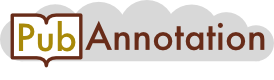
PMC:7547912 / 3978-10972
Annnotations
LitCovid-PD-MONDO
{"project":"LitCovid-PD-MONDO","denotations":[{"id":"T10","span":{"begin":1163,"end":1171},"obj":"Disease"}],"attributes":[{"id":"A10","pred":"mondo_id","subj":"T10","obj":"http://purl.obolibrary.org/obo/MONDO_0100096"}],"text":"Origin of data set\nAir quality index (AQI) reports daily air quality and its elevated level is associated with public health risks (Szyszkowicz 2019). Based on different national quality standards and dose-response relationships of pollutants, countries have different air quality indices (Zhang et al. 2020; Sofia et al. 2020). The Indian national air quality index considers eight pollutants (PM10, PM2.5, NO2, SO2, NH3, CO, O3, and Pb) with a 24-hourly averaging period. It is subdivided into six categories i.e., good (0–50), satisfactory (51–100), moderately polluted (101–200), poor (201–300), very poor (301–400), and severe (401–500) as shown in Fig. 1 (Perera 2018; Ghorani-Azam et al. 2016). The sub-indices for individual pollutants at a monitoring location are calculated using its 24-hourly average concentration value (8-hourly in case of CO and O3) and health breakpoint concentration range. The worst sub-index is the AQI for that location (https://app.cpcbccr.com/AQI_India/). An increment in AQI causes acute and chronic mode health concern especially in the older age people and in children (Januszek et al. 2020; Pant et al. 2020). Due to the COVID-19 pandemic confinement, there is a significant reduction in the level of such toxic pollutants globally (Selvam et al. 2020; Singh and Chauhan 2020).\nFig. 1 Indian national air quality index—category and range\nIn the present study, concentrations of different pollutants i.e., PM2.5 (diameter \u003c 2.5 μm), PM10 (diameter \u003c 10 μm), NO2, NH3, SO2, CO, ozone, and air quality index (AQI) were acquired from open access internet sources provided by the Central Pollution Control Board (CPCB), Ministry of Environment, Forests, and Climate Change (https://app.cpcbccr.com/AQI_India/). The data were recorded daily from January 1, 2020 to May 31, 2020, which is subdivided into two groups: (a) pre-lockdown period—January 1, 2020 to March 23, 2020, and (b) lockdown period—March 24, 2020 to May 31, 2020 at 17:00 IST among four different air quality monitoring stations of the CPCB for four major metropolitan cities in India i.e., site 1—ITO, Delhi, site 2—Worli, Mumbai, site 3—Jadavpur, Kolkata, and site 4—Manali Village, Chennai as shown in Fig. 2. For air quality assessment, % variations of air pollutants during the confinement period were compared with pre-lockdown values.\nFig. 2 The geography of monitoring stations among the populous sites of four major metropolitan cities in India\nThe air quality index is a piecewise linear function of the pollutant concentration. At the boundary between AQI categories, there is a discontinuous jump of one AQI unit. To convert from concentration to AQI, this equation is used:\\documentclass[12pt]{minimal} \t\t\t\t\\usepackage{amsmath} \t\t\t\t\\usepackage{wasysym} \t\t\t\t\\usepackage{amsfonts} \t\t\t\t\\usepackage{amssymb} \t\t\t\t\\usepackage{amsbsy} \t\t\t\t\\usepackage{mathrsfs} \t\t\t\t\\usepackage{upgreek} \t\t\t\t\\setlength{\\oddsidemargin}{-69pt} \t\t\t\t\\begin{document}$$I=\\frac{I_{\\mathrm{high}}-{I}_{\\mathrm{low}}}{C_{\\mathrm{high}}-{C}_{\\mathrm{low}}}\\ \\left(C-{C}_{\\mathrm{low}}\\right)+{I}_{\\mathrm{low}}$$\\end{document}I=Ihigh−IlowChigh−ClowC−Clow+Ilow\nIf multiple pollutants are measured, the calculated AQI is the highest value calculated from the above equation applied for each pollutant.whereIThe (air quality) index,\nCThe pollutant concentration,\nClowThe concentration breakpoint that is ≤C,\nChighThe concentration breakpoint that is ≥C,\nIlowThe index breakpoint corresponding to Clow,\nIhighThe index breakpoint corresponding to Chigh.\nMoreover, we have used unpaired Welch’s two-sample t test analysis to measure the statistically significant reduction in average AQI for all four sites, as t test allows us to compare the average values of the two data sets and determine if they came from the same population. The formula for calculating t-statistics is given as:\\documentclass[12pt]{minimal} \t\t\t\t\\usepackage{amsmath} \t\t\t\t\\usepackage{wasysym} \t\t\t\t\\usepackage{amsfonts} \t\t\t\t\\usepackage{amssymb} \t\t\t\t\\usepackage{amsbsy} \t\t\t\t\\usepackage{mathrsfs} \t\t\t\t\\usepackage{upgreek} \t\t\t\t\\setlength{\\oddsidemargin}{-69pt} \t\t\t\t\\begin{document}$$t=\\frac{{\\overline{x}}_1-{\\overline{x}}_2}{\\sqrt{\\frac{s_1^2}{n_1}-\\frac{s_2^2}{n_2}}}$$\\end{document}t=x¯1−x¯2s12n1−s22n2where, \\documentclass[12pt]{minimal} \t\t\t\t\\usepackage{amsmath} \t\t\t\t\\usepackage{wasysym} \t\t\t\t\\usepackage{amsfonts} \t\t\t\t\\usepackage{amssymb} \t\t\t\t\\usepackage{amsbsy} \t\t\t\t\\usepackage{mathrsfs} \t\t\t\t\\usepackage{upgreek} \t\t\t\t\\setlength{\\oddsidemargin}{-69pt} \t\t\t\t\\begin{document}$${\\overline{x}}_1$$\\end{document}x¯1 and \\documentclass[12pt]{minimal} \t\t\t\t\\usepackage{amsmath} \t\t\t\t\\usepackage{wasysym} \t\t\t\t\\usepackage{amsfonts} \t\t\t\t\\usepackage{amssymb} \t\t\t\t\\usepackage{amsbsy} \t\t\t\t\\usepackage{mathrsfs} \t\t\t\t\\usepackage{upgreek} \t\t\t\t\\setlength{\\oddsidemargin}{-69pt} \t\t\t\t\\begin{document}$${\\overline{x}}_2$$\\end{document}x¯2 are the sample means, n1 and n2 are the sample sizes, and \\documentclass[12pt]{minimal} \t\t\t\t\\usepackage{amsmath} \t\t\t\t\\usepackage{wasysym} \t\t\t\t\\usepackage{amsfonts} \t\t\t\t\\usepackage{amssymb} \t\t\t\t\\usepackage{amsbsy} \t\t\t\t\\usepackage{mathrsfs} \t\t\t\t\\usepackage{upgreek} \t\t\t\t\\setlength{\\oddsidemargin}{-69pt} \t\t\t\t\\begin{document}$${s}_1^2$$\\end{document}s12 and \\documentclass[12pt]{minimal} \t\t\t\t\\usepackage{amsmath} \t\t\t\t\\usepackage{wasysym} \t\t\t\t\\usepackage{amsfonts} \t\t\t\t\\usepackage{amssymb} \t\t\t\t\\usepackage{amsbsy} \t\t\t\t\\usepackage{mathrsfs} \t\t\t\t\\usepackage{upgreek} \t\t\t\t\\setlength{\\oddsidemargin}{-69pt} \t\t\t\t\\begin{document}$${s}_2^2$$\\end{document}s22 are the sample variances for samples 1 and 2 respectively.\nTo find out the most prominent pollutant concerning AQI statistically, we have done Pearson’s correlation analysis by the means of plotting heatmaps corresponding to each site. Pearson’s correlation is also known as the “product-moment correlation coefficient” (PMCC) and is suitable for measuring the extent of the linear relationship between any two quantitative variables statistically. A Pearson’s correlation is a number ranging between − 1 and + 1 showing negative to positive linear correlation. Given a pair of random variables (X1, X2), the formula for Pearson’s correlation is given by\\documentclass[12pt]{minimal} \t\t\t\t\\usepackage{amsmath} \t\t\t\t\\usepackage{wasysym} \t\t\t\t\\usepackage{amsfonts} \t\t\t\t\\usepackage{amssymb} \t\t\t\t\\usepackage{amsbsy} \t\t\t\t\\usepackage{mathrsfs} \t\t\t\t\\usepackage{upgreek} \t\t\t\t\\setlength{\\oddsidemargin}{-69pt} \t\t\t\t\\begin{document}$${\\rho}_{X_1,{X}_2}=\\frac{Cov\\left({X}_1,{X}_2\\right)}{\\sigma_{X_1}\\ {\\sigma}_{X_2}}$$\\end{document}ρX1,X2=CovX1X2σX1σX2where Cov(X1, X2) is the covariance between the variables under study and \\documentclass[12pt]{minimal} \t\t\t\t\\usepackage{amsmath} \t\t\t\t\\usepackage{wasysym} \t\t\t\t\\usepackage{amsfonts} \t\t\t\t\\usepackage{amssymb} \t\t\t\t\\usepackage{amsbsy} \t\t\t\t\\usepackage{mathrsfs} \t\t\t\t\\usepackage{upgreek} \t\t\t\t\\setlength{\\oddsidemargin}{-69pt} \t\t\t\t\\begin{document}$${\\sigma}_{X_1},{\\sigma}_{X_2}$$\\end{document}σX1,σX2 are the standard deviation of X1, X2 respectively."}
