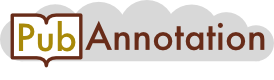
PMC:7519301 / 20081-23837
Annnotations
LitCovid-PD-FMA-UBERON
{"project":"LitCovid-PD-FMA-UBERON","denotations":[{"id":"T82","span":{"begin":643,"end":650},"obj":"Body_part"},{"id":"T83","span":{"begin":743,"end":750},"obj":"Body_part"},{"id":"T84","span":{"begin":1612,"end":1620},"obj":"Body_part"},{"id":"T85","span":{"begin":2030,"end":2038},"obj":"Body_part"},{"id":"T86","span":{"begin":2629,"end":2644},"obj":"Body_part"},{"id":"T87","span":{"begin":2979,"end":2983},"obj":"Body_part"},{"id":"T88","span":{"begin":3178,"end":3184},"obj":"Body_part"},{"id":"T89","span":{"begin":3455,"end":3461},"obj":"Body_part"}],"attributes":[{"id":"A82","pred":"fma_id","subj":"T82","obj":"http://purl.org/sig/ont/fma/fma84116"},{"id":"A83","pred":"fma_id","subj":"T83","obj":"http://purl.org/sig/ont/fma/fma84116"},{"id":"A84","pred":"fma_id","subj":"T84","obj":"http://purl.org/sig/ont/fma/fma14542"},{"id":"A85","pred":"fma_id","subj":"T85","obj":"http://purl.org/sig/ont/fma/fma14542"},{"id":"A86","pred":"fma_id","subj":"T86","obj":"http://purl.org/sig/ont/fma/fma74402"},{"id":"A87","pred":"fma_id","subj":"T87","obj":"http://purl.org/sig/ont/fma/fma74402"},{"id":"A88","pred":"fma_id","subj":"T88","obj":"http://purl.org/sig/ont/fma/fma84116"},{"id":"A89","pred":"fma_id","subj":"T89","obj":"http://purl.org/sig/ont/fma/fma84116"}],"text":"No Evidence of Differentiation of the Viral Population.\nWhile there was only limited evidence of diversification at selected sites, we also assessed whether subpopulations among the globally circulating viral population had become genetically differentiated over time. To do so, we used two measures of population differentiation, the GST and D statistics, which characterize changes in allele frequency across populations and can show fitness differences between subpopulations (30–32). Genetic distances between two subpopulations can range between 0 and 1, indicating no and complete differentiation, respectively. We initially compared 30 genomes sampled from the initial outbreak in Wuhan, China, with subsampled alignments of the 18,484 genomes sampled subsequently across the globe. Although distances varied across genes, the median genetic distance between these subpopulations was small for both GST (0.0049 ± 0.0047) and D (0.0053 ± 0.0272), indicating little differentiation between the initial outbreak and its global derivatives in the pandemic (Fig. 4A). We then compared subpopulations sampled before and after each consecutive week. Similarly, genetic distances between subpopulations were small for both GST (0.0058 ± 0.0096) and D (0.0098 ± 0.0650) and tended to narrow over time rather than diverge (Fig. 4 B and C). Signatures of host adaptation can also be seen in the branching patterns of viral phylogenies. Bursts in transmissibility are emblematic of increases in relative viral fitness and are reflected in imbalances in the phylogeny, which can be estimated at each internal node (SI Appendix, Figs. S9 and S10) (33–35). We estimated phylogenetic η (36, 37) at each internal node of the SARS-CoV-2 phylogeny reconstructed from subsampled (10%) alignments and compared the distribution of estimates through time to phylogenies simulated under models of neutral and positive time-dependent rates (b(t) = beα(t)). Simulation analyses demonstrated that this metric was robust against sampling fraction (SI Appendix, Fig. S10). The distribution of η in the SARS-CoV-2 phylogenies adhered to expectations of the neutral model and deviated significantly (Student’s t test, P \u003c 0.001) from those of positive time-dependent rates for selection coefficients α ≥ 0.2 (Fig. 4 D and E). Together, the SARS-CoV-2 population and phylogenetic dynamics showed little evidence that the global spread of SARS-CoV-2 was related to viral fitness effects.\nFig. 4. Limited evidence of adaptation of the viral population. (A–C) Bootstrapped global estimates of Nei’s GST and Jost’s D for population differentiation for each structural gene. (A) Estimates of Nei’s GST (closed circles) and Jost’s D (open circles) comparing sequences sampled from the Hubei province to sequences subsequently sampled globally. Estimates of (B) Nei’s GST and (C) Jost’s D comparing sequences sampled before or after a specific date. Lines connect the median estimates across datasets for each gene. (D) Ln-transformed phylogenetic η, indicative of the number of iterative events in the sampled subtree, for subtrees from each internal node (after the root) of a down-sampled SARS-CoV-2 whole-genome phylogeny (dark gray), of a phylogeny simulated under neutral parameters (gold), and of a phylogeny simulated under positive time-dependent rates (b(t) = 0.01e0.4t, green). (E) Box plot of ln-transformed phylogenetic η estimates across all down-sampled SARS-CoV-2 whole-genome phylogenies, phylogenies simulated under neutral parameters, and phylogenies simulated under different positive time dependencies, α. Asterisks indicate significant differences in mean values (Student’s t test, P \u003c 0.05) between the SARS-CoV-2 and positive time-dependent phylogenies at each α."}
