease"},{"id":"103","span":{"begin":8535,"end":8547},"obj":"Chemical"},{"id":"105","span":{"begin":9064,"end":9070},"obj":"Species"},{"id":"109","span":{"begin":9543,"end":9551},"obj":"Disease"},{"id":"110","span":{"begin":9680,"end":9688},"obj":"Disease"},{"id":"111","span":{"begin":9731,"end":9739},"obj":"Disease"}],"attributes":[{"id":"A34","pred":"tao:has_database_id","subj":"34","obj":"Tax:2697049"},{"id":"A35","pred":"tao:has_database_id","subj":"35","obj":"MESH:C000657245"},{"id":"A36","pred":"tao:has_database_id","subj":"36","obj":"MESH:C000657245"},{"id":"A37","pred":"tao:has_database_id","subj":"37","obj":"MESH:D012640"},{"id":"A38","pred":"tao:has_database_id","subj":"38","obj":"MESH:C000657245"},{"id":"A39","pred":"tao:has_database_id","subj":"39","obj":"MESH:C000657245"},{"id":"A45","pred":"tao:has_database_id","subj":"45","obj":"Tax:2697049"},{"id":"A46","pred":"tao:has_database_id","subj":"46","obj":"Tax:2697049"},{"id":"A47","pred":"tao:has_database_id","subj":"47","obj":"MESH:D007239"},{"id":"A48","pred":"tao:has_database_id","subj":"48","obj":"MESH:D007239"},{"id":"A49","pred":"tao:has_database_id","subj":"49","obj":"MESH:D007239"},{"id":"A52","pred":"tao:has_database_id","subj":"52","obj":"Tax:2697049"},{"id":"A53","pred":"tao:has_database_id","subj":"53","obj":"MESH:D007239"},{"id":"A55","pred":"tao:has_database_id","subj":"55","obj":"Tax:2697049"},{"id":"A57","pred":"tao:has_database_id","subj":"57","obj":"MESH:C000657245"},{"id":"A63","pred":"tao:has_database_id","subj":"63","obj":"MESH:D003643"},{"id":"A64","pred":"tao:has_database_id","subj":"64","obj":"MESH:D010272"},{"id":"A65","pred":"tao:has_database_id","subj":"65","obj":"MESH:D012640"},{"id":"A66","pred":"tao:has_database_id","subj":"66","obj":"MESH:D007239"},{"id":"A67","pred":"tao:has_database_id","subj":"67","obj":"MESH:D003643"},{"id":"A85","pred":"tao:has_database_id","subj":"85","obj":"Tax:2697049"},{"id":"A86","pred":"tao:has_database_id","subj":"86","obj":"Tax:9606"},{"id":"A87","pred":"tao:has_database_id","subj":"87","obj":"Tax:9606"},{"id":"A88","pred":"tao:has_database_id","subj":"88","obj":"Tax:9606"},{"id":"A89","pred":"tao:has_database_id","subj":"89","obj":"Tax:9606"},{"id":"A90","pred":"tao:has_database_id","subj":"90","obj":"Tax:9606"},{"id":"A91","pred":"tao:has_database_id","subj":"91","obj":"Tax:9606"},{"id":"A92","pred":"tao:has_database_id","subj":"92","obj":"Tax:9606"},{"id":"A93","pred":"tao:has_database_id","subj":"93","obj":"Tax:9606"},{"id":"A94","pred":"tao:has_database_id","subj":"94","obj":"Tax:9606"},{"id":"A95","pred":"tao:has_database_id","subj":"95","obj":"Tax:9606"},{"id":"A96","pred":"tao:has_database_id","subj":"96","obj":"MESH:D007239"},{"id":"A97","pred":"tao:has_database_id","subj":"97","obj":"MESH:D003643"},{"id":"A98","pred":"tao:has_database_id","subj":"98","obj":"MESH:D007239"},{"id":"A99","pred":"tao:has_database_id","subj":"99","obj":"MESH:D007239"},{"id":"A100","pred":"tao:has_database_id","subj":"100","obj":"MESH:D007239"},{"id":"A101","pred":"tao:has_database_id","subj":"101","obj":"MESH:D007239"},{"id":"A105","pred":"tao:has_database_id","subj":"105","obj":"Tax:9606"},{"id":"A109","pred":"tao:has_database_id","subj":"109","obj":"MESH:D007239"},{"id":"A110","pred":"tao:has_database_id","subj":"110","obj":"MESH:D007239"},{"id":"A111","pred":"tao:has_database_id","subj":"111","obj":"MESH:D007239"}],"namespaces":[{"prefix":"Tax","uri":"https://www.ncbi.nlm.nih.gov/taxonomy/"},{"prefix":"MESH","uri":"https://id.nlm.nih.gov/mesh/"},{"prefix":"Gene","uri":"https://www.ncbi.nlm.nih.gov/gene/"},{"prefix":"CVCL","uri":"https://web.expasy.org/cellosaurus/CVCL_"}],"text":"2. Materials and Methods\n\n2.1. Model Motivation and Application\nThe mathematical model explored in this study is adapted from a recent one developed to investigate environmental transmission of SARS-CoV-2 during the early-stage outbreak dynamics of coronavirus disease 2019 (COVID-19), with parameter values based on fits to actual country outbreak data [22]. In this study, we utilize this model to examine questions about the evolution of free-living survival. While the phenomenon we examine is a very relevant one that manifests in the real world, we want to emphasize that none of the methods or results in this study are intended to be applied to the current COVID-19 pandemic (as of September, 2020). This study is an