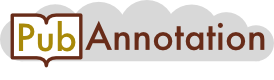
PMC:7551987 / 18718-25807
Annnotations
2_test
{"project":"2_test","denotations":[{"id":"32971954-32083643-143921962","span":{"begin":768,"end":770},"obj":"32083643"},{"id":"32971954-32446187-143921963","span":{"begin":771,"end":773},"obj":"32446187"},{"id":"32971954-15682873-143921964","span":{"begin":3195,"end":3197},"obj":"15682873"},{"id":"32971954-25087040-143921965","span":{"begin":4257,"end":4258},"obj":"25087040"},{"id":"32971954-23594543-143921966","span":{"begin":4259,"end":4260},"obj":"23594543"},{"id":"32971954-16756387-143921967","span":{"begin":4261,"end":4263},"obj":"16756387"}],"text":"3. Results\n\n3.1. Model Sensitivity Analysis\nFigure 2 depicts a tornado plot that communicates the sensitivity of the model to permutations in parameters. Across features, the model is most sensitive to parameters that are considered virulence-associated (Table 3) and is relatively less sensitive to survival-associated parameters (Table 4). Similar to other features, R0 (Figure 2D) of the model is most sensitive to the parameters ⍵, βA, and ν. The sensitivity of R0 to changes in ⍵ reflects the importance of the rate of conversion to the symptomatic state on model dynamics. In addition, βA has a very important influence on the model, consistent with other findings for COVID-19 that have emphasized the importance of asymptomatic transmission in disease spread [25,26,27].\n\n3.2. Illustrative Dynamics of Model System\nBased on the parameter values in Table 2, Figure 3A demonstrates the base dynamics of the model playing out over the first 100 days while Figure 3B shows the dynamics within the environment over the course of 250 days. In these dynamics, the population begins to be fixed for susceptible hosts. The disease dynamics manifest in the shapes of the curves corresponding to exposed, asymptomatic, and symptomatic individuals. Note the long tail of the curve corresponding to contamination by the environment. The environment remains infectious even after the infected populations have declined in number. The length and shape of this tail are influenced by the free-living survival of the virus.\n\n3.3. The Epidemic Consequences of Varying Virulence and Survival\nIn the next analysis, we examine the epidemic consequences of varying traits associated with survival and virulence. One can consider this as a scenario where we compare the endpoints of evolution of different virus populations (corresponding to combinations of values of survival and virulence) and calculating how these evolved populations manifest in epidemic terms.\nIn Figure 4, we observe how dynamics of the outbreak are influenced across a space of combinations of traits altering virulence (see Table 3 for a list of virulence-associated parameters) and survival (see Table 4 for a list of survival-associated parameters), changed by ±5% (10% overall). In Figure 4D, we demonstrate how changes in virulence and free-living survival traits influence R0, with variation in virulence-related traits having the largest effect on R0. Of note is how the range in R0 values varies widely across virulence–survival values, from nearly 2.0 to 3.7 (Figure 4D).\n\n3.4. Implications of Virulence–Survival Relationships at Their Relative Extremes\nHaving observed how outbreak dynamics are influenced by variation in traits that alter virulence–survival phenotypes, we then examined how each outbreak metric is influenced by the extreme (±5%) values of the trait combinations considered. Specifically, we assess how a change in pathogen survival affects outbreak dynamics, based on two expected relationships between survival and virulence traits.\n\n3.5. Positive Correlation Between Survival and Virulence\nIn a positive correlation scenario, high values for survival would be associated with high values for virulence [4,13]. Because the correlations we observe are often not exactly linear, we utilize quadrants to express a trend, allowing for some variance around the expected “line”. In Figure 5, the positive correlation scenario can be represented by combinations of virulence and survival residing in quadrants I and III.\nIf host–pathogen evolution proceeds according to a positive correlation scenario, all outbreak metrics would show an increase in severity as both survival and virulence increase. Across the range of variation in virulence and survival traits considered (5% above and below the nominal value), the peak number of infected individuals increases by approximately 35%, the rate at which the peak is reached increases by approximately 16%, the total number of infected individuals after 30 days increases by approximately 98%, and R0 increases by approximately 94% (Figure 6 and Table 5).