LitCovid-PD-CLO
{"project":"LitCovid-PD-CLO","denotations":[{"id":"T19","span":{"begin":444,"end":448},"obj":"http://purl.obolibrary.org/obo/CLO_0001568"},{"id":"T20","span":{"begin":669,"end":673},"obj":"http://purl.obolibrary.org/obo/CLO_0001185"},{"id":"T21","span":{"begin":747,"end":748},"obj":"http://purl.obolibrary.org/obo/CLO_0001020"},{"id":"T22","span":{"begin":1203,"end":1204},"obj":"http://purl.obolibrary.org/obo/CLO_0001020"},{"id":"T23","span":{"begin":1853,"end":1854},"obj":"http://purl.obolibrary.org/obo/CLO_0001020"},{"id":"T24","span":{"begin":1916,"end":1917},"obj":"http://purl.obolibrary.org/obo/CLO_0001021"},{"id":"T25","span":{"begin":2482,"end":2483},"obj":"http://purl.obolibrary.org/obo/CLO_0001020"},{"id":"T26","span":{"begin":2591,"end":2592},"obj":"http://purl.obolibrary.org/obo/CLO_0001020"},{"id":"T27","span":{"begin":3584,"end":3588},"obj":"http://purl.obolibrary.org/obo/UBERON_0000473"},{"id":"T28","span":{"begin":3689,"end":3693},"obj":"http://purl.obolibrary.org/obo/UBERON_0000473"},{"id":"T29","span":{"begin":5961,"end":5962},"obj":"http://purl.obolibrary.org/obo/CLO_0001020"},{"id":"T30","span":{"begin":5988,"end":5989},"obj":"http://purl.obolibrary.org/obo/CLO_0001020"},{"id":"T31","span":{"begin":6080,"end":6081},"obj":"http://purl.obolibrary.org/obo/CLO_0001020"},{"id":"T32","span":{"begin":6434,"end":6437},"obj":"http://purl.obolibrary.org/obo/CLO_0051142"}],"text":"Origin of data set\nAir quality index (AQI) reports daily air quality and its elevated level is associated with public health risks (Szyszkowicz 2019). Based on different national quality standards and dose-response relationships of pollutants, countries have different air quality indices (Zhang et al. 2020; Sofia et al. 2020). The Indian national air quality index considers eight pollutants (PM10, PM2.5, NO2, SO2, NH3, CO, O3, and Pb) with a 24-hourly averaging period. It is subdivided into six categories i.e., good (0–50), satisfactory (51–100), moderately polluted (101–200), poor (201–300), very poor (301–400), and severe (401–500) as shown in Fig. 1 (Perera 2018; Ghorani-Azam et al. 2016). The sub-indices for individual pollutants at a monitoring location are calculated using its 24-hourly average concentration value (8-hourly in case of CO and O3) and health breakpoint concentration range. The worst sub-index is the AQI for that location (https://app.cpcbccr.com/AQI_India/). An increment in AQI causes acute and chronic mode health concern especially in the older age people and in children (Januszek et al. 2020; Pant et al. 2020). Due to the COVID-19 pandemic confinement, there is a significant reduction in the level of such toxic pollutants globally (Selvam et al. 2020; Singh and Chauhan 2020).\nFig. 1 Indian national air quality index—category and range\nIn the present study, concentrations of different pollutants i.e., PM2.5 (diameter \u003c 2.5 μm), PM10 (diameter \u003c 10 μm), NO2, NH3, SO2, CO, ozone, and air quality index (AQI) were acquired from open access internet sources provided by the Central Pollution Control Board (CPCB), Ministry of Environment, Forests, and Climate Change (https://app.cpcbccr.com/AQI_India/). The data were recorded daily from January 1, 2020 to May 31, 2020, which is subdivided into two groups: (a) pre-lockdown period—January 1, 2020 to March 23, 2020, and (b) lockdown period—March 24, 2020 to May 31, 2020 at 17:00 IST among four different air quality monitoring stations of the CPCB for four major metropolitan cities in India i.e., site 1—ITO, Delhi, site 2—Worli, Mumbai, site 3—Jadavpur, Kolkata, and site 4—Manali Village, Chennai as shown in Fig. 2. For air quality assessment, % variations of air pollutants during the confinement period were compared with pre-lockdown values.\nFig. 2 The geography of monitoring stations among the populous sites of four major metropolitan cities in India\nThe air quality index is a piecewise linear function of the pollutant concentration. At the boundary between AQI categories, there is a discontinuous jump of one AQI unit. To convert from concentration to AQI, this equation is used:\\documentclass[12pt]{minimal} \t\t\t\t\\usepackage{amsmath} \t\t\t\t\\usepackage{wasysym} \t\t\t\t\\usepackage{amsfonts} \t\t\t\t\\usepackage{amssymb} \t\t\t\t\\usepackage{amsbsy} \t\t\t\t\\usepackage{mathrsfs} \t\t\t\t\\usepackage{upgreek} \t\t\t\t\\setlength{\\oddsidemargin}{-69pt} \t\t\t\t\\begin{document}$$I=\\frac{I_{\\mathrm{high}}-{I}_{\\mathrm{low}}}{C_{\\mathrm{high}}-{C}_{\\mathrm{low}}}\\ \\left(C-{C}_{\\mathrm{low}}\\right)+{I}_{\\mathrm{low}}$$\\end{document}I=Ihigh−IlowChigh−ClowC−Clow+Ilow\nIf multiple pollutants are measured, the calculated AQI is the highest value calculated from the above equation applied for each pollutant.whereIThe (air quality) index,\nCThe pollutant concentration,\nClowThe concentration breakpoint that is ≤C,\nChighThe concentration breakpoint that is ≥C,\nIlowThe index breakpoint corresponding to Clow,\nIhighThe index breakpoint corresponding to Chigh.\nMoreover, we have used unpaired Welch’s two-sample t test analysis to measure the statistically significant reduction in average AQI for all four sites, as t test allows us to compare the average values of the two data sets and determine if they came from the same population. The formula for calculating t-statistics is given as:\\documentclass[12pt]{minimal} \t\t\t\t\\usepackage{amsmath} \t\t\t\t\\usepackage{wasysym} \t\t\t\t\\usepackage{amsfonts} \t\t\t\t\\usepackage{amssymb} \t\t\t\t\\usepackage{amsbsy} \t\t\t\t\\usepackage{mathrsfs} \t\t\t\t\\usepackage{upgreek} \t\t\t\t\\setlength{\\oddsidemargin}{-69pt} \t\t\t\t\\begin{document}$$t=\\frac{{\\overline{x}}_1-{\\overline{x}}_2}{\\sqrt{\\frac{s_1^2}{n_1}-\\frac{s_2^2}{n_2}}}$$\\end{document}t=x¯1−x¯2s12n1−s22n2where, \\documentclass[12pt]{minimal} \t\t\t\t\\usepackage{amsmath} \t\t\t\t\\usepackage{wasysym} \t\t\t\t\\usepackage{amsfonts} \t\t\t\t\\usepackage{amssymb} \t\t\t\t\\usepackage{amsbsy} \t\t\t\t\\usepackage{mathrsfs} \t\t\t\t\\usepackage{upgreek} \t\t\t\t\\setlength{\\oddsidemargin}{-69pt} \t\t\t\t\\begin{document}$${\\overline{x}}_1$$\\end{document}x¯1 and \\documentclass[12pt]{minimal} \t\t\t\t\\usepackage{amsmath} \t\t\t\t\\usepackage{wasysym} \t\t\t\t\\usepackage{amsfonts} \t\t\t\t\\usepackage{amssymb} \t\t\t\t\\usepackage{amsbsy} \t\t\t\t\\usepackage{mathrsfs} \t\t\t\t\\usepackage{upgreek} \t\t\t\t\\setlength{\\oddsidemargin}{-69pt} \t\t\t\t\\begin{document}$${\\overline{x}}_2$$\\end{document}x¯2 are the sample means, n1 and n2 are the sample sizes, and \\documentclass[12pt]{minimal} \t\t\t\t\\usepackage{amsmath} \t\t\t\t\\usepackage{wasysym} \t\t\t\t\\usepackage{amsfonts} \t\t\t\t\\usepackage{amssymb} \t\t\t\t\\usepackage{amsbsy} \t\t\t\t\\usepackage{mathrsfs} \t\t\t\t\\usepackage{upgreek} \t\t\t\t\\setlength{\\oddsidemargin}{-69pt} \t\t\t\t\\begin{document}$${s}_1^2$$\\end{document}s12 and \\documentclass[12pt]{minimal} \t\t\t\t\\usepackage{amsmath} \t\t\t\t\\usepackage{wasysym} \t\t\t\t\\usepackage{amsfonts} \t\t\t\t\\usepackage{amssymb} \t\t\t\t\\usepackage{amsbsy} \t\t\t\t\\usepackage{mathrsfs} \t\t\t\t\\usepackage{upgreek} \t\t\t\t\\setlength{\\oddsidemargin}{-69pt} \t\t\t\t\\begin{document}$${s}_2^2$$\\end{document}s22 are the sample variances for samples 1 and 2 respectively.