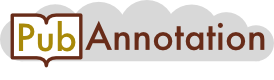
PMC:7402624 / 74109-75186
Annnotations
LitCovid-PubTator
{"project":"LitCovid-PubTator","denotations":[{"id":"2163","span":{"begin":61,"end":65},"obj":"Chemical"},{"id":"2169","span":{"begin":149,"end":157},"obj":"Species"},{"id":"2170","span":{"begin":415,"end":422},"obj":"Species"},{"id":"2171","span":{"begin":751,"end":755},"obj":"Chemical"},{"id":"2172","span":{"begin":140,"end":148},"obj":"Disease"},{"id":"2173","span":{"begin":406,"end":414},"obj":"Disease"}],"attributes":[{"id":"A2169","pred":"tao:has_database_id","subj":"2169","obj":"Tax:9606"},{"id":"A2170","pred":"tao:has_database_id","subj":"2170","obj":"Tax:9606"},{"id":"A2172","pred":"tao:has_database_id","subj":"2172","obj":"MESH:C000657245"},{"id":"A2173","pred":"tao:has_database_id","subj":"2173","obj":"MESH:C000657245"}],"namespaces":[{"prefix":"Tax","uri":"https://www.ncbi.nlm.nih.gov/taxonomy/"},{"prefix":"MESH","uri":"https://id.nlm.nih.gov/mesh/"},{"prefix":"Gene","uri":"https://www.ncbi.nlm.nih.gov/gene/"},{"prefix":"CVCL","uri":"https://web.expasy.org/cellosaurus/CVCL_"}],"text":"Visualizing variation of flow cytometric features across the UMAP embedding space\nA feature-weighted kernel density was computed across all COVID-19 patients, and was displayed as a contour plot (Fig. 6G and fig. S8, A to D). Whereas traditional kernel density methods apply the same base kernel function to every point to visualize point density, here the base kernel function centered at each individual COVID-19 patient sample was instead weighted (multiplied) by the Z-transform (mean-centered and standard deviation-scaled) of the log-transformed input feature prior to computing the overall kernel density. This weighting procedure facilitated visualization of the overall feature gradients (going from relatively low-to-high expression) across UMAP coordinates independent of the different range of each input feature. A radially symmetric two-dimensional Gaussian was used as the base kernel function with a variance parameter equal to one-half, which was tuned to be sufficiently broad in order to smooth out local discontinuities and best visualize feature gradients."}
LitCovid-PD-MONDO
{"project":"LitCovid-PD-MONDO","denotations":[{"id":"T438","span":{"begin":140,"end":148},"obj":"Disease"},{"id":"T439","span":{"begin":406,"end":414},"obj":"Disease"}],"attributes":[{"id":"A438","pred":"mondo_id","subj":"T438","obj":"http://purl.obolibrary.org/obo/MONDO_0100096"},{"id":"A439","pred":"mondo_id","subj":"T439","obj":"http://purl.obolibrary.org/obo/MONDO_0100096"}],"text":"Visualizing variation of flow cytometric features across the UMAP embedding space\nA feature-weighted kernel density was computed across all COVID-19 patients, and was displayed as a contour plot (Fig. 6G and fig. S8, A to D). Whereas traditional kernel density methods apply the same base kernel function to every point to visualize point density, here the base kernel function centered at each individual COVID-19 patient sample was instead weighted (multiplied) by the Z-transform (mean-centered and standard deviation-scaled) of the log-transformed input feature prior to computing the overall kernel density. This weighting procedure facilitated visualization of the overall feature gradients (going from relatively low-to-high expression) across UMAP coordinates independent of the different range of each input feature. A radially symmetric two-dimensional Gaussian was used as the base kernel function with a variance parameter equal to one-half, which was tuned to be sufficiently broad in order to smooth out local discontinuities and best visualize feature gradients."}
LitCovid-PD-CLO