attempt at responsible theoretical biology, with data-informed models and inferences that are germane to the natural world. However, neither do we support the extrapolation of these findings to any particular aspect of COVID-19 nor should they inform a policy or intervention. The model applies to a number of scenarios that include outbreaks in a naïve host population. This describes situations such as the evolution of novel viral lineages, viral spillover events, or host shifts, where a virus with a preexisting relationship between virulence and survival emerges in a population of new hosts. Another such scenario where this model applies is one where a virus has already emerged but evolves in a subpopulation in the novel hosts before a migration event of some kind introduces the evolved virus population to a fully susceptible population of hosts.\n\n2.2. Model Description\nThe model is implemented via a set of ordinary differential equations, defined by Equations (1)–(6). It implements viral free-living survival via the “Waterborne Abiotic or other Indirect Transmission (WAIT)” modelling framework, coupling individuals and the pathogen within the environment [23,24].\nWithin the model, the βw term allows for individuals to become infected via viral pathogen deposited in the environment and terms 𝜎A and 𝜎I allow asymptomatic and symptomatic individuals to deposit pathogens into the environment, respectively. Adapted from the more traditional SEIR (susceptible-exposed-infected-recovered) model, the SEAIR-W (susceptible-exposed-asymptomatic-infected-recovered-WAIT) model interrogates the consequences of the two hypotheses outlined above while representing the dynamics of a very relevant disease system (SARS-CoV-2) that includes an asymptomatic infectious population. While the importance of asymptomatic transmission was debated early in the pandemic, many studies have affirmed its role in the spread of disease [25,26,27]. Though environmental transmission of SARS-CoV-2 remains a controversial topic, it is plausible that asymptomatic individuals may spread disease through frequent contact with the environment, thus increasing the proportion of virus that is free-living [28]. We acknowledge that mathematical models of epidemics can be limited by “identifiability,” which can obfuscate the relative importance of some routes of transmission. In models that have both indirect and direct routes of transmission, it can be very difficult to conclude that one route is predominant [29,30,31]. (1) dSdt=μN−S−βAA+βIIN+βWWS (2) dEdt=βAA+βIIN+βWWS−ε+μE (3) dAdt=εE−ω+μA (4) dIdt=1−pωA−v+μII (5) dRdt=pωA+vI−μR (6) dWdt=σAA+σIIN1−W−kW\nFigure 1 depicts the compartmental diagram for the model. The direction of the arrows corresponds to the flow of the individuals and the pathogen through the system. Note that individuals can move directly from the asymptomatically infected compartment to the recovered compartment (bypassing the symptomatic compartment) via what we call a “mild track”. The dashed arrows represent WAIT coupling to the environment. The model is inspired by one developed to interrogate environmental transmission of SARS-CoV-2 [22].\n\n2.3. Simulations of Outbreaks\nThe system was numerically integrated using the “odeint” solver in the Scipy 1.4—Python scientific computation suite [32]. The simulations track the populations for each of the compartments listed in Figure 1. Each model run occurred over 250 days, which amounts to over 8 months of the epidemic or 5× the peak of the outbreak. This length of time is consistent with the antecedent SARS-CoV-2 model [22], long enough for the dynamics of the system to manifest. Note however that, for this study, we are especially interested in the early window of an outbreak: the first 30 days. We focus on this window because this is the time frame that best captures the underlying physics of an epidemic, as 30 days is often before populations are able to adjust their individual behaviors. The code constructed for the analysis in this study is publicly available on github: https://github.com/OgPlexus/Pharaohlocks.\n\n2.4. Population Definitions and Parameter Values\nTable 1 outlines the definitions of each population and provides the initial population values used for all simulations conducted in this study. The nominal parameter values used are defined in Table 2. The initial values are drawn from the aforementioned COVID-19 outbreak study, derived from empirical findings and country-level outbreak data [22].