\nTo find out the most prominent pollutant concerning AQI statistically, we have done Pearson’s correlation analysis by the means of plotting heatmaps corresponding to each site. Pearson’s correlation is also known as the “product-moment correlation coefficient” (PMCC) and is suitable for measuring the extent of the linear relationship between any two quantitative variables statistically. A Pearson’s correlation is a number ranging between − 1 and + 1 showing negative to positive linear correlation. Given a pair of random variables (X1, X2), the formula for Pearson’s correlation is given by\\documentclass[12pt]{minimal} \t\t\t\t\\usepackage{amsmath} \t\t\t\t\\usepackage{wasysym} \t\t\t\t\\usepackage{amsfonts} \t\t\t\t\\usepackage{amssymb} \t\t\t\t\\usepackage{amsbsy} \t\t\t\t\\usepackage{mathrsfs} \t\t\t\t\\usepackage{upgreek} \t\t\t\t\\setlength{\\oddsidemargin}{-69pt} \t\t\t\t\\begin{document}$${\\rho}_{X_1,{X}_2}=\\frac{Cov\\left({X}_1,{X}_2\\right)}{\\sigma_{X_1}\\ {\\sigma}_{X_2}}$$\\end{document}ρX1,X2=CovX1X2σX1σX2where Cov(X1, X2) is the covariance between the variables under study and \\documentclass[12pt]{minimal} \t\t\t\t\\usepackage{amsmath} \t\t\t\t\\usepackage{wasysym} \t\t\t\t\\usepackage{amsfonts} \t\t\t\t\\usepackage{amssymb} \t\t\t\t\\usepackage{amsbsy} \t\t\t\t\\usepackage{mathrsfs} \t\t\t\t\\usepackage{upgreek} \t\t\t\t\\setlength{\\oddsidemargin}{-69pt} \t\t\t\t\\begin{document}$${\\sigma}_{X_1},{\\sigma}_{X_2}$$\\end{document}σX1,σX2 are the standard deviation of X1, X2 respectively."}
LitCovid-PD-CHEBI
{"project":"LitCovid-PD-CHEBI","denotations":[{"id":"T27","span":{"begin":408,"end":411},"obj":"Chemical"},{"id":"T29","span":{"begin":413,"end":416},"obj":"Chemical"},{"id":"T30","span":{"begin":418,"end":421},"obj":"Chemical"},{"id":"T31","span":{"begin":423,"end":425},"obj":"Chemical"},{"id":"T32","span":{"begin":427,"end":429},"obj":"Chemical"},{"id":"T33","span":{"begin":435,"end":437},"obj":"Chemical"},{"id":"T36","span":{"begin":853,"end":855},"obj":"Chemical"},{"id":"T37","span":{"begin":860,"end":862},"obj":"Chemical"},{"id":"T38","span":{"begin":1499,"end":1502},"obj":"Chemical"},{"id":"T40","span":{"begin":1504,"end":1507},"obj":"Chemical"},{"id":"T41","span":{"begin":1509,"end":1512},"obj":"Chemical"},{"id":"T42","span":{"begin":1514,"end":1516},"obj":"Chemical"},{"id":"T43","span":{"begin":1518,"end":1523},"obj":"Chemical"}],"attributes":[{"id":"A27","pred":"chebi_id","subj":"T27","obj":"http://purl.obolibrary.org/obo/CHEBI_16301"},{"id":"A28","pred":"chebi_id","subj":"T27","obj":"http://purl.obolibrary.org/obo/CHEBI_33101"},{"id":"A29","pred":"chebi_id","subj":"T29","obj":"http://purl.obolibrary.org/obo/CHEBI_18422"},{"id":"A30","pred":"chebi_id","subj":"T30","obj":"http://purl.obolibrary.org/obo/CHEBI_16134"},{"id":"A31","pred":"chebi_id","subj":"T31","obj":"http://purl.obolibrary.org/obo/CHEBI_17245"},{"id":"A32","pred":"chebi_id","subj":"T32","obj":"http://purl.obolibrary.org/obo/CHEBI_25812"},{"id":"A33","pred":"chebi_id","subj":"T33","obj":"http://purl.obolibrary.org/obo/CHEBI_25016"},{"id":"A34","pred":"chebi_id","subj":"T33","obj":"http://purl.obolibrary.org/obo/CHEBI_27889"},{"id":"A35","pred":"chebi_id","subj":"T33","obj":"http://purl.obolibrary.org/obo/CHEBI_49807"},{"id":"A36","pred":"chebi_id","subj":"T36","obj":"http://purl.obolibrary.org/obo/CHEBI_17245"},{"id":"A37","pred":"chebi_id","subj":"T37","obj":"http://purl.obolibrary.org/obo/CHEBI_25812"},{"id":"A38","pred":"chebi_id","subj":"T38","obj":"http://purl.obolibrary.org/obo/CHEBI_16301"},{"id":"A39","pred":"chebi_id","subj":"T38","obj":"http://purl.obolibrary.org/obo/CHEBI_33101"},{"id":"A40","pred":"chebi_id","subj":"T40","obj":"http://purl.obolibrary.org/obo/CHEBI_16134"},{"id":"A41","pred":"chebi_id","subj":"T41","obj":"http://purl.obolibrary.org/obo/CHEBI_18422"},{"id":"A42","pred":"chebi_id","subj":"T42","obj":"http://purl.obolibrary.org/obo/CHEBI_17245"},{"id":"A43","pred":"chebi_id","subj":"T43","obj":"http://purl.obolibrary.org/obo/CHEBI_25812"}],"text":"Origin of data set\nAir quality index (AQI) reports daily air quality and its elevated level is associated with public health risks (Szyszkowicz 2019). Based on different national quality standards and dose-response relationships of pollutants, countries have different air quality indices (Zhang et al. 2020; Sofia et al. 2020). The Indian national air quality index considers eight pollutants (PM10, PM2.5, NO2, SO2, NH3, CO, O3, and Pb) with a 24-hourly averaging period. It is subdivided into six categories i.e., good (0–50), satisfactory (51–100), moderately polluted (101–200), poor (201–300), very poor (301–400), and severe (401–500) as shown in Fig. 1 (Perera 2018; Ghorani-Azam et al. 2016). The sub-indices for individual pollutants at a monitoring location are calculated using its 24-hourly average concentration value (8-hourly in case of CO and O3) and health breakpoint concentration range. The worst sub-index is the AQI for that location (https://app.cpcbccr.com/AQI_India/). An increment in AQI causes acute and chronic mode health concern especially in the older age people and in children (Januszek et al. 2020; Pant et al. 2020). Due to the COVID-19 pandemic confinement, there is a significant reduction in the level of such toxic pollutants globally (Selvam et al. 2020; Singh and Chauhan 2020).\nFig. 1 Indian national air quality index—category and range\nIn the present study, concentrations of different pollutants i.e., PM2.5 (diameter \u003c 2.5 μm), PM10 (diameter \u003c 10 μm), NO2, NH3, SO2, CO, ozone, and air quality index (AQI) were acquired from open access internet sources provided by the Central Pollution Control Board (CPCB), Ministry of Environment, Forests, and Climate Change (https://app.cpcbccr.com/AQI_India/). The data were recorded daily from January 1, 2020 to May 31, 2020, which is subdivided into two groups: (a) pre-lockdown period—January 1, 2020 to March 23, 2020, and (b) lockdown period—March 24, 2020 to May 31, 2020 at 17:00 IST among four different air quality monitoring stations of the CPCB for four major metropolitan cities in India i.e., site 1—ITO, Delhi, site 2—Worli, Mumbai, site 3—Jadavpur, Kolkata, and site 4—Manali Village, Chennai as shown in Fig. 2. For air quality assessment, % variations of air pollutants during the confinement period were compared with pre-lockdown values.\nFig. 2 The geography of monitoring stations among the populous sites of four major metropolitan cities in India\nThe air quality index is a piecewise linear function of the pollutant concentration. At the boundary between AQI categories, there is a discontinuous jump of one AQI unit. To convert from concentration to AQI, this equation is used:\\documentclass[12pt]{minimal} \t\t\t\t\\usepackage{amsmath} \t\t\t\t\\usepackage{wasysym} \t\t\t\t\\usepackage{amsfonts} \t\t\t\t\\usepackage{amssymb} \t\t\t\t\\usepackage{amsbsy} \t\t\t\t\\usepackage{mathrsfs} \t\t\t\t\\usepackage{upgreek} \t\t\t\t\\setlength{\\oddsidemargin}{-69pt} \t\t\t\t\\begin{document}$$I=\\frac{I_{\\mathrm{high}}-{I}_{\\mathrm{low}}}{C_{\\mathrm{high}}-{C}_{\\mathrm{low}}}\\ \\left(C-{C}_{\\mathrm{low}}\\right)+{I}_{\\mathrm{low}}$$\\end{document}I=Ihigh−IlowChigh−ClowC−Clow+Ilow\nIf multiple pollutants are measured, the calculated AQI is the highest value calculated from the above equation applied for each pollutant.whereIThe (air quality) index,\nCThe pollutant concentration,\nClowThe concentration breakpoint that is ≤C,\nChighThe concentration breakpoint that is ≥C,\nIlowThe index breakpoint corresponding to Clow,\nIhighThe index breakpoint corresponding to Chigh.