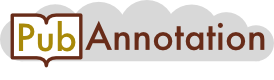
PMC:7497282 / 100360-101884
Annnotations
MyTest
{"project":"MyTest","denotations":[{"id":"32741232-20619075-29927541","span":{"begin":1252,"end":1254},"obj":"20619075"},{"id":"32741232-25316864-29927542","span":{"begin":1256,"end":1258},"obj":"25316864"},{"id":"32741232-23521018-29927543","span":{"begin":1515,"end":1517},"obj":"23521018"}],"namespaces":[{"prefix":"_base","uri":"https://www.uniprot.org/uniprot/testbase"},{"prefix":"UniProtKB","uri":"https://www.uniprot.org/uniprot/"},{"prefix":"uniprot","uri":"https://www.uniprot.org/uniprotkb/"}],"text":"6.2. Different assumptions for modeling populations and mixing\nAs shown in Tables 2 and 3, the models reviewed differed with respect to the populations modeled and the mixing assumptions used. DEB and SC models typically assume homogeneous mixing of individuals in a population, although they may account for preferential mixing by age, subpopulation, or other factors, and include births, deaths, aging, and immigration. Part of the complexity of KRI transmission models comes from the use of population-specific demographic and immunization history data for inputs and the inclusion of preferential mixing by age and/or subpopulation. The inclusion of undervaccinated subpopulations in DEB models probably only partially captures some of the population heterogeneity in under-vaccinated communities, but does so better than ignoring this heterogeneity for some analytical questions.\nIB models seek to capture the full richness of the complexity of transmission, but they do so with considerable computational costs. IB models track each individual in a population, which can offer advantages that include simulating die-out directly, but require many assumptions about the spatial distribution and contact patterns for each individual in the model [24, 41].\nOne limitation of transmission models broadly arises from assumptions about mixing at the model boundaries. Most models characterize transmission within a closed population, but they can allow for importations and exportations of viruses as appropriate [33, 202]."}
2_test
{"project":"2_test","denotations":[{"id":"32741232-20619075-29927541","span":{"begin":1252,"end":1254},"obj":"20619075"},{"id":"32741232-25316864-29927542","span":{"begin":1256,"end":1258},"obj":"25316864"},{"id":"32741232-23521018-29927543","span":{"begin":1515,"end":1517},"obj":"23521018"}],"text":"6.2. Different assumptions for modeling populations and mixing\nAs shown in Tables 2 and 3, the models reviewed differed with respect to the populations modeled and the mixing assumptions used. DEB and SC models typically assume homogeneous mixing of individuals in a population, although they may account for preferential mixing by age, subpopulation, or other factors, and include births, deaths, aging, and immigration. Part of the complexity of KRI transmission models comes from the use of population-specific demographic and immunization history data for inputs and the inclusion of preferential mixing by age and/or subpopulation. The inclusion of undervaccinated subpopulations in DEB models probably only partially captures some of the population heterogeneity in under-vaccinated communities, but does so better than ignoring this heterogeneity for some analytical questions.\nIB models seek to capture the full richness of the complexity of transmission, but they do so with considerable computational costs. IB models track each individual in a population, which can offer advantages that include simulating die-out directly, but require many assumptions about the spatial distribution and contact patterns for each individual in the model [24, 41].\nOne limitation of transmission models broadly arises from assumptions about mixing at the model boundaries. Most models characterize transmission within a closed population, but they can allow for importations and exportations of viruses as appropriate [33, 202]."}