\n\n2.5. Virulence Definition\nIn this study, we define virulence as the capacity to cause a disease. In order to measure it, we utilize a set of parameters that uniformly increase the rate or probability of causing symptomatic disease or the severity of those symptoms (including death). Our definition is more comprehensive than many other models of parasite virulence (e.g., [4,13]), which tend to focus on a single aspect of the natural history of disease associated with harm to a host (e.g., the fitness consequences of an infection on the host population or the case fatality rate). Instead of having to justify a definition built around a single term (e.g., the term associated with fatality), we took a collective approach to defining virulence through all terms that foment the viral-induced onset of symptomatic disease and death. This definition allows for the reality of pleiotropic effects in viral pathogens, where adaptations can have multiple effects on the natural history of disease [2,33]. Our definition of virulence emphasizes terms that influence host wellness and/or are symptoms of disease. The iteration of virulence used in this study also undermines the potential for overly weighting only one or a small number of parameters under a large umbrella of virulence. Because so many varying definitions exist for virulence, we have also performed calculations according to a different definition of virulence, one that exclusively considers terms that have a detrimental direct effect on the host and neither of the terms that reflect symptoms of severe disease (𝜎a and 𝜎I). These calculations can be found in the Supplementary Materials.\nThe collection of parameters that we use to define virulence are as follows: the infected population death rate (𝜇I), the incubation period of SARS-CoV-2 (𝜂), the rate of transfer from asymptomatic to symptomatic (1/⍵), the infected population recovery rate (ν), the percent of individuals that move from the asymptomatic to the recovered compartment without showing symptoms (the “mild” recovery track, p), the contact rate of people with people × the transmission probability of people to people by an asymptomatic individual (βA), the contact rate of people with people × the transmission probability of people to people by an asymptomatically infected person (βI), the contact rate of people with the environment × the probability of shedding by an asymptomatic individual to the environmental (𝜎A), the contact rate of people with the environment × the probability of symptomatically infected individuals shedding in the environment (𝜎I), and the average number of days before infection (1/ε).\nTable 3 outlines the direction in which each of the virulence-associated parameters are modulated as virulence decreases or increases. An up arrow (↑) indicates the parameter increases (by an equivalent percent) when the percent virulence is changed. A down arrow (↓) indicates the parameter decreases (by an equivalent percent) when the percent change in virulence is applied. Changes in virulence are then defined, in this study, as an equivalent uniform (percent) change in each of the parameters listed above. For the purposes of our study, we modify virulence by changing all parameters associated with virulence by 5%. One could also disambiguate virulence into changes in individual subcomponents; however, that is not the focus of this current study.\n\n2.6. Survival Definition\nSurvival is defined as the set of parameters that, when uniformly modulated, increases the pathogen’s probability of surviving the outside environment and successfully infecting a new host [2]. In our model, this includes both the waning virus rate in the environment (k) and the contact rate of an individual with the environment × the transmission probability of the environment to people (βw). Table 4 outlines the direction (increasing or decreasing) in which these parameters are modulated when survival is decreased or increased. Within both models, a (percent) change in survival is defined as an equivalent uniform (percent) change in the survival parameters.