\nMoreover, we have used unpaired Welch’s two-sample t test analysis to measure the statistically significant reduction in average AQI for all four sites, as t test allows us to compare the average values of the two data sets and determine if they came from the same population. The formula for calculating t-statistics is given as:\\documentclass[12pt]{minimal} \t\t\t\t\\usepackage{amsmath} \t\t\t\t\\usepackage{wasysym} \t\t\t\t\\usepackage{amsfonts} \t\t\t\t\\usepackage{amssymb} \t\t\t\t\\usepackage{amsbsy} \t\t\t\t\\usepackage{mathrsfs} \t\t\t\t\\usepackage{upgreek} \t\t\t\t\\setlength{\\oddsidemargin}{-69pt} \t\t\t\t\\begin{document}$$t=\\frac{{\\overline{x}}_1-{\\overline{x}}_2}{\\sqrt{\\frac{s_1^2}{n_1}-\\frac{s_2^2}{n_2}}}$$\\end{document}t=x¯1−x¯2s12n1−s22n2where, \\documentclass[12pt]{minimal} \t\t\t\t\\usepackage{amsmath} \t\t\t\t\\usepackage{wasysym} \t\t\t\t\\usepackage{amsfonts} \t\t\t\t\\usepackage{amssymb} \t\t\t\t\\usepackage{amsbsy} \t\t\t\t\\usepackage{mathrsfs} \t\t\t\t\\usepackage{upgreek} \t\t\t\t\\setlength{\\oddsidemargin}{-69pt} \t\t\t\t\\begin{document}$${\\overline{x}}_1$$\\end{document}x¯1 and \\documentclass[12pt]{minimal} \t\t\t\t\\usepackage{amsmath} \t\t\t\t\\usepackage{wasysym} \t\t\t\t\\usepackage{amsfonts} \t\t\t\t\\usepackage{amssymb} \t\t\t\t\\usepackage{amsbsy} \t\t\t\t\\usepackage{mathrsfs} \t\t\t\t\\usepackage{upgreek} \t\t\t\t\\setlength{\\oddsidemargin}{-69pt} \t\t\t\t\\begin{document}$${\\overline{x}}_2$$\\end{document}x¯2 are the sample means, n1 and n2 are the sample sizes, and \\documentclass[12pt]{minimal} \t\t\t\t\\usepackage{amsmath} \t\t\t\t\\usepackage{wasysym} \t\t\t\t\\usepackage{amsfonts} \t\t\t\t\\usepackage{amssymb} \t\t\t\t\\usepackage{amsbsy} \t\t\t\t\\usepackage{mathrsfs} \t\t\t\t\\usepackage{upgreek} \t\t\t\t\\setlength{\\oddsidemargin}{-69pt} \t\t\t\t\\begin{document}$${s}_1^2$$\\end{document}s12 and \\documentclass[12pt]{minimal} \t\t\t\t\\usepackage{amsmath} \t\t\t\t\\usepackage{wasysym} \t\t\t\t\\usepackage{amsfonts} \t\t\t\t\\usepackage{amssymb} \t\t\t\t\\usepackage{amsbsy} \t\t\t\t\\usepackage{mathrsfs} \t\t\t\t\\usepackage{upgreek} \t\t\t\t\\setlength{\\oddsidemargin}{-69pt} \t\t\t\t\\begin{document}$${s}_2^2$$\\end{document}s22 are the sample variances for samples 1 and 2 respectively.\nTo find out the most prominent pollutant concerning AQI statistically, we have done Pearson’s correlation analysis by the means of plotting heatmaps corresponding to each site. Pearson’s correlation is also known as the “product-moment correlation coefficient” (PMCC) and is suitable for measuring the extent of the linear relationship between any two quantitative variables statistically. A Pearson’s correlation is a number ranging between − 1 and + 1 showing negative to positive linear correlation. Given a pair of random variables (X1, X2), the formula for Pearson’s correlation is given by\\documentclass[12pt]{minimal} \t\t\t\t\\usepackage{amsmath} \t\t\t\t\\usepackage{wasysym} \t\t\t\t\\usepackage{amsfonts} \t\t\t\t\\usepackage{amssymb} \t\t\t\t\\usepackage{amsbsy} \t\t\t\t\\usepackage{mathrsfs} \t\t\t\t\\usepackage{upgreek} \t\t\t\t\\setlength{\\oddsidemargin}{-69pt} \t\t\t\t\\begin{document}$${\\rho}_{X_1,{X}_2}=\\frac{Cov\\left({X}_1,{X}_2\\right)}{\\sigma_{X_1}\\ {\\sigma}_{X_2}}$$\\end{document}ρX1,X2=CovX1X2σX1σX2where Cov(X1, X2) is the covariance between the variables under study and \\documentclass[12pt]{minimal} \t\t\t\t\\usepackage{amsmath} \t\t\t\t\\usepackage{wasysym} \t\t\t\t\\usepackage{amsfonts} \t\t\t\t\\usepackage{amssymb} \t\t\t\t\\usepackage{amsbsy} \t\t\t\t\\usepackage{mathrsfs} \t\t\t\t\\usepackage{upgreek} \t\t\t\t\\setlength{\\oddsidemargin}{-69pt} \t\t\t\t\\begin{document}$${\\sigma}_{X_1},{\\sigma}_{X_2}$$\\end{document}σX1,σX2 are the standard deviation of X1, X2 respectively."}
LitCovid-PubTator
{"project":"LitCovid-PubTator","denotations":[{"id":"42","span":{"begin":1087,"end":1093},"obj":"Species"},{"id":"43","span":{"begin":1101,"end":1109},"obj":"Species"},{"id":"44","span":{"begin":423,"end":425},"obj":"Chemical"},{"id":"45","span":{"begin":427,"end":429},"obj":"Chemical"},{"id":"46","span":{"begin":435,"end":437},"obj":"Chemical"},{"id":"47","span":{"begin":853,"end":855},"obj":"Chemical"},{"id":"48","span":{"begin":860,"end":862},"obj":"Chemical"},{"id":"49","span":{"begin":1163,"end":1171},"obj":"Disease"},{"id":"51","span":{"begin":6557,"end":6560},"obj":"Species"}],"attributes":[{"id":"A42","pred":"tao:has_database_id","subj":"42","obj":"Tax:9606"},{"id":"A43","pred":"tao:has_database_id","subj":"43","obj":"Tax:9606"},{"id":"A44","pred":"tao:has_database_id","subj":"44","obj":"MESH:D002248"},{"id":"A45","pred":"tao:has_database_id","subj":"45","obj":"MESH:D010126"},{"id":"A46","pred":"tao:has_database_id","subj":"46","obj":"MESH:D007854"},{"id":"A47","pred":"tao:has_database_id","subj":"47","obj":"MESH:D002248"},{"id":"A48","pred":"tao:has_database_id","subj":"48","obj":"MESH:D010126"},{"id":"A49","pred":"tao:has_database_id","subj":"49","obj":"MESH:C000657245"},{"id":"A51","pred":"tao:has_database_id","subj":"51","obj":"Tax:11118"}],"namespaces":[{"prefix":"Tax","uri":"https://www.ncbi.nlm.nih.gov/taxonomy/"},{"prefix":"MESH","uri":"https://id.nlm.nih.gov/mesh/"},{"prefix":"Gene","uri":"https://www.ncbi.nlm.nih.gov/gene/"},{"prefix":"CVCL","uri":"https://web.expasy.org/cellosaurus/CVCL_"}],"text":"Origin of data set\nAir quality index (AQI) reports daily air quality and its elevated level is associated with public health risks (Szyszkowicz 2019). Based on different national quality standards and dose-response relationships of pollutants, countries have different air quality indices (Zhang et al. 2020; Sofia et al. 2020). The Indian national air quality index considers eight pollutants (PM10, PM2.5, NO2, SO2, NH3, CO, O3, and Pb) with a 24-hourly averaging period. It is subdivided into six categories i.e., good (0–50), satisfactory (51–100), moderately polluted (101–200), poor (201–300), very poor (301–400), and severe (401–500) as shown in Fig. 1 (Perera 2018; Ghorani-Azam et al. 2016). The sub-indices for individual pollutants at a monitoring location are calculated using its 24-hourly average concentration value (8-hourly in case of CO and O3) and health breakpoint concentration range. The worst sub-index is the AQI for that location (https://app.cpcbccr.com/AQI_India/). An increment in AQI causes acute and chronic mode health concern especially in the older age people and in children (Januszek et al. 2020; Pant et al. 2020). Due to the COVID-19 pandemic confinement, there is a significant reduction in the level of such toxic pollutants globally (Selvam et al. 2020; Singh and Chauhan 2020).\nFig. 1 Indian national air quality index—category and range\nIn the present study, concentrations of different pollutants i.e., PM2.5 (diameter \u003c 2.5 μm), PM10 (diameter \u003c 10 μm), NO2, NH3, SO2, CO, ozone, and air quality index (AQI) were acquired from open access internet sources provided by the Central Pollution Control Board (CPCB), Ministry of Environment, Forests, and Climate Change (https://app.cpcbccr.com/AQI_India/). The data were recorded daily from January 1, 2020 to May 31, 2020, which is subdivided into two groups: (a) pre-lockdown period—January 1, 2020 to March 23, 2020, and (b) lockdown period—March 24, 2020 to May 31, 2020 at 17:00 IST among four different air quality monitoring stations of the CPCB for four major metropolitan cities in India i.e., site 1—ITO, Delhi, site 2—Worli, Mumbai, site 3—Jadavpur, Kolkata, and site 4—Manali Village, Chennai as shown in Fig. 2. For air quality assessment, % variations of air pollutants during the confinement period were compared with pre-lockdown values.