LitCovid-PD-MONDO
{"project":"LitCovid-PD-MONDO","denotations":[{"id":"T97","span":{"begin":1715,"end":1723},"obj":"Disease"},{"id":"T98","span":{"begin":1715,"end":1719},"obj":"Disease"},{"id":"T99","span":{"begin":2080,"end":2088},"obj":"Disease"},{"id":"T100","span":{"begin":2080,"end":2084},"obj":"Disease"},{"id":"T101","span":{"begin":2316,"end":2324},"obj":"Disease"},{"id":"T102","span":{"begin":2316,"end":2320},"obj":"Disease"},{"id":"T103","span":{"begin":2413,"end":2421},"obj":"Disease"},{"id":"T104","span":{"begin":2413,"end":2417},"obj":"Disease"},{"id":"T105","span":{"begin":3161,"end":3169},"obj":"Disease"},{"id":"T106","span":{"begin":3161,"end":3165},"obj":"Disease"},{"id":"T107","span":{"begin":3438,"end":3446},"obj":"Disease"},{"id":"T108","span":{"begin":3438,"end":3442},"obj":"Disease"},{"id":"T109","span":{"begin":3695,"end":3703},"obj":"Disease"},{"id":"T110","span":{"begin":3695,"end":3699},"obj":"Disease"}],"attributes":[{"id":"A97","pred":"mondo_id","subj":"T97","obj":"http://purl.obolibrary.org/obo/MONDO_0005091"},{"id":"A98","pred":"mondo_id","subj":"T98","obj":"http://purl.obolibrary.org/obo/MONDO_0005091"},{"id":"A99","pred":"mondo_id","subj":"T99","obj":"http://purl.obolibrary.org/obo/MONDO_0005091"},{"id":"A100","pred":"mondo_id","subj":"T100","obj":"http://purl.obolibrary.org/obo/MONDO_0005091"},{"id":"A101","pred":"mondo_id","subj":"T101","obj":"http://purl.obolibrary.org/obo/MONDO_0005091"},{"id":"A102","pred":"mondo_id","subj":"T102","obj":"http://purl.obolibrary.org/obo/MONDO_0005091"},{"id":"A103","pred":"mondo_id","subj":"T103","obj":"http://purl.obolibrary.org/obo/MONDO_0005091"},{"id":"A104","pred":"mondo_id","subj":"T104","obj":"http://purl.obolibrary.org/obo/MONDO_0005091"},{"id":"A105","pred":"mondo_id","subj":"T105","obj":"http://purl.obolibrary.org/obo/MONDO_0005091"},{"id":"A106","pred":"mondo_id","subj":"T106","obj":"http://purl.obolibrary.org/obo/MONDO_0005091"},{"id":"A107","pred":"mondo_id","subj":"T107","obj":"http://purl.obolibrary.org/obo/MONDO_0005091"},{"id":"A108","pred":"mondo_id","subj":"T108","obj":"http://purl.obolibrary.org/obo/MONDO_0005091"},{"id":"A109","pred":"mondo_id","subj":"T109","obj":"http://purl.obolibrary.org/obo/MONDO_0005091"},{"id":"A110","pred":"mondo_id","subj":"T110","obj":"http://purl.obolibrary.org/obo/MONDO_0005091"}],"text":"No Evidence of Differentiation of the Viral Population.\nWhile there was only limited evidence of diversification at selected sites, we also assessed whether subpopulations among the globally circulating viral population had become genetically differentiated over time. To do so, we used two measures of population differentiation, the GST and D statistics, which characterize changes in allele frequency across populations and can show fitness differences between subpopulations (30–32). Genetic distances between two subpopulations can range between 0 and 1, indicating no and complete differentiation, respectively. We initially compared 30 genomes sampled from the initial outbreak in Wuhan, China, with subsampled alignments of the 18,484 genomes sampled subsequently across the globe. Although distances varied across genes, the median genetic distance between these subpopulations was small for both GST (0.0049 ± 0.0047) and D (0.0053 ± 0.0272), indicating little differentiation between the initial outbreak and its global derivatives in the pandemic (Fig. 4A). We then compared subpopulations sampled before and after each consecutive week. Similarly, genetic distances between subpopulations were small for both GST (0.0058 ± 0.0096) and D (0.0098 ± 0.0650) and tended to narrow over time rather than diverge (Fig. 4 B and C). Signatures of host adaptation can also be seen in the branching patterns of viral phylogenies. Bursts in transmissibility are emblematic of increases in relative viral fitness and are reflected in imbalances in the phylogeny, which can be estimated at each internal node (SI Appendix, Figs. S9 and S10) (33–35). We estimated phylogenetic η (36, 37) at each internal node of the SARS-CoV-2 phylogeny reconstructed from subsampled (10%) alignments and compared the distribution of estimates through time to phylogenies simulated under models of neutral and positive time-dependent rates (b(t) = beα(t)). Simulation analyses demonstrated that this metric was robust against sampling fraction (SI Appendix, Fig. S10). The distribution of η in the SARS-CoV-2 phylogenies adhered to expectations of the neutral model and deviated significantly (Student’s t test, P \u003c 0.001) from those of positive time-dependent rates for selection coefficients α ≥ 0.2 (Fig. 4 D and E). Together, the SARS-CoV-2 population and phylogenetic dynamics showed little evidence that the global spread of SARS-CoV-2 was related to viral fitness effects.\nFig. 4. Limited evidence of adaptation of the viral population. (A–C) Bootstrapped global estimates of Nei’s GST and Jost’s D for population differentiation for each structural gene. (A) Estimates of Nei’s GST (closed circles) and Jost’s D (open circles) comparing sequences sampled from the Hubei province to sequences subsequently sampled globally. Estimates of (B) Nei’s GST and (C) Jost’s D comparing sequences sampled before or after a specific date. Lines connect the median estimates across datasets for each gene. (D) Ln-transformed phylogenetic η, indicative of the number of iterative events in the sampled subtree, for subtrees from each internal node (after the root) of a down-sampled SARS-CoV-2 whole-genome phylogeny (dark gray), of a phylogeny simulated under neutral parameters (gold), and of a phylogeny simulated under positive time-dependent rates (b(t) = 0.01e0.4t, green). (E) Box plot of ln-transformed phylogenetic η estimates across all down-sampled SARS-CoV-2 whole-genome phylogenies, phylogenies simulated under neutral parameters, and phylogenies simulated under different positive time dependencies, α. Asterisks indicate significant differences in mean values (Student’s t test, P \u003c 0.05) between the SARS-CoV-2 and positive time-dependent phylogenies at each α."}
LitCovid-PD-CLO
{"project":"LitCovid-PD-CLO","denotations":[{"id":"T178","span":{"begin":823,"end":833},"obj":"http://purl.obolibrary.org/obo/OGG_0000000002"},{"id":"T179","span":{"begin":1327,"end":1328},"obj":"http://purl.obolibrary.org/obo/CLO_0001021"},{"id":"T180","span":{"begin":1628,"end":1630},"obj":"http://purl.obolibrary.org/obo/CLO_0008935"},{"id":"T181","span":{"begin":1644,"end":1646},"obj":"http://purl.obolibrary.org/obo/CLO_0001000"},{"id":"T182","span":{"begin":1678,"end":1680},"obj":"http://purl.obolibrary.org/obo/CLO_0001313"},{"id":"T183","span":{"begin":1923,"end":1927},"obj":"http://purl.obolibrary.org/obo/CLO_0002040"},{"id":"T184","span":{"begin":2188,"end":2192},"obj":"http://purl.obolibrary.org/obo/UBERON_0000473"},{"id":"T185","span":{"begin":2528,"end":2529},"obj":"http://purl.obolibrary.org/obo/CLO_0001020"},{"id":"T186","span":{"begin":2640,"end":2644},"obj":"http://purl.obolibrary.org/obo/OGG_0000000002"},{"id":"T187","span":{"begin":2647,"end":2648},"obj":"http://purl.obolibrary.org/obo/CLO_0001020"},{"id":"T188","span":{"begin":2828,"end":2829},"obj":"http://purl.obolibrary.org/obo/CLO_0001021"},{"id":"T189","span":{"begin":2902,"end":2903},"obj":"http://purl.obolibrary.org/obo/CLO_0001020"},{"id":"T190","span":{"begin":2979,"end":2983},"obj":"http://purl.obolibrary.org/obo/OGG_0000000002"},{"id":"T191","span":{"begin":3146,"end":3147},"obj":"http://purl.obolibrary.org/obo/CLO_0001020"},{"id":"T192","span":{"begin":3211,"end":3212},"obj":"http://purl.obolibrary.org/obo/CLO_0001020"},{"id":"T193","span":{"begin":3273,"end":3274},"obj":"http://purl.obolibrary.org/obo/CLO_0001020"},{"id":"T194","span":{"begin":3332,"end":3336},"obj":"http://purl.obolibrary.org/obo/CLO_0002040"},{"id":"T195","span":{"begin":3667,"end":3671},"obj":"http://purl.obolibrary.org/obo/UBERON_0000473"}],"text":"No Evidence of Differentiation of the Viral Population.