\nThroughout this study, the impact of changes in virulence and survival (and the relationship between these traits) are assessed with respect to the following four epidemic metrics: the number of infected individuals (asymptomatic and symptomatic) at the maximum (when the outbreak is at its most severe), the rate at which the peak infected population is reached (tmax−1), the total infected population after 30 days, and the basic reproductive ratio (R0). Importantly, among these signatures, the basic reproductive ratio is the most frequently used in epidemiology and benefits from familiarity and mathematical formalism (see Section 2.7). The other signatures are determined through simulations of an epidemic for a given set of parameter values. Nonetheless, this study’s inclusion of multiple features of the epidemic allows us to examine how variation in virus life-history traits may influence different aspects of an epidemic in peculiar ways.\n\n2.7. Basic Reproductive Ratio\nEquations (7)–(9) give the analytic expression of the basic reproductive ratio (R0) for the model used in this study. This expression for R0 can be deconstructed into two components. Equation (8) only contains parameters associated with person to person transmission (Rp), while Equation (9) solely contains parameters associated with transmission from the environment (Re). In the Supplementary Materials, we provide additional information on these terms and their derivations. Applying the parameters values in Table 2, the numerical value of the basic reproductive ratio is given as R0 ~ 2.82. (7) R0=RpRp2+4Re22 (8) Rp=εβAμI+v+βI1−pωμ+εμ+ωμI+v (9) Re2=εβWσAμI+v+σI1−pωkμ+εμ+ωμI+v"}
LitCovid-sentences
{"project":"LitCovid-sentences","denotations":[{"id":"T45","span":{"begin":0,"end":2},"obj":"Sentence"},{"id":"T46","span":{"begin":3,"end":24},"obj":"Sentence"},{"id":"T47","span":{"begin":26,"end":30},"obj":"Sentence"},{"id":"T48","span":{"begin":31,"end":63},"obj":"Sentence"},{"id":"T49","span":{"begin":64,"end":359},"obj":"Sentence"},{"id":"T50","span":{"begin":360,"end":462},"obj":"Sentence"},{"id":"T51","span":{"begin":463,"end":707},"obj":"Sentence"},{"id":"T52","span":{"begin":708,"end":848},"obj":"Sentence"},{"id":"T53","span":{"begin":849,"end":1001},"obj":"Sentence"},{"id":"T54","span":{"begin":1002,"end":1095},"obj":"Sentence"},{"id":"T55","span":{"begin":1096,"end":1323},"obj":"Sentence"},{"id":"T56","span":{"begin":1324,"end":1583},"obj":"Sentence"},{"id":"T57","span":{"begin":1585,"end":1589},"obj":"Sentence"},{"id":"T58","span":{"begin":1590,"end":1607},"obj":"Sentence"},{"id":"T59","span":{"begin":1608,"end":1708},"obj":"Sentence"},{"id":"T60","span":{"begin":1709,"end":1907},"obj":"Sentence"},{"id":"T61","span":{"begin":1908,"end":2151},"obj":"Sentence"},{"id":"T62","span":{"begin":2152,"end":2514},"obj":"Sentence"},{"id":"T63","span":{"begin":2515,"end":2672},"obj":"Sentence"},{"id":"T64","span":{"begin":2673,"end":2929},"obj":"Sentence"},{"id":"T65","span":{"begin":2930,"end":3095},"obj":"Sentence"},{"id":"T66","span":{"begin":3096,"end":3380},"obj":"Sentence"},{"id":"T67","span":{"begin":3381,"end":3438},"obj":"Sentence"},{"id":"T68","span":{"begin":3439,"end":3546},"obj":"Sentence"},{"id":"T69","span":{"begin":3547,"end":3735},"obj":"Sentence"},{"id":"T70","span":{"begin":3736,"end":3797},"obj":"Sentence"},{"id":"T71","span":{"begin":3798,"end":3898},"obj":"Sentence"},{"id":"T72","span":{"begin":3900,"end":3904},"obj":"Sentence"},{"id":"T73","span":{"begin":3905,"end":3929},"obj":"Sentence"},{"id":"T74","span":{"begin":3930,"end":4052},"obj":"Sentence"},{"id":"T75","span":{"begin":4053,"end":4139},"obj":"Sentence"},{"id":"T76","span":{"begin":4140,"end":4257},"obj":"Sentence"},{"id":"T77","span":{"begin":4258,"end":4390},"obj":"Sentence"},{"id":"T78","span":{"begin":4391,"end":4509},"obj":"Sentence"},{"id":"T79","span":{"begin":4510,"end":4708},"obj":"Sentence"},{"id":"T80","span":{"begin":4709,"end":4835},"obj":"Sentence"},{"id":"T81","span":{"begin":4837,"end":4841},"obj":"Sentence"},{"id":"T82","span":{"begin":4842,"end":4885},"obj":"Sentence"},{"id":"T83","span":{"begin":4886,"end":5030},"obj":"Sentence"},{"id":"T84","span":{"begin":5031,"end":5088},"obj":"Sentence"},{"id":"T85","span":{"begin":5089,"end":5236},"obj":"Sentence"},{"id":"T86","span":{"begin":5238,"end":5242},"obj":"Sentence"},{"id":"T87","span":{"begin":5243,"end":5263},"obj":"Sentence"},{"id":"T88","span":{"begin":5264,"end":5334},"obj":"Sentence"},{"id":"T89","span":{"begin":5335,"