{"project":"LitCovid-PD-CLO","denotations":[{"id":"T1053","span":{"begin":82,"end":83},"obj":"http://purl.obolibrary.org/obo/CLO_0001020"},{"id":"T1054","span":{"begin":180,"end":181},"obj":"http://purl.obolibrary.org/obo/CLO_0001020"},{"id":"T1055","span":{"begin":217,"end":218},"obj":"http://purl.obolibrary.org/obo/CLO_0001020"},{"id":"T1056","span":{"begin":826,"end":827},"obj":"http://purl.obolibrary.org/obo/CLO_0001020"},{"id":"T1057","span":{"begin":914,"end":915},"obj":"http://purl.obolibrary.org/obo/CLO_0001020"}],"text":"Visualizing variation of flow cytometric features across the UMAP embedding space\nA feature-weighted kernel density was computed across all COVID-19 patients, and was displayed as a contour plot (Fig. 6G and fig. S8, A to D). Whereas traditional kernel density methods apply the same base kernel function to every point to visualize point density, here the base kernel function centered at each individual COVID-19 patient sample was instead weighted (multiplied) by the Z-transform (mean-centered and standard deviation-scaled) of the log-transformed input feature prior to computing the overall kernel density. This weighting procedure facilitated visualization of the overall feature gradients (going from relatively low-to-high expression) across UMAP coordinates independent of the different range of each input feature. A radially symmetric two-dimensional Gaussian was used as the base kernel function with a variance parameter equal to one-half, which was tuned to be sufficiently broad in order to smooth out local discontinuities and best visualize feature gradients."}
LitCovid-PD-CHEBI
{"project":"LitCovid-PD-CHEBI","denotations":[{"id":"T50300","span":{"begin":213,"end":215},"obj":"Chemical"},{"id":"T64153","span":{"begin":284,"end":288},"obj":"Chemical"},{"id":"T67801","span":{"begin":357,"end":361},"obj":"Chemical"},{"id":"T2551","span":{"begin":888,"end":892},"obj":"Chemical"}],"attributes":[{"id":"A47010","pred":"chebi_id","subj":"T50300","obj":"http://purl.obolibrary.org/obo/CHEBI_29385"},{"id":"A95782","pred":"chebi_id","subj":"T64153","obj":"http://purl.obolibrary.org/obo/CHEBI_22695"},{"id":"A87264","pred":"chebi_id","subj":"T67801","obj":"http://purl.obolibrary.org/obo/CHEBI_22695"},{"id":"A26577","pred":"chebi_id","subj":"T2551","obj":"http://purl.obolibrary.org/obo/CHEBI_22695"}],"text":"Visualizing variation of flow cytometric features across the UMAP embedding space\nA feature-weighted kernel density was computed across all COVID-19 patients, and was displayed as a contour plot (Fig. 6G and fig. S8, A to D). Whereas traditional kernel density methods apply the same base kernel function to every point to visualize point density, here the base kernel function centered at each individual COVID-19 patient sample was instead weighted (multiplied) by the Z-transform (mean-centered and standard deviation-scaled) of the log-transformed input feature prior to computing the overall kernel density. This weighting procedure facilitated visualization of the overall feature gradients (going from relatively low-to-high expression) across UMAP coordinates independent of the different range of each input feature. A radially symmetric two-dimensional Gaussian was used as the base kernel function with a variance parameter equal to one-half, which was tuned to be sufficiently broad in order to smooth out local discontinuities and best visualize feature gradients."}
LitCovid-sentences
{"project":"LitCovid-sentences","denotations":[{"id":"T460","span":{"begin":0,"end":81},"obj":"Sentence"},{"id":"T461","span":{"begin":82,"end":225},"obj":"Sentence"},{"id":"T462","span":{"begin":226,"end":612},"obj":"Sentence"},{"id":"T463","span":{"begin":613,"end":825},"obj":"Sentence"},{"id":"T464","span":{"begin":826,"end":1077},"obj":"Sentence"}],"namespaces":[{"prefix":"_base","uri":"http://pubannotation.org/ontology/tao.owl#"}],"text":"Visualizing variation of flow cytometric features across the UMAP embedding space\nA feature-weighted kernel density was computed across all COVID-19 patients, and was displayed as a contour plot (Fig. 6G and fig. S8, A to D). Whereas traditional kernel density methods apply the same base kernel function to every point to visualize point density, here the base kernel function centered at each individual COVID-19 patient sample was instead weighted (multiplied) by the Z-transform (mean-centered and standard deviation-scaled) of the log-transformed input feature prior to computing the overall kernel density. This weighting procedure facilitated visualization of the overall feature gradients (going from relatively low-to-high expression) across UMAP coordinates independent of the different range of each input feature. A radially symmetric two-dimensional Gaussian was used as the base kernel function with a variance parameter equal to one-half, which was tuned to be sufficiently broad in order to smooth out local discontinuities and best visualize feature gradients."}