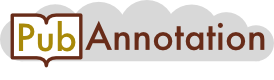
PMC:7417788 / 75331-78047
Annnotations
LitCovid-PMC-OGER-BB
{"project":"LitCovid-PMC-OGER-BB","denotations":[{"id":"T1513","span":{"begin":61,"end":66},"obj":"SO:0000150"},{"id":"T1514","span":{"begin":111,"end":117},"obj":"SO:0001505"},{"id":"T1515","span":{"begin":429,"end":435},"obj":"SO:0001026"},{"id":"T1516","span":{"begin":490,"end":492},"obj":"SO:0000028"},{"id":"T1517","span":{"begin":613,"end":615},"obj":"SO:0000028"},{"id":"T1518","span":{"begin":648,"end":650},"obj":"SO:0000028"},{"id":"T1519","span":{"begin":1176,"end":1181},"obj":"SO:0000150"},{"id":"T1520","span":{"begin":1358,"end":1365},"obj":"SO:0001026"},{"id":"T1521","span":{"begin":1549,"end":1551},"obj":"SO:0000028"},{"id":"T1522","span":{"begin":1778,"end":1780},"obj":"SO:0000028"},{"id":"T1523","span":{"begin":2553,"end":2558},"obj":"SP_6;NCBITaxon:9606"}],"text":"scATAC-seq data processing\n\nscATAC-seq processing\nscATAC-seq reads were aligned to the GRCh38 (hg38) reference genome and quantified using CellRanger-ATAC count (10x Genomics, v.1.0.0).\n\nscATAC-seq quality control\nTo ensure that each cell was both adequately sequenced and had a high signal-to-background ratio, we filtered cells with less than 1,000 unique fragments and enrichment at TSSs \u003c 8. To calculate TSS enrichment \u003e 2, genome-wide Tn5-corrected insertions were aggregated ± 2,000 bp relative (TSS-strand-corrected) to each unique TSS. This profile was normalized to the mean accessibility ± 1,900–2,000 bp from the TSS, smoothed every 51 bp and the maximum smoothed value was reported as TSS enrichment in R. To construct a counts matrix for each cell by each feature (peaks), we read each fragment.tsv.gz fill into a GenomicRanges object. For each Tn5 insertion, which can be thought of as the “start” and “end” of the ATAC fragments, we used findOverlaps to find all overlaps with the feature by insertions. Then we added a column with the unique id (integer) cell barcode to the overlaps object and fed this into a sparseMatrix in R. To calculate the fraction of reads/insertions in peaks, we used the colSums of the sparseMatrix and divided it by the number of insertions for each cell id barcode using table in R.\n\nscATAC-seq visualization in genomic regions\nTo visualize scATAC-seq data, we read the fragments into a GenomicRanges object in R. We then computed sliding windows across each region we wanted to visualize for every 100 bp “slidingWindows (region, 100, 100)”. We computed a counts matrix for Tn5-corrected insertions as described above and then binarized this matrix. We then returned all non-zero indices (binarization) from the matrix (cell × 100-bp intervals) and plotted them in ggplot2 in R with “geom_tile”. For visualizing aggregate scATAC-seq data, the binarized matrix above was summed and normalized. Scale factors were computed by taking the binarized sum in the global peak set and normalizing to 10,000,000. Tracks were then plotted in Loupe Cell Browser, an interactive visualization software that shows scATAC-seq peak profiles for scATAC-seq cell clusters, similar to the analysis done in this manuscript and described at https://support.10xgenomics.com/single-cellatac/software/visualization/latest/what-is-loupe-cell-browser.\n\nchromVAR\nWe measured global TF activity using chromVAR15. We used the cell-by-peaks and the Catalog of Inferred Sequence Binding Preferences (CIS-BP) motif (from chromVAR motifs “human_pwms_v1”) matches within these peaks from motifmatchr. We then computed the GC-bias-corrected deviation scores using the chromVAR “deviationScores” function."}
LitCovid-PD-FMA-UBERON
{"project":"LitCovid-PD-FMA-UBERON","denotations":[{"id":"T553","span":{"begin":111,"end":117},"obj":"Body_part"},{"id":"T554","span":{"begin":234,"end":238},"obj":"Body_part"},{"id":"T555","span":{"begin":324,"end":329},"obj":"Body_part"},{"id":"T556","span":{"begin":429,"end":435},"obj":"Body_part"},{"id":"T557","span":{"begin":757,"end":761},"obj":"Body_part"},{"id":"T558","span":{"begin":1072,"end":1076},"obj":"Body_part"},{"id":"T559","span":{"begin":1295,"end":1299},"obj":"Body_part"},{"id":"T560","span":{"begin":1767,"end":1771},"obj":"Body_part"},{"id":"T561","span":{"begin":2084,"end":2088},"obj":"Body_part"},{"id":"T562","span":{"begin":2187,"end":2200},"obj":"Body_part"},{"id":"T563","span":{"begin":2187,"end":2191},"obj":"Body_part"},{"id":"T564","span":{"begin":2359,"end":2363},"obj":"Body_part"},{"id":"T565","span":{"begin":2444,"end":2448},"obj":"Body_part"}],"attributes":[{"id":"A553","pred":"fma_id","subj":"T553","obj":"http://purl.org/sig/ont/fma/fma84116"},{"id":"A554","pred":"fma_id","subj":"T554","obj