\nFig. 2 The geography of monitoring stations among the populous sites of four major metropolitan cities in India\nThe air quality index is a piecewise linear function of the pollutant concentration. At the boundary between AQI categories, there is a discontinuous jump of one AQI unit. To convert from concentration to AQI, this equation is used:\\documentclass[12pt]{minimal} \t\t\t\t\\usepackage{amsmath} \t\t\t\t\\usepackage{wasysym} \t\t\t\t\\usepackage{amsfonts} \t\t\t\t\\usepackage{amssymb} \t\t\t\t\\usepackage{amsbsy} \t\t\t\t\\usepackage{mathrsfs} \t\t\t\t\\usepackage{upgreek} \t\t\t\t\\setlength{\\oddsidemargin}{-69pt} \t\t\t\t\\begin{document}$$I=\\frac{I_{\\mathrm{high}}-{I}_{\\mathrm{low}}}{C_{\\mathrm{high}}-{C}_{\\mathrm{low}}}\\ \\left(C-{C}_{\\mathrm{low}}\\right)+{I}_{\\mathrm{low}}$$\\end{document}I=Ihigh−IlowChigh−ClowC−Clow+Ilow\nIf multiple pollutants are measured, the calculated AQI is the highest value calculated from the above equation applied for each pollutant.whereIThe (air quality) index,\nCThe pollutant concentration,\nClowThe concentration breakpoint that is ≤C,\nChighThe concentration breakpoint that is ≥C,\nIlowThe index breakpoint corresponding to Clow,\nIhighThe index breakpoint corresponding to Chigh.\nMoreover, we have used unpaired Welch’s two-sample t test analysis to measure the statistically significant reduction in average AQI for all four sites, as t test allows us to compare the average values of the two data sets and determine if they came from the same population. The formula for calculating t-statistics is given as:\\documentclass[12pt]{minimal} \t\t\t\t\\usepackage{amsmath} \t\t\t\t\\usepackage{wasysym} \t\t\t\t\\usepackage{amsfonts} \t\t\t\t\\usepackage{amssymb} \t\t\t\t\\usepackage{amsbsy} \t\t\t\t\\usepackage{mathrsfs} \t\t\t\t\\usepackage{upgreek} \t\t\t\t\\setlength{\\oddsidemargin}{-69pt} \t\t\t\t\\begin{document}$$t=\\frac{{\\overline{x}}_1-{\\overline{x}}_2}{\\sqrt{\\frac{s_1^2}{n_1}-\\frac{s_2^2}{n_2}}}$$\\end{document}t=x¯1−x¯2s12n1−s22n2where, \\documentclass[12pt]{minimal} \t\t\t\t\\usepackage{amsmath} \t\t\t\t\\usepackage{wasysym} \t\t\t\t\\usepackage{amsfonts} \t\t\t\t\\usepackage{amssymb} \t\t\t\t\\usepackage{amsbsy} \t\t\t\t\\usepackage{mathrsfs} \t\t\t\t\\usepackage{upgreek} \t\t\t\t\\setlength{\\oddsidemargin}{-69pt} \t\t\t\t\\begin{document}$${\\overline{x}}_1$$\\end{document}x¯1 and \\documentclass[12pt]{minimal} \t\t\t\t\\usepackage{amsmath} \t\t\t\t\\usepackage{wasysym} \t\t\t\t\\usepackage{amsfonts} \t\t\t\t\\usepackage{amssymb} \t\t\t\t\\usepackage{amsbsy} \t\t\t\t\\usepackage{mathrsfs} \t\t\t\t\\usepackage{upgreek} \t\t\t\t\\setlength{\\oddsidemargin}{-69pt} \t\t\t\t\\begin{document}$${\\overline{x}}_2$$\\end{document}x¯2 are the sample means, n1 and n2 are the sample sizes, and \\documentclass[12pt]{minimal} \t\t\t\t\\usepackage{amsmath} \t\t\t\t\\usepackage{wasysym} \t\t\t\t\\usepackage{amsfonts} \t\t\t\t\\usepackage{amssymb} \t\t\t\t\\usepackage{amsbsy} \t\t\t\t\\usepackage{mathrsfs} \t\t\t\t\\usepackage{upgreek} \t\t\t\t\\setlength{\\oddsidemargin}{-69pt} \t\t\t\t\\begin{document}$${s}_1^2$$\\end{document}s12 and \\documentclass[12pt]{minimal} \t\t\t\t\\usepackage{amsmath} \t\t\t\t\\usepackage{wasysym} \t\t\t\t\\usepackage{amsfonts} \t\t\t\t\\usepackage{amssymb} \t\t\t\t\\usepackage{amsbsy} \t\t\t\t\\usepackage{mathrsfs} \t\t\t\t\\usepackage{upgreek} \t\t\t\t\\setlength{\\oddsidemargin}{-69pt} \t\t\t\t\\begin{document}$${s}_2^2$$\\end{document}s22 are the sample variances for samples 1 and 2 respectively.\nTo find out the most prominent pollutant concerning AQI statistically, we have done Pearson’s correlation analysis by the means of plotting heatmaps corresponding to each site. Pearson’s correlation is also known as the “product-moment correlation coefficient” (PMCC) and is suitable for measuring the extent of the linear relationship between any two quantitative variables statistically. A Pearson’s correlation is a number ranging between − 1 and + 1 showing negative to positive linear correlation. Given a pair of random variables (X1, X2), the formula for Pearson’s correlation is given by\\documentclass[12pt]{minimal} \t\t\t\t\\usepackage{amsmath} \t\t\t\t\\usepackage{wasysym} \t\t\t\t\\usepackage{amsfonts} \t\t\t\t\\usepackage{amssymb} \t\t\t\t\\usepackage{amsbsy} \t\t\t\t\\usepackage{mathrsfs} \t\t\t\t\\usepackage{upgreek} \t\t\t\t\\setlength{\\oddsidemargin}{-69pt} \t\t\t\t\\begin{document}$${\\rho}_{X_1,{X}_2}=\\frac{Cov\\left({X}_1,{X}_2\\right)}{\\sigma_{X_1}\\ {\\sigma}_{X_2}}$$\\end{document}ρX1,X2=CovX1X2σX1σX2where Cov(X1, X2) is the covariance between the variables under study and \\documentclass[12pt]{minimal} \t\t\t\t\\usepackage{amsmath} \t\t\t\t\\usepackage{wasysym} \t\t\t\t\\usepackage{amsfonts} \t\t\t\t\\usepackage{amssymb} \t\t\t\t\\usepackage{amsbsy} \t\t\t\t\\usepackage{mathrsfs} \t\t\t\t\\usepackage{upgreek} \t\t\t\t\\setlength{\\oddsidemargin}{-69pt} \t\t\t\t\\begin{document}$${\\sigma}_{X_1},{\\sigma}_{X_2}$$\\end{document}σX1,σX2 are the standard deviation of X1, X2 respectively."}
2_test
{"project":"2_test","denotations":[{"id":"33040289-32783875-28470647","span":{"begin":1289,"end":1293},"obj":"32783875"}],"text":"Origin of data set\nAir quality index (AQI) reports daily air quality and its elevated level is associated with public health risks (Szyszkowicz 2019). Based on different national quality standards and dose-response relationships of pollutants, countries have different air quality indices (Zhang et al. 2020; Sofia et al. 2020). The Indian national air quality index considers eight pollutants (PM10, PM2.5, NO2, SO2, NH3, CO, O3, and Pb) with a 24-hourly averaging period. It is subdivided into six categories i.e., good (0–50), satisfactory (51–100), moderately polluted (101–200), poor (201–300), very poor (301–400), and severe (401–500) as shown in Fig. 1 (Perera 2018; Ghorani-Azam et al. 2016). The sub-indices for individual pollutants at a monitoring location are calculated using its 24-hourly average concentration value (8-hourly in case of CO and O3) and health breakpoint concentration range. The worst sub-index is the AQI for that location (https://app.cpcbccr.com/AQI_India/). An increment in AQI causes acute and chronic mode health concern especially in the older age people and in children (Januszek et al. 2020; Pant et al. 2020). Due to the COVID-19 pandemic confinement, there is a significant reduction in the level of such toxic pollutants globally (Selvam et al. 2020; Singh and Chauhan 2020).\nFig. 1 Indian national air quality index—category and range\nIn the present study, concentrations of different pollutants i.e., PM2.5 (diameter \u003c 2.5 μm), PM10 (diameter \u003c 10 μm), NO2, NH3, SO2, CO, ozone, and air quality index (AQI) were acquired from open access internet sources provided by the Central Pollution Control Board (CPCB), Ministry of Environment, Forests, and Climate Change (https://app.cpcbccr.com/AQI_India/). The data were recorded daily from January 1, 2020 to May 31, 2020, which is subdivided into two groups: (a) pre-lockdown period—January 1, 2020 to March 23, 2020, and (b) lockdown period—March 24, 2020 to May 31, 2020 at 17:00 IST among four different air quality monitoring stations of the CPCB for four major metropolitan cities in India i.e., site 1—ITO, Delhi, site 2—Worli, Mumbai, site 3—Jadavpur, Kolkata, and site 4—Manali Village, Chennai as shown in Fig. 2. For air quality assessment, % variations of air pollutants during the confinement period were compared with pre-lockdown values.