\n\n3.6. Negative Correlation Between Survival and Virulence\nIn a negative correlation scenario, high values for survival would be associated with low values for virulence [2,9,15] and a low peak in total infected population. Pathogens with a life history that exhibits negative virulence–survival associations would likely appear in quadrants II and IV in Figure 5.\nUnder negative correlation, outbreak severity decreases as survival increases. Across the measured range of variation in virulence–survival traits, the peak number of infected individuals decreases by approximately 23%, the rate at which the epidemic peak is reached decreases by 0.15%, the total number of infected individuals decreases by 3%, and R0 decreases by approximately 84% (Figure 6 and Table 6). Across all metrics considered, the effects of increased viral survival on outbreak dynamics is more extreme under the positive correlation than the negative correlation scenarios (Figure 6).\n\n3.7. Dynamics of Epidemics at Extreme Values for Virus Free-Living Survival\nIn Figure 7, we observe the disease dynamics at extreme values for survival and the dynamics corresponding to the fraction of the environment that is contaminated with infectious virus. Consistent with the data represented in Figure 6, we observe that minimum and maximum simulations differ more substantially for extreme survival scenarios in the positive correlation scenario than for the negative correlation scenario.\nThe feature of different outbreaks that varies most ostensibly between the correlation scenarios is the time needed to reach the peak number of infected individuals. In positive correlation simulations, one can observe that the low virulence, low survival scenario (Figure 7A,B) takes longer to reach the peak number of infected individuals. Most notably, however, the low virulence, low survival setting has a far smaller peak of environmental contamination and shorter tail relative to its high virulence, high survival counterpart (Figure 7D). Similarly, intriguing findings exist in the comparison between the simulation sets corresponding to extremes in the negative correlation setting (Figure 7E–H). Especially notable is the difference in the length of the tail of the environmental contamination for the high virulence, low survival combination (Figure 7F) vs. the low virulence, low survival combination variant (Figure 7H). The explanation is that, in this model, higher virulence influences (among many other things) the rate at which the virus is shed into the environment from either the asymptomatic (𝜎A) or symptomatic (𝜎I) host. We observe how the high virulence, low survival simulation (Figure 7E) features a symptomatic peak that is larger in size and is prolonged relative to the lower virulence counterpart (Figure 7G). This relatively large symptomatic population sheds infectious virus into the environment for a longer period of time, contributing to the long tail of contaminated environments observed in Figure 5F."}
LitCovid-PubTator
{"project":"LitCovid-PubTator","denotations":[{"id":"113","span":{"begin":675,"end":683},"obj":"Disease"},{"id":"115","span":{"begin":1378,"end":1386},"obj":"Disease"},{"id":"118","span":{"begin":3815,"end":3823},"obj":"Disease"},{"id":"119","span":{"begin":3958,"end":3966},"obj":"Disease"},{"id":"121","span":{"begin":4289,"end":4297},"obj":"Disease"},{"id":"124","span":{"begin":4618,"end":4626},"obj":"Disease"},{"id":"125","span":{"begin":4758,"end":4766},"obj":"Disease"},{"id":"128","span":{"begin":5692,"end":5700},"obj":"Disease"},{"id":"129","span":{"begin":5868,"end":5876},"obj":"Disease"}],"attributes":[{"id":"A113","pred":"tao:has_database_id","subj":"113","obj":"MESH:C000657245"},{"id":"A115","pred":"tao:has_database_id","subj":"115","obj":"MESH:D007239"},{"id":"A118","pred":"tao:has_database_id","subj":"118","obj":"MESH:D007239"},{"id":"A119","pred":"tao:has_database_id","subj":"119","obj":"MESH:D007239"},{"id":"A121","pred":"tao:has_database_id","subj":"121","obj":"MESH:D007239"},{"id":"A124","pred":"tao:has_database_id","subj":"124","obj":"MESH:D007239"},{"id":"A125","pred":"tao:has_database_id","subj":"125","obj":"MESH:D007239"},{"id":"A128","pred":"tao:has_database_id","subj":"128","obj":"MESH:D007239"},{"id":"A129","pred":"tao:has_database_id","subj":"129","obj":"MESH:D007239"}],"namespaces":[{"prefix":"Tax","uri":"https://www.ncbi.nlm.nih.gov/taxonomy/"},{"prefix":"MESH","uri":"https://id.nlm.nih.gov/mesh/"},{"prefix":"Gene","uri":"https://www.ncbi.nlm.nih.gov/gene/"},{"prefix":"CVCL","uri":"https://web.expasy.org/cellosaurus/CVCL_"}],"text":"3. Results\n\n3.1. Model