":"http://purl.org/sig/ont/fma/fma68646"},{"id":"A555","pred":"fma_id","subj":"T555","obj":"http://purl.org/sig/ont/fma/fma68646"},{"id":"A556","pred":"fma_id","subj":"T556","obj":"http://purl.org/sig/ont/fma/fma84116"},{"id":"A557","pred":"fma_id","subj":"T557","obj":"http://purl.org/sig/ont/fma/fma68646"},{"id":"A558","pred":"fma_id","subj":"T558","obj":"http://purl.org/sig/ont/fma/fma68646"},{"id":"A559","pred":"fma_id","subj":"T559","obj":"http://purl.org/sig/ont/fma/fma68646"},{"id":"A560","pred":"fma_id","subj":"T560","obj":"http://purl.org/sig/ont/fma/fma68646"},{"id":"A561","pred":"fma_id","subj":"T561","obj":"http://purl.org/sig/ont/fma/fma68646"},{"id":"A562","pred":"fma_id","subj":"T562","obj":"http://purl.org/sig/ont/fma/fma62807"},{"id":"A563","pred":"fma_id","subj":"T563","obj":"http://purl.org/sig/ont/fma/fma68646"},{"id":"A564","pred":"fma_id","subj":"T564","obj":"http://purl.org/sig/ont/fma/fma68646"},{"id":"A565","pred":"fma_id","subj":"T565","obj":"http://purl.org/sig/ont/fma/fma68646"}],"text":"scATAC-seq data processing\n\nscATAC-seq processing\nscATAC-seq reads were aligned to the GRCh38 (hg38) reference genome and quantified using CellRanger-ATAC count (10x Genomics, v.1.0.0).\n\nscATAC-seq quality control\nTo ensure that each cell was both adequately sequenced and had a high signal-to-background ratio, we filtered cells with less than 1,000 unique fragments and enrichment at TSSs \u003c 8. To calculate TSS enrichment \u003e 2, genome-wide Tn5-corrected insertions were aggregated ± 2,000 bp relative (TSS-strand-corrected) to each unique TSS. This profile was normalized to the mean accessibility ± 1,900–2,000 bp from the TSS, smoothed every 51 bp and the maximum smoothed value was reported as TSS enrichment in R. To construct a counts matrix for each cell by each feature (peaks), we read each fragment.tsv.gz fill into a GenomicRanges object. For each Tn5 insertion, which can be thought of as the “start” and “end” of the ATAC fragments, we used findOverlaps to find all overlaps with the feature by insertions. Then we added a column with the unique id (integer) cell barcode to the overlaps object and fed this into a sparseMatrix in R. To calculate the fraction of reads/insertions in peaks, we used the colSums of the sparseMatrix and divided it by the number of insertions for each cell id barcode using table in R.\n\nscATAC-seq visualization in genomic regions\nTo visualize scATAC-seq data, we read the fragments into a GenomicRanges object in R. We then computed sliding windows across each region we wanted to visualize for every 100 bp “slidingWindows (region, 100, 100)”. We computed a counts matrix for Tn5-corrected insertions as described above and then binarized this matrix. We then returned all non-zero indices (binarization) from the matrix (cell × 100-bp intervals) and plotted them in ggplot2 in R with “geom_tile”. For visualizing aggregate scATAC-seq data, the binarized matrix above was summed and normalized. Scale factors were computed by taking the binarized sum in the global peak set and normalizing to 10,000,000. Tracks were then plotted in Loupe Cell Browser, an interactive visualization software that shows scATAC-seq peak profiles for scATAC-seq cell clusters, similar to the analysis done in this manuscript and described at https://support.10xgenomics.com/single-cellatac/software/visualization/latest/what-is-loupe-cell-browser.\n\nchromVAR\nWe measured global TF activity using chromVAR15. We used the cell-by-peaks and the Catalog of Inferred Sequence Binding Preferences (CIS-BP) motif (from chromVAR motifs “human_pwms_v1”) matches within these peaks from motifmatchr. We then computed the GC-bias-corrected deviation scores using the chromVAR “deviationScores” function."}
LitCovid-PD-UBERON
{"project":"LitCovid-PD-UBERON","denotations":[{"id":"T42","span":{"begin":1940,"end":1945},"obj":"Body_part"},{"id":"T43","span":{"begin":2187,"end":2200},"obj":"Body_part"}],"attributes":[{"id":"A42","pred":"uberon_id","subj":"T42","obj":"http://purl.obolibrary.org/obo/UBERON_0002542"},{"id":"A43","pred":"uberon_id","subj":"T43","obj":"http://purl.obolibrary.org/obo/UBERON_0034922"}],"text":"scATAC-seq data processing\n\nscATAC-seq processing\nscATAC-seq reads were aligned to the GRCh38 (hg38) reference genome and quantified using CellRanger-ATAC count (10x Genomics, v.1.0.0).