end":5521},"obj":"Sentence"},{"id":"T90","span":{"begin":5522,"end":5822},"obj":"Sentence"},{"id":"T91","span":{"begin":5823,"end":6074},"obj":"Sentence"},{"id":"T92","span":{"begin":6075,"end":6242},"obj":"Sentence"},{"id":"T93","span":{"begin":6243,"end":6348},"obj":"Sentence"},{"id":"T94","span":{"begin":6349,"end":6523},"obj":"Sentence"},{"id":"T95","span":{"begin":6524,"end":6831},"obj":"Sentence"},{"id":"T96","span":{"begin":6832,"end":6895},"obj":"Sentence"},{"id":"T97","span":{"begin":6896,"end":7894},"obj":"Sentence"},{"id":"T98","span":{"begin":7895,"end":8029},"obj":"Sentence"},{"id":"T99","span":{"begin":8030,"end":8145},"obj":"Sentence"},{"id":"T100","span":{"begin":8146,"end":8272},"obj":"Sentence"},{"id":"T101","span":{"begin":8273,"end":8408},"obj":"Sentence"},{"id":"T102","span":{"begin":8409,"end":8519},"obj":"Sentence"},{"id":"T103","span":{"begin":8520,"end":8653},"obj":"Sentence"},{"id":"T104","span":{"begin":8655,"end":8659},"obj":"Sentence"},{"id":"T105","span":{"begin":8660,"end":8679},"obj":"Sentence"},{"id":"T106","span":{"begin":8680,"end":8873},"obj":"Sentence"},{"id":"T107","span":{"begin":8874,"end":9076},"obj":"Sentence"},{"id":"T108","span":{"begin":9077,"end":9215},"obj":"Sentence"},{"id":"T109","span":{"begin":9216,"end":9347},"obj":"Sentence"},{"id":"T110","span":{"begin":9348,"end":9804},"obj":"Sentence"},{"id":"T111","span":{"begin":9805,"end":9990},"obj":"Sentence"},{"id":"T112","span":{"begin":9991,"end":10098},"obj":"Sentence"},{"id":"T113","span":{"begin":10099,"end":10300},"obj":"Sentence"},{"id":"T114","span":{"begin":10302,"end":10306},"obj":"Sentence"},{"id":"T115","span":{"begin":10307,"end":10331},"obj":"Sentence"},{"id":"T116","span":{"begin":10332,"end":10449},"obj":"Sentence"},{"id":"T117","span":{"begin":10450,"end":10514},"obj":"Sentence"},{"id":"T118","span":{"begin":10515,"end":10706},"obj":"Sentence"},{"id":"T119","span":{"begin":10707,"end":10810},"obj":"Sentence"},{"id":"T120","span":{"begin":10811,"end":11015},"obj":"Sentence"}],"namespaces":[{"prefix":"_base","uri":"http://pubannotation.org/ontology/tao.owl#"}],"text":"2. Materials and Methods\n\n2.1. Model Motivation and Application\nThe mathematical model explored in this study is adapted from a recent one developed to investigate environmental transmission of SARS-CoV-2 during the early-stage outbreak dynamics of coronavirus disease 2019 (COVID-19), with parameter values based on fits to actual country outbreak data [22]. In this study, we utilize this model to examine questions about the evolution of free-living survival. While the phenomenon we examine is a very relevant one that manifests in the real world, we want to emphasize that none of the methods or results in this study are intended to be applied to the current COVID-19 pandemic (as of September, 2020). This study is an attempt at responsible theoretical biology, with data-informed models and inferences that are germane to the natural world. However, neither do we support the extrapolation of these findings to any particular aspect of COVID-19 nor should they inform a policy or intervention. The model applies to a number of scenarios that include outbreaks in a naïve host population. This describes situations such as the evolution of novel viral lineages, viral spillover events, or host shifts, where a virus with a preexisting relationship between virulence and survival emerges in a population of new hosts. Another such scenario where this model applies is one where a virus has already emerged but evolves in a subpopulation in the novel hosts before a migration event of some kind introduces the evolved virus population to a fully susceptible population of hosts.\n\n2.2. Model Description\nThe model is implemented via a set of ordinary differential equations, defined by Equations (1)–(6). It implements viral free-living survival via the “Waterborne Abiotic or other Indirect Transmission (WAIT)” modelling framework, coupling individuals and the pathogen within the environment [23,24].