Sensitivity Analysis\nFigure 2 depicts a tornado plot that communicates the sensitivity of the model to permutations in parameters. Across features, the model is most sensitive to parameters that are considered virulence-associated (Table 3) and is relatively less sensitive to survival-associated parameters (Table 4). Similar to other features, R0 (Figure 2D) of the model is most sensitive to the parameters ⍵, βA, and ν. The sensitivity of R0 to changes in ⍵ reflects the importance of the rate of conversion to the symptomatic state on model dynamics. In addition, βA has a very important influence on the model, consistent with other findings for COVID-19 that have emphasized the importance of asymptomatic transmission in disease spread [25,26,27].\n\n3.2. Illustrative Dynamics of Model System\nBased on the parameter values in Table 2, Figure 3A demonstrates the base dynamics of the model playing out over the first 100 days while Figure 3B shows the dynamics within the environment over the course of 250 days. In these dynamics, the population begins to be fixed for susceptible hosts. The disease dynamics manifest in the shapes of the curves corresponding to exposed, asymptomatic, and symptomatic individuals. Note the long tail of the curve corresponding to contamination by the environment. The environment remains infectious even after the infected populations have declined in number. The length and shape of this tail are influenced by the free-living survival of the virus.\n\n3.3. The Epidemic Consequences of Varying Virulence and Survival\nIn the next analysis, we examine the epidemic consequences of varying traits associated with survival and virulence. One can consider this as a scenario where we compare the endpoints of evolution of different virus populations (corresponding to combinations of values of survival and virulence) and calculating how these evolved populations manifest in epidemic terms.\nIn Figure 4, we observe how dynamics of the outbreak are influenced across a space of combinations of traits altering virulence (see Table 3 for a list of virulence-associated parameters) and survival (see Table 4 for a list of survival-associated parameters), changed by ±5% (10% overall). In Figure 4D, we demonstrate how changes in virulence and free-living survival traits influence R0, with variation in virulence-related traits having the largest effect on R0. Of note is how the range in R0 values varies widely across virulence–survival values, from nearly 2.0 to 3.7 (Figure 4D).\n\n3.4. Implications of Virulence–Survival Relationships at Their Relative Extremes\nHaving observed how outbreak dynamics are influenced by variation in traits that alter virulence–survival phenotypes, we then examined how each outbreak metric is influenced by the extreme (±5%) values of the trait combinations considered. Specifically, we assess how a change in pathogen survival affects outbreak dynamics, based on two expected relationships between survival and virulence traits.\n\n3.5. Positive Correlation Between Survival and Virulence\nIn a positive correlation scenario, high values for survival would be associated with high values for virulence [4,13]. Because the correlations we observe are often not exactly linear, we utilize quadrants to express a trend, allowing for some variance around the expected “line”. In Figure 5, the positive correlation scenario can be represented by combinations of virulence and survival residing in quadrants I and III.\nIf host–pathogen evolution proceeds according to a positive correlation scenario, all outbreak metrics would show an increase in severity as both survival and virulence increase. Across the range of variation in virulence and survival traits considered (5% above and below the nominal value), the peak number of infected individuals increases by approximately 35%, the rate at which the peak is reached increases by approximately 16%, the total number of infected individuals after 30 days increases by approximately 98%, and R0 increases by approximately 94% (Figure 6 and Table 5).