\n\nscATAC-seq quality control\nTo ensure that each cell was both adequately sequenced and had a high signal-to-background ratio, we filtered cells with less than 1,000 unique fragments and enrichment at TSSs \u003c 8. To calculate TSS enrichment \u003e 2, genome-wide Tn5-corrected insertions were aggregated ± 2,000 bp relative (TSS-strand-corrected) to each unique TSS. This profile was normalized to the mean accessibility ± 1,900–2,000 bp from the TSS, smoothed every 51 bp and the maximum smoothed value was reported as TSS enrichment in R. To construct a counts matrix for each cell by each feature (peaks), we read each fragment.tsv.gz fill into a GenomicRanges object. For each Tn5 insertion, which can be thought of as the “start” and “end” of the ATAC fragments, we used findOverlaps to find all overlaps with the feature by insertions. Then we added a column with the unique id (integer) cell barcode to the overlaps object and fed this into a sparseMatrix in R. To calculate the fraction of reads/insertions in peaks, we used the colSums of the sparseMatrix and divided it by the number of insertions for each cell id barcode using table in R.\n\nscATAC-seq visualization in genomic regions\nTo visualize scATAC-seq data, we read the fragments into a GenomicRanges object in R. We then computed sliding windows across each region we wanted to visualize for every 100 bp “slidingWindows (region, 100, 100)”. We computed a counts matrix for Tn5-corrected insertions as described above and then binarized this matrix. We then returned all non-zero indices (binarization) from the matrix (cell × 100-bp intervals) and plotted them in ggplot2 in R with “geom_tile”. For visualizing aggregate scATAC-seq data, the binarized matrix above was summed and normalized. Scale factors were computed by taking the binarized sum in the global peak set and normalizing to 10,000,000. Tracks were then plotted in Loupe Cell Browser, an interactive visualization software that shows scATAC-seq peak profiles for scATAC-seq cell clusters, similar to the analysis done in this manuscript and described at https://support.10xgenomics.com/single-cellatac/software/visualization/latest/what-is-loupe-cell-browser.\n\nchromVAR\nWe measured global TF activity using chromVAR15. We used the cell-by-peaks and the Catalog of Inferred Sequence Binding Preferences (CIS-BP) motif (from chromVAR motifs “human_pwms_v1”) matches within these peaks from motifmatchr. We then computed the GC-bias-corrected deviation scores using the chromVAR “deviationScores” function."}
LitCovid-PD-MONDO
{"project":"LitCovid-PD-MONDO","denotations":[{"id":"T142","span":{"begin":409,"end":412},"obj":"Disease"},{"id":"T143","span":{"begin":503,"end":506},"obj":"Disease"},{"id":"T144","span":{"begin":540,"end":543},"obj":"Disease"},{"id":"T145","span":{"begin":625,"end":628},"obj":"Disease"},{"id":"T146","span":{"begin":698,"end":701},"obj":"Disease"},{"id":"T147","span":{"begin":2516,"end":2519},"obj":"Disease"}],"attributes":[{"id":"A142","pred":"mondo_id","subj":"T142","obj":"http://purl.obolibrary.org/obo/MONDO_0001881"},{"id":"A143","pred":"mondo_id","subj":"T143","obj":"http://purl.obolibrary.org/obo/MONDO_0001881"},{"id":"A144","pred":"mondo_id","subj":"T144","obj":"http://purl.obolibrary.org/obo/MONDO_0001881"},{"id":"A145","pred":"mondo_id","subj":"T145","obj":"http://purl.obolibrary.org/obo/MONDO_0001881"},{"id":"A146","pred":"mondo_id","subj":"T146","obj":"http://purl.obolibrary.org/obo/MONDO_0001881"},{"id":"A147","pred":"mondo_id","subj":"T147","obj":"http://purl.obolibrary.org/obo/MONDO_0004647"}],"text":"scATAC-seq data processing\n\nscATAC-seq processing\nscATAC-seq reads were aligned to the GRCh38 (hg38) reference genome and quantified using CellRanger-ATAC count (10x Genomics, v.1.0.0).\n\nscATAC-seq quality control\nTo ensure that each cell was both adequately sequenced and had a high signal-to-background ratio, we filtered cells with less than 1,000 unique fragments and enrichment at TSSs \u003c 8. To calculate TSS enrichment \u003e 2, genome-wide Tn5-corrected insertions were aggregated ± 2,000 bp relative (TSS-strand-corrected) to each unique TSS. This profile was normalized to the mean accessibility ± 1,900–2,000 bp from the TSS, smoothed every 51 bp and the maximum smoothed value was reported as TSS enrichment in R. To construct a counts matrix for each cell by each feature (peaks), we read each fragment.tsv.gz fill into a GenomicRanges object. For each Tn5 insertion, which can be thought of as the “start” and “end” of the ATAC fragments, we used findOverlaps to find all overlaps with the feature by insertions. Then we added a column with the unique id (integer) cell barcode to the overlaps object and fed this into a sparseMatrix in R. To calculate the fraction of reads/insertions in peaks, we used the colSums of the sparseMatrix and divided it by the number of insertions for each cell id barcode using table in R.