\nWhile there was only limited evidence of diversification at selected sites, we also assessed whether subpopulations among the globally circulating viral population had become genetically differentiated over time. To do so, we used two measures of population differentiation, the GST and D statistics, which characterize changes in allele frequency across populations and can show fitness differences between subpopulations (30–32). Genetic distances between two subpopulations can range between 0 and 1, indicating no and complete differentiation, respectively. We initially compared 30 genomes sampled from the initial outbreak in Wuhan, China, with subsampled alignments of the 18,484 genomes sampled subsequently across the globe. Although distances varied across genes, the median genetic distance between these subpopulations was small for both GST (0.0049 ± 0.0047) and D (0.0053 ± 0.0272), indicating little differentiation between the initial outbreak and its global derivatives in the pandemic (Fig. 4A). We then compared subpopulations sampled before and after each consecutive week. Similarly, genetic distances between subpopulations were small for both GST (0.0058 ± 0.0096) and D (0.0098 ± 0.0650) and tended to narrow over time rather than diverge (Fig. 4 B and C). Signatures of host adaptation can also be seen in the branching patterns of viral phylogenies. Bursts in transmissibility are emblematic of increases in relative viral fitness and are reflected in imbalances in the phylogeny, which can be estimated at each internal node (SI Appendix, Figs. S9 and S10) (33–35). We estimated phylogenetic η (36, 37) at each internal node of the SARS-CoV-2 phylogeny reconstructed from subsampled (10%) alignments and compared the distribution of estimates through time to phylogenies simulated under models of neutral and positive time-dependent rates (b(t) = beα(t)). Simulation analyses demonstrated that this metric was robust against sampling fraction (SI Appendix, Fig. S10). The distribution of η in the SARS-CoV-2 phylogenies adhered to expectations of the neutral model and deviated significantly (Student’s t test, P \u003c 0.001) from those of positive time-dependent rates for selection coefficients α ≥ 0.2 (Fig. 4 D and E). Together, the SARS-CoV-2 population and phylogenetic dynamics showed little evidence that the global spread of SARS-CoV-2 was related to viral fitness effects.\nFig. 4. Limited evidence of adaptation of the viral population. (A–C) Bootstrapped global estimates of Nei’s GST and Jost’s D for population differentiation for each structural gene. (A) Estimates of Nei’s GST (closed circles) and Jost’s D (open circles) comparing sequences sampled from the Hubei province to sequences subsequently sampled globally. Estimates of (B) Nei’s GST and (C) Jost’s D comparing sequences sampled before or after a specific date. Lines connect the median estimates across datasets for each gene. (D) Ln-transformed phylogenetic η, indicative of the number of iterative events in the sampled subtree, for subtrees from each internal node (after the root) of a down-sampled SARS-CoV-2 whole-genome phylogeny (dark gray), of a phylogeny simulated under neutral parameters (gold), and of a phylogeny simulated under positive time-dependent rates (b(t) = 0.01e0.4t, green). (E) Box plot of ln-transformed phylogenetic η estimates across all down-sampled SARS-CoV-2 whole-genome phylogenies, phylogenies simulated under neutral parameters, and phylogenies simulated under different positive time dependencies, α. Asterisks indicate significant differences in mean values (Student’s t test, P \u003c 0.05) between the SARS-CoV-2 and positive time-dependent phylogenies at each α."}
LitCovid-PD-CHEBI
{"project":"LitCovid-PD-CHEBI","denotations":[{"id":"T69","span":{"begin":1609,"end":1611},"obj":"Chemical"},{"id":"T70","span":{"begin":2027,"end":2029},"obj":"Chemical"},{"id":"T71","span":{"begin":2989,"end":2991},"obj":"Chemical"},{"id":"T72","span":{"begin":3259,"end":3263},"obj":"Chemical"}],"attributes":[{"id":"A69","pred":"chebi_id","subj":"T69","obj":"http://purl.obolibrary.org/obo/CHEBI_90326"},{"id":"A70","pred":"chebi_id","subj":"T70","obj":"http://purl.obolibrary.org/obo/CHEBI_90326"},{"id":"A71","pred":"chebi_id","subj":"T71","obj":"http://purl.obolibrary.org/obo/CHEBI_33319"},{"id":"A72","pred":"chebi_id","subj":"T72","obj":"http://purl.obolibrary.org/obo/CHEBI_29287"},{"id":"A73","pred":"chebi_id","subj":"T72","obj":"http://purl.obolibrary.org/obo/CHEBI_30050"}],"text":"No Evidence of Differentiation of the Viral Population.