\n\n3.6. Negative Correlation Between Survival and Virulence\nIn a negative correlation scenario, high values for survival would be associated with low values for virulence [2,9,15] and a low peak in total infected population. Pathogens with a life history that exhibits negative virulence–survival associations would likely appear in quadrants II and IV in Figure 5.\nUnder negative correlation, outbreak severity decreases as survival increases. Across the measured range of variation in virulence–survival traits, the peak number of infected individuals decreases by approximately 23%, the rate at which the epidemic peak is reached decreases by 0.15%, the total number of infected individuals decreases by 3%, and R0 decreases by approximately 84% (Figure 6 and Table 6). Across all metrics considered, the effects of increased viral survival on outbreak dynamics is more extreme under the positive correlation than the negative correlation scenarios (Figure 6).\n\n3.7. Dynamics of Epidemics at Extreme Values for Virus Free-Living Survival\nIn Figure 7, we observe the disease dynamics at extreme values for survival and the dynamics corresponding to the fraction of the environment that is contaminated with infectious virus. Consistent with the data represented in Figure 6, we observe that minimum and maximum simulations differ more substantially for extreme survival scenarios in the positive correlation scenario than for the negative correlation scenario.\nThe feature of different outbreaks that varies most ostensibly between the correlation scenarios is the time needed to reach the peak number of infected individuals. In positive correlation simulations, one can observe that the low virulence, low survival scenario (Figure 7A,B) takes longer to reach the peak number of infected individuals. Most notably, however, the low virulence, low survival setting has a far smaller peak of environmental contamination and shorter tail relative to its high virulence, high survival counterpart (Figure 7D). Similarly, intriguing findings exist in the comparison between the simulation sets corresponding to extremes in the negative correlation setting (Figure 7E–H). Especially notable is the difference in the length of the tail of the environmental contamination for the high virulence, low survival combination (Figure 7F) vs. the low virulence, low survival combination variant (Figure 7H). The explanation is that, in this model, higher virulence influences (among many other things) the rate at which the virus is shed into the environment from either the asymptomatic (𝜎A) or symptomatic (𝜎I) host. We observe how the high virulence, low survival simulation (Figure 7E) features a symptomatic peak that is larger in size and is prolonged relative to the lower virulence counterpart (Figure 7G). This relatively large symptomatic population sheds infectious virus into the environment for a longer period of time, contributing to the long tail of contaminated environments observed in Figure 5F."}
LitCovid-sentences
{"project":"LitCovid-sentences","denotations":[{"id":"T121","span":{"begin":0,"end":2},"obj":"Sentence"},{"id":"T122","span":{"begin":3,"end":10},"obj":"Sentence"},{"id":"T123","span":{"begin":12,"end":16},"obj":"Sentence"},{"id":"T124","span":{"begin":17,"end":43},"obj":"Sentence"},{"id":"T125","span":{"begin":44,"end":153},"obj":"Sentence"},{"id":"T126","span":{"begin":154,"end":341},"obj":"Sentence"},{"id":"T127","span":{"begin":342,"end":446},"obj":"Sentence"},{"id":"T128","span":{"begin":447,"end":578},"obj":"Sentence"},{"id":"T129","span":{"begin":579,"end":778},"obj":"Sentence"},{"id":"T130","span":{"begin":780,"end":784},"obj":"Sentence"},{"id":"T131","span":{"begin":785,"end":822},"obj":"Sentence"},{"id":"T132","span":{"begin":823,"end":1041},"obj":"Sentence"},{"id":"T133","span":{"begin":1042,"end":1117},"obj":"Sentence"},{"id":"T134","span":{"begin":1118,"end":1244},"obj":"Sentence"},{"id":"T135","span":{"begin":1245,"end":1327},"obj":"Sentence"},{"id":"T136","span":{"begin":1328,"end":1423},"obj":"Sentence"},{"id":"T137","span":{"begin":1424,"end":1514},"obj":"Sentence"},{"id":"T138","span":{"begin":1516,"end":1520},"obj":"Sentence"},{"id":"T139","span":{"begin":1521,"end":1580},"obj":"Sentence"},{"id":"T140","span":{"begin":1581,"end":1697},"obj":"Sentence"},{"id":"T141","span":{"begin":1698,"end":1950},"obj":"Sentence"},{"id":"T142","span":{"begin":1951,"end":2241},"obj":"Sentence"},{"id":"T143","span":{"begin":2242,"end":2417},"obj":"Sentence"},{"id":"T144","span":{"begin":2418,"end":2539},"obj":"Sentence"},{"id":"T145","span":{"begin":2541,"end":2545},"obj":"Sentence"},{"id":