\n\nscATAC-seq visualization in genomic regions\nTo visualize scATAC-seq data, we read the fragments into a GenomicRanges object in R. We then computed sliding windows across each region we wanted to visualize for every 100 bp “slidingWindows (region, 100, 100)”. We computed a counts matrix for Tn5-corrected insertions as described above and then binarized this matrix. We then returned all non-zero indices (binarization) from the matrix (cell × 100-bp intervals) and plotted them in ggplot2 in R with “geom_tile”. For visualizing aggregate scATAC-seq data, the binarized matrix above was summed and normalized. Scale factors were computed by taking the binarized sum in the global peak set and normalizing to 10,000,000. Tracks were then plotted in Loupe Cell Browser, an interactive visualization software that shows scATAC-seq peak profiles for scATAC-seq cell clusters, similar to the analysis done in this manuscript and described at https://support.10xgenomics.com/single-cellatac/software/visualization/latest/what-is-loupe-cell-browser.\n\nchromVAR\nWe measured global TF activity using chromVAR15. We used the cell-by-peaks and the Catalog of Inferred Sequence Binding Preferences (CIS-BP) motif (from chromVAR motifs “human_pwms_v1”) matches within these peaks from motifmatchr. We then computed the GC-bias-corrected deviation scores using the chromVAR “deviationScores” function."}
LitCovid-PD-CLO
{"project":"LitCovid-PD-CLO","denotations":[{"id":"T1210","span":{"begin":234,"end":238},"obj":"http://purl.obolibrary.org/obo/GO_0005623"},{"id":"T1211","span":{"begin":277,"end":278},"obj":"http://purl.obolibrary.org/obo/CLO_0001020"},{"id":"T1212","span":{"begin":284,"end":290},"obj":"http://purl.obolibrary.org/obo/SO_0000418"},{"id":"T1213","span":{"begin":324,"end":329},"obj":"http://purl.obolibrary.org/obo/GO_0005623"},{"id":"T1214","span":{"begin":732,"end":733},"obj":"http://purl.obolibrary.org/obo/CLO_0001020"},{"id":"T1215","span":{"begin":757,"end":761},"obj":"http://purl.obolibrary.org/obo/GO_0005623"},{"id":"T1216","span":{"begin":826,"end":827},"obj":"http://purl.obolibrary.org/obo/CLO_0001020"},{"id":"T1217","span":{"begin":842,"end":848},"obj":"http://purl.obolibrary.org/obo/BFO_0000030"},{"id":"T1218","span":{"begin":1034,"end":1035},"obj":"http://purl.obolibrary.org/obo/CLO_0001020"},{"id":"T1219","span":{"begin":1072,"end":1076},"obj":"http://purl.obolibrary.org/obo/GO_0005623"},{"id":"T1220","span":{"begin":1101,"end":1107},"obj":"http://purl.obolibrary.org/obo/BFO_0000030"},{"id":"T1221","span":{"begin":1126,"end":1127},"obj":"http://purl.obolibrary.org/obo/CLO_0001020"},{"id":"T1222","span":{"begin":1295,"end":1299},"obj":"http://purl.obolibrary.org/obo/GO_0005623"},{"id":"T1223","span":{"begin":1431,"end":1432},"obj":"http://purl.obolibrary.org/obo/CLO_0001020"},{"id":"T1224","span":{"begin":1447,"end":1453},"obj":"http://purl.obolibrary.org/obo/BFO_0000030"},{"id":"T1225","span":{"begin":1601,"end":1602},"obj":"http://purl.obolibrary.org/obo/CLO_0001020"},{"id":"T1226","span":{"begin":1767,"end":1771},"obj":"http://purl.obolibrary.org/obo/GO_0005623"},{"id":"T1227","span":{"begin":2084,"end":2088},"obj":"http://purl.obolibrary.org/obo/GO_0005623"},{"id":"T1228","span":{"begin":2187,"end":2200},"obj":"http://purl.obolibrary.org/obo/UBERON_0034922"},{"id":"T1229","span":{"begin":2359,"end":2363},"obj":"http://purl.obolibrary.org/obo/GO_0005623"},{"id":"T1230","span":{"begin":2405,"end":2413},"obj":"http://purl.obolibrary.org/obo/CLO_0001658"},{"id":"T1231","span":{"begin":2444,"end":2448},"obj":"http://purl.obolibrary.org/obo/GO_0005623"}],"text":"scATAC-seq data processing\n\nscATAC-seq processing\nscATAC-seq reads were aligned to the GRCh38 (hg38) reference genome and quantified using CellRanger-ATAC count (10x Genomics, v.1.0.0).\n\nscATAC-seq quality control\nTo ensure that each cell was both adequately sequenced and had a high signal-to-background ratio, we filtered cells with less than 1,000 unique fragments and enrichment at TSSs \u003c 8. To calculate TSS enrichment \u003e 2, genome-wide Tn5-corrected insertions were aggregated ± 2,000 bp relative (TSS-strand-corrected) to each unique TSS. This profile was normalized to the mean accessibility ± 1,900–2,000 bp from the TSS, smoothed every 51 bp and the maximum smoothed value was reported as TSS enrichment in R. To construct a counts matrix for each cell by each feature (peaks), we read each fragment.tsv.gz fill into a GenomicRanges object. For each Tn5 insertion, which can be thought of as the “start” and “end” of the ATAC fragments, we used findOverlaps to find all overlaps with the feature by insertions. Then we added a column with the unique id (integer) cell barcode to the overlaps object and fed this into a sparseMatrix in R. To calculate the fraction of reads/insertions in peaks, we used the colSums of the sparseMatrix and divided it by the number of insertions for each cell id barcode using table in R.