\nWithin the model, the βw term allows for individuals to become infected via viral pathogen deposited in the environment and terms 𝜎A and 𝜎I allow asymptomatic and symptomatic individuals to deposit pathogens into the environment, respectively. Adapted from the more traditional SEIR (susceptible-exposed-infected-recovered) model, the SEAIR-W (susceptible-exposed-asymptomatic-infected-recovered-WAIT) model interrogates the consequences of the two hypotheses outlined above while representing the dynamics of a very relevant disease system (SARS-CoV-2) that includes an asymptomatic infectious population. While the importance of asymptomatic transmission was debated early in the pandemic, many studies have affirmed its role in the spread of disease [25,26,27]. Though environmental transmission of SARS-CoV-2 remains a controversial topic, it is plausible that asymptomatic individuals may spread disease through frequent contact with the environment, thus increasing the proportion of virus that is free-living [28]. We acknowledge that mathematical models of epidemics can be limited by “identifiability,” which can obfuscate the relative importance of some routes of transmission. In models that have both indirect and direct routes of transmission, it can be very difficult to conclude that one route is predominant [29,30,31]. (1) dSdt=μN−S−βAA+βIIN+βWWS (2) dEdt=βAA+βIIN+βWWS−ε+μE (3) dAdt=εE−ω+μA (4) dIdt=1−pωA−v+μII (5) dRdt=pωA+vI−μR (6) dWdt=σAA+σIIN1−W−kW\nFigure 1 depicts the compartmental diagram for the model. The direction of the arrows corresponds to the flow of the individuals and the pathogen through the system. Note that individuals can move directly from the asymptomatically infected compartment to the recovered compartment (bypassing the symptomatic compartment) via what we call a “mild track”. The dashed arrows represent WAIT coupling to the environment. The model is inspired by one developed to interrogate environmental transmission of SARS-CoV-2 [22].\n\n2.3. Simulations of Outbreaks\nThe system was numerically integrated using the “odeint” solver in the Scipy 1.4—Python scientific computation suite [32]. The simulations track the populations for each of the compartments listed in Figure 1. Each model run occurred over 250 days, which amounts to over 8 months of the epidemic or 5× the peak of the outbreak. This length of time is consistent with the antecedent SARS-CoV-2 model [22], long enough for the dynamics of the system to manifest. Note however that, for this study, we are especially interested in the early window of an outbreak: the first 30 days. We focus on this window because this is the time frame that best captures the underlying physics of an epidemic, as 30 days is often before populations are able to adjust their individual behaviors. The code constructed for the analysis in this study is publicly available on github: https://github.com/OgPlexus/Pharaohlocks.\n\n2.4. Population Definitions and Parameter Values\nTable 1 outlines the definitions of each population and provides the initial population values used for all simulations conducted in this study. The nominal parameter values used are defined in Table 2. The initial values are drawn from the aforementioned COVID-19 outbreak study, derived from empirical findings and country-level outbreak data [22].\n\n2.5. Virulence Definition\nIn this study, we define virulence as the capacity to cause a disease. In order to measure it, we utilize a set of parameters that uniformly increase the rate or probability of causing symptomatic disease or the severity of those symptoms (including death). Our definition is more comprehensive than many other models of parasite virulence (e.g., [4,13]), which tend to focus on a single aspect of the natural history of disease associated with harm to a host (e.g., the fitness consequences of an infection on the host population or the case fatality rate). Instead of having to justify a definition built around a single term (e.g., the term associated with fatality), we took a collective approach to defining virulence through all terms that foment the viral-induced onset of symptomatic disease and death. This definition allows for the reality of pleiotropic effects in viral pathogens, where adaptations can have multiple effects on the natural history of disease [2,33]. Our definition of virulence emphasizes terms that influence host wellness and/or are symptoms of disease. The iteration of virulence used in this study also undermines the potential for overly weighting only one or a small number of parameters under a large umbrella of virulence. Because so many varying definitions exist for virulence, we have also performed calculations according to a different definition of virulence, one that exclusively considers terms that have a detrimental direct effect on the host and neither of the terms that reflect symptoms of severe disease (𝜎a and 𝜎I). These calculations can be found in the Supplementary Materials.