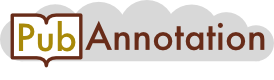
PMC:7417788 / 67017-67739
Annnotations
LitCovid-PMC-OGER-BB
To analyze the scRNA-seq data, we log normalized data (1 + counts per 10,000) with the ‘‘sc.pp.normalize_total’’ function before clustering, reduction and performing 2-dimensional t-SNE algorithm clustering with the first 50 principal components. This was done following PCA on top 5,000 most variable genes by using “sc.pp.highly_variable_genes” function in Scanpy with the default parameters. Dimensionality method and identification of significant clusters and was performed using Leiden clustering algorithm which uses a shared nearest neighbour modularity optimization-based clustering algorithm. Marker genes for each significant cluster were found using the function sc.tl.rank_genes_groups with default parameters.
LitCovid-sentences
To analyze the scRNA-seq data, we log normalized data (1 + counts per 10,000) with the ‘‘sc.pp.normalize_total’’ function before clustering, reduction and performing 2-dimensional t-SNE algorithm clustering with the first 50 principal components. This was done following PCA on top 5,000 most variable genes by using “sc.pp.highly_variable_genes” function in Scanpy with the default parameters. Dimensionality method and identification of significant clusters and was performed using Leiden clustering algorithm which uses a shared nearest neighbour modularity optimization-based clustering algorithm. Marker genes for each significant cluster were found using the function sc.tl.rank_genes_groups with default parameters.
LitCovid-PD-MONDO
To analyze the scRNA-seq data, we log normalized data (1 + counts per 10,000) with the ‘‘sc.pp.normalize_total’’ function before clustering, reduction and performing 2-dimensional t-SNE algorithm clustering with the first 50 principal components. This was done following PCA on top 5,000 most variable genes by using “sc.pp.highly_variable_genes” function in Scanpy with the default parameters. Dimensionality method and identification of significant clusters and was performed using Leiden clustering algorithm which uses a shared nearest neighbour modularity optimization-based clustering algorithm. Marker genes for each significant cluster were found using the function sc.tl.rank_genes_groups with default parameters.
LitCovid-PubTator
To analyze the scRNA-seq data, we log normalized data (1 + counts per 10,000) with the ‘‘sc.pp.normalize_total’’ function before clustering, reduction and performing 2-dimensional t-SNE algorithm clustering with the first 50 principal components. This was done following PCA on top 5,000 most variable genes by using “sc.pp.highly_variable_genes” function in Scanpy with the default parameters. Dimensionality method and identification of significant clusters and was performed using Leiden clustering algorithm which uses a shared nearest neighbour modularity optimization-based clustering algorithm. Marker genes for each significant cluster were found using the function sc.tl.rank_genes_groups with default parameters.
LitCovid-PD-CLO
To analyze the scRNA-seq data, we log normalized data (1 + counts per 10,000) with the ‘‘sc.pp.normalize_total’’ function before clustering, reduction and performing 2-dimensional t-SNE algorithm clustering with the first 50 principal components. This was done following PCA on top 5,000 most variable genes by using “sc.pp.highly_variable_genes” function in Scanpy with the default parameters. Dimensionality method and identification of significant clusters and was performed using Leiden clustering algorithm which uses a shared nearest neighbour modularity optimization-based clustering algorithm. Marker genes for each significant cluster were found using the function sc.tl.rank_genes_groups with default parameters.
LitCovid-PD-CHEBI
To analyze the scRNA-seq data, we log normalized data (1 + counts per 10,000) with the ‘‘sc.pp.normalize_total’’ function before clustering, reduction and performing 2-dimensional t-SNE algorithm clustering with the first 50 principal components. This was done following PCA on top 5,000 most variable genes by using “sc.pp.highly_variable_genes” function in Scanpy with the default parameters. Dimensionality method and identification of significant clusters and was performed using Leiden clustering algorithm which uses a shared nearest neighbour modularity optimization-based clustering algorithm. Marker genes for each significant cluster were found using the function sc.tl.rank_genes_groups with default parameters.