\nFig. 2 The geography of monitoring stations among the populous sites of four major metropolitan cities in India\nThe air quality index is a piecewise linear function of the pollutant concentration. At the boundary between AQI categories, there is a discontinuous jump of one AQI unit. To convert from concentration to AQI, this equation is used:\\documentclass[12pt]{minimal} \t\t\t\t\\usepackage{amsmath} \t\t\t\t\\usepackage{wasysym} \t\t\t\t\\usepackage{amsfonts} \t\t\t\t\\usepackage{amssymb} \t\t\t\t\\usepackage{amsbsy} \t\t\t\t\\usepackage{mathrsfs} \t\t\t\t\\usepackage{upgreek} \t\t\t\t\\setlength{\\oddsidemargin}{-69pt} \t\t\t\t\\begin{document}$$I=\\frac{I_{\\mathrm{high}}-{I}_{\\mathrm{low}}}{C_{\\mathrm{high}}-{C}_{\\mathrm{low}}}\\ \\left(C-{C}_{\\mathrm{low}}\\right)+{I}_{\\mathrm{low}}$$\\end{document}I=Ihigh−IlowChigh−ClowC−Clow+Ilow\nIf multiple pollutants are measured, the calculated AQI is the highest value calculated from the above equation applied for each pollutant.whereIThe (air quality) index,\nCThe pollutant concentration,\nClowThe concentration breakpoint that is ≤C,\nChighThe concentration breakpoint that is ≥C,\nIlowThe index breakpoint corresponding to Clow,\nIhighThe index breakpoint corresponding to Chigh.\nMoreover, we have used unpaired Welch’s two-sample t test analysis to measure the statistically significant reduction in average AQI for all four sites, as t test allows us to compare the average values of the two data sets and determine if they came from the same population. The formula for calculating t-statistics is given as:\\documentclass[12pt]{minimal} \t\t\t\t\\usepackage{amsmath} \t\t\t\t\\usepackage{wasysym} \t\t\t\t\\usepackage{amsfonts} \t\t\t\t\\usepackage{amssymb} \t\t\t\t\\usepackage{amsbsy} \t\t\t\t\\usepackage{mathrsfs} \t\t\t\t\\usepackage{upgreek} \t\t\t\t\\setlength{\\oddsidemargin}{-69pt} \t\t\t\t\\begin{document}$$t=\\frac{{\\overline{x}}_1-{\\overline{x}}_2}{\\sqrt{\\frac{s_1^2}{n_1}-\\frac{s_2^2}{n_2}}}$$\\end{document}t=x¯1−x¯2s12n1−s22n2where, \\documentclass[12pt]{minimal} \t\t\t\t\\usepackage{amsmath} \t\t\t\t\\usepackage{wasysym} \t\t\t\t\\usepackage{amsfonts} \t\t\t\t\\usepackage{amssymb} \t\t\t\t\\usepackage{amsbsy} \t\t\t\t\\usepackage{mathrsfs} \t\t\t\t\\usepackage{upgreek} \t\t\t\t\\setlength{\\oddsidemargin}{-69pt} \t\t\t\t\\begin{document}$${\\overline{x}}_1$$\\end{document}x¯1 and \\documentclass[12pt]{minimal} \t\t\t\t\\usepackage{amsmath} \t\t\t\t\\usepackage{wasysym} \t\t\t\t\\usepackage{amsfonts} \t\t\t\t\\usepackage{amssymb} \t\t\t\t\\usepackage{amsbsy} \t\t\t\t\\usepackage{mathrsfs} \t\t\t\t\\usepackage{upgreek} \t\t\t\t\\setlength{\\oddsidemargin}{-69pt} \t\t\t\t\\begin{document}$${\\overline{x}}_2$$\\end{document}x¯2 are the sample means, n1 and n2 are the sample sizes, and \\documentclass[12pt]{minimal} \t\t\t\t\\usepackage{amsmath} \t\t\t\t\\usepackage{wasysym} \t\t\t\t\\usepackage{amsfonts} \t\t\t\t\\usepackage{amssymb} \t\t\t\t\\usepackage{amsbsy} \t\t\t\t\\usepackage{mathrsfs} \t\t\t\t\\usepackage{upgreek} \t\t\t\t\\setlength{\\oddsidemargin}{-69pt} \t\t\t\t\\begin{document}$${s}_1^2$$\\end{document}s12 and \\documentclass[12pt]{minimal} \t\t\t\t\\usepackage{amsmath} \t\t\t\t\\usepackage{wasysym} \t\t\t\t\\usepackage{amsfonts} \t\t\t\t\\usepackage{amssymb} \t\t\t\t\\usepackage{amsbsy} \t\t\t\t\\usepackage{mathrsfs} \t\t\t\t\\usepackage{upgreek} \t\t\t\t\\setlength{\\oddsidemargin}{-69pt} \t\t\t\t\\begin{document}$${s}_2^2$$\\end{document}s22 are the sample variances for samples 1 and 2 respectively.\nTo find out the most prominent pollutant concerning AQI statistically, we have done Pearson’s correlation analysis by the means of plotting heatmaps corresponding to each site. Pearson’s correlation is also known as the “product-moment correlation coefficient” (PMCC) and is suitable for measuring the extent of the linear relationship between any two quantitative variables statistically. A Pearson’s correlation is a number ranging between − 1 and + 1 showing negative to positive linear correlation. Given a pair of random variables (X1, X2), the formula for Pearson’s correlation is given by\\documentclass[12pt]{minimal} \t\t\t\t\\usepackage{amsmath} \t\t\t\t\\usepackage{wasysym} \t\t\t\t\\usepackage{amsfonts} \t\t\t\t\\usepackage{amssymb} \t\t\t\t\\usepackage{amsbsy} \t\t\t\t\\usepackage{mathrsfs} \t\t\t\t\\usepackage{upgreek} \t\t\t\t\\setlength{\\oddsidemargin}{-69pt} \t\t\t\t\\begin{document}$${\\rho}_{X_1,{X}_2}=\\frac{Cov\\left({X}_1,{X}_2\\right)}{\\sigma_{X_1}\\ {\\sigma}_{X_2}}$$\\end{document}ρX1,X2=CovX1X2σX1σX2where Cov(X1, X2) is the covariance between the variables under study and \\documentclass[12pt]{minimal} \t\t\t\t\\usepackage{amsmath} \t\t\t\t\\usepackage{wasysym} \t\t\t\t\\usepackage{amsfonts} \t\t\t\t\\usepackage{amssymb} \t\t\t\t\\usepackage{amsbsy} \t\t\t\t\\usepackage{mathrsfs} \t\t\t\t\\usepackage{upgreek} \t\t\t\t\\setlength{\\oddsidemargin}{-69pt} \t\t\t\t\\begin{document}$${\\sigma}_{X_1},{\\sigma}_{X_2}$$\\end{document}σX1,σX2 are the standard deviation of X1, X2 respectively."}
LitCovid-sentences
{"project":"LitCovid-sentences","denotations":[{"id":"T25","span":{"begin":0,"end":18},"obj":"Sentence"},{"id":"T26","span":{"begin":19,"end":150},"obj":"Sentence"},{"id":"T27","span":{"begin":151,"end":302},"obj":"Sentence"},{"id":"T28","span":{"begin":303,"end":321},"obj":"Sentence"},{"id":"T29","span":{"begin":322,"end":328},"obj":"Sentence"},{"id":"T30","span":{"begin":329,"end":473},"obj":"Sentence"},{"id":"T31","span":{"begin":474,"end":694},"obj":"Sentence"},{"id":"T32","span":{"begin":695,"end":701},"obj":"Sentence"},{"id":"T33","span":{"begin":702,"end":906},"obj":"Sentence"},{"id":"T34","span":{"begin":907,"end":993},"obj":"Sentence"},{"id":"T35","span":{"begin":994,"end":1126},"obj":"Sentence"},{"id":"T36","span":{"begin":1127,"end":1144},"obj":"Sentence"},{"id":"T37","span":{"begin":1145,"end":1151},"obj":"Sentence"},{"id":"T38","span":{"begin":1152,"end":1288},"obj":"Sentence"},{"id":"T39","span":{"begin":1289,"end":1319},"obj":"Sentence"},{"id":"T40","span":{"begin":1320,"end":1379},"obj":"Sentence"},{"id":"T41","span":{"begin":1380,"end":1747},"obj":"Sentence"},{"id":"T42","span":{"begin":1748,"end":2215},"obj":"Sentence"},{"id":"T43","span":{"begin":2216,"end":2344},"obj":"Sentence"},{"id":"T44","span":{"begin":2345,"end":2456},"obj":"Sentence"},{"id":"T45","span":{"begin":2457,"end":2541},"obj":"Sentence"},{"id":"T46","span":{"begin":2542,"end":2628},"obj":"Sentence"},{"id":"T47","span":{"begin":2629,"end":3141},"obj":"Sentence"},{"id":"T48","span":{"begin":3142,"end":3311},"obj":"Sentence"},{"id":"T49","span":{"begin":3312,"end":3341},"obj":"Sentence"},{"id":"T50","span":{"begin":3342,"end":3386},"obj":"Sentence"},{"id":"T51","span":{"begin":3387,"end":3432},"obj":"Sentence"},{"id":"T52","span":{"begin":3433,"end":3480},"obj":"Sentence"},{"id":"T53","span":{"begin":3481,"end":3530},"obj":"Sentence"},{"id":"T54","span":{"begin":3531,"end":3807},"obj":"Sentence"},{"id":"T55","span":{"begin":3808,"end":5570},"obj":"Sentence"},{"id":"T56","span":{"begin":5571,"end":5747},"obj":"Sentence"},{"id":"T57","span":{"begin":5748,"end":5960},"obj":"Sentence"},{"id":"T58","span":{"begin":5961,"end":6073},"obj":"Sentence"},{"id":"T59","span":{"begin":6074,"end":6994},"obj":"Sentence"}],"namespaces":[{"prefix":"_base","uri":"http://pubannotation.org/ontology/tao.owl#"}],"text":"Origin of data set\nAir quality index (AQI) reports daily air quality and its elevated level is associated with public health risks (Szyszkowicz 2019). Based on different national quality standards and dose-response relationships of pollutants, countries have different air quality indices (Zhang et al. 2020; Sofia et al. 2020). The Indian national air quality index considers eight pollutants (PM10, PM2.5, NO2, SO2, NH3, CO, O3, and Pb) with a 24-hourly averaging period. It is subdivided into six categories i.e., good (0–50), satisfactory (51–100), moderately polluted (101–200), poor (201–300), very poor (301–400), and severe (401–500) as shown in Fig. 1 (Perera 2018; Ghorani-Azam et al. 2016). The sub-indices for individual pollutants at a monitoring location are calculated using its 24-hourly average concentration value (8-hourly in case of CO and O3) and health breakpoint concentration range. The worst sub-index is the AQI for that location (https://app.cpcbccr.com/AQI_India/). An increment in AQI causes acute and chronic mode health concern especially in the older age people and in children (Januszek et al. 2020; Pant et al. 2020). Due to the COVID-19 pandemic confinement, there is a significant reduction in the level of such toxic pollutants globally (Selvam et al. 2020; Singh and Chauhan 2020).\nFig. 1 Indian national air quality index—category and range\nIn the present study, concentrations of different pollutants i.e., PM2.5 (diameter \u003c 2.5 μm), PM10 (diameter \u003c 10 μm), NO2, NH3, SO2, CO, ozone, and air quality index (AQI) were acquired from open access internet sources provided by the Central Pollution Control Board (CPCB), Ministry of Environment, Forests, and Climate Change (https://app.cpcbccr.com/AQI_India/). The data were recorded daily from January 1, 2020 to May 31, 2020, which is subdivided into two groups: (a) pre-lockdown period—January 1, 2020 to March 23, 2020, and (b) lockdown period—March 24, 2020 to May 31, 2020 at 17:00 IST among four different air quality monitoring stations of the CPCB for four major metropolitan cities in India i.e., site 1—ITO, Delhi, site 2—Worli, Mumbai, site 3—Jadavpur, Kolkata, and site 4—Manali Village, Chennai as shown in Fig. 2. For air quality assessment, % variations of air pollutants during the confinement period were compared with pre-lockdown values.\nFig. 2 The geography of monitoring stations among the populous sites of four major metropolitan cities in India\nThe air quality index is a piecewise linear function of the pollutant concentration. At the boundary between AQI categories, there is a discontinuous jump of one AQI unit. To convert from concentration to AQI, this equation is used:\\documentclass[12pt]{minimal} \t\t\t\t\\usepackage{amsmath} \t\t\t\t\\usepackage{wasysym} \t\t\t\t\\usepackage{amsfonts} \t\t\t\t\\usepackage{amssymb} \t\t\t\t\\usepackage{amsbsy} \t\t\t\t\\usepackage{mathrsfs} \t\t\t\t\\usepackage{upgreek} \t\t\t\t\\setlength{\\oddsidemargin}{-69pt} \t\t\t\t\\begin{document}$$I=\\frac{I_{\\mathrm{high}}-{I}_{\\mathrm{low}}}{C_{\\mathrm{high}}-{C}_{\\mathrm{low}}}\\ \\left(C-{C}_{\\mathrm{low}}\\right)+{I}_{\\mathrm{low}}$$\\end{document}I=Ihigh−IlowChigh−ClowC−Clow+Ilow\nIf multiple pollutants are measured, the calculated AQI is the highest value calculated from the above equation applied for each pollutant.whereIThe (air quality) index,\nCThe pollutant concentration,\nClowThe concentration breakpoint that is ≤C,\nChighThe concentration breakpoint that is ≥C,\nIlowThe index breakpoint corresponding to Clow,\nIhighThe index breakpoint corresponding to Chigh.\nMoreover, we have used unpaired Welch’s two-sample t test analysis to measure the statistically significant reduction in average AQI for all four sites, as t test allows us to compare the average values of the two data sets and determine if they came from the same population. The formula for calculating t-statistics is given as:\\documentclass[12pt]{minimal} \t\t\t\t\\usepackage{amsmath} \t\t\t\t\\usepackage{wasysym} \t\t\t\t\\usepackage{amsfonts} \t\t\t\t\\usepackage{amssymb} \t\t\t\t\\usepackage{amsbsy} \t\t\t\t\\usepackage{mathrsfs} \t\t\t\t\\usepackage{upgreek} \t\t\t\t\\setlength{\\oddsidemargin}{-69pt} \t\t\t\t\\begin{document}$$t=\\frac{{\\overline{x}}_1-{\\overline{x}}_2}{\\sqrt{\\frac{s_1^2}{n_1}-\\frac{s_2^2}{n_2}}}$$\\end{document}t=x¯1−x¯2s12n1−s22n2where, \\documentclass[12pt]{minimal} \t\t\t\t\\usepackage{amsmath} \t\t\t\t\\usepackage{wasysym} \t\t\t\t\\usepackage{amsfonts} \t\t\t\t\\usepackage{amssymb} \t\t\t\t\\usepackage{amsbsy} \t\t\t\t\\usepackage{mathrsfs} \t\t\t\t\\usepackage{upgreek} \t\t\t\t\\setlength{\\oddsidemargin}{-69pt} \t\t\t\t\\begin{document}$${\\overline{x}}_1$$\\end{document}x¯1 and \\documentclass[12pt]{minimal} \t\t\t\t\\usepackage{amsmath} \t\t\t\t\\usepackage{wasysym} \t\t\t\t\\usepackage{amsfonts} \t\t\t\t\\usepackage{amssymb} \t\t\t\t\\usepackage{amsbsy} \t\t\t\t\\usepackage{mathrsfs} \t\t\t\t\\usepackage{upgreek} \t\t\t\t\\setlength{\\oddsidemargin}{-69pt} \t\t\t\t\\begin{document}$${\\overline{x}}_2$$\\end{document}x¯2 are the sample means, n1 and n2 are the sample sizes, and \\documentclass[12pt]{minimal} \t\t\t\t\\usepackage{amsmath} \t\t\t\t\\usepackage{wasysym} \t\t\t\t\\usepackage{amsfonts} \t\t\t\t\\usepackage{amssymb} \t\t\t\t\\usepackage{amsbsy} \t\t\t\t\\usepackage{mathrsfs} \t\t\t\t\\usepackage{upgreek} \t\t\t\t\\setlength{\\oddsidemargin}{-69pt} \t\t\t\t\\begin{document}$${s}_1^2$$\\end{document}s12 and \\documentclass[12pt]{minimal} \t\t\t\t\\usepackage{amsmath} \t\t\t\t\\usepackage{wasysym} \t\t\t\t\\usepackage{amsfonts} \t\t\t\t\\usepackage{amssymb} \t\t\t\t\\usepackage{amsbsy} \t\t\t\t\\usepackage{mathrsfs} \t\t\t\t\\usepackage{upgreek} \t\t\t\t\\setlength{\\oddsidemargin}{-69pt} \t\t\t\t\\begin{document}$${s}_2^2$$\\end{document}s22 are the sample variances for samples 1 and 2 respectively.\nTo find out the most prominent pollutant concerning AQI statistically, we have done Pearson’s correlation analysis by the means of plotting heatmaps corresponding to each site. Pearson’s correlation is also known as the “product-moment correlation coefficient” (PMCC) and is suitable for measuring the extent of the linear relationship between any two quantitative variables statistically. A Pearson’s correlation is a number ranging between − 1 and + 1 showing negative to positive linear correlation. Given a pair of random variables (X1, X2), the formula for Pearson’s correlation is given by\\documentclass[12pt]{minimal} \t\t\t\t\\usepackage{amsmath} \t\t\t\t\\usepackage{wasysym} \t\t\t\t\\usepackage{amsfonts} \t\t\t\t\\usepackage{amssymb} \t\t\t\t\\usepackage{amsbsy} \t\t\t\t\\usepackage{mathrsfs} \t\t\t\t\\usepackage{upgreek} \t\t\t\t\\setlength{\\oddsidemargin}{-69pt} \t\t\t\t\\begin{document}$${\\rho}_{X_1,{X}_2}=\\frac{Cov\\left({X}_1,{X}_2\\right)}{\\sigma_{X_1}\\ {\\sigma}_{X_2}}$$\\end{document}ρX1,X2=CovX1X2σX1σX2where Cov(X1, X2) is the covariance between the variables under study and \\documentclass[12pt]{minimal} \t\t\t\t\\usepackage{amsmath} \t\t\t\t\\usepackage{wasysym} \t\t\t\t\\usepackage{amsfonts} \t\t\t\t\\usepackage{amssymb} \t\t\t\t\\usepackage{amsbsy} \t\t\t\t\\usepackage{mathrsfs} \t\t\t\t\\usepackage{upgreek} \t\t\t\t\\setlength{\\oddsidemargin}{-69pt} \t\t\t\t\\begin{document}$${\\sigma}_{X_1},{\\sigma}_{X_2}$$\\end{document}σX1,σX2 are the standard deviation of X1, X2 respectively."}
MyTest