\n\nscATAC-seq visualization in genomic regions\nTo visualize scATAC-seq data, we read the fragments into a GenomicRanges object in R. We then computed sliding windows across each region we wanted to visualize for every 100 bp “slidingWindows (region, 100, 100)”. We computed a counts matrix for Tn5-corrected insertions as described above and then binarized this matrix. We then returned all non-zero indices (binarization) from the matrix (cell × 100-bp intervals) and plotted them in ggplot2 in R with “geom_tile”. For visualizing aggregate scATAC-seq data, the binarized matrix above was summed and normalized. Scale factors were computed by taking the binarized sum in the global peak set and normalizing to 10,000,000. Tracks were then plotted in Loupe Cell Browser, an interactive visualization software that shows scATAC-seq peak profiles for scATAC-seq cell clusters, similar to the analysis done in this manuscript and described at https://support.10xgenomics.com/single-cellatac/software/visualization/latest/what-is-loupe-cell-browser.\n\nchromVAR\nWe measured global TF activity using chromVAR15. We used the cell-by-peaks and the Catalog of Inferred Sequence Binding Preferences (CIS-BP) motif (from chromVAR motifs “human_pwms_v1”) matches within these peaks from motifmatchr. We then computed the GC-bias-corrected deviation scores using the chromVAR “deviationScores” function."}
LitCovid-PD-CHEBI
{"project":"LitCovid-PD-CHEBI","denotations":[{"id":"T51166","span":{"begin":2402,"end":2404},"obj":"Chemical"},{"id":"T166","span":{"begin":2520,"end":2522},"obj":"Chemical"},{"id":"T84158","span":{"begin":2635,"end":2637},"obj":"Chemical"}],"attributes":[{"id":"A28638","pred":"chebi_id","subj":"T51166","obj":"http://purl.obolibrary.org/obo/CHEBI_74862"},{"id":"A60487","pred":"chebi_id","subj":"T166","obj":"http://purl.obolibrary.org/obo/CHEBI_29865"},{"id":"A2979","pred":"chebi_id","subj":"T84158","obj":"http://purl.obolibrary.org/obo/CHEBI_73890"}],"text":"scATAC-seq data processing\n\nscATAC-seq processing\nscATAC-seq reads were aligned to the GRCh38 (hg38) reference genome and quantified using CellRanger-ATAC count (10x Genomics, v.1.0.0).\n\nscATAC-seq quality control\nTo ensure that each cell was both adequately sequenced and had a high signal-to-background ratio, we filtered cells with less than 1,000 unique fragments and enrichment at TSSs \u003c 8. To calculate TSS enrichment \u003e 2, genome-wide Tn5-corrected insertions were aggregated ± 2,000 bp relative (TSS-strand-corrected) to each unique TSS. This profile was normalized to the mean accessibility ± 1,900–2,000 bp from the TSS, smoothed every 51 bp and the maximum smoothed value was reported as TSS enrichment in R. To construct a counts matrix for each cell by each feature (peaks), we read each fragment.tsv.gz fill into a GenomicRanges object. For each Tn5 insertion, which can be thought of as the “start” and “end” of the ATAC fragments, we used findOverlaps to find all overlaps with the feature by insertions. Then we added a column with the unique id (integer) cell barcode to the overlaps object and fed this into a sparseMatrix in R. To calculate the fraction of reads/insertions in peaks, we used the colSums of the sparseMatrix and divided it by the number of insertions for each cell id barcode using table in R.\n\nscATAC-seq visualization in genomic regions\nTo visualize scATAC-seq data, we read the fragments into a GenomicRanges object in R. We then computed sliding windows across each region we wanted to visualize for every 100 bp “slidingWindows (region, 100, 100)”. We computed a counts matrix for Tn5-corrected insertions as described above and then binarized this matrix. We then returned all non-zero indices (binarization) from the matrix (cell × 100-bp intervals) and plotted them in ggplot2 in R with “geom_tile”. For visualizing aggregate scATAC-seq data, the binarized matrix above was summed and normalized. Scale factors were computed by taking the binarized sum in the global peak set and normalizing to 10,000,000. Tracks were then plotted in Loupe Cell Browser, an interactive visualization software that shows scATAC-seq peak profiles for scATAC-seq cell clusters, similar to the analysis done in this manuscript and described at https://support.10xgenomics.com/single-cellatac/software/visualization/latest/what-is-loupe-cell-browser.\n\nchromVAR\nWe measured global TF activity using chromVAR15. We used the cell-by-peaks and the Catalog of Inferred Sequence Binding Preferences (CIS-BP) motif (from chromVAR motifs “human_pwms_v1”) matches within these peaks from motifmatchr. We then computed the GC-bias-corrected deviation scores using the chromVAR “deviationScores” function."}