\nWhile there was only limited evidence of diversification at selected sites, we also assessed whether subpopulations among the globally circulating viral population had become genetically differentiated over time. To do so, we used two measures of population differentiation, the GST and D statistics, which characterize changes in allele frequency across populations and can show fitness differences between subpopulations (30–32). Genetic distances between two subpopulations can range between 0 and 1, indicating no and complete differentiation, respectively. We initially compared 30 genomes sampled from the initial outbreak in Wuhan, China, with subsampled alignments of the 18,484 genomes sampled subsequently across the globe. Although distances varied across genes, the median genetic distance between these subpopulations was small for both GST (0.0049 ± 0.0047) and D (0.0053 ± 0.0272), indicating little differentiation between the initial outbreak and its global derivatives in the pandemic (Fig. 4A). We then compared subpopulations sampled before and after each consecutive week. Similarly, genetic distances between subpopulations were small for both GST (0.0058 ± 0.0096) and D (0.0098 ± 0.0650) and tended to narrow over time rather than diverge (Fig. 4 B and C). Signatures of host adaptation can also be seen in the branching patterns of viral phylogenies. Bursts in transmissibility are emblematic of increases in relative viral fitness and are reflected in imbalances in the phylogeny, which can be estimated at each internal node (SI Appendix, Figs. S9 and S10) (33–35). We estimated phylogenetic η (36, 37) at each internal node of the SARS-CoV-2 phylogeny reconstructed from subsampled (10%) alignments and compared the distribution of estimates through time to phylogenies simulated under models of neutral and positive time-dependent rates (b(t) = beα(t)). Simulation analyses demonstrated that this metric was robust against sampling fraction (SI Appendix, Fig. S10). The distribution of η in the SARS-CoV-2 phylogenies adhered to expectations of the neutral model and deviated significantly (Student’s t test, P \u003c 0.001) from those of positive time-dependent rates for selection coefficients α ≥ 0.2 (Fig. 4 D and E). Together, the SARS-CoV-2 population and phylogenetic dynamics showed little evidence that the global spread of SARS-CoV-2 was related to viral fitness effects.\nFig. 4. Limited evidence of adaptation of the viral population. (A–C) Bootstrapped global estimates of Nei’s GST and Jost’s D for population differentiation for each structural gene. (A) Estimates of Nei’s GST (closed circles) and Jost’s D (open circles) comparing sequences sampled from the Hubei province to sequences subsequently sampled globally. Estimates of (B) Nei’s GST and (C) Jost’s D comparing sequences sampled before or after a specific date. Lines connect the median estimates across datasets for each gene. (D) Ln-transformed phylogenetic η, indicative of the number of iterative events in the sampled subtree, for subtrees from each internal node (after the root) of a down-sampled SARS-CoV-2 whole-genome phylogeny (dark gray), of a phylogeny simulated under neutral parameters (gold), and of a phylogeny simulated under positive time-dependent rates (b(t) = 0.01e0.4t, green). (E) Box plot of ln-transformed phylogenetic η estimates across all down-sampled SARS-CoV-2 whole-genome phylogenies, phylogenies simulated under neutral parameters, and phylogenies simulated under different positive time dependencies, α. Asterisks indicate significant differences in mean values (Student’s t test, P \u003c 0.05) between the SARS-CoV-2 and positive time-dependent phylogenies at each α."}
LitCovid-PubTator
{"project":"LitCovid-PubTator","denotations":[{"id":"305","span":{"begin":1715,"end":1725},"obj":"Species"},{"id":"306","span":{"begin":2080,"end":2090},"obj":"Species"},{"id":"307","span":{"begin":2316,"end":2326},"obj":"Species"},{"id":"308","span":{"begin":2413,"end":2423},"obj":"Species"},{"id":"309","span":{"begin":436,"end":443},"obj":"Disease"},{"id":"310","span":{"begin":1499,"end":1512},"obj":"Disease"},{"id":"311","span":{"begin":2439,"end":2452},"obj":"Disease"},{"id":"315","span":{"begin":3161,"end":3171},"obj":"Species"},{"id":"316","span":{"begin":3438,"end":3448},"obj":"Species"},{"id":"317","span":{"begin":3695,"end":3705},"obj":"Species"}],"attributes":[{"id":"A305","pred":"tao:has_database_id","subj":"305","obj":"Tax:2697049"},{"id":"A306","pred":"tao:has_database_id","subj":"306","obj":"Tax:2697049"},{"id":"A307","pred":"tao:has_database_id","subj":"307","obj":"Tax:2697049"},{"id":"A308","pred":"tao:has_database_id","subj":"308","obj":"Tax:2697049"},{"id":"A309","pred":"tao:has_database_id","subj":"309","obj":"MESH:D012640"},{"id":"A315","pred":"tao:has_database_id","subj":"315","obj":"Tax:2697049"},{"id":"A316","pred":"tao:has_database_id","subj":"316","obj":"Tax:2697049"},{"id":"A317","pred":"tao:has_database_id","subj":"317","obj":"Tax:2697049"}],"namespaces":[{"prefix":"Tax","uri":"https://www.ncbi.nlm.nih.gov/taxonomy/"},{"prefix":"MESH","uri":"https://id.nlm.nih.gov/mesh/"},{"prefix":"Gene","uri":"https://www.ncbi.nlm.nih.gov/gene/"},{"prefix":"CVCL","uri":"https://web.expasy.org/cellosaurus/CVCL_"}],"text":"No Evidence of Differentiation of the Viral Population.