{"project":"MyTest","denotations":[{"id":"33040289-32783875-28470647","span":{"begin":1289,"end":1293},"obj":"32783875"}],"namespaces":[{"prefix":"_base","uri":"https://www.uniprot.org/uniprot/testbase"},{"prefix":"UniProtKB","uri":"https://www.uniprot.org/uniprot/"},{"prefix":"uniprot","uri":"https://www.uniprot.org/uniprotkb/"}],"text":"Origin of data set\nAir quality index (AQI) reports daily air quality and its elevated level is associated with public health risks (Szyszkowicz 2019). Based on different national quality standards and dose-response relationships of pollutants, countries have different air quality indices (Zhang et al. 2020; Sofia et al. 2020). The Indian national air quality index considers eight pollutants (PM10, PM2.5, NO2, SO2, NH3, CO, O3, and Pb) with a 24-hourly averaging period. It is subdivided into six categories i.e., good (0–50), satisfactory (51–100), moderately polluted (101–200), poor (201–300), very poor (301–400), and severe (401–500) as shown in Fig. 1 (Perera 2018; Ghorani-Azam et al. 2016). The sub-indices for individual pollutants at a monitoring location are calculated using its 24-hourly average concentration value (8-hourly in case of CO and O3) and health breakpoint concentration range. The worst sub-index is the AQI for that location (https://app.cpcbccr.com/AQI_India/). An increment in AQI causes acute and chronic mode health concern especially in the older age people and in children (Januszek et al. 2020; Pant et al. 2020). Due to the COVID-19 pandemic confinement, there is a significant reduction in the level of such toxic pollutants globally (Selvam et al. 2020; Singh and Chauhan 2020).\nFig. 1 Indian national air quality index—category and range\nIn the present study, concentrations of different pollutants i.e., PM2.5 (diameter \u003c 2.5 μm), PM10 (diameter \u003c 10 μm), NO2, NH3, SO2, CO, ozone, and air quality index (AQI) were acquired from open access internet sources provided by the Central Pollution Control Board (CPCB), Ministry of Environment, Forests, and Climate Change (https://app.cpcbccr.com/AQI_India/). The data were recorded daily from January 1, 2020 to May 31, 2020, which is subdivided into two groups: (a) pre-lockdown period—January 1, 2020 to March 23, 2020, and (b) lockdown period—March 24, 2020 to May 31, 2020 at 17:00 IST among four different air quality monitoring stations of the CPCB for four major metropolitan cities in India i.e., site 1—ITO, Delhi, site 2—Worli, Mumbai, site 3—Jadavpur, Kolkata, and site 4—Manali Village, Chennai as shown in Fig. 2. For air quality assessment, % variations of air pollutants during the confinement period were compared with pre-lockdown values.\nFig. 2 The geography of monitoring stations among the populous sites of four major metropolitan cities in India\nThe air quality index is a piecewise linear function of the pollutant concentration. At the boundary between AQI categories, there is a discontinuous jump of one AQI unit. To convert from concentration to AQI, this equation is used:\\documentclass[12pt]{minimal} \t\t\t\t\\usepackage{amsmath} \t\t\t\t\\usepackage{wasysym} \t\t\t\t\\usepackage{amsfonts} \t\t\t\t\\usepackage{amssymb} \t\t\t\t\\usepackage{amsbsy} \t\t\t\t\\usepackage{mathrsfs} \t\t\t\t\\usepackage{upgreek} \t\t\t\t\\setlength{\\oddsidemargin}{-69pt} \t\t\t\t\\begin{document}$$I=\\frac{I_{\\mathrm{high}}-{I}_{\\mathrm{low}}}{C_{\\mathrm{high}}-{C}_{\\mathrm{low}}}\\ \\left(C-{C}_{\\mathrm{low}}\\right)+{I}_{\\mathrm{low}}$$\\end{document}I=Ihigh−IlowChigh−ClowC−Clow+Ilow\nIf multiple pollutants are measured, the calculated AQI is the highest value calculated from the above equation applied for each pollutant.whereIThe (air quality) index,\nCThe pollutant concentration,\nClowThe concentration breakpoint that is ≤C,\nChighThe concentration breakpoint that is ≥C,\nIlowThe index breakpoint corresponding to Clow,\nIhighThe index breakpoint corresponding to Chigh.\nMoreover, we have used unpaired Welch’s two-sample t test analysis to measure the statistically significant reduction in average AQI for all four sites, as t test allows us to compare the average values of the two data sets and determine if they came from the same population. The formula for calculating t-statistics is given as:\\documentclass[12pt]{minimal} \t\t\t\t\\usepackage{amsmath} \t\t\t\t\\usepackage{wasysym} \t\t\t\t\\usepackage{amsfonts} \t\t\t\t\\usepackage{amssymb} \t\t\t\t\\usepackage{amsbsy} \t\t\t\t\\usepackage{mathrsfs} \t\t\t\t\\usepackage{upgreek} \t\t\t\t\\setlength{\\oddsidemargin}{-69pt} \t\t\t\t\\begin{document}$$t=\\frac{{\\overline{x}}_1-{\\overline{x}}_2}{\\sqrt{\\frac{s_1^2}{n_1}-\\frac{s_2^2}{n_2}}}$$\\end{document}t=x¯1−x¯2s12n1−s22n2where, \\documentclass[12pt]{minimal} \t\t\t\t\\usepackage{amsmath} \t\t\t\t\\usepackage{wasysym} \t\t\t\t\\usepackage{amsfonts} \t\t\t\t\\usepackage{amssymb} \t\t\t\t\\usepackage{amsbsy} \t\t\t\t\\usepackage{mathrsfs} \t\t\t\t\\usepackage{upgreek} \t\t\t\t\\setlength{\\oddsidemargin}{-69pt} \t\t\t\t\\begin{document}$${\\overline{x}}_1$$\\end{document}x¯1 and \\documentclass[12pt]{minimal} \t\t\t\t\\usepackage{amsmath} \t\t\t\t\\usepackage{wasysym} \t\t\t\t\\usepackage{amsfonts} \t\t\t\t\\usepackage{amssymb} \t\t\t\t\\usepackage{amsbsy} \t\t\t\t\\usepackage{mathrsfs} \t\t\t\t\\usepackage{upgreek} \t\t\t\t\\setlength{\\oddsidemargin}{-69pt} \t\t\t\t\\begin{document}$${\\overline{x}}_2$$\\end{document}x¯2 are the sample means, n1 and n2 are the sample sizes, and \\documentclass[12pt]{minimal} \t\t\t\t\\usepackage{amsmath} \t\t\t\t\\usepackage{wasysym} \t\t\t\t\\usepackage{amsfonts} \t\t\t\t\\usepackage{amssymb} \t\t\t\t\\usepackage{amsbsy} \t\t\t\t\\usepackage{mathrsfs} \t\t\t\t\\usepackage{upgreek} \t\t\t\t\\setlength{\\oddsidemargin}{-69pt} \t\t\t\t\\begin{document}$${s}_1^2$$\\end{document}s12 and \\documentclass[12pt]{minimal} \t\t\t\t\\usepackage{amsmath} \t\t\t\t\\usepackage{wasysym} \t\t\t\t\\usepackage{amsfonts} \t\t\t\t\\usepackage{amssymb} \t\t\t\t\\usepackage{amsbsy} \t\t\t\t\\usepackage{mathrsfs} \t\t\t\t\\usepackage{upgreek} \t\t\t\t\\setlength{\\oddsidemargin}{-69pt} \t\t\t\t\\begin{document}$${s}_2^2$$\\end{document}s22 are the sample variances for samples 1 and 2 respectively.\nTo find out the most prominent pollutant concerning AQI statistically, we have done Pearson’s correlation analysis by the means of plotting heatmaps corresponding to each site. Pearson’s correlation is also known as the “product-moment correlation coefficient” (PMCC) and is suitable for measuring the extent of the linear relationship between any two quantitative variables statistically. A Pearson’s correlation is a number ranging between − 1 and + 1 showing negative to positive linear correlation. Given a pair of random variables (X1, X2), the formula for Pearson’s correlation is given by\\documentclass[12pt]{minimal} \t\t\t\t\\usepackage{amsmath} \t\t\t\t\\usepackage{wasysym} \t\t\t\t\\usepackage{amsfonts} \t\t\t\t\\usepackage{amssymb} \t\t\t\t\\usepackage{amsbsy} \t\t\t\t\\usepackage{mathrsfs} \t\t\t\t\\usepackage{upgreek} \t\t\t\t\\setlength{\\oddsidemargin}{-69pt} \t\t\t\t\\begin{document}$${\\rho}_{X_1,{X}_2}=\\frac{Cov\\left({X}_1,{X}_2\\right)}{\\sigma_{X_1}\\ {\\sigma}_{X_2}}$$\\end{document}ρX1,X2=CovX1X2σX1σX2where Cov(X1, X2) is the covariance between the variables under study and \\documentclass[12pt]{minimal} \t\t\t\t\\usepackage{amsmath} \t\t\t\t\\usepackage{wasysym} \t\t\t\t\\usepackage{amsfonts} \t\t\t\t\\usepackage{amssymb} \t\t\t\t\\usepackage{amsbsy} \t\t\t\t\\usepackage{mathrsfs} \t\t\t\t\\usepackage{upgreek} \t\t\t\t\\setlength{\\oddsidemargin}{-69pt} \t\t\t\t\\begin{document}$${\\sigma}_{X_1},{\\sigma}_{X_2}$$\\end{document}σX1,σX2 are the standard deviation of X1, X2 respectively."}