LitCovid-PD-GO-BP
{"project":"LitCovid-PD-GO-BP","denotations":[{"id":"T309","span":{"begin":2402,"end":2404},"obj":"http://purl.obolibrary.org/obo/GO_0000981"}],"text":"scATAC-seq data processing\n\nscATAC-seq processing\nscATAC-seq reads were aligned to the GRCh38 (hg38) reference genome and quantified using CellRanger-ATAC count (10x Genomics, v.1.0.0).\n\nscATAC-seq quality control\nTo ensure that each cell was both adequately sequenced and had a high signal-to-background ratio, we filtered cells with less than 1,000 unique fragments and enrichment at TSSs \u003c 8. To calculate TSS enrichment \u003e 2, genome-wide Tn5-corrected insertions were aggregated ± 2,000 bp relative (TSS-strand-corrected) to each unique TSS. This profile was normalized to the mean accessibility ± 1,900–2,000 bp from the TSS, smoothed every 51 bp and the maximum smoothed value was reported as TSS enrichment in R. To construct a counts matrix for each cell by each feature (peaks), we read each fragment.tsv.gz fill into a GenomicRanges object. For each Tn5 insertion, which can be thought of as the “start” and “end” of the ATAC fragments, we used findOverlaps to find all overlaps with the feature by insertions. Then we added a column with the unique id (integer) cell barcode to the overlaps object and fed this into a sparseMatrix in R. To calculate the fraction of reads/insertions in peaks, we used the colSums of the sparseMatrix and divided it by the number of insertions for each cell id barcode using table in R.\n\nscATAC-seq visualization in genomic regions\nTo visualize scATAC-seq data, we read the fragments into a GenomicRanges object in R. We then computed sliding windows across each region we wanted to visualize for every 100 bp “slidingWindows (region, 100, 100)”. We computed a counts matrix for Tn5-corrected insertions as described above and then binarized this matrix. We then returned all non-zero indices (binarization) from the matrix (cell × 100-bp intervals) and plotted them in ggplot2 in R with “geom_tile”. For visualizing aggregate scATAC-seq data, the binarized matrix above was summed and normalized. Scale factors were computed by taking the binarized sum in the global peak set and normalizing to 10,000,000. Tracks were then plotted in Loupe Cell Browser, an interactive visualization software that shows scATAC-seq peak profiles for scATAC-seq cell clusters, similar to the analysis done in this manuscript and described at https://support.10xgenomics.com/single-cellatac/software/visualization/latest/what-is-loupe-cell-browser.\n\nchromVAR\nWe measured global TF activity using chromVAR15. We used the cell-by-peaks and the Catalog of Inferred Sequence Binding Preferences (CIS-BP) motif (from chromVAR motifs “human_pwms_v1”) matches within these peaks from motifmatchr. We then computed the GC-bias-corrected deviation scores using the chromVAR “deviationScores” function."}
LitCovid-sentences
{"project":"LitCovid-sentences","denotations":[{"id":"T428","span":{"begin":0,"end":26},"obj":"Sentence"},{"id":"T429","span":{"begin":28,"end":49},"obj":"Sentence"},{"id":"T430","span":{"begin":50,"end":185},"obj":"Sentence"},{"id":"T431","span":{"begin":187,"end":213},"obj":"Sentence"},{"id":"T432","span":{"begin":214,"end":395},"obj":"Sentence"},{"id":"T433","span":{"begin":396,"end":544},"obj":"Sentence"},{"id":"T434","span":{"begin":545,"end":718},"obj":"Sentence"},{"id":"T435","span":{"begin":719,"end":849},"obj":"Sentence"},{"id":"T436","span":{"begin":850,"end":1019},"obj":"Sentence"},{"id":"T437","span":{"begin":1020,"end":1146},"obj":"Sentence"},{"id":"T438","span":{"begin":1147,"end":1328},"obj":"Sentence"},{"id":"T439","span":{"begin":1330,"end":1373},"obj":"Sentence"},{"id":"T440","span":{"begin":1374,"end":1459},"obj":"Sentence"},{"id":"T441","span":{"begin":1460,"end":1588},"obj":"Sentence"},{"id":"T442","span":{"begin":1589,"end":1696},"obj":"Sentence"},{"id":"T443","span":{"begin":1697,"end":1842},"obj":"Sentence"},{"id":"T444","span":{"begin":1843,"end":1939},"obj":"Sentence"},{"id":"T445","span":{"begin":1940,"end":2049},"obj":"Sentence"},{"id":"T446","span":{"begin":2050,"end":2372},"obj":"Sentence"},{"id":"T447","span":{"begin":2374,"end":2382},"obj":"Sentence"},{"id":"T448","span":{"begin":2383,"end":2431},"obj":"Sentence"},{"id":"T449","span":{"begin":2432,"end":2613},"obj":"Sentence"},{"id":"T450","span":{"begin":2614,"end":2716},"obj":"Sentence"}],"namespaces":[{"prefix":"_base","uri":"http://pubannotation.org/ontology/tao.owl#"}],"text":"scATAC-seq data processing\n\nscATAC-seq processing\nscATAC-seq reads were aligned to the GRCh38 (hg38) reference genome and quantified using CellRanger-ATAC count (10x Genomics, v.1.0.0).