\nThe collection of parameters that we use to define virulence are as follows: the infected population death rate (𝜇I), the incubation period of SARS-CoV-2 (𝜂), the rate of transfer from asymptomatic to symptomatic (1/⍵), the infected population recovery rate (ν), the percent of individuals that move from the asymptomatic to the recovered compartment without showing symptoms (the “mild” recovery track, p), the contact rate of people with people × the transmission probability of people to people by an asymptomatic individual (βA), the contact rate of people with people × the transmission probability of people to people by an asymptomatically infected person (βI), the contact rate of people with the environment × the probability of shedding by an asymptomatic individual to the environmental (𝜎A), the contact rate of people with the environment × the probability of symptomatically infected individuals shedding in the environment (𝜎I), and the average number of days before infection (1/ε).\nTable 3 outlines the direction in which each of the virulence-associated parameters are modulated as virulence decreases or increases. An up arrow (↑) indicates the parameter increases (by an equivalent percent) when the percent virulence is changed. A down arrow (↓) indicates the parameter decreases (by an equivalent percent) when the percent change in virulence is applied. Changes in virulence are then defined, in this study, as an equivalent uniform (percent) change in each of the parameters listed above. For the purposes of our study, we modify virulence by changing all parameters associated with virulence by 5%. One could also disambiguate virulence into changes in individual subcomponents; however, that is not the focus of this current study.\n\n2.6. Survival Definition\nSurvival is defined as the set of parameters that, when uniformly modulated, increases the pathogen’s probability of surviving the outside environment and successfully infecting a new host [2]. In our model, this includes both the waning virus rate in the environment (k) and the contact rate of an individual with the environment × the transmission probability of the environment to people (βw). Table 4 outlines the direction (increasing or decreasing) in which these parameters are modulated when survival is decreased or increased. Within both models, a (percent) change in survival is defined as an equivalent uniform (percent) change in the survival parameters.\nThroughout this study, the impact of changes in virulence and survival (and the relationship between these traits) are assessed with respect to the following four epidemic metrics: the number of infected individuals (asymptomatic and symptomatic) at the maximum (when the outbreak is at its most severe), the rate at which the peak infected population is reached (tmax−1), the total infected population after 30 days, and the basic reproductive ratio (R0). Importantly, among these signatures, the basic reproductive ratio is the most frequently used in epidemiology and benefits from familiarity and mathematical formalism (see Section 2.7). The other signatures are determined through simulations of an epidemic for a given set of parameter values. Nonetheless, this study’s inclusion of multiple features of the epidemic allows us to examine how variation in virus life-history traits may influence different aspects of an epidemic in peculiar ways.\n\n2.7. Basic Reproductive Ratio\nEquations (7)–(9) give the analytic expression of the basic reproductive ratio (R0) for the model used in this study. This expression for R0 can be deconstructed into two components. Equation (8) only contains parameters associated with person to person transmission (Rp), while Equation (9) solely contains parameters associated with transmission from the environment (Re). In the Supplementary Materials, we provide additional information on these terms and their derivations. Applying the parameters values in Table 2, the numerical value of the basic reproductive ratio is given as R0 ~ 2.82. (7) R0=RpRp2+4Re22 (8) Rp=εβAμI+v+βI1−pωμ+εμ+ωμI+v (9) Re2=εβWσAμI+v+σI1−pωkμ+εμ+ωμI+v"}