"T146","span":{"begin":2546,"end":2621},"obj":"Sentence"},{"id":"T147","span":{"begin":2622,"end":2861},"obj":"Sentence"},{"id":"T148","span":{"begin":2862,"end":3021},"obj":"Sentence"},{"id":"T149","span":{"begin":3023,"end":3027},"obj":"Sentence"},{"id":"T150","span":{"begin":3028,"end":3079},"obj":"Sentence"},{"id":"T151","span":{"begin":3080,"end":3199},"obj":"Sentence"},{"id":"T152","span":{"begin":3200,"end":3361},"obj":"Sentence"},{"id":"T153","span":{"begin":3362,"end":3502},"obj":"Sentence"},{"id":"T154","span":{"begin":3503,"end":3681},"obj":"Sentence"},{"id":"T155","span":{"begin":3682,"end":4086},"obj":"Sentence"},{"id":"T156","span":{"begin":4088,"end":4092},"obj":"Sentence"},{"id":"T157","span":{"begin":4093,"end":4144},"obj":"Sentence"},{"id":"T158","span":{"begin":4145,"end":4309},"obj":"Sentence"},{"id":"T159","span":{"begin":4310,"end":4450},"obj":"Sentence"},{"id":"T160","span":{"begin":4451,"end":4529},"obj":"Sentence"},{"id":"T161","span":{"begin":4530,"end":4857},"obj":"Sentence"},{"id":"T162","span":{"begin":4858,"end":5048},"obj":"Sentence"},{"id":"T163","span":{"begin":5050,"end":5054},"obj":"Sentence"},{"id":"T164","span":{"begin":5055,"end":5125},"obj":"Sentence"},{"id":"T165","span":{"begin":5126,"end":5311},"obj":"Sentence"},{"id":"T166","span":{"begin":5312,"end":5547},"obj":"Sentence"},{"id":"T167","span":{"begin":5548,"end":5713},"obj":"Sentence"},{"id":"T168","span":{"begin":5714,"end":5889},"obj":"Sentence"},{"id":"T169","span":{"begin":5890,"end":6094},"obj":"Sentence"},{"id":"T170","span":{"begin":6095,"end":6254},"obj":"Sentence"},{"id":"T171","span":{"begin":6255,"end":6482},"obj":"Sentence"},{"id":"T172","span":{"begin":6483,"end":6693},"obj":"Sentence"},{"id":"T173","span":{"begin":6694,"end":6889},"obj":"Sentence"},{"id":"T174","span":{"begin":6890,"end":7089},"obj":"Sentence"}],"namespaces":[{"prefix":"_base","uri":"http://pubannotation.org/ontology/tao.owl#"}],"text":"3. Results\n\n3.1. Model Sensitivity Analysis\nFigure 2 depicts a tornado plot that communicates the sensitivity of the model to permutations in parameters. Across features, the model is most sensitive to parameters that are considered virulence-associated (Table 3) and is relatively less sensitive to survival-associated parameters (Table 4). Similar to other features, R0 (Figure 2D) of the model is most sensitive to the parameters ⍵, βA, and ν. The sensitivity of R0 to changes in ⍵ reflects the importance of the rate of conversion to the symptomatic state on model dynamics. In addition, βA has a very important influence on the model, consistent with other findings for COVID-19 that have emphasized the importance of asymptomatic transmission in disease spread [25,26,27].\n\n3.2. Illustrative Dynamics of Model System\nBased on the parameter values in Table 2, Figure 3A demonstrates the base dynamics of the model playing out over the first 100 days while Figure 3B shows the dynamics within the environment over the course of 250 days. In these dynamics, the population begins to be fixed for susceptible hosts. The disease dynamics manifest in the shapes of the curves corresponding to exposed, asymptomatic, and symptomatic individuals. Note the long tail of the curve corresponding to contamination by the environment. The environment remains infectious even after the infected populations have declined in number. The length and shape of this tail are influenced by the free-living survival of the virus.\n\n3.3. The Epidemic Consequences of Varying Virulence and Survival\nIn the next analysis, we examine the epidemic consequences of varying traits associated with survival and virulence. One can consider this as a scenario where we compare the endpoints of evolution of different virus populations (corresponding to combinations of values of survival and virulence) and calculating how these evolved populations manifest in epidemic terms.\nIn Figure 4, we observe how dynamics of the outbreak are influenced across a space of combinations of traits altering virulence (see Table 3 for a list of virulence-associated parameters) and survival (see Table 4 for a list of survival-associated parameters), changed by ±5% (10% overall). In Figure 4D, we demonstrate how changes in virulence and free-living survival traits influence R0, with variation in virulence-related traits having the largest effect on R0. Of note is how the range in R0 values varies widely across virulence–survival values, from nearly 2.0 to 3.7 (Figure 4D).