\n\nscATAC-seq quality control\nTo ensure that each cell was both adequately sequenced and had a high signal-to-background ratio, we filtered cells with less than 1,000 unique fragments and enrichment at TSSs \u003c 8. To calculate TSS enrichment \u003e 2, genome-wide Tn5-corrected insertions were aggregated ± 2,000 bp relative (TSS-strand-corrected) to each unique TSS. This profile was normalized to the mean accessibility ± 1,900–2,000 bp from the TSS, smoothed every 51 bp and the maximum smoothed value was reported as TSS enrichment in R. To construct a counts matrix for each cell by each feature (peaks), we read each fragment.tsv.gz fill into a GenomicRanges object. For each Tn5 insertion, which can be thought of as the “start” and “end” of the ATAC fragments, we used findOverlaps to find all overlaps with the feature by insertions. Then we added a column with the unique id (integer) cell barcode to the overlaps object and fed this into a sparseMatrix in R. To calculate the fraction of reads/insertions in peaks, we used the colSums of the sparseMatrix and divided it by the number of insertions for each cell id barcode using table in R.\n\nscATAC-seq visualization in genomic regions\nTo visualize scATAC-seq data, we read the fragments into a GenomicRanges object in R. We then computed sliding windows across each region we wanted to visualize for every 100 bp “slidingWindows (region, 100, 100)”. We computed a counts matrix for Tn5-corrected insertions as described above and then binarized this matrix. We then returned all non-zero indices (binarization) from the matrix (cell × 100-bp intervals) and plotted them in ggplot2 in R with “geom_tile”. For visualizing aggregate scATAC-seq data, the binarized matrix above was summed and normalized. Scale factors were computed by taking the binarized sum in the global peak set and normalizing to 10,000,000. Tracks were then plotted in Loupe Cell Browser, an interactive visualization software that shows scATAC-seq peak profiles for scATAC-seq cell clusters, similar to the analysis done in this manuscript and described at https://support.10xgenomics.com/single-cellatac/software/visualization/latest/what-is-loupe-cell-browser.\n\nchromVAR\nWe measured global TF activity using chromVAR15. We used the cell-by-peaks and the Catalog of Inferred Sequence Binding Preferences (CIS-BP) motif (from chromVAR motifs “human_pwms_v1”) matches within these peaks from motifmatchr. We then computed the GC-bias-corrected deviation scores using the chromVAR “deviationScores” function."}
LitCovid-PD-GlycoEpitope
{"project":"LitCovid-PD-GlycoEpitope","denotations":[{"id":"T1","span":{"begin":441,"end":444},"obj":"GlycoEpitope"},{"id":"T2","span":{"begin":859,"end":862},"obj":"GlycoEpitope"},{"id":"T3","span":{"begin":1621,"end":1624},"obj":"GlycoEpitope"}],"attributes":[{"id":"A1","pred":"glyco_epitope_db_id","subj":"T1","obj":"http://www.glycoepitope.jp/epitopes/AN0083"},{"id":"A2","pred":"glyco_epitope_db_id","subj":"T2","obj":"http://www.glycoepitope.jp/epitopes/AN0083"},{"id":"A3","pred":"glyco_epitope_db_id","subj":"T3","obj":"http://www.glycoepitope.jp/epitopes/AN0083"}],"text":"scATAC-seq data processing\n\nscATAC-seq processing\nscATAC-seq reads were aligned to the GRCh38 (hg38) reference genome and quantified using CellRanger-ATAC count (10x Genomics, v.1.0.0).\n\nscATAC-seq quality control\nTo ensure that each cell was both adequately sequenced and had a high signal-to-background ratio, we filtered cells with less than 1,000 unique fragments and enrichment at TSSs \u003c 8. To calculate TSS enrichment \u003e 2, genome-wide Tn5-corrected insertions were aggregated ± 2,000 bp relative (TSS-strand-corrected) to each unique TSS. This profile was normalized to the mean accessibility ± 1,900–2,000 bp from the TSS, smoothed every 51 bp and the maximum smoothed value was reported as TSS enrichment in R. To construct a counts matrix for each cell by each feature (peaks), we read each fragment.tsv.gz fill into a GenomicRanges object. For each Tn5 insertion, which can be thought of as the “start” and “end” of the ATAC fragments, we used findOverlaps to find all overlaps with the feature by insertions. Then we added a column with the unique id (integer) cell barcode to the overlaps object and fed this into a sparseMatrix in R. To calculate the fraction of reads/insertions in peaks, we used the colSums of the sparseMatrix and divided it by the number of insertions for each cell id barcode using table in R.