\nWhile there was only limited evidence of diversification at selected sites, we also assessed whether subpopulations among the globally circulating viral population had become genetically differentiated over time. To do so, we used two measures of population differentiation, the GST and D statistics, which characterize changes in allele frequency across populations and can show fitness differences between subpopulations (30–32). Genetic distances between two subpopulations can range between 0 and 1, indicating no and complete differentiation, respectively. We initially compared 30 genomes sampled from the initial outbreak in Wuhan, China, with subsampled alignments of the 18,484 genomes sampled subsequently across the globe. Although distances varied across genes, the median genetic distance between these subpopulations was small for both GST (0.0049 ± 0.0047) and D (0.0053 ± 0.0272), indicating little differentiation between the initial outbreak and its global derivatives in the pandemic (Fig. 4A). We then compared subpopulations sampled before and after each consecutive week. Similarly, genetic distances between subpopulations were small for both GST (0.0058 ± 0.0096) and D (0.0098 ± 0.0650) and tended to narrow over time rather than diverge (Fig. 4 B and C). Signatures of host adaptation can also be seen in the branching patterns of viral phylogenies. Bursts in transmissibility are emblematic of increases in relative viral fitness and are reflected in imbalances in the phylogeny, which can be estimated at each internal node (SI Appendix, Figs. S9 and S10) (33–35). We estimated phylogenetic η (36, 37) at each internal node of the SARS-CoV-2 phylogeny reconstructed from subsampled (10%) alignments and compared the distribution of estimates through time to phylogenies simulated under models of neutral and positive time-dependent rates (b(t) = beα(t)). Simulation analyses demonstrated that this metric was robust against sampling fraction (SI Appendix, Fig. S10). The distribution of η in the SARS-CoV-2 phylogenies adhered to expectations of the neutral model and deviated significantly (Student’s t test, P \u003c 0.001) from those of positive time-dependent rates for selection coefficients α ≥ 0.2 (Fig. 4 D and E). Together, the SARS-CoV-2 population and phylogenetic dynamics showed little evidence that the global spread of SARS-CoV-2 was related to viral fitness effects.\nFig. 4. Limited evidence of adaptation of the viral population. (A–C) Bootstrapped global estimates of Nei’s GST and Jost’s D for population differentiation for each structural gene. (A) Estimates of Nei’s GST (closed circles) and Jost’s D (open circles) comparing sequences sampled from the Hubei province to sequences subsequently sampled globally. Estimates of (B) Nei’s GST and (C) Jost’s D comparing sequences sampled before or after a specific date. Lines connect the median estimates across datasets for each gene. (D) Ln-transformed phylogenetic η, indicative of the number of iterative events in the sampled subtree, for subtrees from each internal node (after the root) of a down-sampled SARS-CoV-2 whole-genome phylogeny (dark gray), of a phylogeny simulated under neutral parameters (gold), and of a phylogeny simulated under positive time-dependent rates (b(t) = 0.01e0.4t, green). (E) Box plot of ln-transformed phylogenetic η estimates across all down-sampled SARS-CoV-2 whole-genome phylogenies, phylogenies simulated under neutral parameters, and phylogenies simulated under different positive time dependencies, α. Asterisks indicate significant differences in mean values (Student’s t test, P \u003c 0.05) between the SARS-CoV-2 and positive time-dependent phylogenies at each α."}
LitCovid-sentences