\n\n3.4. Implications of Virulence–Survival Relationships at Their Relative Extremes\nHaving observed how outbreak dynamics are influenced by variation in traits that alter virulence–survival phenotypes, we then examined how each outbreak metric is influenced by the extreme (±5%) values of the trait combinations considered. Specifically, we assess how a change in pathogen survival affects outbreak dynamics, based on two expected relationships between survival and virulence traits.\n\n3.5. Positive Correlation Between Survival and Virulence\nIn a positive correlation scenario, high values for survival would be associated with high values for virulence [4,13]. Because the correlations we observe are often not exactly linear, we utilize quadrants to express a trend, allowing for some variance around the expected “line”. In Figure 5, the positive correlation scenario can be represented by combinations of virulence and survival residing in quadrants I and III.\nIf host–pathogen evolution proceeds according to a positive correlation scenario, all outbreak metrics would show an increase in severity as both survival and virulence increase. Across the range of variation in virulence and survival traits considered (5% above and below the nominal value), the peak number of infected individuals increases by approximately 35%, the rate at which the peak is reached increases by approximately 16%, the total number of infected individuals after 30 days increases by approximately 98%, and R0 increases by approximately 94% (Figure 6 and Table 5).\n\n3.6. Negative Correlation Between Survival and Virulence\nIn a negative correlation scenario, high values for survival would be associated with low values for virulence [2,9,15] and a low peak in total infected population. Pathogens with a life history that exhibits negative virulence–survival associations would likely appear in quadrants II and IV in Figure 5.\nUnder negative correlation, outbreak severity decreases as survival increases. Across the measured range of variation in virulence–survival traits, the peak number of infected individuals decreases by approximately 23%, the rate at which the epidemic peak is reached decreases by 0.15%, the total number of infected individuals decreases by 3%, and R0 decreases by approximately 84% (Figure 6 and Table 6). Across all metrics considered, the effects of increased viral survival on outbreak dynamics is more extreme under the positive correlation than the negative correlation scenarios (Figure 6).\n\n3.7. Dynamics of Epidemics at Extreme Values for Virus Free-Living Survival\nIn Figure 7, we observe the disease dynamics at extreme values for survival and the dynamics corresponding to the fraction of the environment that is contaminated with infectious virus. Consistent with the data represented in Figure 6, we observe that minimum and maximum simulations differ more substantially for extreme survival scenarios in the positive correlation scenario than for the negative correlation scenario.\nThe feature of different outbreaks that varies most ostensibly between the correlation scenarios is the time needed to reach the peak number of infected individuals. In positive correlation simulations, one can observe that the low virulence, low survival scenario (Figure 7A,B) takes longer to reach the peak number of infected individuals. Most notably, however, the low virulence, low survival setting has a far smaller peak of environmental contamination and shorter tail relative to its high virulence, high survival counterpart (Figure 7D). Similarly, intriguing findings exist in the comparison between the simulation sets corresponding to extremes in the negative correlation setting (Figure 7E–H). Especially notable is the difference in the length of the tail of the environmental contamination for the high virulence, low survival combination (Figure 7F) vs. the low virulence, low survival combination variant (Figure 7H). The explanation is that, in this model, higher virulence influences (among many other things) the rate at which the virus is shed into the environment from either the asymptomatic (𝜎A) or symptomatic (𝜎I) host. We observe how the high virulence, low survival simulation (Figure 7E) features a symptomatic peak that is larger in size and is prolonged relative to the lower virulence counterpart (Figure 7G). This relatively large symptomatic population sheds infectious virus into the environment for a longer period of time, contributing to the long tail of contaminated environments observed in Figure 5F."}