\n\nscATAC-seq visualization in genomic regions\nTo visualize scATAC-seq data, we read the fragments into a GenomicRanges object in R. We then computed sliding windows across each region we wanted to visualize for every 100 bp “slidingWindows (region, 100, 100)”. We computed a counts matrix for Tn5-corrected insertions as described above and then binarized this matrix. We then returned all non-zero indices (binarization) from the matrix (cell × 100-bp intervals) and plotted them in ggplot2 in R with “geom_tile”. For visualizing aggregate scATAC-seq data, the binarized matrix above was summed and normalized. Scale factors were computed by taking the binarized sum in the global peak set and normalizing to 10,000,000. Tracks were then plotted in Loupe Cell Browser, an interactive visualization software that shows scATAC-seq peak profiles for scATAC-seq cell clusters, similar to the analysis done in this manuscript and described at https://support.10xgenomics.com/single-cellatac/software/visualization/latest/what-is-loupe-cell-browser.\n\nchromVAR\nWe measured global TF activity using chromVAR15. We used the cell-by-peaks and the Catalog of Inferred Sequence Binding Preferences (CIS-BP) motif (from chromVAR motifs “human_pwms_v1”) matches within these peaks from motifmatchr. We then computed the GC-bias-corrected deviation scores using the chromVAR “deviationScores” function."}
LitCovid-PubTator
{"project":"LitCovid-PubTator","denotations":[{"id":"1505","span":{"begin":95,"end":99},"obj":"Gene"}],"attributes":[{"id":"A1505","pred":"tao:has_database_id","subj":"1505","obj":"Gene:8549"}],"namespaces":[{"prefix":"Tax","uri":"https://www.ncbi.nlm.nih.gov/taxonomy/"},{"prefix":"MESH","uri":"https://id.nlm.nih.gov/mesh/"},{"prefix":"Gene","uri":"https://www.ncbi.nlm.nih.gov/gene/"},{"prefix":"CVCL","uri":"https://web.expasy.org/cellosaurus/CVCL_"}],"text":"scATAC-seq data processing\n\nscATAC-seq processing\nscATAC-seq reads were aligned to the GRCh38 (hg38) reference genome and quantified using CellRanger-ATAC count (10x Genomics, v.1.0.0).\n\nscATAC-seq quality control\nTo ensure that each cell was both adequately sequenced and had a high signal-to-background ratio, we filtered cells with less than 1,000 unique fragments and enrichment at TSSs \u003c 8. To calculate TSS enrichment \u003e 2, genome-wide Tn5-corrected insertions were aggregated ± 2,000 bp relative (TSS-strand-corrected) to each unique TSS. This profile was normalized to the mean accessibility ± 1,900–2,000 bp from the TSS, smoothed every 51 bp and the maximum smoothed value was reported as TSS enrichment in R. To construct a counts matrix for each cell by each feature (peaks), we read each fragment.tsv.gz fill into a GenomicRanges object. For each Tn5 insertion, which can be thought of as the “start” and “end” of the ATAC fragments, we used findOverlaps to find all overlaps with the feature by insertions. Then we added a column with the unique id (integer) cell barcode to the overlaps object and fed this into a sparseMatrix in R. To calculate the fraction of reads/insertions in peaks, we used the colSums of the sparseMatrix and divided it by the number of insertions for each cell id barcode using table in R.\n\nscATAC-seq visualization in genomic regions\nTo visualize scATAC-seq data, we read the fragments into a GenomicRanges object in R. We then computed sliding windows across each region we wanted to visualize for every 100 bp “slidingWindows (region, 100, 100)”. We computed a counts matrix for Tn5-corrected insertions as described above and then binarized this matrix. We then returned all non-zero indices (binarization) from the matrix (cell × 100-bp intervals) and plotted them in ggplot2 in R with “geom_tile”. For visualizing aggregate scATAC-seq data, the binarized matrix above was summed and normalized. Scale factors were computed by taking the binarized sum in the global peak set and normalizing to 10,000,000. Tracks were then plotted in Loupe Cell Browser, an interactive visualization software that shows scATAC-seq peak profiles for scATAC-seq cell clusters, similar to the analysis done in this manuscript and described at https://support.10xgenomics.com/single-cellatac/software/visualization/latest/what-is-loupe-cell-browser.\n\nchromVAR\nWe measured global TF activity using chromVAR15. We used the cell-by-peaks and the Catalog of Inferred Sequence Binding Preferences (CIS-BP) motif (from chromVAR motifs “human_pwms_v1”) matches within these peaks from motifmatchr. We then computed the GC-bias-corrected deviation scores using the chromVAR “deviationScores” function."}