{"project":"LitCovid-sentences","denotations":[{"id":"T125","span":{"begin":0,"end":55},"obj":"Sentence"},{"id":"T126","span":{"begin":56,"end":268},"obj":"Sentence"},{"id":"T127","span":{"begin":269,"end":487},"obj":"Sentence"},{"id":"T128","span":{"begin":488,"end":617},"obj":"Sentence"},{"id":"T129","span":{"begin":618,"end":789},"obj":"Sentence"},{"id":"T130","span":{"begin":790,"end":1069},"obj":"Sentence"},{"id":"T131","span":{"begin":1070,"end":1149},"obj":"Sentence"},{"id":"T132","span":{"begin":1150,"end":1336},"obj":"Sentence"},{"id":"T133","span":{"begin":1337,"end":1431},"obj":"Sentence"},{"id":"T134","span":{"begin":1432,"end":1627},"obj":"Sentence"},{"id":"T135","span":{"begin":1628,"end":1648},"obj":"Sentence"},{"id":"T136","span":{"begin":1649,"end":1938},"obj":"Sentence"},{"id":"T137","span":{"begin":1939,"end":2050},"obj":"Sentence"},{"id":"T138","span":{"begin":2051,"end":2301},"obj":"Sentence"},{"id":"T139","span":{"begin":2302,"end":2461},"obj":"Sentence"},{"id":"T140","span":{"begin":2462,"end":2469},"obj":"Sentence"},{"id":"T141","span":{"begin":2471,"end":2813},"obj":"Sentence"},{"id":"T142","span":{"begin":2814,"end":2918},"obj":"Sentence"},{"id":"T143","span":{"begin":2919,"end":3595},"obj":"Sentence"},{"id":"T144","span":{"begin":3596,"end":3756},"obj":"Sentence"}],"namespaces":[{"prefix":"_base","uri":"http://pubannotation.org/ontology/tao.owl#"}],"text":"No Evidence of Differentiation of the Viral Population.\nWhile there was only limited evidence of diversification at selected sites, we also assessed whether subpopulations among the globally circulating viral population had become genetically differentiated over time. To do so, we used two measures of population differentiation, the GST and D statistics, which characterize changes in allele frequency across populations and can show fitness differences between subpopulations (30–32). Genetic distances between two subpopulations can range between 0 and 1, indicating no and complete differentiation, respectively. We initially compared 30 genomes sampled from the initial outbreak in Wuhan, China, with subsampled alignments of the 18,484 genomes sampled subsequently across the globe. Although distances varied across genes, the median genetic distance between these subpopulations was small for both GST (0.0049 ± 0.0047) and D (0.0053 ± 0.0272), indicating little differentiation between the initial outbreak and its global derivatives in the pandemic (Fig. 4A). We then compared subpopulations sampled before and after each consecutive week. Similarly, genetic distances between subpopulations were small for both GST (0.0058 ± 0.0096) and D (0.0098 ± 0.0650) and tended to narrow over time rather than diverge (Fig. 4 B and C). Signatures of host adaptation can also be seen in the branching patterns of viral phylogenies. Bursts in transmissibility are emblematic of increases in relative viral fitness and are reflected in imbalances in the phylogeny, which can be estimated at each internal node (SI Appendix, Figs. S9 and S10) (33–35). We estimated phylogenetic η (36, 37) at each internal node of the SARS-CoV-2 phylogeny reconstructed from subsampled (10%) alignments and compared the distribution of estimates through time to phylogenies simulated under models of neutral and positive time-dependent rates (b(t) = beα(t)). Simulation analyses demonstrated that this metric was robust against sampling fraction (SI Appendix, Fig. S10). The distribution of η in the SARS-CoV-2 phylogenies adhered to expectations of the neutral model and deviated significantly (Student’s t test, P \u003c 0.001) from those of positive time-dependent rates for selection coefficients α ≥ 0.2 (Fig. 4 D and E). Together, the SARS-CoV-2 population and phylogenetic dynamics showed little evidence that the global spread of SARS-CoV-2 was related to viral fitness effects.\nFig. 4. Limited evidence of adaptation of the viral population. (A–C) Bootstrapped global estimates of Nei’s GST and Jost’s D for population differentiation for each structural gene. (A) Estimates of Nei’s GST (closed circles) and Jost’s D (open circles) comparing sequences sampled from the Hubei province to sequences subsequently sampled globally. Estimates of (B) Nei’s GST and (C) Jost’s D comparing sequences sampled before or after a specific date. Lines connect the median estimates across datasets for each gene. (D) Ln-transformed phylogenetic η, indicative of the number of iterative events in the sampled subtree, for subtrees from each internal node (after the root) of a down-sampled SARS-CoV-2 whole-genome phylogeny (dark gray), of a phylogeny simulated under neutral parameters (gold), and of a phylogeny simulated under positive time-dependent rates (b(t) = 0.01e0.4t, green). (E) Box plot of ln-transformed phylogenetic η estimates across all down-sampled SARS-CoV-2 whole-genome phylogenies, phylogenies simulated under neutral parameters, and phylogenies simulated under different positive time dependencies, α. Asterisks indicate significant differences in mean values (Student’s t test, P \u003c 0.05) between the SARS-CoV-2 and positive time-dependent phylogenies at each α."}
2_test
{"project":"2_test","denotations":[{"id":"32868447-4519626-132542397","span":{"begin":480,"end":482},"obj":"4519626"},{"id":"32868447-19238703-132542397","span":{"begin":480,"end":482},"obj":"19238703"},{"id":"32868447-22883857-132542397","span":{"begin":480,"end":482},"obj":"22883857"},{"id":"32868447-26147205-132542398","span":{"begin":1641,"end":1643},"obj":"26147205"},{"id":"32868447-28472435-132542398","span":{"begin":1641,"end":1643},"obj":"28472435"},{"id":"32868447-26658901-132542399","span":{"begin":1678,"end":1680},"obj":"26658901"},{"id":"32868447-31700680-132542400","span":{"begin":1682,"end":1684},"obj":"31700680"}],"text":"No Evidence of Differentiation of the Viral Population.\nWhile there was only limited evidence of diversification at selected sites, we also assessed whether subpopulations among the globally circulating viral population had become genetically differentiated over time. To do so, we used two measures of population differentiation, the GST and D statistics, which characterize changes in allele frequency across populations and can show fitness differences between subpopulations (30–32). Genetic distances between two subpopulations can range between 0 and 1, indicating no and complete differentiation, respectively. We initially compared 30 genomes sampled from the initial outbreak in Wuhan, China, with subsampled alignments of the 18,484 genomes sampled subsequently across the globe. Although distances varied across genes, the median genetic distance between these subpopulations was small for both GST (0.0049 ± 0.0047) and D (0.0053 ± 0.0272), indicating little differentiation between the initial outbreak and its global derivatives in the pandemic (Fig. 4A). We then compared subpopulations sampled before and after each consecutive week. Similarly, genetic distances between subpopulations were small for both GST (0.0058 ± 0.0096) and D (0.0098 ± 0.0650) and tended to narrow over time rather than diverge (Fig. 4 B and C). Signatures of host adaptation can also be seen in the branching patterns of viral phylogenies. Bursts in transmissibility are emblematic of increases in relative viral fitness and are reflected in imbalances in the phylogeny, which can be estimated at each internal node (SI Appendix, Figs. S9 and S10) (33–35). We estimated phylogenetic η (36, 37) at each internal node of the SARS-CoV-2 phylogeny reconstructed from subsampled (10%) alignments and compared the distribution of estimates through time to phylogenies simulated under models of neutral and positive time-dependent rates (b(t) = beα(t)). Simulation analyses demonstrated that this metric was robust against sampling fraction (SI Appendix, Fig. S10). The distribution of η in the SARS-CoV-2 phylogenies adhered to expectations of the neutral model and deviated significantly (Student’s t test, P \u003c 0.001) from those of positive time-dependent rates for selection coefficients α ≥ 0.2 (Fig. 4 D and E). Together, the SARS-CoV-2 population and phylogenetic dynamics showed little evidence that the global spread of SARS-CoV-2 was related to viral fitness effects.\nFig. 4. Limited evidence of adaptation of the viral population. (A–C) Bootstrapped global estimates of Nei’s GST and Jost’s D for population differentiation for each structural gene. (A) Estimates of Nei’s GST (closed circles) and Jost’s D (open circles) comparing sequences sampled from the Hubei province to sequences subsequently sampled globally. Estimates of (B) Nei’s GST and (C) Jost’s D comparing sequences sampled before or after a specific date. Lines connect the median estimates across datasets for each gene. (D) Ln-transformed phylogenetic η, indicative of the number of iterative events in the sampled subtree, for subtrees from each internal node (after the root) of a down-sampled SARS-CoV-2 whole-genome phylogeny (dark gray), of a phylogeny simulated under neutral parameters (gold), and of a phylogeny simulated under positive time-dependent rates (b(t) = 0.01e0.4t, green). (E) Box plot of ln-transformed phylogenetic η estimates across all down-sampled SARS-CoV-2 whole-genome phylogenies, phylogenies simulated under neutral parameters, and phylogenies simulated under different positive time dependencies, α. Asterisks indicate significant differences in mean values (Student’s t test, P \u003c 0.05) between the SARS-CoV-2 and positive time-dependent phylogenies at each α."}